1. Introduction
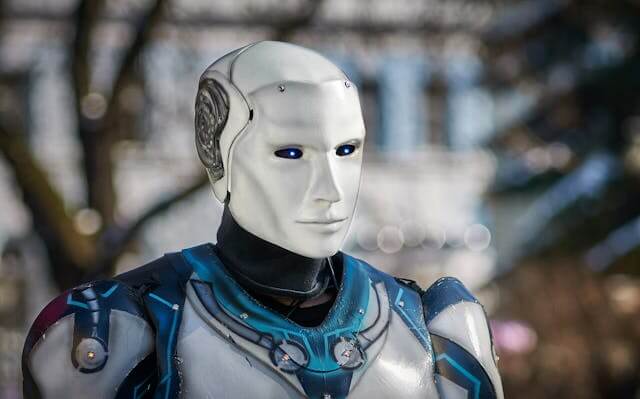
Introduction: No-code data science is a revolutionary approach that empowers individuals to perform complex data analysis without the need for traditional programming skills. This paradigm shift in the field of data science is fueled by the rapid development of user-friendly tools and platforms that democratize data analysis and machine learning. As organizations increasingly recognize the value of data-driven decision-making, the demand for no-code solutions continues to grow.
The advent of no-code technologies is steadily dismantling the conventional hurdles to entrance in data science, such as coding requirements and technical competence. Without creating a single line of code, these platforms allow users with a variety of backgrounds, such as subject matter experts, analysts, and business professionals, to take advantage of advanced analytics approaches. No-code data science creates new opportunities for innovation and industry collaboration by streamlining the data exploration, modeling, and deployment processes.🎛
2. The Emergence of No-Code Data Science
The approaches used in the field of data science have significantly evolved in recent years. In the past, data science required sophisticated programming and coding abilities to handle, examine, and draw conclusions from enormous databases. But as no-code platforms and technologies proliferate, the way data science is used is changing fundamentally.
No-code platforms have become quite effective tools that allow people to undertake complex data analysis tasks even without a lot of programming experience. To simplify the data science workflow, these platforms frequently make use of drag-and-drop interfaces, pre-built algorithms, and automated procedures. Thus, without having to be fluent in coding languages like Python or R, people from a variety of disciplines, including business, marketing, and healthcare, may now take advantage of the advantages of data science.
The use of no-code platforms in data science has made complex analytics capabilities more accessible to a wider audience. These technologies enable more people to use data for problem-solving and decision-making by removing the requirement for specialist coding knowledge. In addition to quickening the rate of innovation, this democratization promotes a more welcoming and cooperative atmosphere where people of all technical backgrounds may collaborate on data-driven projects.
It is evident that the rise of no-code platforms is a turning point in the development of data science as we look to the future of this profession. These tools are transforming how businesses approach data-driven decision-making and opening the door for a new era of creativity and discovery in data science by making advanced analytics more approachable and user-friendly.✉️
3. Advantages of No-Code Data Science
Significant benefits come with no-code data science, particularly in terms of accessibility for non-technical users. It is no longer necessary to have substantial coding knowledge to interact with and analyze data; people from different fields may do so with ease. The democratization of data science encourages firms to approach problem-solving and decision-making from a more inclusive perspective. Technical obstacles no longer prevent non-technical individuals from utilizing strong data tools to extract important insights.
The quicker prototyping and deployment times associated with no-code data science are another important benefit. Write sophisticated scripts and spend a lot of time troubleshooting them using traditional coding methods. On the other hand, no-code platforms offer user-friendly interfaces that simplify the procedure, enabling users to quickly test theories and effectively implement models. Quicker iterations are made possible by this experimentation agility, which helps teams quickly adjust to changing requirements and increase overall productivity.
Based on everything mentioned above, we can say that the emergence of no-code data science represents a paradigm change in analytics toward democratization and efficiency. Organizations may open up new avenues for innovation and expansion in the data-driven future by simplifying the prototyping and deployment processes and enabling non-technical users to utilize sophisticated data tools.
4. Implications for Data Scientists
Data scientists' primary responsibilities are changing from coding to more strategic duties in a no-code world. Data scientists can concentrate on high-impact tasks like evaluating results, offering insights, and directing decision-making based on data analysis by automating repetitive operations. This change enables them to considerably contribute to corporate goals and make better use of their analytical skills.
