1. Introduction
The dynamic and quickly expanding subject of data science is the extraction of knowledge and insights from data using a variety of methods. The need for qualified experts who can interpret this data is growing as the amount of data that is available keeps growing. Aspiring data scientists must a strong set of abilities that include technical know-how, critical thinking, and excellent communication to succeed in this cutthroat environment. These abilities are essential for converting unprocessed data into useful insights and promoting well-informed decision-making in businesses. We will examine the fundamental abilities needed to start a lucrative career in data science in this blog post.🔷
2. Essential Technical Skills
It is important to acquire a few key technical abilities in order to work as a data scientist. First and foremost, data manipulation and analysis require a solid foundation in programming languages like Python and R. Because of their extensive libraries and tools designed specifically for data science workloads, these flexible languages are highly utilized.
Cleaning and data manipulation are yet another essential skill set. In order to extract valuable insights from handling massive datasets, one must possess the capacity to clean, preprocess, and organize data quickly. Accurate analysis and modeling are facilitated by a solid foundation in this domain.
Additionally essential are a strong grasp of statistical analysis and mathematical proficiency. For data scientists to properly evaluate data and make inferences from it, they need to be at ease with statistical principles. Mathematical proficiency offers the foundation for efficiently creating and improving analytical models.
Lastly, it is critical for prospective data scientists to be knowledgeable with machine learning algorithms. Professionals may create prediction models, find patterns in data, and make defensible decisions based on insights from intricate datasets by applying a variety of machine learning approaches.
3. Proficiency in Data Visualization
Any aspirant data scientist must possess proficiency in data visualization. Effectively presenting complicated data findings to a variety of stakeholders requires the ability to do so in a clear, aesthetically pleasing manner. In the industry, tools like Tableau, Power BI, and Matplotlib are frequently used to create powerful visuals that aid in comprehending correlations, trends, and patterns in the data.
By transforming numbers and statistics into captivating pictures, data visualization improves storytelling in addition to making data interpretation simpler. Being skilled with these technologies as a data scientist enables you to produce interactive reports, dashboards, and infographics that can communicate your findings more effectively than standard text-based summaries. When delivering findings to non-technical audiences, who might not possess the same level of knowledge in evaluating raw data, this talent is extremely valuable.🗜
You can improve the usability and accessibility of your data science projects for decision-makers by becoming an expert in data visualization tools and methodologies. Whether it's detecting patterns, correlations, or outliers in a dataset, being able to visualize these results can help organizations make better decisions. Gaining proficiency in data visualization allows you to create visually captivating tales that drive real effect and unlock the full potential of your analytical work.
4. Understanding of Big Data Technologies
Anyone who wants to work as a professional data scientist needs to understand big data technology. Because Hadoop, Spark, and SQL are frequently used for organizing and analyzing huge datasets, proficiency with these tools is crucial. With the use of these technologies, data scientists can effectively process enormous volumes of information and derive insightful information that can influence important decisions.
Large datasets can be processed and stored in a distributed manner across computer clusters using the Hadoop platform. In contrast, Spark is renowned for its quickness and user-friendliness while handling intricate analytical jobs. To efficiently manipulate and query databases, one must have a solid understanding of SQL (Structured Query Language). In today's data-driven environment, data scientists with proficiency in these techniques are highly sought-after specialists due to their ability to handle vast amounts of data with agility and precision.
One of the main characteristics of high-achieving data scientists is their ability to handle massive datasets effectively. Working with big data involves not just technical expertise but also strategic thought to maximize the performance of processes and algorithms. Aspiring data scientists can improve their analytical skills and significantly impact their organizations by becoming proficient in handling large datasets and big data technologies.⌚️
5. Business Acumen and Domain Knowledge
Data scientists need to possess both domain expertise and commercial insight. Comprehending the sector in which you operate enables you to proficiently contextualize data results. This comprehension facilitates the conversion of data insights into workable business plans. Strong business acumen in data science enables them to close the gap between unprocessed data and useful results, giving their firms' decision-makers important new information. Their profound comprehension of data analytics and business operations enables them to make real and significant contributions to the company's success.
6. Soft Skills for Success
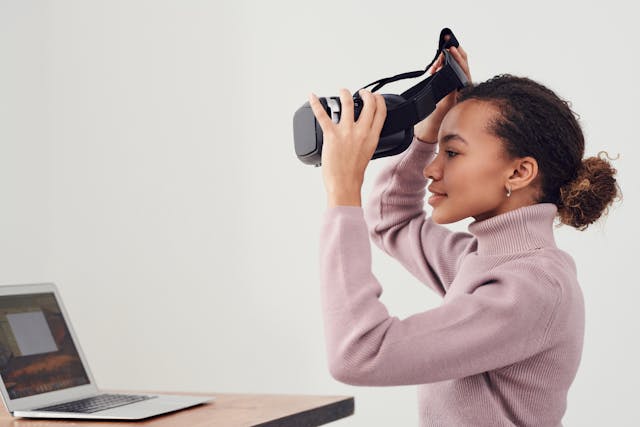
For a data scientist to succeed, soft skills are essential. Since dealing with varied team members is a common part of data science, effective collaboration within teams requires strong communication skills. Effective problem-solving skills are essential for taking on challenging data problems and producing insightful conclusions. Two essential characteristics that spur creativity and keep data scientists abreast of the newest developments in their industry are a curious attitude and a love for lifelong learning. Gaining proficiency in these soft skills can significantly improve a data scientist's overall effect and career advancement in this dynamic field.
