1. Introduction
Working with data has become more important than ever in the current digital era. Data is essential to current corporate operations because it provides insights and influences decision-making in a variety of industries. Professionals with strong data-related abilities are in more demand as businesses look to use data to achieve a competitive advantage. The need for data specialists is greater than ever as companies embrace digital transformation and look to leverage their data. This blog article examines the realities of handling data in a variety of professions and highlights the potential and difficulties that arise.
2. The Basics of Working with Data
In order to gain insightful knowledge, working with data entails gathering, evaluating, and interpreting information. Qualitative and quantitative data can be either organized or unstructured. While unstructured data is more complicated and necessitates sophisticated processing techniques, structured data is easier to examine and neatly fits into predetermined categories. While quantitative data deals with numbers and measurements and offers statistical insights, qualitative data identifies qualities or attributes and provides depth and context.
Data can take many different forms, including customer reviews, sales numbers, demographics, and social media activities. Every kind of data has a distinct function in guiding decision-making procedures. Businesses may successfully adjust their analytics strategy by knowing the significance of various data kinds. Organizations can obtain detailed insights into many facets of their operations and customer behavior by utilizing a variety of data sources.
Working with data helps organizations to improve processes, innovate products and services, and make well-informed decisions based on facts rather than gut feeling. This is true whether working with organized sales records or unstructured social media sentiments. It enables businesses to remain competitive in the data-driven world of today by revealing insightful patterns and trends that spur innovation and expansion.🔷
3. Challenges Faced in Working with Data
Handling data has a unique set of difficulties. The most common problem is making sure the data is of high quality. Data that is erroneous, lacking, or inconsistent can seriously affect how decisions are made and produce unsatisfactory results. In order to preserve the accuracy and dependability of their data, organizations frequently battle with cleansing and validating it.
Working with data presents a huge problem due to privacy concerns. Businesses must manage complex legal requirements to appropriately protect customer information in light of growing legislation like the CCPA and GDPR. A careful balance between innovation and protecting sensitive data is needed to ensure compliance while using data for analysis and insights.
Another challenge for data professionals is organizing and evaluating massive datasets. The sheer amount of data produced nowadays might overwhelm legacy systems, causing processing delays and performance bottlenecks. To effectively extract valuable insights from large datasets, it becomes essential to implement storage, retrieval, and processing procedures that are efficient.
Working with data presents more than just technical difficulties; it also raises ethical issues. As a result of the massive collection and analysis of personal data by organizations, concerns about accountability, transparency, and consent are raised. Throughout its existence, data must be handled responsibly to uphold stakeholder and customer confidence.
4. Tools and Technologies for Working with Data
A number of tools and technologies stand out as being crucial for experts in the field of data analysis. Python is a popular tool among scientists and data analysts because to its ease of use and adaptability. Its robust libraries, including NumPy, Pandas, and Scikit-learn, increase the efficiency of operations like data cleansing, analysis, and machine learning.😽
Another well-known programming language designed especially for statistical computing and graphics is called R. Deep statistical work in academia and industry frequently uses R due to its wide array of tools for data manipulation and analysis, as well as its sophisticated visualization capabilities.
Structured Query Language, or SQL, is essential for communicating with databases. SQL queries enable retrieval, manipulation, and management of data in relational databases. Considering how common relational databases are in many different sectors, it is essential.
As one of the best tools for data visualization, Tableau excels at converting unprocessed data into visually clear representations like interactive dashboards and charts. Without the need for in-depth programming experience, users may generate intelligent reports from complicated datasets thanks to its intuitive interface.
Professionals use technologies such as Python, R, SQL, and Tableau to leverage data and find patterns, insights, and trends that are essential for making well-informed decisions. These solutions' smooth incorporation into workflows simplifies procedures and reveals the full potential that lies behind huge datasets. The convergence of technology and analytics not only boosts productivity but also gives firms the ability to make informed strategic choices.😀
5. Impact of Working with Data in Business
Data has emerged as a crucial resource in today's business environment for organizations trying to obtain a competitive edge. Businesses may improve their entire operations, make well-informed decisions, and create strong plans by utilizing data efficiently. Organizations can gain a better understanding of internal operations, customer preferences, and market trends by utilizing data-driven insights.
Businesses like Netflix and Amazon are good examples of how to use data to your advantage. Netflix enhances customer happiness and retention rates by using data analytics to personalize suggestions for its users. Similar to this, Amazon uses massive data analysis to forecast customer demand, improve supply chain management, and provide customized shopping experiences with features like product recommendations and targeted ads. 🥸
Integrating data-driven approaches into business operations not only enhances decision-making but also drives innovation and agility in an increasingly competitive environment.
