1. Introduction
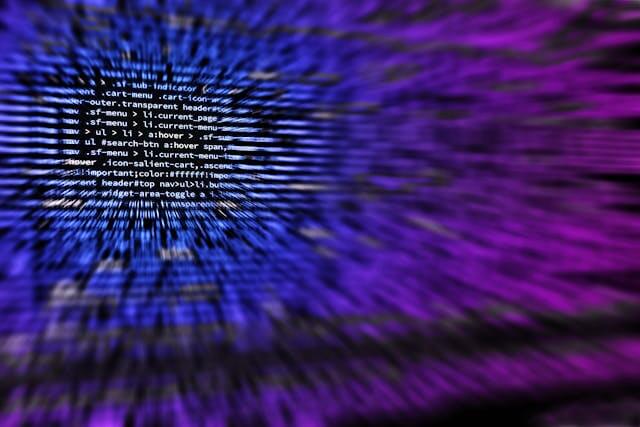
Introduction: In today's data-driven world, the demand for skilled professionals who can analyze and derive insights from large datasets is higher than ever. Data science talent refers to individuals with expertise in statistics, machine learning, programming, and domain knowledge who can effectively extract value from data. Hiring the right data science professionals is crucial for businesses looking to leverage their data effectively and gain a competitive edge in the market. These experts play a key role in uncovering patterns, trends, and valuable insights that drive informed decision-making and business growth.
2. Identifying Your Needs
When hiring data science expertise, knowing your needs is essential. Start by determining the particular data science needs of your organization. Recognize your obstacles and the ways in which data might assist in overcoming them. You can more easily determine areas where a data scientist can have the greatest influence by determining the gaps in your present data strategies.
Next, ascertain the knowledge and abilities required for the position. Think about if you require someone with expertise in statistical analysis, big data technology, machine learning, or a particular domain. Identify the primary duties of the position and ensure that the required skill set is in place. This transparency will draw in the right applicants and guarantee that they can successfully support your team in using data to make decisions.
3. Crafting a Detailed Job Description
When hiring someone with data science expertise, creating a thorough job description is essential. The first step in drawing in the best applicants for the job is to create a thorough job description. Candidates are better able to understand what is expected of them when the duties and expectations of the post are outlined in clear terms.
Emphasize the main duties that the data scientist will be expected to perform in order to create an effective job description. This could entail doing things like creating algorithms, evaluating large, complicated data sets, and interpreting the outcomes to inform business choices. A comprehensive description of these duties will help prospective candidates understand exactly what the position entails.
Defining the credentials and abilities needed for the role is equally vital. It can be beneficial to highlight certain technical talents in order to draw in candidates with the necessary experience. Examples of these skills include machine learning algorithms, statistical analysis tools, and programming languages like R or Python. It's crucial to highlight soft skills like communication, problem-solving, and critical thinking while looking for a well-rounded individual for the data science position.
4. Evaluating Technical Skills
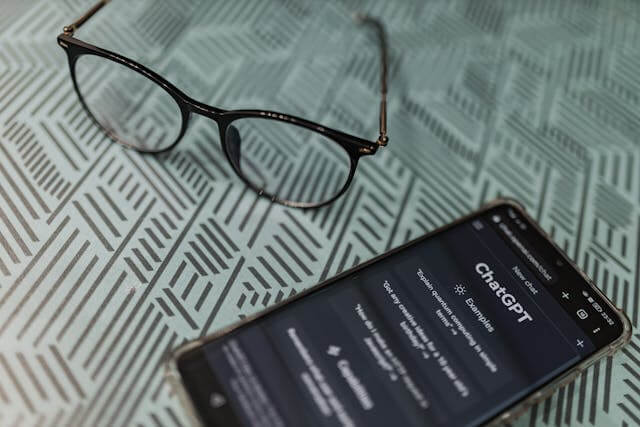
It's critical to determine the fundamental technical competencies needed for the position while assessing potential data scientists. Data science requires a foundational understanding of machine learning algorithms like regression, clustering, and classification in addition to programming languages like Python and R. For a data scientist, having proficiency with data visualization programs like Tableau or Power BI can be quite beneficial.
There are several ways to evaluate a candidate's technical proficiency during the recruiting process. Technical interviews can be used to assess a candidate's programming and problem-solving capabilities by having them solve real-world problems or take part in coding challenges. Inquiring about previous projects completed by applicants or performing technical evaluations might reveal information about their grasp of machine learning principles and how they are used.
Asking applicants to present their GitHub repositories or portfolio can provide insight into their coding style and real-world experience. Quantifying candidates' technical skills successfully can also be facilitated by using online platforms for data science-specific skill evaluations. Employers may make sure they are selecting data science people with the technical aptitude needed to succeed in the position by combining these strategies.
5. Assessing Problem-Solving Abilities
Evaluating problem-solving skills is essential when interviewing for data science positions. In this line of work, the ability to think critically and solve problems is essential. During the interview process, take into consideration using case studies or practical exercises to evaluate a candidate's problem-solving skills. These methods can reveal how applicants handle difficult data-related problems and arrive at wise choices. You can more accurately judge their fit for the position and gauge their potential to perform well in a dynamic, data-driven workplace by seeing how they solve problems.
6. Judging Communication Skills
In today's collaborative work environment, data science professionals must possess effective communication skills in order to thrive. Data scientists must be able to work well with others in a team, cooperate on projects successfully, and clearly convey their findings to stakeholders. Ineffective teamwork, misunderstandings, and incorrect perception of outcomes can all be caused by poor communication.
