1. Introduction
Introduction: In the realm of machine learning, data annotation plays a crucial role in training models to recognize patterns and make accurate predictions. Data annotation involves labeling or tagging data to provide context and information to algorithms. This process helps algorithms learn from the labeled data and improve their accuracy over time. Outsourcing data annotation is a common practice where businesses or organizations rely on third-party service providers to annotate their data instead of handling it in-house.
Businesses that outsource data annotation assign this labor-intensive process to outside specialists who are skilled at effectively annotating big datasets. An internal annotation team would have required time and resources, which firms can save by outsourcing this process. Through outsourcing, businesses can benefit from the experience of seasoned annotators who are knowledgeable about a range of annotation tools and methodologies.
2. Pros of Outsourcing Data Annotation for Machine Learning
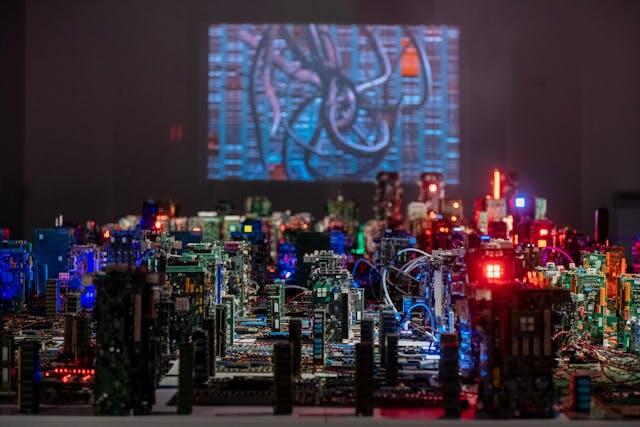
There are a number of significant benefits to outsourcing the machine learning process of data annotation. First off, it can greatly increase scalability and efficiency. Employing outside specialists in data annotation allows businesses to quickly and precisely identify massive amounts of data, which is essential for efficiently training machine learning models.
Another important advantage of outsourcing data annotation is cost-effectiveness. For this particular task, hiring and training an internal staff might be expensive and time-consuming. Businesses that outsource can pay for just the services they require, saving money on expenses related to staffing a full-time annotation crew.
Through outsourcing, one can obtain specialized knowledge and resources that might not be available internally. In order to guarantee high-quality annotations, data annotation service providers frequently use cutting-edge tools and techniques and have a wealth of expertise working with different types of data. In the end, this knowledge may result in ML models that are more accurate and better overall outcomes.
3. Cons of Outsourcing Data Annotation for Machine Learning
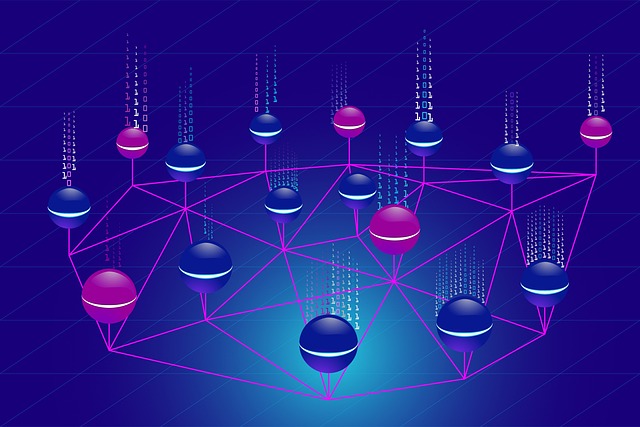
There are disadvantages to outsourcing data annotation for machine learning, and security and privacy issues are at the top of the list. There is always a chance that private information will be compromised or leaked when entrusting sensitive data to outside parties. It is imperative that you thoroughly inspect and have faith in the outsourcing partner to protect the security and integrity of the data that is being annotated.
Collaborating with external annotators frequently presents communication obstacles. Annotation instructions or comments may not be transferred efficiently due to misinterpretations, delays, or language problems. Misunderstandings, incorrect annotations, and general project delays may result from this. When outsourcing data annotation procedures, it is crucial to have clear communication channels and protocols in place to minimize these problems.
Another drawback of outsourcing data annotation for machine learning is quality control. It can be difficult to maintain uniform annotation quality across several annotators. Different skill levels or interpretations might lead to variations in annotation accuracy and adherence to norms. For machine learning initiatives, the accuracy and dependability of annotated data that is outsourced must be guaranteed by putting strong quality control systems in place and conducting frequent audits.
4. Factors to Consider Before Outsourcing Data Annotation
There are a few important things to think about before choosing to outsource your data annotation process for machine learning applications. The significance of data security measures should come first. It is essential to be sure that your data annotation partner has strong security measures in place to safeguard your confidential data. This covers access controls, encryption techniques, and adherence to laws governing data protection such as HIPAA and GDPR.
