1. Introduction
Introduction: Data fusion is the process of integrating multiple data sources to produce more consistent, accurate, and useful information than that provided by any individual source. In today's data-driven world, where vast amounts of data are generated every second, data fusion plays a crucial role in extracting meaningful insights and making informed decisions. By combining diverse datasets from various sources such as sensors, databases, and social media platforms, organizations can enhance their understanding of complex phenomena and derive deeper insights that would be unattainable through individual datasets alone.
2. Types of Data Fusion
When merging data from several sources to enhance decision-making and derive more precise insights, data fusion plays a critical role. Sensor level fusion, feature level fusion, and decision level fusion are the three primary categories of data fusion techniques.
1. Sensor level fusion is the process of combining unprocessed data from several sensors to produce a larger, more trustworthy dataset. The resulting dataset provides a more complete picture of the environment or system under observation by combining data from many sensors, such as cameras or temperature gauges.
2. Merging features or attributes that have been derived from separate datasets is the main goal of feature level fusion. By merging certain traits or patterns found in several datasets, this technique seeks to improve the quality and richness of the data. Applications where merging different characteristics can yield a more comprehensive view of the underlying data can benefit from feature-level fusion.
3. Decision level fusion is the process of combining choices or results that were separately produced by various algorithms or datasets. The goal of decision-level fusion is to create a cohesive and more reliable final result by combining decisions from many sources. This approach is especially useful in situations where a group decision needs to be made by combining different outcomes.
Effective use of these data fusion techniques can result in increased precision, dependability, and overall efficiency in a number of industries, including healthcare analytics, autonomous cars, surveillance systems, and more.
3. Challenges in Data Fusion
In today's data-driven world, data fusion—the process of merging data from several sources to provide more precise, thorough, and trustworthy information—is a potent instrument. Nonetheless, a number of obstacles may prevent it from working well.
First and foremost, problems with data quality are a major obstacle to effective data fusion. Inconsistencies in timeliness, precision, completeness, and consistency amongst several datasets may compromise the fused data's overall dependability. It is imperative to guarantee that the input data satisfies specific quality requirements in order for data fusion techniques to yield significant and practical insights.
Second, data fusion initiatives become more complex due to the heterogeneity of data sources. Integrating and aligning information from multiple sources can be difficult since data can have different forms, structures, and schemas. Before fusion can occur, this variability necessitates the use of complex normalization, transformation, and correlation procedures to harmonize the data.
Real-time processing and scalability pose major challenges to the implementation of effective data fusion systems. Conventional technologies may not be able to process the sheer amount of data in a timely manner as it continues to rise dramatically. These difficulties are exacerbated by the need for real-time processing, which calls for quick decisions and answers based on fused data that is current.
Organizations can use a number of tactics and best practices to get beyond these data fusion obstacles and get better results. Addressing problems with data quality at the source can be facilitated by putting in place strong data governance structures that give priority to quality assurance procedures. The reliability of input datasets for fusion activities can be improved by organizations by setting explicit criteria for data collection, storage, and maintenance.
In data fusion initiatives, adopting standards-based methods to manage diverse data sources can simplify integration efforts. Common protocols, ontologies, or mapping techniques can help different datasets work together more easily and produce more accurate results by streamlining the merging process.
Applying cutting-edge technology to data fusion applications can increase scalability and offer real-time capabilities, such as distributed processing frameworks or cloud computing platforms. In order to fulfill stringent processing time limitations and effectively handle massive volumes of different datasets, these contemporary infrastructures offer increased computational power and flexibility.
From the above, we can conclude that
addressing challenges like
data quality issues,
heterogeneity of sources,
and scalability concerns
is essential for successful
data fusion implementation.
