1. Introduction
In today's data-driven environment, effective cooperation between modelers and data analysts is essential. To analyze data, modelers create intricate statistical models, and data analysts offer the know-how to collect, clean, and get ready data for modeling. In order to provide accurate insights and well-informed decision-making in a variety of industries, including marketing, finance, healthcare, and more, a collaborative connection is essential. Together, these two crucial positions may unleash a multitude of insightful data that promotes innovation and corporate success.
2. Understanding Roles
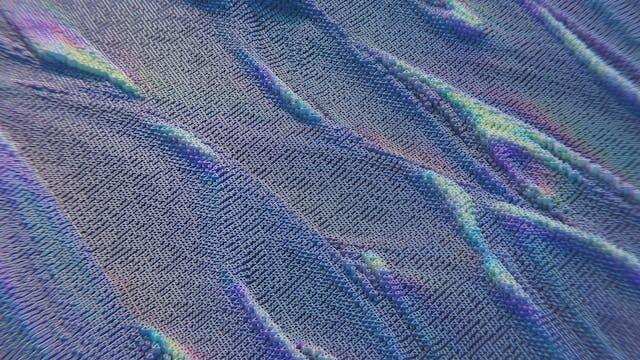
It is crucial to clarify the different responsibilities that modelers and data analysts play in a project in order to promote smooth collaboration between them. To extract valuable insights, data analysts are tasked with gathering, processing, and interpreting data. In order to support decision-making processes, they concentrate on identifying trends, patterns, and anomalies in data sets. Modelers, on the other hand, are experts in developing mathematical models and algorithms that use the examined data to provide forecasts or optimize solutions.
It is easier to avoid responsibility overlaps and guarantee that each team member is able to use their area of expertise when these roles are clearly defined. Working with unstructured data, data analysts convert it into a format appropriate for modeling assignments. Using this organized data, modelers create simulations or predictive models that can predict results or spot trends in the data.
Teams can improve the effectiveness of their collaborative efforts, minimize misunderstandings, and expedite workflow by making these roles clear from the beginning of a project. Working together and communicating effectively throughout the project lifecycle, modelers and data analysts are enabled by this fundamental knowledge.
3. Establishing Communication Channels
Efficient communication is essential to keeping modelers and data analysts on good terms. A successful collaboration requires the establishment of channels of communication that are both clear and efficient. The distance between these two teams can be reduced by making use of platforms like video conferencing software, project management tools, and messaging apps.
Modelers and data analysts can quickly exchange information by using messaging programs like as Slack or Microsoft Teams, which facilitate real-time feedback, sharing of updates, and questioning. This instantaneous communication makes problem-solving and teamwork easier.
Workflows can be streamlined by using project management systems like Trello or Asana, which organize activities, set deadlines, and monitor progress. Modelers and data analysts may make sure that everyone is in agreement on project milestones and stays on the same page by using these tools.
Software for video conferences, like as Zoom or Google Meet, might be useful for more in-depth conversations or brainstorming sessions. In-person or virtual interactions between team members can foster rapport and improve comprehension of one another's viewpoints.
Incorporating a mix of these communication platforms and methods can create a supportive environment for modelers and data analysts to work together seamlessly towards achieving common goals.
4. Setting Common Goals
Establishing shared objectives is essential to promoting productive cooperation between data analysts and modelers. Everyone will be working toward the same goals when their aims are in alignment. Modelers and data analysts can efficiently allocate resources, prioritize activities, and optimize workflow processes by establishing clear goals at the beginning of a project. This alignment encourages transparency, reduces miscommunication, and eventually improves project outcomes.
Modelers and data analysts can work together to create shared objectives by holding frequent meetings and conversations. Through fostering candid communication and brainstorming sessions, groups can come up with broad goals that are in line with the goals of the project and the expectations of its stakeholders. Through these talks, team members are able to fully grasp the needs and scope of the project while also utilizing their individual areas of expertise.
Determining key performance indicators (KPIs), which act as standards for gauging achievement, is necessary before setting shared objectives. Modelers and data analysts can better understand their goals and monitor their progress by setting up KPIs that are directly linked to the project's objectives. Teams can adjust to changing conditions, make required course corrections, and make sure they are on track to achieve or surpass expectations by routinely monitoring these KPIs.
Establishing shared objectives is crucial to fostering collaboration between data analysts and modelers. Teams can improve their efficiency, productivity, and overall performance in delivering meaningful data-driven solutions by defining clear KPIs, promoting collaboration, and aligning objectives. Setting a high priority on goal alignment fosters a sense of shared purpose that inspires creativity and excellence in all project endeavors, in addition to strengthening teamwork.