It's critical to evaluate candidates' communication abilities during the interview process when hiring individuals with experience in data science. Asking candidates about previous projects where they had to collaborate with a team or share their findings is one method to assess this. Keep an eye on how they express themselves, whether they can simplify difficult concepts, and whether they interact with people in a courteous and straightforward way.
Employing techniques like role-playing or assigning applicants a brief presentation challenge might yield insightful information about their ability to communicate under duress. Seek applicants that can actively listen, adjust their communication style to the needs of the audience, and show empathy for the viewpoints of their teammates. During the hiring process, you can make sure that the members of your data science team are not only technically skilled but also have the ability to work well with others by giving priority to communication skills.
7. Cultural Fit and Team Dynamics
Hiring data science talent requires careful consideration of team dynamics and cultural fit. For long-term success, it is critical to understand how important it is to match a candidate's values with your organization. Assessing candidates' compatibility can be done in part by using techniques including behavioral interviews, team-based tests, and looking through their prior work experiences in comparable settings. You can make sure that your workplace is cohesive and collaborative, which encourages creativity and productivity, by evaluating how well a prospective hire fits in with the team dynamics and corporate culture.
8. Experience and Qualifications
Finding the right balance between academic credentials and practical experience is essential when hiring talent in data science. Academic credentials offer the fundamental information and abilities needed to comprehend intricate algorithms and statistical techniques. Conversely, applicants with real-world experience are better equipped to solve problems, get useful insights, and put theoretical knowledge into practice.
Assessing a candidate's prior work, initiatives, or studies can help you evaluate them more effectively. Request samples of their work that show they can use data-driven techniques to solve challenges. Analyze the results of their labor by examining measures like increased productivity, cost savings, or revenue production. Take into account their ability to explain intricate technical ideas to stakeholders who are not technical, as this is a critical competency for data science positions.
Seek applicants who have a curiosity for learning and professional development in addition to their technical proficiency. Examine their knowledge of the various tools and technologies frequently utilized in data science initiatives. To assess their flexibility and people skills, find out if they have any experience interacting with professionals from other backgrounds or working in cross-functional teams. In changing data science workplaces, a candidate who possesses both academic knowledge and practical experience is more likely to succeed.
9. Diversity and Inclusion
Diversity in data science teams is essential for developing creative ideas and promoting corporate success in today's data-driven world. Accepting variety brings people together who have different viewpoints, backgrounds, and abilities, which can stimulate more creative and thorough problem-solving in a team.😎
Organizations can use tactics like setting diversity goals, utilizing inclusive language in job descriptions, collaborating with diverse recruitment channels, training interviewers on unconscious bias, and guaranteeing diverse representation on interview panels to ensure inclusivity throughout the hiring process.
Putting diversity and inclusion first not only creates a more lively and dynamic work atmosphere, but it also helps with decision-making and, in the end, improves the performance of data science teams as a whole. Through proactive recruitment methods that prioritize diversity and inclusivity, organizations may create more robust teams capable of addressing intricate problems and propelling innovation.
10. Salary Negotiation and Benefits
It's critical to look up industry norms and take the candidate's experience level into account when negotiating a salary for data science skills. Offering top talent a competitive wage that is in line with market rates might draw them in and show that the company values their abilities. While calculating pay packages, other factors like geography, job requirements, and the company's financial resources should be considered.
Offering alluring bonuses and benefits in addition to a competitive compensation might make a big difference in a candidate's decision to join your company. In addition to standard benefits like health insurance and retirement plans, your company can differentiate itself from rivals by providing special services like flexible work schedules, chances for professional growth, or even wellness initiatives. In a market where hiring is competitive, knowing what matters most to data science specialists and designing benefits packages appropriately can help draw and keep top talent.
Organizations can establish themselves as desired employers in the data science profession by offering competitive pay along with enticing benefit packages. Long-term staff retention and satisfaction are enhanced by investing in these areas, which also aid in attracting top personnel.
11. Assessing Growth Potential
Building a strong team requires considering development potential when hiring data science talent. Seek applicants who exhibit a readiness to pick up new skills and adjust to different processes and technology. Inquire about their prior experiences implementing new techniques or tools, as this may provide insight into their potential to advance within your company. When conducting interviews, think about designing scenario-based questions to assess candidates' problem-solving agility and approach to issues. Seeking out people with a broad skill set or a history of lifelong learning can be signs that someone on your team has a lot of room to grow. In the face of changing technological trends, you can make sure that your data science team stays creative and productive by emphasizing the recruitment of personnel who are flexible and willing to learn new skills.
12. Conclusion
As I mentioned before, take into account the particular abilities needed for your project, such as statistical analysis, programming languages, and domain knowledge, when hiring data science personnel. Make sure that it is in line with your company's objectives and culture in order to promote a productive workplace. Candidates with inventiveness, problem-solving skills, and a commitment to lifelong learning should be given preference.📣
The success of your initiatives and the overall results of your organization can be greatly impacted by the judgments you make when hiring data science specialists. You can create a strong data science team that fosters creativity and produces insightful reports for your company by taking the time to fully understand your goals, carefully evaluate candidates' talents, and include a variety of viewpoints in the hiring process.
Keep in mind that identifying the proper data science talent involves more than just technical skills; it also involves finding people who can work well with others, convincingly express their ideas, and adjust to changing difficulties in the quickly shifting data field. By concentrating on these important factors, you may improve your data science skills and set up your business for success in the data-driven world of today.