1. Introduction
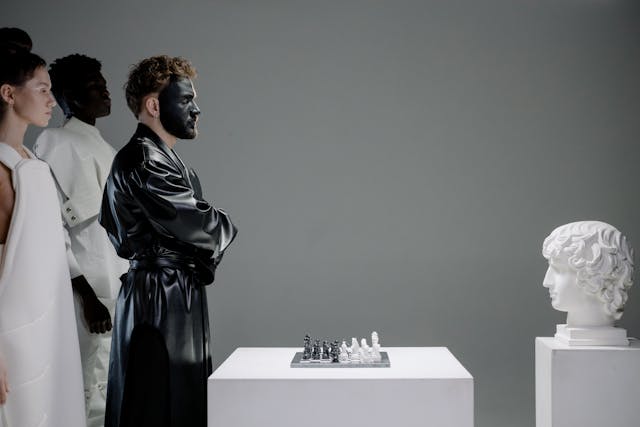
Introduction: In today's digital age, Data Science has emerged as a powerful tool driving innovation and transformation across various industries. It involves the use of scientific methods, algorithms, and systems to extract insights and knowledge from data in various forms. The applications of Data Science have been revolutionary in disrupting traditional business practices by enabling companies to make informed decisions, predict trends, personalize customer experiences, optimize operations, and much more.
Real-world Impact:
Data science is having a real-world impact on a number of industries, including marketing, banking, e-commerce, and healthcare. To obtain a competitive advantage, businesses are utilizing data science approaches such as big data processing, predictive analytics, and machine learning. Utilizing Data Science is changing the way businesses run and compete in the market. Examples of these applications include recommendation systems that improve customer interaction and predictive maintenance that lowers downtime in manufacturing plants.✍️
2. Predictive Analytics in Financial Services
Data science has become an essential tool for banks and other financial organizations to forecast client behavior in the constantly changing financial services industry. These organizations may use enormous amounts of data to predict client demands, preferences, and possible hazards by utilizing predictive analytics. They may more efficiently modify their products and services, reduce risks, and boost consumer happiness thanks to this proactive strategy.
In the financial sector, one noteworthy application of predictive analytics is the way banks anticipate creditworthiness using machine learning algorithms. To determine the likelihood that loan applicants would default, these algorithms examine market patterns, previous transactional data, and a variety of risk factors. Financial organizations can reduce possible losses and make better lending decisions by accurately anticipating credit risk.
How insurance companies use predictive analytics to tailor rates based on individual risk profiles is another interesting case study. Insurers can forecast the possibility of a policyholder submitting a claim by looking at lifestyle characteristics, claim history, and demographic data. Insurance companies are able to more competitively price policies and customize coverage for particular client segments because to this detailed insight.
Predictive analytics is being used by wealth management companies to provide clients with individualized investment suggestions. These companies are able to create customized portfolios that match the risk tolerance and financial goals of each client by examining market data, economic indicators, and individual investing goals. Over time, this proactive approach improves investment performance while also increasing client pleasure. 📚
Financial services have undergone a transformation thanks to predictive analytics, which provides organizations with useful information based on data-driven decision-making. We may anticipate even more advancements in this area as technology develops, which will improve client experiences and significantly streamline business processes in the finance sector.
3. Personalized Marketing through Machine Learning
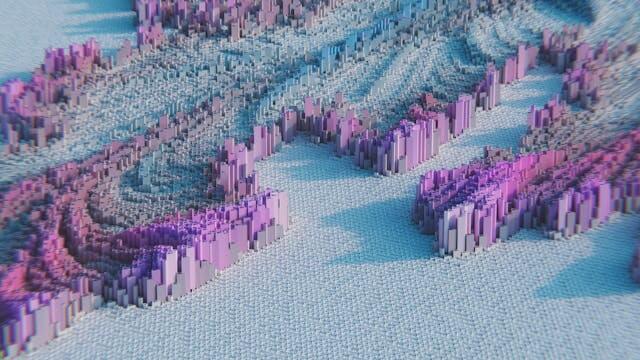
Personalized marketing has emerged as a key component of profitable business plans in the current digital era. By utilizing machine learning algorithms, businesses may gain remarkable precision in customizing marketing efforts by delving deeply into client data. Through extensive data analysis on consumer behavior, tastes, and demographics, companies are able to provide highly targeted advertising that speaks directly to specific customers.