6. Ethical Considerations in Data Work
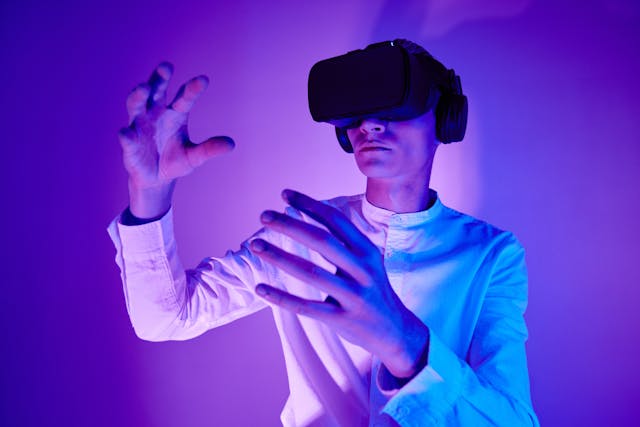
When it comes to handling data, ethical issues are quite important. There are important ethical considerations to take into account when it comes to the gathering, use, and storage of data. To guarantee that people's rights and privacy are maintained, data handling must be done with the utmost care and honesty.
When managing sensitive data, privacy and security must always come first. Establishing strong security measures is crucial for organizations in order to protect data from breaches and unwanted access. In addition to being required by law, following privacy laws and regulations shows an organization's dedication to moral business conduct.
Setting ethics first in data work does more than just comply with regulations; it also develops industry integrity and stakeholder trust. Organizations can effectively manage data responsibly while safeguarding individual rights and promoting openness by adhering to ethical norms in data practices.
7. The Future of Working with Data
Working with data promises to change significantly in the future as machine learning and artificial intelligence continue to revolutionize data science and analytics. The application of augmented analytics, where AI algorithms will automate data preparation, insight discovery, and even provide explanations for discoveries, is one significant trend that is anticipated to gain traction. With this change, non-technical people will be able to interact with data more effectively and make data-driven decisions without needing to possess sophisticated analytical abilities.
We should expect a sharp increase in the use of edge computing to process massive amounts of data produced by Internet of Things devices in real time. Decentralized processing will speed up decision-making and lower latency in vital applications including smart cities, healthcare monitoring systems, and autonomous cars. With the inclusion of techniques like federated learning, which enable training on decentralized data sources without compromising privacy or security, machine learning models are expected to grow more complex and accurate.
The growing worries about privacy, bias, and transparency also point to a rising emphasis on ethical considerations in data utilization in the years to come. Regulations and procedures that guarantee equity, responsibility, and openness in the gathering, use, and sharing of data will be increasingly necessary as AI technology influence decisions in a wider range of businesses. Encouraging ethical conduct will be essential to preserving regulators' and customers' trust.
With ongoing developments in artificial intelligence and machine learning, the field of data science is poised for tremendous innovation and expansion. Organizations may discover new insights from their data assets to create better business outcomes while addressing changing societal concerns about privacy and ethics by keeping up with developing trends and appropriately utilizing cutting-edge technologies.
8. Data Visualization Techniques
A clear and efficient means of communicating insights is dependent on effective data visualization. Data visualization can aid in the simplification of complex information, facilitating stakeholders' understanding of important lessons and decision-making. The effective communication of sales trends, customer behavior patterns, or financial performance measures can be greatly impacted by the selection of an appropriate visualization technique.
For professionals handling data, tools like Tableau and Power BI have become essential. With the use of these platforms' extensive visualization capabilities, users may produce interactive graphs, charts, dashboards, and reports. With the help of these tools, analysts may successfully identify outliers, uncover trends, and tell a story with raw data transformed into visually captivating graphics.
The overall effect of data analysis presentations can be improved by selecting the right visualization technique based on the audience and particular dataset. Every sort of visualization—from heat maps and treemaps to bar charts and scatter plots—has a distinct function in emphasizing certain facets of the data. Knowing whether to use a histogram over a bubble chart or a line graph over a pie chart is crucial to producing visualizations that engage users and help them make better decisions.
9. Overcoming Data Bias
For any dataset to be analyzed with accuracy and dependability, data bias must be addressed and overcome. Gathering a more representative dataset by diversifying sources is one efficient way to reduce bias. A more inclusive and wide-ranging viewpoint can be achieved by incorporating demographic, geographic, and other pertinent aspects.