7. Experience with Data Wrangling
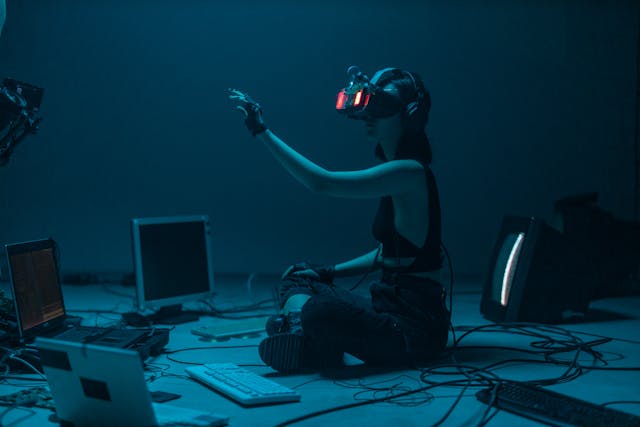
For any aspirant data scientist, the ability to manipulate data is essential. To prepare untidy datasets for analysis, one must be able to clean and arrange them. Managing outliers, inconsistent data, and missing numbers are frequently steps in this procedure. For effective data manipulation and reshaping, a thorough understanding of tools like SQL for database querying and Pandas for Python is necessary.
Data scientists that are proficient with Pandas can handle a variety of data manipulation tasks, including grouping, filtering, sorting, and aggregating data sets. Data scientists that are proficient in SQL can write queries that filter, join, and transform data straight from databases, allowing them to extract insightful information. Combining these abilities enables data scientists to create reliable datasets that are the basis for precise modeling and analysis in a range of initiatives.
In addition to technical know-how, data wrangling calls for critical thinking and problem-solving skills. Effectively navigating complex datasets is crucial for data scientists in order to identify patterns and relationships that lead to significant findings. Proficiency in data cleansing and transformation provides professionals with the necessary groundwork to address practical issues in several industries where decision-making relies heavily on structured information.
To sum up what I've written so far, prospective data scientists should have expertise with data wrangling as a core ability. The capacity to efficiently extract insightful information from raw data requires the ability to clean up complicated datasets using tools like Pandas or SQL. By developing these qualities, people can become more proficient analysts, expedite the process of preparing data, and eventually succeed in the exciting subject of data science.
8. Familiarity with Data Mining Techniques
Aspiring data scientists must be familiar with data mining techniques. To properly analyze data, this entails knowing and applying techniques like clustering, classification, and regression. Using these methods, data scientists can detect patterns and trends in datasets, which helps them to draw insightful conclusions and make decisions based on the information. Proficiency in data mining techniques and algorithms enables practitioners to reveal latent links inside intricate datasets, so making a valuable contribution to data-driven strategies and solutions.
9. Mastery of Data Modeling
Any aspirant data scientist must be proficient in data modeling. This ability entails creating and refining predictive models in order to efficiently extract insights from data. It is essential to comprehend different modeling strategies, including decision trees, neural networks, and linear regression. In addition, a data scientist needs to know how to assess the accuracy and performance of models using metrics such as F1 score, precision, and recall. A data scientist who is skilled in data modeling may build reliable models that offer insightful information for wise business decisions.
10. Expertise in Machine Learning Applications
Being knowledgeable with a variety of machine learning applications is essential for success as a data scientist. Gaining an understanding of techniques like Support Vector Machines (SVMs) and decision trees is crucial to maximizing their potential for efficient analysis of complicated datasets. Gaining proficiency in cross-validation methods for model validation guarantees predictive models' accuracy and dependability. In the discipline of data science, these abilities are essential for creating solid solutions and making defensible choices based on data-driven insights.
11. Specialization in Deep Learning
Deep learning specialization is essential for aspiring data scientists. Understanding neural network topologies and frameworks, such as TensorFlow, which are essential tools in the field, is necessary for this. Deep learning is an important skill set to master for people entering the field of data science because of its many applications, including image identification and natural language processing (NLP). Individuals can become experts in developing sophisticated models and resolving challenging issues in the fields of machine learning and artificial intelligence by concentrating on this specialization.
12. Practical Project Experience
Extensive hands-on project experience is essential for prospective data scientists. Present practical projects on sites such as GitHub to prospective employers to showcase your abilities. By using these projects to put your problem-solving abilities to use, you will not only improve your comprehension but also get real-world experience that will distinguish you as a data scientist. In the data science industry, placing a strong emphasis on practical project work can significantly increase your reputation and marketability.