1. Introduction
Product managers need to be adept at properly interpreting data in the data-driven business environment of today. Analyzing and interpreting data is necessary to find important patterns and insights. This ability is crucial for product managers because it allows them to comprehend consumer behavior, spot areas for improvement, and make well-informed judgments.
Product managers need guidance from data interpretation to create strategies and products that work. Product managers can receive a thorough grasp of their target market and market expectations by evaluating data from a variety of sources, including user behavior indicators, market trends, and customer feedback. They can now make data-driven decisions that support corporate objectives and spur product innovation thanks to this understanding.
Product managers can find patterns, correlations, and anomalies in datasets by having a solid understanding of data interpretation. They may efficiently optimize product features, pricing tactics, and marketing campaigns by utilizing this information. Product managers can now correctly assess the impact of their projects and modify their plans in response to real-time insights thanks to data interpretation.
After reviewing the material above, we can say that product managers who want to succeed in the cutthroat industry of today must have a firm grasp of data interpretation. Product managers may use data to improve decision-making, encourage innovation, and provide solutions that align with the needs and preferences of customers by developing this skill set.
2. The Role of Data in Product Management
When it comes to product management, data is essential for determining strategic choices. Product managers use data to understand consumer behavior, industry trends, and the effectiveness of their offerings. Product managers may make well-informed judgments that propel product development and improve user experience by proficiently analyzing data. Product managers can use data as a compass to steer them toward innovative and profitable ideas.
Data influences product management decision-making by offering useful information that results in better-informed decisions. Product managers can make decisions about features, pricing, marketing tactics, and other aspects of their business by using data analysis to find patterns, trends, and opportunities. They can gauge the performance of new product launches, better understand consumer preferences, and streamline the entire product roadmap with the use of data. Product managers can reduce risks and increase the likelihood of developing successful solutions that appeal to their target market by utilizing data-driven insights.
Key choices throughout the product development lifecycle are frequently guided by data insights, which are the driving force behind successful products. By using data to tailor suggestions for customers, companies like Netflix have changed the entertainment sector and increased user engagement and retention. Another excellent illustration of how data analytics generates revenue is Amazon's recommendation engine, which makes product recommendations based on users' browsing interests and histories. Through the analysis of user behavior data, market trends, and competitor information, product managers can identify areas for innovation and effectively offer solutions that address changing customer needs.
In summary, it is imperative to comprehend the significance of data in product administration to enable well-informed decision-making that fosters innovation and prosperity. Product managers may improve the overall performance of their products and define their plans by leveraging the power of data analytics. Promising goods frequently stem from a thorough comprehension of data insights that help businesses maintain an advantage in a cutthroat industry. To design solutions that have an impact on customers and propel business success, product managers must become experts at interpreting data.
3. Understanding Key Data Concepts
Product managers must comprehend fundamental data ideas in order to make wise judgments. Key performance indicators (KPIs), return on investment (ROI), and attrition rate are examples of common data terminology that are essential to product management. A product's churn rate indicates client retention, ROI establishes its financial sustainability, and KPIs aid in measuring its success. These ideas offer crucial insights that influence the tactics used in product development.
To properly optimize the processes involved in product development, product managers must understand these data concepts. They can pinpoint opportunities for development and establish quantifiable objectives for their goods by examining KPIs. Prioritizing features that generate the greatest returns and effectively allocating resources are made easier with an understanding of ROI. Product managers can take proactive measures to address problems and improve customer happiness by keeping an eye on the churn rate, which will ultimately result in better goods.
After putting everything above together, we can say that having a solid understanding of important data principles enables product managers to lead successful product development projects by utilizing insightful knowledge obtained from correctly analyzing data.
4. Leveraging Data for Product Improvement
Product managers that want to improve their products successfully must leverage data for product improvement. Product managers can make wise decisions about new product development and enhancements by employing data analytics to obtain insightful knowledge about customer behaviors, preferences, and pain points. Product managers utilize A/B testing, which compares many iterations of a product with actual consumers to see which works better based on predetermined metrics, as one important technique to leverage data.
Product managers can utilize data to spot patterns and trends that point to areas where their products need to be improved. Analyzing user feedback and behavior data, for example, can reveal common problems or bottlenecks in a product that require attention. Product managers are able to give priority to additions or upgrades that will have the biggest effects on customer retention and happiness by using this proactive strategy.
Case studies that demonstrate how data is used effectively to improve products can provide insightful information on how successful businesses have used data analytics to spur innovation and expansion. Netflix is one such instance, which tailors content curation and suggestions to each individual user based on a vast amount of viewing data. Netflix continuously improves its algorithms to recommend material that is catered to individual preferences by examining viewing patterns, ratings, and other behavioral data. This increases user pleasure and engagement.