Data scientists have the chance to advance their knowledge in other areas, such problem-solving, communication, and domain expertise, as data science technologies become more accessible and user-friendly. They can work more closely with stakeholders from various departments to better understand particular business needs and customize solutions. With these additional competencies, data scientists can expand their influence inside their companies and take on roles that go beyond typical data analysis duties.
For those working in data science, the shift towards a no-code methodology is an exciting moment. Through opportunities for upskilling, data scientists may refocus their attention on strategic objectives and expand their positions to become crucial partners in leveraging data-driven insights to drive business success.
5. Industries Embracing No-Code Data Science
Because no-code data science tools are so easily accessible and effective, many sectors are adopting them at a rapid pace. One such industry is healthcare, where experts without in-depth coding experience may use these technologies to evaluate patient data, enhance treatments, and boost operational efficiencies. Another industry gaining from no-code solutions is retail, which uses customer data to improve customer experiences and customize marketing campaigns.
No-code data science is being used by financial organizations for trend forecasting, fraud detection, and risk analysis. Financial specialists may swiftly and correctly make well-informed decisions with this strategy. No-code platforms are being used by the education industry to monitor learning outcomes, evaluate student performance, and improve teaching strategies.
One example of a successful application of no-code data science in a case study is a healthcare provider that used predictive analytics to lower readmission rates and enhance patient care outcomes. One retail company segmented its customer base based on purchasing patterns using no-code technologies, which led to highly successful focused marketing efforts. These illustrations show how different sectors might take advantage of real-world advantages and expansion prospects by utilizing no-code data science.
6. Challenges and Limitations
No-code tools have many advantages, but they also have several disadvantages and restrictions. The possible lack of customization and flexibility offered by traditional coding is one of the main issues. Complex or extremely particular requirements that are easily implemented by bespoke code may be difficult for no-code systems to support. For experienced users who require customized solutions for their data science projects, this constraint could be a barrier.
The limited functionality of no-code technologies might not always be adequate when data science activities become more complex and specialized. Some integrations, special data processing techniques, or complex algorithms may call for a degree of customization beyond what the current no-code systems can offer. One of the primary obstacles to fully utilizing no-code solutions is the difference between what users can accomplish with them and what they can accomplish with traditional coding techniques.
The capabilities of no-code platforms are constantly being improved by developers and tool makers in an effort to solve these concerns of complexity and customisation. These tools can be made more flexible and able to handle a wider variety of data science activities by adding more sophisticated capabilities, integrations, and customization choices. Facilitating the transfer of users from no-code to low-code or full-code settings can aid in bridging the divide between data science process complexity and simplicity.
While employing no-code tools for data science projects has some drawbacks, technological breakthroughs and a focus on user input are driving enhancements that make these tools more capable and flexible. The future of data science is not simply in conventional coding techniques, but also in creative solutions that solve the difficulties of complexity and personalization while democratizing access to analytical powers.
7. Future Trends in No-Code Data Science
Looking ahead to data science, it is certain that the no-code trend will significantly change the field. Predictions show a notable trend towards no-code data science tools and platforms due to technological developments and a growing emphasis on accessibility and usability. These technologies make data science more accessible by allowing people who don't know how to code to examine large, complicated datasets and draw insightful conclusions.
The evolution of no-code solutions is being shaped by innovations like drag-and-drop modeling features, natural language processing (NLP) interfaces, and automated machine learning (AutoML). With the help of more complex autoML algorithms, users can now create precise prediction models automatically, eliminating the need for human interaction. NLP interfaces facilitate natural language interaction with data, hence facilitating the extraction of valuable insights by non-technical people. The process of developing and implementing machine learning models is made simpler by drag-and-drop modeling features, which lowers the entrance barrier for prospective data scientists.
Additionally, augmented analytics—where AI helps people prepare data, find trends, and automatically generate insights—holds promise for the future of no-code data science. It is anticipated that this trend would simplify decision-making procedures and enable firms to more effectively get value from their data. It is expected that the performance of no-code tools will be further improved by developments in deep learning algorithms and neural network topologies, allowing users to more easily take on more challenging analytical tasks.
After putting everything above together, we can say that the development of no-code solutions is clearly linked to the future of data science. These tools will be essential in speeding up the digital transformation of many industries as they develop and become more advanced. No-code data science is positioned to transform how we approach analytics and spur creativity in the years to come by enabling people with a variety of backgrounds and skill sets to effectively harness the power of data.
