1. Introduction:
The significance of data quality management in today's data-driven environment cannot be emphasized. Gaining insights, reaching company objectives, and making well-informed decisions all depend on having accurate and high-quality data. Organizations run the danger of drawing incorrect conclusions that could result in inefficiencies and lost opportunities if they don't implement appropriate data quality procedures.
Adhering to a structured methodology is crucial for guaranteeing data veracity at scale. Organizations may improve the quality of their data throughout its lifecycle with the help of the six stages for growing data reliability. The goal of these phases is to create a strong basis for precise analysis and decision-making, and they cover everything from initial data gathering to continuing monitoring and maintenance. Every phase is essential to preserving the reliability and integrity of the data that powers corporate processes.
2. Understanding Data Quality:
Any company hoping to use its data to make choices must have a solid understanding of data quality. The accuracy, completeness, dependability, and applicability of data utilized in decision-making processes are referred to as data quality. It matters because inaccurate assumptions and erroneous tactics resulting from poor data quality can eventually affect the success of businesses.
Erroneous information, missing values, duplicates, inconsistencies, and errors are common problems with data quality. Inaccurate data might be the result of old systems providing inaccurate information or human error during input. Disparities in the way data is recorded or organized in various sources lead to inconsistencies. Redundant records resulting from duplications can distort analyses. Decisions based on outdated information may be flawed since it does not accurately reflect the current situation. Gaps caused by missing values in datasets might compromise the reliability of conclusions derived from them.
3. Setting the Foundation:
Establishing a solid foundation is essential for guaranteeing dependable and trustworthy data in the field of data quality management. Setting specific goals and objectives that complement the overall business plan is a crucial step in this process. Organizations can efficiently direct their efforts towards enhancing data timeliness, accuracy, completeness, and consistency by establishing these goals early on.🤏
Building a robust data quality team is vital for successfully managing data quality projects. To do this, a group of knowledgeable experts in data governance, stewardship, and analysis must be put together. For every team member to be fully covered in every facet of data quality management, their roles and duties should be clearly defined.
Organizations may build a strong foundation for increasing data reliability and promoting enhanced decision-making processes based on high-quality data inputs by defining clear objectives and targets and putting together a dedicated data quality team.
4. Data Profiling and Assessment:
Scaling data dependability in the field of data quality management requires careful data assessment and profiling. This crucial phase entails exploring the depths of your present datasets to acquire a thorough grasp of their current condition. Businesses can find interesting trends, correlations, and anomalies in their data repositories by using data profiling.
You can identify areas that need improvement at this stage by evaluating the quality of your current data sources. Organizations can find redundant information, inconsistencies, incompleteness, and inaccuracies in their datasets by doing this critical examination. Improving the overall quality and integrity of your data requires an understanding of the advantages and disadvantages of your data sets.
Data profiling gives you an in-depth picture of the condition of your data by exposing important details about its composition, quality, and structure. It enables companies to examine a range of characteristics in their datasets, including duplicates, erroneous entries, outliers, and missing numbers. This in-depth exploration of data sets' complexities provides the groundwork for deliberating wisely on how to improve the general dependability and credibility of the data assets in your company.
Organizations can build key performance indicators (KPIs) pertaining to data quality metrics by implementing thorough assessment processes. Businesses can create clear criteria for tracking improvements in their data quality over time by setting these KPIs early in the data profiling and assessment phase. By using this tactical method, businesses can monitor advancements efficiently and make sure that efforts to improve data reliability are paying off. 💍
Organizations may improve the scalability and dependability of their precious data assets by devoting time and money to thorough data profiling and assessment methods. Accepting these fundamental phases sets the path for putting focused tactics into practice that promote ongoing enhancements in data quality management throughout the company.
5. Data Cleaning and Standardization:
In the data quality management process, one crucial stage is Data Cleaning and Standardization. This stage involves implementing systematic processes to clean and standardize data, ensuring accuracy and consistency across the board. By employing specialized tools and techniques, organizations can enhance the quality of their data by eliminating errors, inconsistencies, and duplications. Data cleaning aims to correct or remove inaccurate records, while standardization ensures that data formats, conventions, and values are uniform throughout the database. This stage plays a vital role in improving the overall reliability of data for better decision-making and operational efficiency.