By adopting effective strategies
and leveraging advanced technologies,😎
organizations can unlock the full potential
of integrated datasets
to drive informed decision-making
and gain valuable insights into complex problems.🤩
4. Techniques for Data Fusion
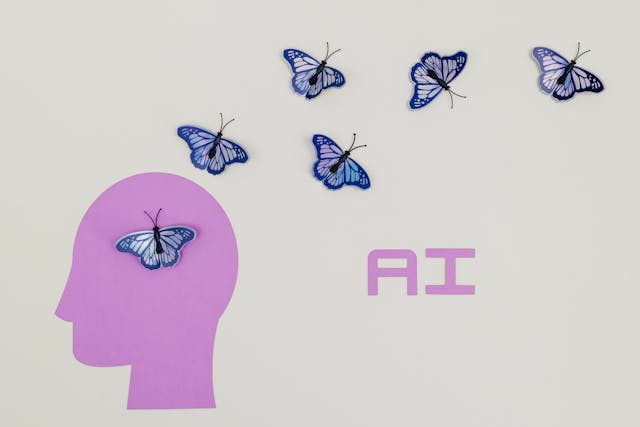
In the field of data fusion, a number of strategies are essential for combining different data sources to derive meaningful information. When merging data from many sources, Bayesian approaches offer a reliable framework that takes uncertainty and previous knowledge into account. Enhancing the accuracy of fused data, Bayesian techniques capture the probabilistic correlations between data sets.
Because machine learning algorithms, such neural networks, can recognize intricate patterns and relationships in diverse data, they provide effective tools for data fusion. Neural networks are capable of integrating different kinds of data efficiently, allowing for complex fusion from several sources. They can handle massive amounts of data in real-time applications thanks to their scalability and agility, which speeds up the fusion process.
Integrating machine learning algorithms with Bayesian approaches offers a viable way to tackle data fusion problems in a comprehensive way. Through the combination of neural network predictive power and Bayesian approaches' probabilistic reasoning, a synergistic effect is realized that improves fused data output efficiency and accuracy. From sensor networks to healthcare systems, this integrated methodology opens the door to overcoming challenging fusion issues in a variety of domains.📗
5. Applications of Data Fusion
Applications such as surveillance systems and medical diagnosis and treatment depend heavily on data fusion. In order to improve situational awareness and decision-making capabilities, data fusion is used in surveillance systems to merge information from many sources, including sensors, cameras, and databases. Through the integration of data from various sensors, such as motion detectors and video feeds, the system can offer security operations more thorough insights.
Data fusion makes it possible for medical practitioners to examine several sets of patient data in order to diagnose patients more accurately and create individualized treatment plans for them. A more comprehensive understanding of a patient's health status can be obtained by medical professionals by combining patient history, test findings, imaging scans, and genetic data. By customizing interventions to meet the needs of each patient, this all-encompassing strategy improves treatment results and increases diagnostic accuracy.
By combining the combined knowledge of several data sources, data fusion strengthens sectors such as healthcare and surveillance systems, facilitating improved decision-making and producing better results for all parties.
6. Importance of Accuracy in Data Fusion
Accuracy in data fusion is paramount as it directly influences decision-making processes. When disparate data sets are fused together, the accuracy of the final output determines the quality and reliability of insights extracted. Inaccurate data can lead to erroneous conclusions and poor decisions, potentially affecting business strategies, resource allocation, and overall performance. Ensuring high accuracy in data fusion involves addressing challenges such as data quality, consistency, and relevance across sources. By maintaining accurate fusion processes, organizations can enhance the credibility of their analytics, leading to more informed decisions and ultimately better outcomes. Accurate data fusion promotes trust among stakeholders and helps mitigate risks associated with faulty information.
7. The Role of AI in Enhancing Data Fusion
The application of AI, especially deep learning, has completely changed the way we examine intricate patterns in datasets when it comes to data fusion. Deep learning algorithms have demonstrated remarkable proficiency in identifying complex patterns in large volumes of data that would be practically unfeasible for conventional algorithms to handle efficiently. Deep learning models are highly adept at recognizing and deriving significant insights from a wide range of data sources by utilizing multi-layered neural networks that replicate the architecture of the human brain.
Convolutional neural networks (CNNs) and recurrent neural networks (RNNs) are two deep learning approaches that AI can use to improve data fusion. These techniques combine different forms of data, such as text, photos, and sensor readings. Due to their ability to recognize characteristics at many levels of abstraction, CNNs are very effective at picture recognition tasks. However, RNNs are great at processing time series and textual data because of their long-term memory retention, which makes them perfect for sequential data analysis.