5. Sharing Insights and Findings
For successful project outcomes and mutual understanding, modelers and data analysts must share insights and conclusions in a collaborative setting. To assist this sharing process effectively, teams might employ many strategies.🖍
First of all, regular channels of communication like team meetings, status reports, or collaborative work sessions can generate opportunities for structured insight sharing. Fostering a discourse that is open and allows modelers and data analysts to freely express their viewpoints can result in a more thorough understanding of the project's development.
Transparency and accessibility can also be improved by employing collaborative tools and platforms that facilitate the real-time exchange of results and techniques. Collaboration solutions such as version control repositories, project management software, and shared documentation systems can facilitate information sharing and keep team members in sync.
Collaborating across functional lines can be facilitated by planning knowledge-sharing sessions or seminars where team members demonstrate their models, discuss approaches, or share their findings. This not only facilitates the sharing of insightful information but also encourages a culture of ongoing development within the team.
By embracing these approaches to sharing insights and findings, modelers and data analysts can establish a strong foundation for collaboration built on trust, transparency, and collective learning.
6. Implementing Version Control Systems
Maintaining a smooth working relationship between data analysts and modelers requires the implementation of version control systems. Teams may efficiently manage changes made to datasets and code by using version control systems like Git, which promotes improved collaboration and transparency throughout the project.⌨️
With the use of these tools, users can effortlessly manage iterations, making it simple to compare various model or dataset versions. Version control eliminates conflicts and ensures a more efficient workflow by allowing team members to work simultaneously on the same project without worrying about overwriting each other's work.🔶
By offering a central location for all project files to be kept and documented, version control systems improve workflow efficiency. This facilitates tracking of advancement as well as streamlines troubleshooting procedures and allows for the prompt discovery of problems or bugs that may occur throughout the development stage.
Incorporating version control systems into the collaborative process between modelers and data analysts is vital for guaranteeing smooth operations, good communication, and efficient project management.🗓
7. Addressing Challenges Proactively
In order to keep modelers and data analysts happy, it is imperative that problems are handled early on. These professions frequently struggle with poor communication, conflicting priorities, and a lack of respect for one another's jobs. Regular check-ins and the use of collaboration tools like shared dashboards can assist prevent miscommunication by ensuring that everyone is in agreement.
It's critical to set clear expectations and goals early on in a project to avoid conflicts over priority. Setting up regular alignment sessions where both parties explain their aims and how they contribute to the larger project can help create understanding and unity. Respect and cooperation between team members can be greatly increased by cultivating an environment where people value one another's skills and contributions.
Setting up comprehensive data validation procedures and carrying out routine data quality checks will assist identify any issues early on and reduce challenges related to data quality concerns. Improving the general quality of insights obtained from the data can also be accomplished by teaching modelers and data analysts on best methods for data gathering, cleaning, and maintenance.
By being proactive in addressing these common challenges, modelers and data analysts can work more efficiently together, leading to better outcomes for the projects they collaborate on.
8. Regular Check-ins and Updates
In order to ensure that modelers and data analysts collaborate effectively, regular check-ins and updates are essential. These meetings assist in upholding openness, encouraging coordination, and resolving any issues that may come up throughout the project. By setting up these frequent get-togethers, both teams may exchange updates on their progress, discuss ideas to improve workflow, and keep focused on their objectives. To ensure that everyone is working toward the same goals and to prevent misunderstandings, effective communication through regular check-ins is essential. Furthermore, it facilitates prompt modifications and enhancements when required, resulting in superior outcomes.
9. Continuous Learning and Skill Development
Continuous learning and skill development are crucial elements in maintaining a harmonious relationship between modelers and data analysts. Advocating for ongoing learning initiatives not only enhances skills but also fosters collaboration and ensures that teams stay abreast of industry developments. Encouraging team members to engage in continuous learning aids in keeping their knowledge and abilities up-to-date, allowing them to adapt to evolving technologies and methodologies effectively. By supporting ongoing training programs, workshops, or courses, organizations can create an environment that values growth and improvement. This emphasis on continuous learning not only benefits individual team members by enhancing their expertise but also boosts the collective capacity of the team as a whole. It encourages a culture of curiosity, innovation, and adaptability within the team, leading to increased productivity and efficiency in tackling complex data projects.
Modelers and data analysts can fill in any skill gaps by continuing their education. In order for experts working in the data science area to collaborate effectively, they must constantly refresh their skills due to the rapid evolution of technologies. Through the promotion of continuous learning programs, companies may guarantee that their groups have the know-how to work together on projects efficiently, utilizing one another's advantages and working together to achieve successful results.👋
Drawing from the aforementioned, it is imperative to prioritize ongoing education and skill enhancement in order to cultivate a fruitful collaboration between data analysts and modelers. By investing in continuing education opportunities for team members, firms may build a dynamic and collaborative workplace where individuals are empowered to better their capabilities continually. Team members' professional development is aided by this dedication to learning, which also helps teams quickly adjust to changes in the business while continuously producing high-caliber work.