6. Data Quality Monitoring:
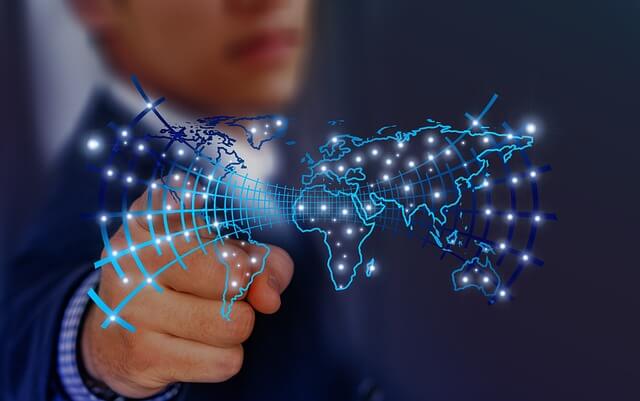
One of the most important steps in guaranteeing data reliability in an organization is data quality monitoring. Businesses are able to actively evaluate the state of their data at any given time by putting up monitoring systems to track important data quality parameters. This entails setting up warnings and alerts that quickly identify abnormalities or mistakes in real-time, enabling swift correction.
By putting strong data quality monitoring procedures in place, businesses may proactively identify problems before they get out of hand and reduce the chance that choices will be made based on false or incomplete information. Organizations may uphold a high degree of data integrity and confidence in their analytics and reporting outputs by consistently checking data quality parameters. This proactive strategy strengthens the business's ability to make decisions and increases operational efficiency.
Organizations can spot patterns or trends that could affect the accuracy or completeness of their data by using efficient data quality monitoring. Teams can quickly solve underlying problems and avoid negative effects on business operations thanks to this insightful information. Companies may maintain a continuous level of dependability in their data-driven projects by being alert and sensitive to changes in data quality measurements.
Reactive approaches are no longer adequate for controlling data quality in the fast-paced business world of today. Companies need to take proactive steps, like putting in place thorough monitoring systems that offer instantaneous insights into the condition of their data assets. Organizations may stay ahead of possible problems and uphold high levels of confidence in their data integrity by utilizing automated warnings and notifications for anomalies or errors.
An organization's comprehensive data management strategy must include data quality monitoring. By guaranteeing that the required level of quality is constantly met across all datasets, it supports efforts in data cleansing, enrichment, and governance. Creating a culture of continuous monitoring helps the business understand the value of reliable data and cultivates a mindset that is committed to upholding high standards at all phases of the data lifecycle.
Based on the information presented above, we can infer that any business that wants to scale data reliability must invest in strong mechanisms for monitoring data quality. Through the deployment of sophisticated monitoring systems and prompt alerting mechanisms, enterprises may proactively protect the accuracy of their data assets and make better decisions grounded in factual information. Adopting a proactive stance when it comes to data quality management not only improves operational effectiveness but also fosters a culture that values excellence and reliability when managing organizational data.
7. Continuous Improvement Strategies:
Continuous improvement techniques are crucial for sustaining and improving data reliability over time in the context of data quality management. Establishing feedback loops that enable continuous evaluations and modifications to the data quality procedures in place is a critical component of this. Organizations are able to pinpoint areas for improvement and implement the necessary modifications to guarantee data consistency and correctness by gathering input from users, stakeholders, and system monitoring tools.
Maintaining high standards for data quality requires the organization to cultivate a culture of continual improvement. This entails encouraging an attitude that prioritizes learning from failures, welcoming innovation in data management techniques, and placing a high priority on routine assessments of data quality performance. The business may adapt its data quality management procedures to efficiently meet contemporary demands when staff members at all levels see the value of data dependability and are motivated to actively contribute to its improvement.
Companies may proactively address data quality concerns as they arise, more effectively adapt to changing business needs, and eventually scale their data dependability initiatives for long-term success by integrating feedback loops and fostering a culture of continuous improvement.
8. Data Governance Framework:
A strong data governance framework is necessary in the field of data quality management to guarantee the accuracy and consistency of data. This framework entails creating standards, policies, and practices that control data management inside a company. Companies may optimize their data processes and reduce errors by implementing well-defined procedures for managing data, starting from collection and ending with storage and utilization.
Ensuring compliance with laws like the Health Insurance Portability and Accountability Act (HIPAA) and the General Data Protection Regulation (GDPR) is an essential component of a data governance framework. These laws provide stringent guidelines for the gathering, storing, and use of personal data in order to safeguard data security and preserve individual privacy. Following these rules not only keeps businesses out of legal hot water but also fosters customer trust by showcasing a dedication to safeguarding private data.
The cooperative efforts of stakeholders from all over an organization are necessary for effective data governance. It involves continual communication, training, and monitoring to ensure that everyone understands their roles and responsibilities for data management. Businesses can create a culture of data accountability and transparency to support their overall data quality management strategy by putting in place a strong Data Governance Framework.