Businesses can now precisely anticipate the demands and behaviors of their customers thanks to machine learning, which enables them to provide tailored messages and offers at the ideal time. Businesses may precisely segment their consumer base using sophisticated algorithms, which guarantees that every campaign reaches the most responsive group. This focused strategy increases conversion rates and improves customer engagement by providing pertinent material that is in line with each user's interests.
The potency of machine learning-powered personalized marketing is in its capacity to cultivate significant relationships between consumers and companies. Companies are able to create customized communications that speak directly to the wants and desires of each individual by knowing the preferences and purchase history of their customers. This degree of customization fosters long-term partnerships and loyalty in addition to increasing consumer happiness.
Businesses are able to optimize their marketing campaigns in real time thanks to data-driven insights obtained by machine learning algorithms. Through ongoing analysis of customer feedback and engagement analytics, companies can optimize their campaigns for optimal impact and return on investment. With a dynamic approach to marketing, businesses can quickly adjust to shifting consumer preferences and market trends, giving them an advantage over rivals in the quickly changing business environment of today.
To sum up what I've written so far, machine learning-powered personalized marketing transforms the way companies interact with their clients by creating customized experiences that produce outcomes. Companies may develop engaging ads that connect with people on a personal level by utilizing data science to gain a comprehensive understanding of customer behavior. Businesses can enhance the efficacy of their marketing endeavors and establish enduring consumer relationships in a progressively cutthroat market by means of data-driven customized advertising.
4. Supply Chain Optimization with Big Data
The incorporation of big data analytics has significantly transformed supply chain optimization. Businesses can now closely monitor and evaluate a variety of components of their supply chains like never before by utilizing the power of data science. This results in better forecasting accuracy, better decision-making procedures, and eventually higher operational efficiency.
Predictive analytics is one significant way big data is changing supply chain management. Businesses may predict demand variations, optimize delivery routes, and expedite inventory management by evaluating historical data and real-time information. Businesses can decrease stockouts, cut costs associated with extra inventory, and improve overall customer satisfaction by using this proactive approach.
The well-known apparel store Zara is a great illustration of how big data improves supply chain efficiency. Zara uses data analytics to effectively monitor inventory levels, forecast trends, and drastically reduce lead times. Zara is able to maintain high profitability in a fast-paced sector by responding promptly to market demands, reducing markdowns caused by overstocking, and using a data-driven strategy. 📘
5. Healthcare Transformation with AI
Healthcare is undergoing a transformation thanks to the use of AI, which is improving services and patient care. AI can detect possible diseases through predictive diagnosis, allowing for early intervention and patient-specific treatment options. Healthcare workers can now effectively evaluate large volumes of medical data thanks to data science, which produces more precise diagnoses and individualized treatments. AI-powered remote monitoring makes it possible to track patients' health problems continuously and in real time, improving preventive treatment and lowering readmission rates to hospitals. A more proactive and individualized approach to medicine is being made possible by the revolutionary effects of data science in the healthcare industry, which will eventually improve patient outcomes and quality of life.
6. Smart Cities and IoT Integration
The way we approach urban planning and municipal administration is changing dramatically in today's constantly changing digital ecosystem because to the integration of Internet of Things (IoT) devices with data analytics. Thanks to creative data science applications in practical settings, the idea of smart cities is starting to take shape. Through leveraging the potential of Internet of Things (IoT) devices to gather massive volumes of data from diverse sources such as sensors, cameras, and other networked devices, cities may get important information for more sustainable and productive operations.
Urban planning is one innovative use of data science in smart cities. City planners may create more livable and effective urban environments by utilizing data analysis on environmental elements, infrastructure utilization, traffic patterns, and population density. Data-driven urban planning promotes resource efficiency, enhances public services, and raises citizens' standard of living in general.
Another field that stands to gain a great deal from the combination of data analytics and IoT devices is traffic management. Local government agencies can monitor traffic flow, identify areas of congestion, and deploy adaptive traffic control systems by using real-time data gathered from sensors integrated into roadways, traffic signals, and automobiles. Cities may proactively manage traffic conditions to minimize travel time, lessen congestion, and improve road safety by utilizing predictive analytics algorithms.