The necessity of having open lines of communication with annotators is another important component. Setting up effective channels of communication is crucial for communicating project needs, answering queries and concerns quickly, and giving input on annotations. Throughout the annotation process, accuracy and consistency can be maintained with the support of feedback systems and clear instructions.
Techniques for guaranteeing the quality of your annotations are essential to the performance of your machine learning models. Annotated data sets can be made far more accurate and reliable by putting strict quality control procedures into place, such as double-annotating samples, doing routine audits, and giving annotators ongoing training. Regular observation and feedback systems are also essential for identifying and resolving problems early in the annotation process.
5. Case Studies: Successful Outsourcing Examples in Data Annotation
Many actual businesses have used outsourcing to their advantage when it comes to data annotation. Spare5, a platform that links companies with remote laborers for a range of jobs, including data annotation, is one such instance. Spare5 was able to extend its operations fast and affordably by outsourcing their annotation needs to a distributed workforce, which improved the accuracy of their machine learning models.
Scale AI, a business that specializes in offering premium training data for AI applications, is another noteworthy case study. Scale AI has forged strategic alliances with a number of companies to effectively oversee their data labeling procedures. Scale AI provides accurate annotations at scale by fusing human intelligence with cutting-edge technology, freeing up businesses to concentrate on creating reliable machine learning solutions.
These instances of effective outsourcing demonstrate how crucial communication and strategic preparation are when working with outside vendors to annotate data. Businesses that choose dependable partners carefully, set clear expectations, and keep lines of communication open are more likely to have success with their machine learning initiatives.
6. Best Practices for Outsourcing Data Annotation Processes
To guarantee the quality and accuracy of the annotated data, it is essential to adhere to best practices when outsourcing data annotation operations for machine learning. First and foremost, it's critical to provide explicit expectations and guidelines. Give clear directions on the criteria to be used, the annotated data formatting to be done, and any labeling rules that need to be followed.
Second, preserving the integrity of the annotated data requires the implementation of quality control procedures. This can involve establishing feedback loops for adjustments and enhancements, as well as random checks of annotations for correctness and uniformity between annotators.
Last but not least, it's critical to stay in constant contact with your annotation partners. Maintaining an open channel of communication makes it easier to resolve conflicts or misunderstandings quickly, guarantees agreement on project objectives, and develops cooperative working relationships, all of which improve the caliber of the annotated data. Organizations can improve the efficacy and efficiency of their outsourced data annotation procedures for machine learning projects by adhering to these best practices.
7. Ethical Considerations in Data Annotation Outsourcing
It is imperative to tackle any biases in annotated data when using external data annotation services for machine learning. The effectiveness and dependability of the ML algorithm can be impacted by biases in the training data, which can result in distorted model predictions. It's critical to establish quality checks, give annotators clear standards, and include a variety of viewpoints in the annotation process in order to reduce this risk.
In ethical data annotation outsourcing, it is critical to provide equitable treatment and remuneration for annotators. Even though they contribute significantly to the creation of excellent training datasets, annotators frequently receive little recognition and compensation. Annotated data sets for machine learning projects can be improved overall and firms can uphold ethical standards by delivering fair compensation, clear instructions, and a supportive work atmosphere.
8. Future Trends in Data Annotation Outsourcing
Two patterns come to light when we consider how machine learning data annotation operations will be outsourced in the future. First, data labeling activities are being revolutionized by ongoing automation improvements. Automation technologies are accelerating the rate at which enormous datasets may be reliably labeled, decreasing manual workloads, and optimizing the data annotation process. This trend improves the scalability of data annotation activities in addition to increasing efficiency.
The incorporation of AI technology to further enhance data annotation accuracy is the second noteworthy trend that is likely to emerge. Through the application of artificial intelligence algorithms, companies can achieve improved accuracy and consistency in the labeling of intricate datasets. Artificial intelligence (AI) tools can help human annotators by automatically or suggestively classifying some types of data, producing annotated datasets of higher quality that are suitable for training machine learning models.
These upcoming patterns point to a positive development in the field of externalized data annotation for machine learning initiatives. Companies that use automation and AI integration in their data labeling operations should see improvements in efficiency, accuracy, and productivity. In the upcoming years, keep an eye out for how these developments continue to influence the outsourcing environment for data annotation.
9. Conclusion
To sum up everything I've written so far, there are a number of benefits and drawbacks to outsourcing data annotation procedures for machine learning. Positively, outsourcing can scale annotation tasks effectively, save money, give access to qualified annotators, and save time. However, there are drawbacks as well, including worries about data security, possible problems with quality, difficulties with communication, and reliance on outside providers. In order to make an informed choice that is in line with their business objectives and requirements, businesses must carefully consider these variables in relation to their unique needs and resources before opting to outsource data annotation operations.