9. Data Quality Metrics and KPIs:
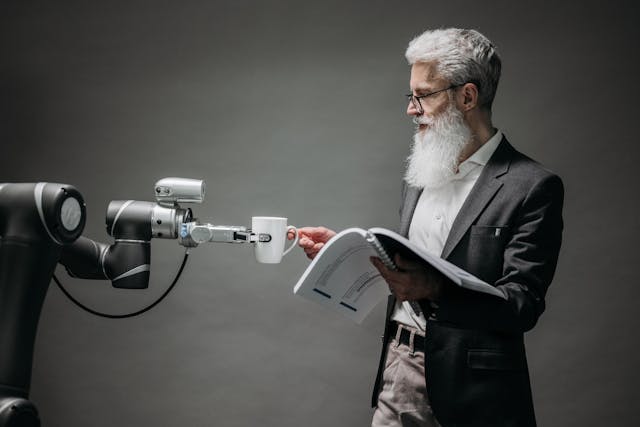
A strong data quality management strategy must include both KPIs and data quality metrics. Organizations are able to test and evaluate their data reliability in a systematic manner by developing key performance indicators (KPIs) that are particular to data quality. These KPIs act as standards to determine the success of data quality programs and point out areas that need improvement.
Setting up measurements is just as important for monitoring long-term gains in data quality. Through the establishment of quantifiable criteria including timeliness, correctness, completeness, and consistency, companies can evaluate their progress in improving data reliability in a quantitative manner. These metrics give stakeholders a clear view of the quality of the data as it stands today and let them set reasonable improvement targets based on observable outcomes.
Organizations can obtain a thorough grasp of their data environment by integrating qualitative and quantitative criteria into data quality metrics. Quantitative measures give specific proof of advancements or regressions in important domains, whilst qualitative evaluations offer insightful analyses of the contextual significance and usefulness of data. Organizations can create a comprehensive picture of their data quality performance and make wise decisions to promote long-lasting improvements by combining these methods.
Key performance indicators (KPIs) and metrics must be continuously monitored and assessed in order for data quality management to be effective. By monitoring these metrics on a regular basis, companies may spot trends, patterns, and abnormalities in their data quality procedures and take prompt action when deviations happen. Organizations may prevent problems before they become more serious by utilizing the real-time insights these measurements provide, guaranteeing the continuous scalability and dependability of their data operations.
Driving meaningful outcomes requires coordinating data quality indicators with overarching organizational goals. Through the integration of KPIs with strategic goals like better decision-making, better customer experiences, or regulatory compliance, organizations may exhibit the direct influence of high-quality data on business success. Because of this alignment, an organization's culture is strengthened to view data integrity as a critical component that makes all other operations possible.
Scaling data reliability within an organization requires establishing strong KPIs and measures for data quality. Organizations can improve their entire data quality posture by methodically comparing performance to predetermined benchmarks and monitoring improvements over time through quantitative measures. In today's increasingly data-driven environment, this proactive strategy not only reduces the risks associated with low-quality data but also opens doors for innovation, efficiency improvements, and competitive advantages.
10. Training and Education Programs:
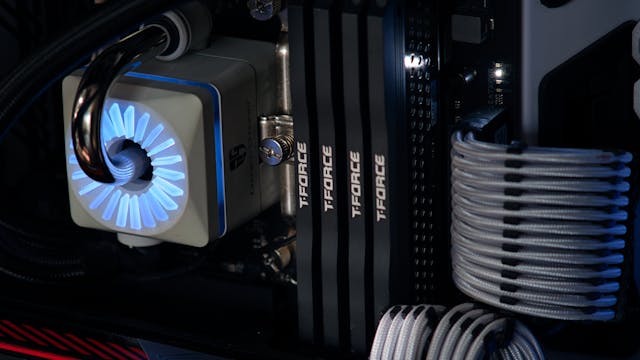
Establishing and upholding a high standard of data quality inside an organization requires both education and training. By holding training sessions centered on data management best practices, staff members are equipped with the information and abilities needed to maintain data dependability requirements. Through training staff members on the importance of their part in maintaining reliable and accurate data, companies may cultivate a culture that prioritizes data integrity at all levels.
These training courses are essential in providing staff members with the skills necessary to actively participate in upholding data quality requirements. Staff members learn how to perform actions that directly affect the quality and dependability of the organization's data through focused education efforts. Through fostering a culture of accountability and ownership for data quality, firms may mobilize their workforce to maintain elevated standards throughout all aspects of operations.
Embracing education and training into company culture improves individual skills and supports overall data quality management initiatives. Offering opportunities for ongoing learning guarantees that staff members remain up to date on the latest developments in data management technologies and best practices. Businesses may develop a workforce that is fervently committed to protecting the integrity of their data assets by highlighting the significance of data quality at all organizational levels.