Regularly checking for any biases during the data collection process is another crucial strategy. Being aware of potential biases from the start makes it possible to make corrections early on and avoid biased conclusions later on. The accuracy of the insights obtained can be further improved by putting into practice reliable algorithms that can identify and fix biases in real-time during data processing.
Examples of biased data leading to incorrect conclusions are common in many different fields. Biased datasets have led to faulty identification in the field of facial recognition technology, for instance, with grave repercussions, especially for underprivileged groups that are disproportionately mistaken. Similar to this, discriminatory practices like racial profiling have been sustained by skewed training datasets used in predictive policing algorithms.
By highlighting these examples, we may both urgently and poignantly remind people of the practical consequences of data bias and the need for proactive actions to address it. Through transparent techniques, varied representation, and ongoing evaluation processes, we can proactively reduce bias in datasets and open the door to more dependable and equitable data-driven decision-making.
10. Continuous Learning in Data Work
To succeed in the ever-changing world of data work, continuous learning is not just a requirement, but also a choice. With the regular introduction of new tools, approaches, and methodologies, the area of data science is always changing. Maintaining your relevance demands a dedication to lifelong learning and skill development. Fortunately, those who want to improve their abilities in data analysis and interpretation have a wealth of tools at their disposal.💽
Online courses provide a practical and adaptable approach to pick up new skills and keep up with data science trends. There are numerous courses available on platforms like Coursera, Udemy, and edX, spanning from basic to expert levels. Machine learning, data visualization, statistical analysis, and other subjects are covered in these courses. Obtaining certifications from respectable organizations is another way to prove your expertise in particular data work domains.
Data professionals may make sure they stay valued and competitive in the job market by devoting time and energy to ongoing learning. In this rapidly evolving business, continuous learning is essential for job advancement, whether it involves learning a new programming language or exploring sophisticated predictive analytics approaches. Remain inquisitive, remain knowledgeable, and accept lifelong learning as an essential component of your data-working experience.
11. Collaborative Approaches to Working with Data
When dealing with data, collaborative approaches not only improve results but also encourage efficiency and innovation. When data professionals collaborate, a variety of knowledge can be combined, producing deeper insights and solutions that could go unnoticed by someone working alone. Organizational growth is fueled by knowledge sharing within teams and between departments, which cultivates a culture of learning and continual improvement.
Create clear communication routes in order to support efficient collaboration between departments or within a data team. All team members are kept informed about project goals and status through regular meetings, progress reports, and clear documentation. Stressing the value of open communication promotes idea sharing and helpful criticism, which strengthens the bonds among coworkers.
Cross-training team members can enhance their skill sets and foster a deeper comprehension of many facets of data analysis. People can improve their technical skills by learning from each other's talents and pooling their experience through seminars or knowledge-sharing events. This improves the team's overall performance and fosters a sense of unity and support for one another.
By enabling real-time project collaboration regardless of physical location, the integration of collaborative tools and platforms further optimizes teamwork. Making use of communication tools, project management software, or shared documents facilitates workflow integration and guarantees that all parties have access to the most recent information. Data teams can overcome obstacles like time zones and distance by using technology to improve cooperation and work effectively toward shared objectives.
12. Conclusion
We may infer from everything mentioned above that dealing with data has its benefits and drawbacks. It calls for a wide range of abilities, including creativity, critical thinking, and technical proficiency. Data scientists help firms make strategic decisions by navigating complicated datasets and gleaning insights. There is a talent scarcity in the data area, which presents a wealth of opportunities for individuals who are eager to further their skills despite the growing demand for data expertise.
Working with data actually requires constant learning and adjusting to new instruments and technology. To thrive in this fast-paced environment, data professionals need develop a curious mentality, enjoy problem-solving, and effectively interact across teams. Those that are enthusiastic about data can make significant contributions and influence the direction of business as industries use big data and analytics to innovate and optimize operations.
I urge anybody who are interested in data to learn more about the opportunities available in this fascinating subject. There are several ways to increase one's proficiency in data analytics, including through in-depth training courses, networking opportunities, and practical projects. A rewarding career with lots of opportunities for advancement and impact can be secured by aspirant data professionals by keeping up with industry changes and routinely improving their abilities. For individuals who are motivated to use the power of information to change the world, venturing into the world of data is a worthwhile adventure that is both difficult and incredibly rewarding.