1. Introduction
Introduction: A Data Scientist is a professional who specializes in analyzing and interpreting complex digital data, often using machine learning and predictive analytics to derive insights. They possess a unique skill set that combines expertise in statistics, programming, domain knowledge, and critical thinking. Understanding the DNA of a Data Scientist is crucial in appreciating their role in today's data-driven world.
Comprehending the varied competencies that Data Scientists bring to the table is crucial to knowing their "DNA." Understanding the complexities of their skill set allows one to recognize the multidisciplinary nature of their work and the value they provide to companies in a variety of sectors. The ability to properly harness the power of data is becoming more and more dependent on data, therefore understanding what makes a data scientist effective is essential.
2. Essential Skills and Knowledge
The ability to combine a variety of technical skills is essential for success as a data scientist. Being proficient in programming languages such as R and Python is essential for handling and analyzing data. Data interpretation is made possible by a solid understanding of statistical principles, and predictive model construction is made possible by a mastery of machine learning methods. The foundation of a data scientist's arsenal are these technical abilities, which enable them to glean insightful information from intricate databases.
To properly leverage data, subject expertise is just as important as technological proficiency. Knowing the industry or profession in which one works gives analysis context and makes it easier to spot pertinent patterns and trends in the data. Domain expertise enables data scientists to ask the proper questions and produce recommendations that are actionable and in line with company goals, whether the field is marketing, finance, or healthcare.
Exceptional data scientists possess critical thinking and problem-solving abilities, which are essential characteristics. The qualities of a skilled practitioner include approaching problems analytically, breaking down difficult issues into digestible parts, and coming up with creative solutions. Data scientists need to be meticulous, skilled at debugging problems, and resilient when faced with setbacks in their analytical pursuits.
A competent data scientist is essentially made up of a combination of technical know-how, subject knowledge, critical thinking skills, and problem-solving ability. Aspiring data scientists can confidently and skillfully traverse the ever-changing world of big data analytics by developing five fundamental elements.
3. Educational Background
Academic backgrounds in disciplines like computer science, mathematics, and statistics are common among data scientists. A solid grounding in these fields gives them the know-how and abilities needed to efficiently evaluate large, complicated data sets. While some data scientists may join the industry with a bachelor's degree, many hold additional degrees in related fields, such as a master's or doctorate.
Professional growth and ongoing education are essential to a successful career as a data scientist. In data science, new methods, instruments, and technological advancements are continually being made. To maintain their competitiveness in the labor market and provide their clients or employers with high-caliber work, data scientists need to stay up to date on these advancements.
Data scientists frequently participate in continuing education through online courses, workshops, conferences, or professional certifications to keep their skills up to date. Data scientists can improve their knowledge base, elevate their level of expertise, and adjust to the ever-changing demands of the industry by devoting time and resources to ongoing learning. In the ever-evolving profession of data science, this dedication to lifelong learning is crucial for being relevant and optimizing employment options. 🤭
4. Tools and Technologies
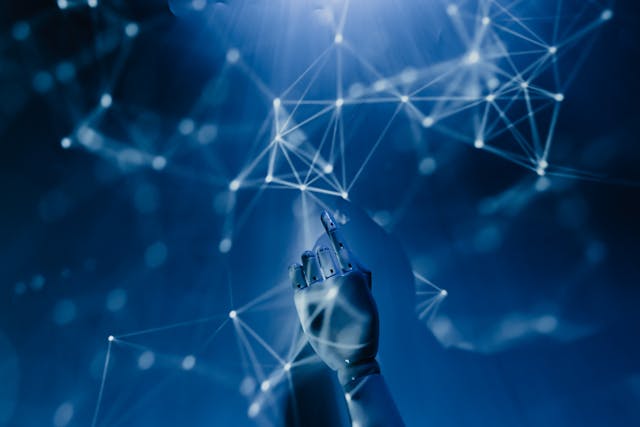
Common data science tools like Python, R, and SQL are essential for data analysis and manipulation. For every data scientist, Python's adaptability, R's statistical capabilities, and SQL's ability to query databases make them indispensable. Professionals may effectively extract significant insights from complex information with the help of these technologies.
Big data and artificial intelligence (AI) are two emerging technologies that have completely changed the data science profession. AI enables sophisticated task automation and predictive modeling, while big data technologies manage enormous amounts of heterogeneous data sets. By utilizing these tools, data scientists can address more challenging issues and get more profound understanding from their research. If one wants to succeed in the ever-changing field of contemporary data analysis, it is imperative that prospective data scientists keep up with these developments.
