1. Introduction
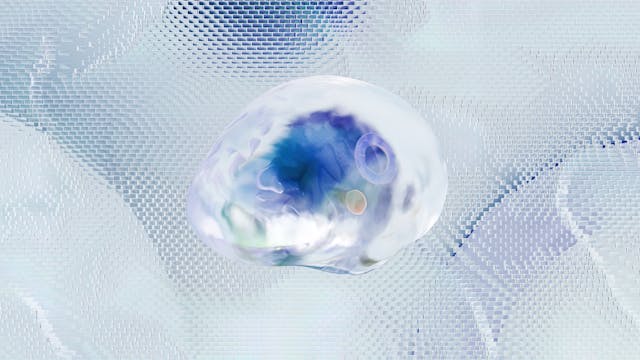
Organizations extensively rely on data to make decisions in today's data-driven society. But problems with data quality are a regular problem that many encounter. These problems are faults in datasets that can have a big influence on decision-making processes. These errors include incompleteness, inconsistency, and other mistakes. It is impossible to overestimate the significance of data quality; reliable and useful insights can only be obtained from data of a high caliber. Inadequate data quality can result in faulty projections, analyses, and poor business consequences. Thus, every business looking to properly exploit its data must address problems with data quality.
2. Common misconceptions about data quality
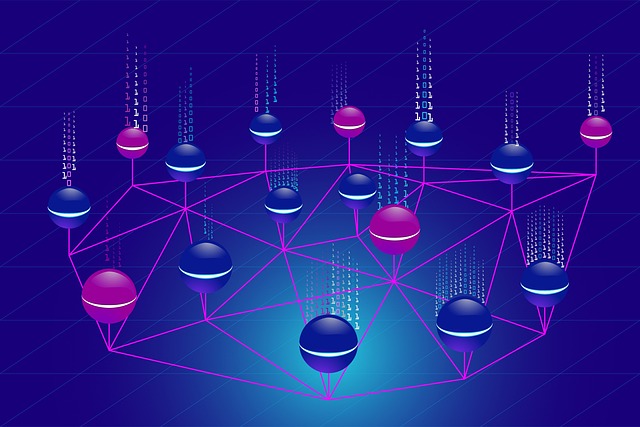
Misconceptions prevalent in the public domain regarding data quality can impede an organization's capacity to fully utilize its data. A common misconception is that data quality only refers to correctness. Although correctness is important, data quality is more than just correctness. It also entails making sure the data is accurate, timely, consistent, relevant, and unique.
Another widespread misperception is that the IT department is the only entity responsible for data quality. Assuring high-quality data is actually a shared duty among all members of the organization. Every person contributes to the preservation and enhancement of data quality, from frontline employees inputting data to management making choices based on data insights. To attain strong data quality procedures, departmental cooperation, standardization, and continuous training are crucial.
By dispelling these myths and encouraging a comprehensive view of data quality, companies can create a culture that recognizes accurate and trustworthy data as a vital resource for facilitating well-informed decision-making and realizing commercial success.
3. Hidden data quality issues in everyday processes
In many different businesses, routine operations frequently contain hidden data quality problems that have a subtle but noticeable impact on company outcomes. Inaccurate prescription information or patient data in the healthcare industry can result in incorrect diagnoses or treatments, endangering the health and safety of patients. Retail companies that depend on faulty product data may encounter inventory inconsistencies, which can lead to overstock or stockout scenarios that affect sales and consumer satisfaction. In a similar vein, financial organizations that depend on inaccurate or out-of-date client data run the risk of noncompliance or incorrect credit assessment determinations.
The outcomes of corporate operations may be significantly impacted by these concealed problems with data quality. In the marketing sector, utilizing erroneous customer data for targeting campaigns can result in resource waste on futile outreach initiatives and the loss of important chances for interaction and customisation. Inaccurate product information in supply chain management can result in delivery schedule delays, which can aggravate customers and possibly cost the company future business. The financial stability and growth prospects of the organization might be compromised by making poor strategic decisions based on inaccurate financial data utilized for reporting or forecasting.
Bad data quality has an impact that goes beyond operational inefficiencies; it also erodes confidence in organizational procedures and decision-making ability. Through the proactive identification and resolution of these covert data quality concerns in routine operations, companies may protect their brand, enhance productivity, and seize fresh chances for expansion and innovation.
4. The cost of ignoring data quality issues
Ignoring data quality issues can have severe consequences, ranging from financial implications to damaging reputational risks and customer impact.
Financially speaking, inaccurate data can result in poor decision-making that costs money by squandering resources and passing up chances. The cost of inaccurate data may mount up rapidly, whether it's from investing in unsuccessful marketing campaigns because of bad customer information or from making poor strategic decisions because of erroneous sales figures.