Spotify's utilization of data to improve their music recommendation algorithm is another interesting case study. Spotify suggests music to users based on their listening history, preferred genres, and interactions with playlists. This helps to keep users on the platform longer. In addition to enhancing customer experience, this data-driven strategy has helped the organization get more subscribers. 🤏
The aforementioned information leads us to the conclusion that product managers are empowered to make well-informed decisions that spur innovation and improve the customer experience when they use data to better their products. Product managers may take actionable insights from raw data and turn them into significant changes in their products by employing a variety of analytical tools and approaches. In today's competitive market scenario, case studies such as Netflix and Spotify show the real benefits of incorporating data-driven approaches into product development processes, which in turn lead to more successful outcomes and long-term growth.
5. Avoiding Common Pitfalls in Data Interpretation
Product managers must be careful when interpreting data to avoid frequent mistakes because they depend on data-driven insights to guide their actions. Deciphering huge datasets can be difficult and sometimes result in errors or the omission of important information. Product managers have to be careful when navigating the complexities of data interpretation to prevent biases, misunderstandings, or poor decisions.
We may address these issues by putting procedures in place that improve the precision and efficiency of data interpretation. To make sure that the focus stays on pertinent indicators in line with company objectives, one strategy is to clearly define objectives and questions before beginning data analysis. Using visual aids like charts or graphs can make complicated data sets easier to understand and make it easier to spot patterns or trends.
Working together with specialists or data analysts can yield insightful discussions and a variety of viewpoints on how to understand data. Asking cross-functional teams for their opinions can help provide a variety of perspectives and reduce the possibility of missing something crucial. Product managers may avoid typical mistakes and improve decision-making processes based on precise and trustworthy insights by promoting a culture of continuous learning and improvement in data interpretation techniques.
6. Implementing Data Interpretation Tools and Techniques
Product managers must use tools and procedures for data interpretation in order to make well-informed judgments. There are several tools accessible, from simple spreadsheet programs like Excel to more complex platforms like Tableau or Power BI. These tools provide data visualization, which facilitates the identification of trends and patterns. Predictive modeling and the analysis of large, complicated data sets can be aided by statistical tools such as R or Python.
Process integration of these tools into product management calls for meticulous preparation and implementation. The first step for product managers should be to determine which critical metrics for their products they must monitor and evaluate. Product managers may guarantee that the insights acquired from data analysis are applicable and actionable by coordinating it with business objectives. To guarantee data accuracy and dependability, a well-defined procedure for data collection, cleaning, and analysis must be in place.
One of the best practices for integrating data interpretation tools is to provide team members with frequent training sessions to improve their proficiency with these tools. Working together with scientists or data analysts can also yield insightful information on deciphering complicated datasets. Sharing insights between various teams within the organization can be facilitated by producing dashboards or reports that highlight important discoveries.
Product managers can use data-driven insights to enhance decision-making procedures and propel product success by putting these tools for data interpretation into practice and adhering to integration best practices.
7. Importance of Continuous Learning and Adaptation
Product managers must always be learning and adapting in order to succeed in the fast-paced digital environment. Product managers must keep up with data trends in order to make wise judgments and stay one step ahead of the competition. Product managers can strengthen their abilities, decision-making procedures, and team innovation by investing in continuing education in data interpretation.
Career advancement is one of the main advantages of ongoing education in data interpretation for product managers. People with the most recent knowledge and abilities typically perform better than their colleagues when industries change and new technologies are developed. Product managers are better positioned for growth within their firms and show a commitment to self-improvement when they actively seek out opportunities to improve their data interpretation talents.
Product managers may spot patterns, glean insightful information, and formulate smart recommendations that propel business performance by being aware of data trends. Product managers can increase their knowledge of data analytics tools and techniques and improve their ability to use data in decision-making by actively participating in continuing education.
Product managers can differentiate themselves in today's data-driven business climate by having the valuable skill of interpreting and analyzing data. In addition to keeping product managers abreast of industry best practices, continuous learning encourages innovation and growth within their companies. Product managers can establish themselves as indispensable resources capable of promoting success and bringing about positive change in their teams by placing a high priority on continuing education in data interpretation.
8. Collaborating with Data Experts
Product managers can reap numerous benefits from collaborating with data specialists. Product managers can leverage the skills of data scientists and analysts to obtain deeper insights and make better decisions by collaborating closely with them. Product managers can successfully utilize the insights gained from complicated datasets by using data professionals' skills and experience to uncover significant patterns.
Product managers and data specialists working together can significantly improve the caliber of insights obtained from data. Product managers can benefit from the distinct viewpoint that data scientists and analysts provide by identifying opportunities and trends that could have gone missed otherwise. Product managers can make sure that their choices are well-informed and supported by solid data-driven evidence by working together on this project.
In summary, improved end-user goods and services can result from the collaboration of product managers and data specialists, as well as more precise forecasts and improved strategic planning. Through tight collaboration, these experts may use one other's proficiencies to optimize data extraction value and provide significant commercial results.