Organizations can obtain deeper insights from their aggregated datasets and more accurate outputs by integrating AI-driven techniques into their data fusion processes. These technologies enable predictive analytics by projecting future trends based on past patterns, in addition to aiding in the discovery of hidden relationships. The integration of artificial intelligence (AI) is expected to remain crucial in augmenting data fusion skills across many businesses and disciplines as we progress deeper into the big data era.
8. Ethical Considerations in Data Fusion
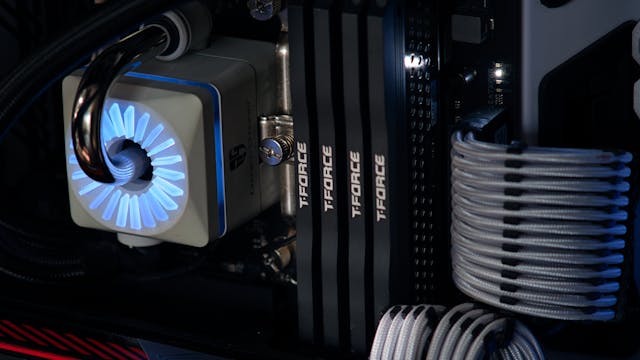
Ethical considerations are critical when it comes to data fusion. Data security and privacy issues are at the center of these conversations. Combining data from many sources—some of which may contain sensitive information about specific people or organizations—is a common practice in data fusion. To preserve confidence and safeguard privacy, it is imperative to guarantee that this data is managed appropriately and securely. 😎
Ensuring the protection of personally identifiable information (PII) during the data fusion process is a significant ethical concern. When disparate databases are combined, there's a chance that ostensibly anonymous data pieces could be combined to reveal individual identities. This underscores the necessity for strict data protection measures and presents a serious danger to privacy.
Another crucial component of ethical considerations in data fusion is data security. It is essential to protect merged data from malicious intent or unauthorized access given the rise in cyberattacks and data breaches. Mitigating the dangers related to storing and processing integrated information can be achieved by the implementation of strong encryption mechanisms, access controls, and frequent security audits.
Integrating data security and addressing privacy issues are critical elements of moral data fusion procedures. Organizations can utilize the benefits of integrated datasets while keeping ethical standards and protecting individuals' privacy rights by giving priority to these factors and putting in place suitable protections.
9. Future Trends in Data Fusion
Integrating data fusion with the Internet of Things (IoT) is where the technology is headed. The sheer amount and diversity of data that IoT devices produce as they continue to infiltrate more areas of our life present both opportunities and difficulties for data fusion. Organizations can obtain a deeper understanding of patterns and trends by fusing data from IoT devices with information from other sources, like social media, weather reports, and more conventional databases.
New opportunities for improving decision-making processes across industries are created by this integration. IoT sensors, for instance, can gather information on crop health, temperature, and soil moisture content in agriculture. Farmers may make better educated judgments about irrigation schedules, pest management methods, and yield optimization tactics by combining this data with weather forecasts and satellite photos.
But there are also a lot of difficulties in integrating data from IoT devices. To enable the successful fusion of various data streams, concerns about data privacy, security, interoperability, and scalability must be carefully considered. Cutting-edge technology like edge computing and machine learning techniques will be needed to manage and process this tsunami of data in real-time as the number of connected devices keeps growing exponentially.
Based on the aforementioned, it is possible that the combination of data fusion and the Internet of Things may completely change how businesses derive value from their data assets. Businesses may use this comprehensive strategy to promote innovation in a variety of applications and generate new insights by addressing privacy issues and overcoming technical obstacles. Staying successful in the digital era will require mastering the art of integrating disparate datasets as we progress towards a more linked world powered by data-driven decision-making processes.
10. Case Studies: Successful Implementations of Data Fusion
Data fusion plays a critical role in the safe and effective navigation of roadways by autonomous cars. These cars are able to make well-informed decisions in real time by combining data from multiple sensors, including GPS, LIDAR, radar, and cameras. One well-known example of a data fusion system used successfully in autonomous driving technology is Tesla's Autopilot. It makes use of sensor data to enable functions like automatic lane changes, lane-keeping assistance, and adaptive cruise control.