Neglecting issues related to the quality of data can also be very dangerous to one's reputation. Customers want businesses to handle their information honestly and securely in today's data-driven society. In addition to undermining trust, data breaches and disinformation brought on by poor data quality can result in significant fines and legal ramifications under laws like GDPR.
Additionally, the influence on customers cannot be understated. Customer unhappiness and possible churn might arise from targeted marketing gone wrong due to inaccurate data. The consequences could be disastrous in terms of reputational damage and even legal repercussions if consumer data is compromised as a result of subpar quality control.
Based on everything mentioned above, we can say that dealing with data quality concerns is an essential part of any successful business strategy, not merely something to do on a best-practice basis. Data quality assurance can be prioritized by enterprises as a basic pillar of their operations, once they realize the true cost of neglecting these concerns, which ranges from financial losses to brand damage and consumer alienation.
5. Factors contributing to data quality issues
Issues with data quality are widespread in many companies and may result from a variety of circumstances. The issues at hand are largely caused by human error and the dependence on manual procedures. Errors that occur during data processing or entry might generate inaccuracies that spread throughout the system. Data quality is further deteriorated by manual inputs, which raise the possibility of inconsistencies and duplications.
A crucial element contributing to problems with data quality is the lack of strong data governance and uniform procedures in a company. Discrepancies and ambiguities are likely to arise in the absence of explicit norms on the collection, storage, and maintenance of data. Enforcing defined standards and establishing appropriate data governance frameworks can greatly improve the overall quality of data assets, facilitating more dependable decision-making processes.
Organizations looking to raise the bar for data quality must address these factors. Businesses can improve the accuracy and integrity of their data repositories by automating processes that would otherwise result in human mistake and enforcing strict data governance guidelines. To make sure that their data continues to be a valued asset rather than a burden, businesses must identify these underlying problems and take proactive steps.
6. Tools and methodologies for improving data quality
Using data profiling and cleansing processes is crucial to improving the quality of the data. Through an analysis of the structure, completeness, and accuracy of the data, data profiling aids in identifying the qualities and features of the data. Through this process, abnormalities, duplicates, and inconsistent data in the dataset are found and fixed. To increase overall data integrity, cleaning procedures include error correction, redundancy removal, and format consistency.
Adding automated validations and checks is just another essential component of enhancing data quality. Continuous monitoring of incoming data for mistakes or inconsistencies is made possible by automated checks. Organizations can quickly discover problems and take corrective action by implementing automated procedures and validation standards. In addition to ensuring high-quality data, this proactive strategy saves time and money by eliminating the need for human error identification and rectification.
7. Strategies for preventing future data quality issues
In order to avoid future problems with data quality, it is imperative to establish a culture of data stewardship. Organizations may guarantee that all staff members understand the value of preserving high-quality data by fostering a sense of accountability and ownership over the data. This entails developing precise policies and processes for managing data, endorsing educational initiatives to improve data literacy among teams, and designating specific data stewards to supervise efforts to improve data quality.
The key to preserving data quality over time is to implement procedures for ongoing monitoring and improvement. Early problem detection can be achieved by putting in place routine inspections and audits to find flaws or discrepancies in data. Organizations can take proactive measures to address any potential abnormalities by implementing automated systems to analyze data quality parameters. By creating feedback loops where users may identify inconsistencies or offer enhancements, it is ensured that data quality stays at the forefront of everyone's concerns.
Organizations can prevent potential issues with data quality before they become more serious by putting in place continuous monitoring and improvement methods and emphasizing a culture of data stewardship. These tactics support an attitude of continuous improvement and accountability when it comes to managing important information, in addition to aiding in the preservation of the integrity of organizational data.
8. Case studies of successful data quality initiatives
An important example of a successful data quality effort case study is a global retail company. This business put in place a thorough data governance program that created distinct accountability for data accuracy, enhanced data quality procedures, and standardized data definitions. They improved the consistency and dependability of their information across departments by combining several databases and simplifying their data management methods.
In a different case, a top financial institution drove its data quality improvements by concentrating on the correctness of customer information. They created automated systems to routinely validate and clean consumer data, greatly lowering the number of inaccuracies in their records. This proactive approach ensured correct communication and individualized services based on trustworthy data, which improved regulatory compliance while also improving client happiness.
Through the implementation of a strong master data management system, a healthcare institution revitalized its efforts to ensure data quality. They reduced duplication mistakes, enhanced data integrity, and increased operational efficiency by establishing a consolidated patient information repository that synchronized with many applications throughout the organization. By providing precise medical histories, this program not only improved patient care but also streamlined the billing process by using consistent and trustworthy financial data.