5. Communication Skills
The foundation of any successful data science endeavor is effective communication. In addition to their technical knowledge, data scientists need to be able to clearly and succinctly explain complicated findings to stakeholders in a variety of organizational positions. Data scientists can make sure that their work has a noticeable influence on company choices and strategy by clearly communicating the insights and recommendations obtained from data analysis.
Since data science initiatives frequently involve specialists from multiple departments, including marketing, finance, and operations, collaboration with cross-functional teams is vital. Data scientists must be able to collaborate well with team members who may have varied specializations and backgrounds. Effective communication facilitates more seamless collaboration and alignment towards shared objectives by bridging the gap between technical language and corporate objectives.
Based on the aforementioned, we may infer that data scientists must develop their communication skills in order to fully realize the value of their analyses and make a significant contribution to the accomplishment of data-driven projects inside their organizations. In today's increasingly data-driven environment, data scientists may lead the way for innovation and success by promoting collaboration across diverse functions through effective communication.
6. Ethical Considerations in Data Science
The field of data science is constantly changing, and ethical considerations are important. In addition to gathering and evaluating enormous volumes of data, data scientists are also responsible for making sure that this process is carried out responsibly. The repercussions of gathering and analyzing data can be profound, affecting both people and society at large.
Preserving data security and privacy is a fundamental ethical consideration in data science. Data scientists handle sensitive data, therefore it's critical to have safeguards in place to prevent misuse or unauthorized access. Maintaining data secrecy serves to establish confidence with stakeholders whose information is being used, in addition to adhering to ethical standards.👋
In their job, data scientists must negotiate difficult ethical conundrums pertaining to responsibility, openness, and permission. Professionals may help ensure that data science is handled more responsibly and with credibility by taking proactive measures to solve these challenges. In order to promote innovation in data science while maintaining integrity and respecting people's right to privacy, ethical considerations must be given top priority.
7. Industry Applications
Data scientists are essential to many different businesses because they use their knowledge to mine data for insightful information. Data scientists examine medical imaging and patient records in the healthcare industry to enhance diagnosis and treatment strategies. They also focus on improving hospital operations and forecasting illness outbreaks.
Data scientists utilize predictive modeling in the banking industry to evaluate risks, identify fraud, and make investment decisions. They create plans for improved financial results by analyzing consumer behavior and market trends. Data scientists assist financial firms in providing clients with services that are tailored to their needs and interests.
Data scientists optimize pricing tactics and offer individualized product recommendations to improve the consumer experience in e-commerce. By analyzing consumer data, they may better understand consumer preferences and buying habits, which helps with inventory management and marketing initiatives. To guarantee safe transactions for clients, data scientists in e-commerce also focus on fraud detection and prevention.
8. Career Path and Growth Opportunities
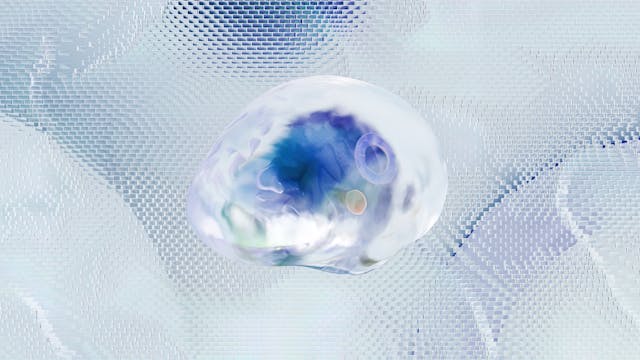
Professionals in the ever-evolving field of data science have a wide range of job options. To ensure efficient data flow inside an organization, data engineers concentrate on building and maintaining data pipelines. Data analysts are adept in deciphering and displaying data to draw insightful conclusions. The creation of effective predictive analytics models and algorithms is the area of expertise for machine learning engineers.
A data science job requires constant upskilling and keeping up with the newest trends and technologies. Acquiring advanced degrees or certifications can improve one's proficiency in particular fields, such as big data or deep learning. Participating in practical initiatives, working together with colleagues, and networking within the field can potentially lead to new chances.
Seeking mentorship from seasoned experts might offer insightful advice for job advancement. Climbing the ladder in the dynamic field of data science requires embracing difficulties, reevaluating skills and knowledge gaps on a regular basis, and setting clear goals. Remember that learning and adapting on the fly is essential for success in this dynamic business.