By implementing comprehensive training and education programs targeted to suit specific roles and responsibilities within the business, firms may empower their workers to champion data reliability projects effectively. These programs facilitate communication, close knowledge gaps, and foster a common awareness of the vital part that each employee plays in maintaining data quality standards. Putting money into training and educational initiatives improves data governance procedures and creates a strong base for implementing dependable data management plans across the entire organization.
11. Integration with Data Management Systems:
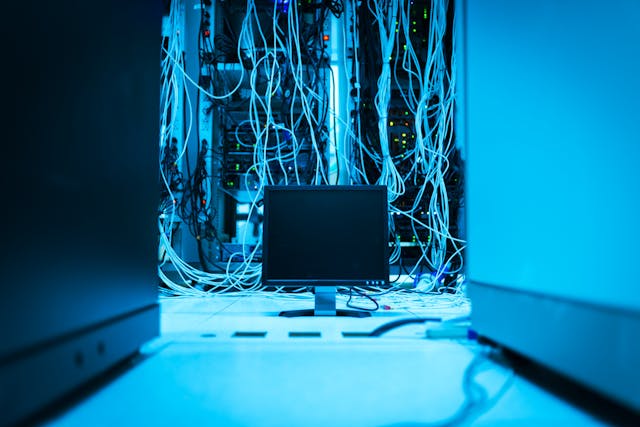
For data to be accurate and dependable, data management solutions must include data quality. Through the seamless integration of data quality procedures into pre-existing workflows and systems, organizations can improve their overall operational efficiency. By automating repetitive operations like data validation and conserving time and resources, the process can be further simplified by utilizing automation tools. This connection decreases manual intervention in the data management process, which boosts efficiency while also improving data dependability.
Maintaining uniform data quality across several platforms and databases is made possible for enterprises through effective integration with data management solutions. Businesses may guarantee that the data they rely on is correct and current by automating processes like data cleansing and standardization. This simplified method improves decision-making processes based on trustworthy information while reducing errors. By embracing automation, data quality management becomes scalable, enabling businesses to handle massive data volumes without sacrificing accuracy.
Integrating quality processes with current systems is essential for maintaining the integrity of organizational information in today's data-driven environment. By lowering human error and quickening the pace of data validation and correction, automation is essential to simplifying these procedures. Through smooth integration with data management systems, businesses may handle their massive amounts of data more efficiently while maintaining the correctness and dependability of the data across a range of operations.
12. Case Studies:
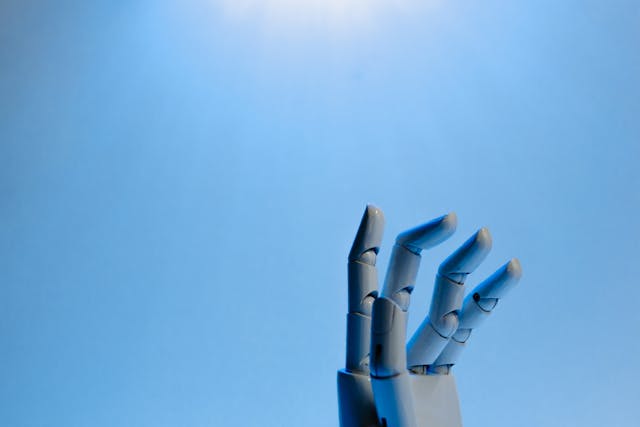
In the final section of our blog on Data Quality Management, we delve into real-life Case Studies to illustrate how companies have effectively enhanced their data reliability through the 6 stages we discussed earlier. 1. Company A: A multinational e-commerce giant adopted a systematic approach starting from data collection processes and investing in advanced data quality tools. By implementing automated checks and validation at every stage, they significantly reduced errors in customer profiles and transactional data.
2. Company B: By clearly defining ownership and accountability at every point of the data lifecycle, this well-known financial institution updated its data governance structure. As a result, reports and analytics became more consistent, which benefited stakeholder decision-making.
3. Company C: By emphasizing employee training and upskilling in effective data handling methods, this healthcare institution was able to increase the quality of its data. Their team was equipped with the requisite skills and expertise, enabling them to guarantee an excellent data quality culture across the entire firm.
These case studies are strong illustrations of how businesses may prioritize data quality management practices through the six crucial steps described in this blog post to alter their operations and decision-making. In today's data-driven world, every company may improve its data reliability and generate better business outcomes through organizational alignment, technology investments, strategic planning, and continuous improvement initiatives.