9. The role of emerging technologies in addressing data quality challenges
The issues that companies confront today with regard to data quality are largely addressed by emerging technology. Businesses may uncover discrepancies, anticipate possible errors, and improve data validation procedures more quickly and effectively than with traditional techniques by utilizing tools like automation, machine learning, and artificial intelligence. These technologies allow for proactive steps to be made to preserve high-quality data standards by enabling real-time monitoring of data quality metrics.
Through the use of cutting-edge technologies like blockchain, data transactions may be made more transparent and secure while maintaining the accuracy and integrity of data across systems. Employing sophisticated algorithms and analytics, businesses can find trends in their datasets that might point to underlying quality problems. By taking a proactive stance, data inaccuracies are prevented before they become more serious issues that could influence decision-making procedures.
It is possible to continuously and instantly collect data in real time from multiple sources when Internet of Things (IoT) devices are integrated. Maintaining the quality of the data will be a difficulty as well as an opportunity given this inflow of data. However, by utilizing the cognitive computing and advanced analytics made possible by these technologies, enterprises can guarantee that incoming data satisfies predetermined quality criteria while also extracting important insights. Businesses may effectively overcome obstacles related to data quality and adjust to the changing information management landscape by embracing emerging technology.
10. Data privacy and security considerations in maintaining high data quality
Ensuring data security and privacy is essential to preserving excellent data quality. Maintaining data integrity requires making sure that private information is shielded against intrusions and illegal access. If data quality problems are not appropriately addressed, they may result in security threats and privacy concerns. Strong protocols must be put in place by organizations to protect data while preserving its accuracy and dependability. Businesses may improve the overall quality of their data and successfully reduce possible dangers by giving privacy and security considerations top priority in their data management procedures. 🫎
11. Conclusion: Prioritizing data quality for long-term success
In conclusion, companies who want to succeed in the long run must prioritize the quality of their data. Companies may make sure their decisions are based on accurate and trustworthy information by proactively addressing data quality issues. Investing in procedures, training, and equipment to uphold high standards for data quality is an ongoing investment in the competitiveness and overall well-being of the company, rather than being a one-time cost.
Ensuring that this data is of excellent quality is therefore essential in today's data-driven environment, where choices are increasingly dependent on analytics and insights gained from massive volumes of data. Inadequate data quality can cause a wide range of challenges, from distorted analytics to problems with regulatory compliance, all of which can have an effect on the bottom line. To protect the integrity of their data assets, organizations must thereby instill a culture of data stewardship and accountability at all levels.
Because data and systems are dynamic, attaining ideal data quality may be an unattainable objective, but constant improvement is essential. Data discrepancies should be quickly identified and corrected through the establishment of regular audits, monitoring systems, and feedback loops. sustaining high-quality data is important, and sustaining coordination amongst IT teams, data analysts, business users, and other stakeholders can help everyone recognize that.
To sum up what I mentioned above, the first step in properly tackling these concerns is realizing that most firms have more problems with data quality than you may have thought. Adopting a proactive strategy to improve and maintain data quality will reduce risks and open up new avenues for growth and innovation. In today's dynamic business environment, putting a deliberate effort into enhancing data quality is in line with the larger objective of developing a high-performing and future-ready firm.
12. Call to action: Steps to assess and address your organization's data quality gaps
To ensure data quality in your organization, follow these steps to assess and address any existing gaps:
1. **Audit Your Data**: Start by performing a comprehensive audit of your data sources, gathering techniques, and storage infrastructure. Determine the sources of the data, the methods used to collect it, and the locations of its storage within your company.
2. **Define Metrics**: To gauge how well your data management procedures are working, set up key performance indicators (KPIs) for data quality. This will assist you in establishing standards for growth.😐
3. **Implement Data Governance**: To control how data is gathered, kept, accessed, and used within your company, establish strong data governance policies and processes. Verify adherence to laws like the CCPA and GDPR.
4. **Invest in Quality Tools**: To aid automate the process of finding and fixing mistakes in your datasets, think about making an investment in data quality tools. Simple data validation checks to sophisticated machine learning algorithms are examples of these technologies.
5. **Develop Your Group**: Make sure your team members receive enough training on keeping high-quality data and the best strategies for doing so. Give students the tools to comprehend the value of precise data and how it affects the processes involved in making decisions.
6. **Regular Monitoring**: Establish routines for monitoring your data to keep tabs on its quality over time. Conduct evaluations and reviews on a regular basis to make sure that data quality requirements are being followed.
7. **Create Data Cleaning Procedures** : Create procedures for routinely cleaning and standardizing data. This can involve fixing mistakes, eliminating duplicate entries, and guaranteeing consistency between different datasets.
By carefully following these procedures, you may detect and resolve any data quality concerns that may already exist within your company, which will result in more trustworthy insights and generally better-informed decision-making processes.