9. Challenges and Future Trends
A major problem in data science nowadays is dealing with biased algorithms. Algorithms can become biased for a variety of reasons, including biased training data, erroneous assumptions, or even unintentional human intervention during the development process. This prejudice may result in unfair outcomes, discrimination, and unequal treatment for particular groups. In addition to creating accurate models, data scientists are increasingly expected to guarantee that these models are impartial and fair.
In the future, data scientists' roles will continue to change in reaction to new trends and technology. The growing significance of accountability and interpretability in algorithmic decision-making is one important trend influencing the direction of data science in the future. The increasing use of algorithms in vital domains such as healthcare, banking, and criminal justice has led to a growing call for transparency about the decision-making process involved.🥧
The incorporation of machine learning and artificial intelligence into a variety of businesses is another significant trend. Working with increasingly sophisticated models and technologies will need Data scientists to adjust while still attending to ethical issues like security and privacy. Data scientists will have to overcome the difficulty of effectively managing bigger and more complicated datasets as data sources continue to grow rapidly due to the introduction of IoT devices and big data.
Data scientists must address problems like algorithmic bias while keeping up with major developments like interpretability, AI integration, and large-scale data management. Through skillfully managing these obstacles and sensibly accepting forthcoming changes, data scientists might sustainably propel innovation and generate constructive social effects via their endeavors.
10. Diversity and Inclusion in Data Science
Due to the diverse viewpoints, experiences, and ideas that diversity brings to the table, it is essential for fostering innovation in data analysis. A diversified workforce, comprising workers from different industries, backgrounds, and cultures, is highly advantageous for data science. Organizations may access a greater pool of skills and creativity by creating an inclusive atmosphere where all opinions are valued and heard, which will result in more complete and strong data-driven solutions.
In the data science field, inclusivity is crucial to fostering a friendly environment where everyone feels appreciated and encouraged to share their own talents and perspectives. Adopting an inclusive mindset not only advances justice and equity but also improves teamwork and problem-solving skills. The inclusion of different voices in decision-making processes and project development enhances the likelihood of relevant, ethical, and broadly influential outcomes.
Through the prioritization of diversity and inclusion in data science processes, organizations may cultivate a dynamic ecosystem that stimulates creativity, innovation, and constructive industry change. Accepting diverse viewpoints improves data analysis quality and helps create a more resilient and dynamic workforce that can handle difficult problems with empathy and agility. Representation is only one aspect of inclusion; another is fostering an environment where all individuals are valued, encouraged, and equipped to thrive in the data science industry.
11. Case Studies
The foundation of a data scientist's work are case studies, which provide insightful information about what makes a project successful. Through the examination of completed data science projects, experts might find unnoticed trends and tactics that contributed to their success. In addition to demonstrating effective practices, these real-world examples encourage aspiring data scientists to further their expertise.
The lessons gleaned from previous data science disasters are just as significant. Any learning process will inevitably involve failure, and in the field of data science, every setback is an opportunity for improvement. Through the analysis of failed projects and the understanding of the mistakes made along the way, data scientists can adjust their strategy, steer clear of typical traps, and come out stronger and more equipped to handle new problems.
Data scientists may expand their knowledge base, hone their analytical abilities, and eventually improve their capacity to confidently and resiliently negotiate the complexity of this dynamic profession by combining the study of successful cases with the acceptance of lessons from mistakes.
12. Conclusion
An analytical approach, topic expertise, and technical proficiency are all ingrained in a data scientist. Data scientists are experts in data visualization techniques, have a solid understanding of statistics, and are fluent in programming languages such as Python and R. Their natural curiosity pushes them to investigate and draw conclusions from intricate datasets.
Professionals in the field of data science need to keep up with the rapid evolution of the industry by improving their current abilities and embracing new technologies and approaches. In such a changing environment, staying current with industry trends and relevant requires ongoing learning. Success in this industry requires a dedication to lifelong learning, whether it is through the mastery of sophisticated machine learning algorithms or a knowledge of the ethical implications of data utilization.
Taking into account everything said above, we can say that a data scientist's DNA includes not only technical skills but also a strong desire to solve problems, astute analytical thinking, good communication talents, and a constant quest for personal growth. A data scientist's job description is dynamic and changes as technology and business requirements do. Therefore, success in this fascinating and dynamic sector depends on being up to date with developing trends through constant learning.