In smart cities, data-driven solutions are also revolutionizing resource optimization. City governments may ensure efficient use and sustainability of resources by examining demand trends and patterns for utilities like electricity and water. By using these insights, preemptive steps can be taken to deal with shortages or inefficiencies before they become more serious problems.
Global case studies highlight the observable advantages of using data science in practical smart city projects. For example, Barcelona has put in place a sophisticated IoT infrastructure along with extensive data analytics to keep an eye on the city's air quality. Targeted initiatives to lower pollution levels and enhance public health outcomes have resulted from this project.
Another prominent example of the usage of IoT devices for a variety of purposes is Singapore. Here, waste management systems that optimize collection routes based on bin fill-level sensors and smart lighting systems that modify brightness based on real-time conditions are just two examples. In addition to improving operational effectiveness, these technologies help Singapore achieve its objective of developing into a resilient and sustainable smart city.
Smarter and more sustainable areas are emerging from the confluence of IoT devices with powerful data analytics capabilities, revolutionizing traditional urban landscapes. Global cities are seeing increased productivity, better services, and a higher standard of living for their citizens due to data-driven solutions in the areas of traffic management, urban planning, and resource optimization. The data-driven smart city era has arrived, bringing with it new opportunities for urban development and a more promising future for future generations.
7. Enhancing Customer Experience with Sentiment Analysis
Sentiment analysis is a game-changer for companies looking to better understand their customers and improve the customer experience. Businesses can assess customer satisfaction levels by employing sentiment analysis tools to examine feedback from a variety of sources, including surveys, social media posts, and reviews. Algorithms for natural language processing are used in this process to categorize text data attitudes as neutral, negative, or positive.
Through data insights from sentiment analysis, customer input plays a critical role in shaping product development and service enhancements. Businesses can better satisfy customer expectations by pinpointing areas for improvement in their services and customizing their strategy based on trends in customer opinion. By resolving problems before they get worse, this proactive strategy not only increases customer happiness but also aids businesses in staying one step ahead of the competition.
By showing a sincere interest in their customers' thoughts and preferences, businesses can strengthen their ties with them by incorporating sentiment research into their daily operations. Long-term brand loyalty and advocacy are increased as well as the entire consumer experience through the use of data-driven strategies.🤨
8. Fraud Detection in E-commerce Using Data Science
One essential use of data science in e-commerce is fraud detection, which helps companies protect themselves from fraudulent activity. E-commerce systems are able to recognize any fraud attempts by analyzing trends and behaviors through the use of sophisticated algorithms and machine learning techniques. Predictive models are essential for avoiding financial losses and preserving client confidence.
Empirical instances demonstrate the efficacy of data science in countering fraudulent activities. For example, businesses such as PayPal employ advanced algorithms to identify anomalous buying patterns and immediately report transactions that appear suspect. These systems can quickly distinguish between genuine and fraudulent transactions by processing large volumes of data, which lowers risks for both consumers and businesses.
Data science technologies are always evolving, which guarantees that e-commerce platforms are always one step ahead of hackers. By means of proactive monitoring and analysis of transactional data, enterprises can augment their security protocols and provide a more secure virtual marketplace for all users.
9. Automation and Optimization in Manufacturing Industries
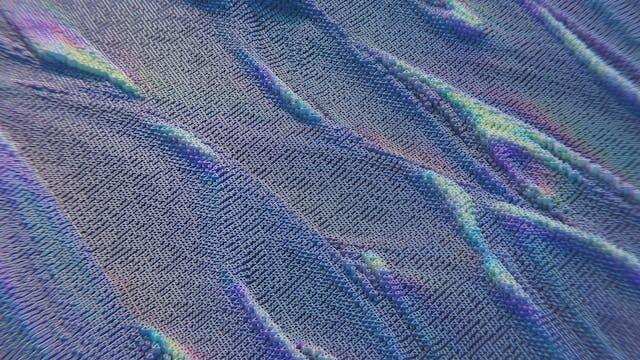
Data science is essential to the transformation of industrial businesses because it makes operations more efficient. Manufacturers can get insights into their operations through data analysis, which can result in more efficient workflows and increased production. One important use of data science is predictive maintenance, which uses analytics to predict equipment problems before they arise and drastically lowers maintenance costs and downtime. Advanced analytics helps quality control by enabling the real-time detection of deviations or flaws, guaranteeing that only top-notch goods are sent onto the market. For manufacturing organizations, these deployments result in increased efficiency and cost savings.