10. Celebrating Achievements Together
Together, celebrating successes is essential to creating a healthy work atmosphere and deepening the relationship between data analysts and modelers. Organizing frequent recognition events, such as quarterly award ceremonies or monthly team lunches where exceptional efforts are recognized, can help to improve team morale and motivation. Another good way to increase collaborative spirit is to promote peer appreciation through shoutouts in team meetings or a dedicated Slack channel.
Another tactic is to put together an easily accessible virtual "wall of fame" that highlights both individual and group accomplishments. This visual depiction can inspire others to pursue excellence by acting as a continual reminder of the team's accomplishments. Organizing small group activities that foster camaraderie and relationship-building among team members, such as volunteering together or taking part in team-building exercises outside of work, can be beneficial.
Giving individualized prizes like gift cards, more paid time off, or chances for professional growth is a great way to recognize hard work and promote excellence. In order to keep the team's culture positive and focused on continual growth, don't forget to recognize and celebrate both large and minor victories. Recognizing accomplishments as a group not only raises spirits but fosters a cooperative, encouraging work atmosphere where all employees feel appreciated and inspired to do well.
11. Handling Disagreements Constructively
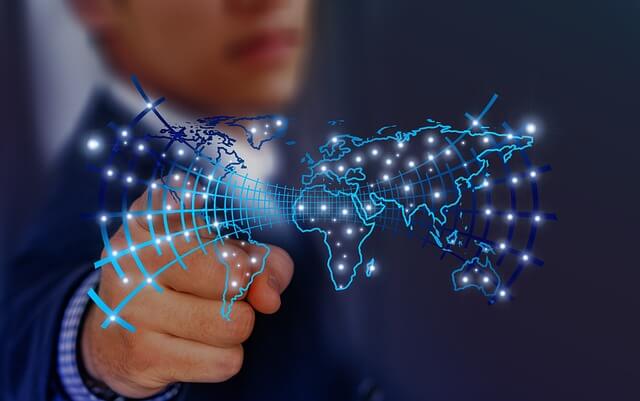
Building a peaceful and fruitful working relationship between data analysts and modelers requires skillfully managing conflicts. Setting open communication and mutual respect as top priorities is crucial for handling conflicts well. Encourage team members to openly share their opinions while paying attention to each other in order to reduce conflict and foster cooperative problem-solving. Creating unambiguous policies for handling disputes and encouraging a problem-solving approach can aid in preventing conflicts from getting worse.😼
Fostering empathy and understanding among team members is a useful tactic for resolving conflicts. It is easier for modelers and data analysts to find common ground when they respect each other's opinions and take into account different points of view. One method to move the conversation away from individual conflicts and toward a mindset of group success is to highlight the common objectives of the project and concentrate on coming up with solutions that will benefit the entire group.
It's crucial to establish a secure environment for conversations where people may voice their worries without worrying about criticism or retaliation. Team leaders should take the initiative to resolve disputes by encouraging fruitful conversations, pointing out misconceptions, and steering the conversation in the direction of finding a solution. Modelers and data analysts can resolve conflicts in a way that improves rather than undermines their collaboration by creating an atmosphere of trust and openness.
Lastly, using data-driven insights to guide decision-making in conflict situations might offer a framework for objectively settling disagreements. Instead of responding emotionally to arguments, modelers and data analysts can approach them with logical reasoning by focusing on factual evidence and quantitative analysis. Teams can have more informed conversations and come to decisions based on facts rather than views when data is used to test theories or support arguments.
After putting everything above together, we can say that effective communication, respect for one another, empathy, and data-driven decision-making are all necessary for managing conflicts between modelers and data analysts in a positive manner. Teams can successfully handle conflicts and sustain constructive working relationships by fostering a climate of open communication, acknowledging and respecting opposing views, providing a safe space for conversations, and using objective evidence to guide resolutions. Collaboration between modelers and data analysts can be strengthened by viewing disagreements as chances for learning and development. This will result in more creative solutions and significant results.
12. Conclusion
Furthermore, as I mentioned previously, good project outcomes depend on modelers and data analysts working together in a seamless manner. The foundation of a productive working relationship is mutual respect, open communication, and awareness of one another's areas of competence. More productive teamwork can be achieved by defining shared objectives, using individual skills, and setting clear expectations. Frequent check-ins, feedback meetings, and open lines of contact facilitate quick resolution of any problems.
Modelers and data analysts can both gain from each other's thoughts and abilities by fostering a collaborative environment that promotes knowledge and idea sharing. Accepting diversity in viewpoints and methods can result in more creative solutions and improved decision-making. Through the prioritization of good communication and teamwork, businesses can optimize their teams' capabilities and attain project outcomes that satisfy stakeholders.