9. Incorporating Data Interpretation into Product Roadmaps
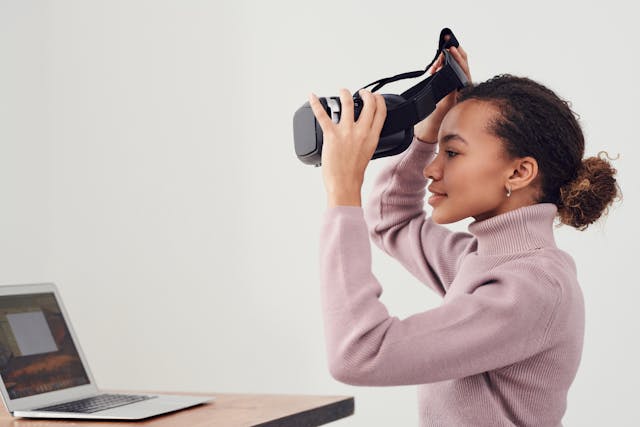
Product managers who want to be successful must include data interpretation in their roadmaps. PMs guarantee that decisions are based on insights rather than conjecture by incorporating data processes into development schedules. A clear road forward is made possible by the effective alignment of data-driven strategies with corporate objectives, which enables products to effectively address real market needs. Products can evolve dynamically depending on real-time feedback and analytics when data interpretation is included into the roadmap. This enables quick adjustments that promote long-term value creation.
Achieving impactful product roadmaps requires ensuring congruence between data-driven initiatives and overarching company objectives. Through constant data analysis and interpretation, Product Managers are able to make well-informed decisions that align with the overall objectives of the firm. This integration promotes an environment where results and metrics are valued above all else, which results in more accurate product planning and execution. Products are positioned for success when data interpretation is firmly ingrained in the roadmap process because they are still intimately linked to the business's strategic direction.
10. Measuring Success through Data Metrics
Product managers must use data metrics to measure success in order to comprehend how well their goods are selling. Establishing key performance indicators (KPIs) makes it easier to monitor and evaluate if a product is accomplishing its objectives. Product managers may make sure their team is working toward particular goals and staying on track with the broader product plan by establishing explicit KPIs.
Sustaining continuous improvement requires regular monitoring and assessment of performance metrics. Product managers can spot areas that need attention and make well-informed decisions based on facts rather than conjecture by looking at data patterns. They are able to efficiently iterate on their products and make the required changes to improve user experience and spur business growth because to their data-driven strategy. Product managers can make strategic decisions that have a favorable impact on their products and the success of their firm as a whole by studying data interpretation.
11. Case Studies: Real-world Applications of Data Interpretation
Aspiring product managers should thoroughly examine case studies that demonstrate how data interpretation is applied in practical settings. Future product managers may learn a great deal by looking at successful products that owe their success to data interpretation techniques. These case studies provide an approachable and concrete means of comprehending how data-driven choices have influenced product success stories. Examining these cases not only offers motivation but also gives product managers the means to handle comparable obstacles in their own professions.
Aspiring product managers can see firsthand how data interpretation has helped to spot market trends, comprehend consumer needs, and guide strategic decision-making through case studies. These tales, which feature both established businesses growing their offerings and startups upending entire industries, provide compelling examples of how data-driven methods affect both product creation and business expansion. Future product managers can gain valuable insights and best practices that they can use in their careers by thoroughly examining each case study.
These case examples highlight how crucial it is to gain a thorough understanding of various data sources, analytical tools, and techniques in order to perform correct data interpretation. Prospective product managers can gain valuable knowledge from the accomplishments and shortcomings of their peers when it comes to utilizing statistical analysis, A/B testing, user behavior tracking, and other methods to extract significant insights from intricate datasets. Through absorbing these stories of innovation propelled by painstaking data analysis, aspiring product managers may augment their capacity to make well-informed choices and propel significant results for their goods.
In order to understand how data interpretation shapes successful products, prospective product managers should study case studies. These real-world examples provide a road map for efficiently using data to drive business growth, user experience enhancements, and product planning. Future product managers can improve their analytical talents, extend their capacity for strategic thought, and eventually guide their products toward higher success in the cutthroat market of today by extracting the most important lessons from these case studies.
12. Conclusion: The Future of Data Interpretation in Product Management
In summary, the field of data interpretation in product management appears to have a bright yet demanding future. Product managers will need to gain a deeper understanding of data analysis as technology develops in order to extract insightful conclusions that spur innovation. Accurate and efficient data interpretation will be essential for negotiating the competitive environment and satisfying client requests.
The dynamic function of data in creating inventive products emphasizes how crucial it is for product managers to always be learning and adapting. In a market that is changing quickly, it will be crucial to adopt new tools and methods for interpreting data in order to remain competitive. Product managers may spot emerging trends, make well-informed decisions, and provide value to organizations and consumers by leveraging data.
Understanding data interpretation is becoming more than simply a useful skill in today's dynamic corporate environment; it is essential for success. Product managers that place a high priority on honing their talents in this area will not only be better able to make decisions, but they will also be able to take advantage of new prospects for professional development.