8. The Ethical Considerations
The importance of ethical considerations cannot be overstated as no-code tools democratize data science. The emergence of no-code platforms prompts concerns regarding data usage practices and morality in decision-making. It's critical to talk about the moral ramifications of using these instruments in order to make sure that justice, accountability, and openness are promoted.
How no-code tools handle private and sensitive data is an important factor to take into account. It is crucial to make sure that data is managed securely and in accordance with laws like the GDPR. These technologies' algorithms may contain prejudice, which raises questions about how that could affect decision-making. To guarantee equitable results, biases must be assessed and reduced.
The possibility of abuse or unforeseen effects when utilizing no-code techniques is another ethical consideration. Decisions based on erroneous or biased data analysis run the danger of not being properly overseen and governed. Establishing explicit policies for the ethical use of these instruments is crucial for organizations. This includes educating staff members about ethical issues and guaranteeing openness in the decision-making procedures.💬
To sum up what I've written thus far, the development of no-code data science tools brings new ethical and responsible use issues in addition to enormous accessibility and efficiency benefits. Through proactive dialogue on these matters and the implementation of risk-reduction measures, companies can leverage the potential of no-code solutions while maintaining moral principles in their data-driven decision-making procedures.
9. Training and Education in No-Code Data Science
The emergence of no-code data science tools is revolutionizing the discipline by enabling non-programmers to access data analysis and machine learning. As long as this tendency persists, prospective professionals will increasingly require instructional resources. More and more organizations and online resources are providing training courses and programs geared at learning no-code data science, enabling students to get the abilities needed to handle data efficiently.📌
Learning pathways that emphasize no-code technologies offer a great starting point for anyone who want to work in data science but don't have any coding skills. These courses usually cover a wide range of subjects, including how to employ user-friendly interfaces for model deployment, data manipulation, visualization, and predictive analytics. Without learning complicated programming languages, people can gain a strong foundation in analyzing and drawing conclusions from data by adhering to standardized curricula created for no-code learners.
Access to this rapidly expanding field of no-code data science is made more accessible by the availability of training choices, whether in the form of formal education programs or self-paced online courses. These tools are essential for bridging the gap between traditional data science approaches and the rapidly changing field of no-code methodologies, as more professionals choose to advance their skills or move into data-related professions. Putting money into education focused on no-code tools gives people the skills they need to succeed in a data-driven environment and adjust to the shifting needs of contemporary enterprises.
10. The Role of AI in No-Code Platforms
Workflows in data science are being revolutionized by the incorporation of artificial intelligence into no-code platforms. The capabilities of no-code tools are being improved by AI-driven features, opening up hard jobs to a wider audience. These developments are simplifying procedures and empowering people to handle data more effectively and efficiently. We can anticipate AI playing an even bigger part in the future in enabling non-technical individuals to harness the power of data science without requiring in-depth coding knowledge.
11. Overcoming Resistance to Change
It's critical to overcome opposition to change while implementing no-code approaches in data science. Techniques like practical training sessions, success stories, and emphasising the efficiency improvements can all help dispel skeptics. Transparency in the algorithms utilized, a focus on accuracy through testing, and the development of a collaborative culture between data professionals and non-coders are all important components in developing trust in automated data solutions. Believing in these technologies will be essential to embracing data science in the future without traditional coding constraints.
12. Conclusion
As I mentioned earlier, the capacity of no-code to democratize data analytics, empower non-technical users, and speed up the process of extracting insights from data is what is driving the move in data science towards a no-code paradigm. No-code tools make it possible for more individuals to interact with and use data in a variety of fields by doing away with the requirement for traditional coding abilities. No-code platforms integrate seamlessly, simplifying intricate procedures and facilitating quick prototype and experimentation.
Leveraging no-code technology has the potential to be disruptive because it can close the gap between data professionals and domain specialists, promoting creativity and cooperation. Data-driven decision-making processes are now actively participatory for persons from diverse disciplines, thanks to user-friendly interfaces and visual workflows. This inclusive method to problem-solving fosters innovation and a variety of viewpoints in addition to increasing efficiency.🙃
It is evident that accessibility, agility, and collaboration will propel new benchmarks of productivity and innovation in the digital age when we see the future of data science via a no-code perspective. Through the utilisation of no-code solutions, entities can extract latent insights from their data assets and foster an ongoing culture of learning and adjustment. The transition to a no-code future represents a fundamental shift in data science approaches toward inclusivity and simplicity, as well as a technological revolution that will empower people.