10. Sustainable Practices Powered by Data Science
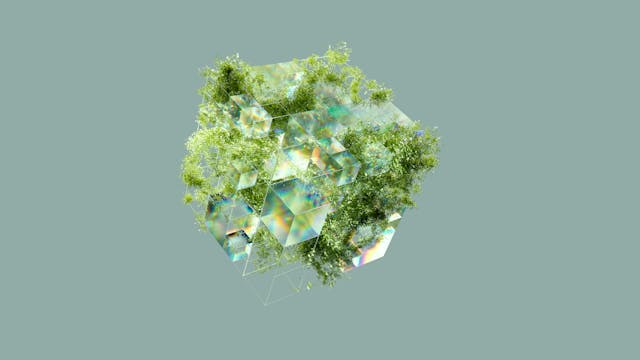
Environmental sustainability is a major issue in the modern world that requires creative solutions. Industry-wide, data science has shown to be a potent instrument for advancing sustainable practices. Businesses can maximize resource use and minimize their carbon footprint by making well-informed decisions through the use of data-driven initiatives. 🤩
In this procedure, predictive modeling is essential because it forecasts patterns and trends using historical data. Businesses can use these data to put in place more effective procedures, including optimizing energy use or cutting down on waste production. Predictive models, for instance, can assist companies in anticipating periods of high energy consumption and modifying their operations accordingly to reduce energy waste.
Businesses can track and evaluate environmental impact measures in real-time thanks to data science, which enables them to make proactive decisions toward sustainability objectives. Businesses can reduce costs and improve their environmental performance while also increasing operational efficiency and driving cost savings by utilizing data. A greener and more sustainable future is being paved with data science, which is genuinely transforming how businesses approach sustainability.
11. Education Revolution through Learning Analytics
Through learning analytics, data science has revolutionized the field of education. Through the examination of student performance data, learning analytics provides educators with important information that enhances learning outcomes. These insights might be anything from recognizing kids who are at risk and may require further support to identifying areas in which instructional strategies may need to be modified.
The creation of adaptive learning platforms is one of the most significant developments in education brought about by data science. These platforms use algorithms to customize learning programs for each student according to their learning preferences, areas of strength, and areas of weakness. This customized strategy not only raises student engagement but also dramatically raises retention and comprehension rates.
In contemporary educational environments, personalized education strategies—made feasible by data science tools—are becoming more and more common. Teachers may now tailor lessons, offer focused interventions, and provide extra resources based on data-driven insights to meet the individual requirements of every student. These data science applications are improving teaching strategies and producing improved student outcomes, which is changing the face of education.
12. Future Prospects: Emerging Trends in Disruptive Data Applications
Looking ahead to the future of disruptive data applications, a number of new trends are poised to completely transform a wide range of industries. Faster computations and advances in disciplines like artificial intelligence and optimization are made possible by quantum computing, which possesses unmatched processing capacity and can handle complicated information. This opens up new opportunities for data science applications.
As more businesses look for real-time insights from data gathered at the edge of their networks, edge analytics is becoming more popular. Businesses can quickly obtain insightful information from data processed closer to the source, which improves decision-making and operational effectiveness.
The combination of data science and blockchain technology presents another fascinating possibility. Blockchain's built-in security features and transparency have the potential to improve data reliability and integrity, revolutionizing sectors including finance, healthcare, and supply chain management. The amalgamation of blockchain technology and data science presents a vast opportunity for optimizing procedures and fostering confidence in data exchanges.
These developments could influence the future terrain of disruptive data applications, providing companies with cutting-edge tools and strategies to spur expansion and maintain an advantage in a cutthroat market. Watch this space as these technologies continue to transform the way we use data to drive meaningful business outcomes.🗯