Another example of a company that effectively demonstrates data fusion in autonomous vehicles is Waymo, an Alphabet Inc. affiliate. Waymo's autonomous vehicles use high-definition maps and sensor data to better understand their surroundings. The vehicles are able to precisely predict and respond to possible road hazards because to this comprehensive information fusion. This integration has improved the safety and dependability of autonomous cars and cleared the path for developments in driverless technology.
11. Implementing a Robust Data Fusion Strategy
Implementing a robust data fusion strategy requires careful planning and execution to ensure reliable and accurate results. Here are some essential steps to consider:
1. **Explain Goals:** Give a clear description of the aims and purposes of your data fusion project. Recognize your objectives and how data fusion fits into your larger research or business objectives.
2. **Information Gathering:** Collect pertinent information from a variety of sources that can offer in-depth understanding of the issue at hand. Make that the data is well-formatted, consistent, and of the highest quality for integration.
3. **Data Preprocessing**: To address missing values, outliers, duplicates, and inconsistencies, clean up and preprocess the gathered data. For smooth integration, standardize data formats and units.
4. **Feature Selection:** Choose pertinent characteristics from several datasets that make a significant contribution to the fusion procedure. To design new informative features, think about employing methodologies such as feature engineering.
5. **Choose Fusion Methods:** Select appropriate fusion algorithms based on the nature of the data sources (e.g., sensor data, textual data) and the desired output format (e.g., decision-making, visualization).
6. **Integration Process:** Integrate the selected fusion methods into a cohesive framework that combines outputs from individual sources effectively while preserving important information.
7. **Verification and Assessment:** To evaluate the correctness and dependability of the fused data, validate it against known outcomes or ground truth. Make use of domain-specific performance measurements, recall, F1 score, and precision.
8. **Iterative Improvement:** Keep improving your fusion plan in response to stakeholder input and validation outcomes. For optimal results, be willing to experiment with different approaches or changing the parameters.
9. **Scalability Considerations:** Design your fusion strategy with scalability in mind to handle increasing volumes of data efficiently as your project grows.
10. **Robustness Testing:** Conduct stress tests and sensitivity analysis to evaluate how well your fusion strategy performs under varying conditions, noisy inputs, or unexpected scenarios.
You may put into practice a strong data fusion strategy that yields dependable insights and accurate results for improved decision-making and problem-solving skills by carefully following these procedures and customizing them to your unique needs.
12. Conclusion
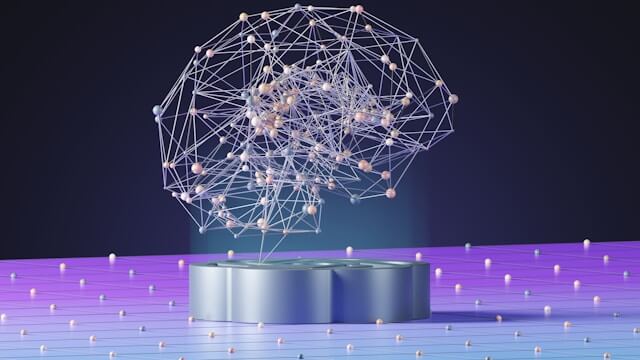
To put what I've said above into brief, data fusion is essential for combining information from many sources to create a more thorough and precise knowledge of complex systems. We have emphasized the difficulties in data fusion throughout this conversation, including data heterogeneity, uncertainty, and scalability. These challenges may make data fusion procedures less successful and result in inaccurate decision-making.
The importance of overcoming these obstacles for effective data fusion cannot be overstated. Organizations can improve the dependability and applicability of fused data outputs by addressing problems with data quality, interoperability, and integration techniques. By overcoming these challenges, organizations and sectors will be able to extract useful insights from their data assets and enhance decision-making procedures.
Prioritizing data consistency, quality, and compatibility across various datasets is essential for effective data fusion. Organizations can fully utilize data fusion approaches and obtain a competitive advantage in today's data-driven economy by investing in strong infrastructure, cutting-edge analytics tools, and interdisciplinary collaboration. By adopting cutting-edge approaches and industry best practices for data fusion, companies may leverage the potential of information convergence to boost productivity and promote long-term expansion.