1. Introduction
In the field of big data analysis, enormous volumes of intricate data containing insightful information are encountered by both specialists and businesses. This enormous amount of data might be intimidating and time-consuming to analyze. For organizations to draw insightful conclusions, make wise decisions, and obtain a competitive edge in the fast-moving market of today, efficient data analysis is essential.
Large datasets are extracted, cleaned, transformed, and interpreted in order to identify patterns, trends, and relationships. This is the fundamental process of big data analysis. It is impossible to overestimate the importance of effective data analysis since it helps businesses to improve customer experiences, anticipate emerging trends, streamline operations, and spur innovation. The capacity to effectively analyze big data helps businesses stand out from the competition in a world where data is being generated at an unprecedented rate. It also gives professionals the ability to find actionable insights that pave the way for success.
2. Understanding the Basics of Big Data Analysis
Understanding the Basics of Big Data Analysis
Large volumes of structured and unstructured data that cannot be handled by conventional database and software processing methods are referred to as "big data." The volume, velocity, and variety of this data make it necessary to analyze it using certain tools and techniques. vast data essentially refers to datasets that are too vast to be handled by traditional technologies.
Big data analysis usually entails the following essential steps: gathering, cleaning, storing, analyzing, and interpreting data. Data collection is the process of compiling information from a variety of sources, including sensors, social media platforms, and transaction records. The gathered data is then cleaned to get rid of mistakes and inconsistencies that could interfere with a precise analysis. Following cleaning, the data is placed in a repository for convenient access during the analysis stage. Analyzing a dataset involves using statistical models and algorithms to extract insights that are then evaluated to help in decision-making.
Big data analysis novices frequently encounter similar difficulties, including managing large datasets, guaranteeing data accuracy and quality, and choosing the right analytical tools. The sheer amount of data might be overwhelming for novices, who could find it difficult to properly absorb and interpret it. It can be difficult to maintain the data's quality and correctness throughout the analysis process if appropriate validation approaches aren't used. Another challenge faced by novices in the field of big data analysis is selecting the appropriate analytical tools that align with the dataset and goals, given the wide range of possibilities available and their ongoing evolution.
3. Tip 1: Define Clear Objectives and Questions
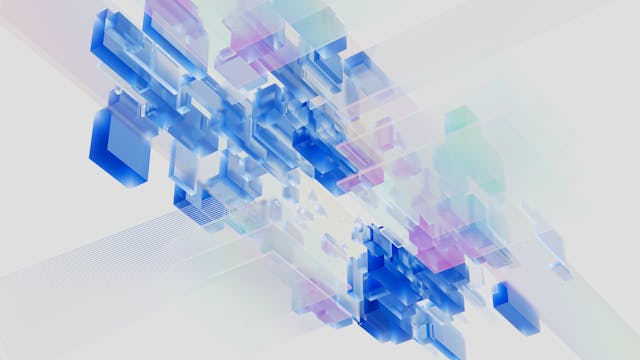
Tip 1: Define Clear Objectives and Questions
Having well-defined goals is essential for doing effective data analysis. Clearly defining your analytical goals is crucial before you go into large datasets. Setting clear objectives gives you a road plan to maintain the effectiveness and focus of your investigation. Having specific goals guarantees that your efforts are focused and effective, whether you're trying to forecast market trends, understand consumer behavior, or increase operational efficiency.
Setting goals also helps to guide the path of your analysis procedure. It aids in deciding which metrics to measure, which data points to consider meaningful, and which analytical methods to use. You may improve the quality of insights obtained from the data and save time and money by streamlining the entire analysis pipeline by defining clear goals that help you limit down the scope.
Formulating clear objectives requires crafting well-crafted questions. Your analysis will be guided toward actionable information by asking questions like "What factors impact sales performance?" or "How does user engagement correlate with revenue?" These inquiries direct your data investigation, encouraging you to find patterns, connections, and trends that support your main goals. Asking insightful questions not only leads to insightful findings but also guarantees that your analysis stays focused and in line with corporate objectives.
4. Tip 2: Choose the Right Tools and Techniques
Choosing the appropriate tools and approaches is essential when diving into large data analysis projects. Python, R, SQL, and machine learning algorithms are examples of common tools. These materials cover a wide range of data analysis and manipulation topics. Take project objectives, complexity, and data volume into account while selecting tools. Making decisions according to these criteria guarantees effective processing and analysis. Use online tutorials, courses, and practice datasets to gain a thorough understanding of these technologies. Gaining proficiency with big data analysis tools is essential for producing effective results. This requires constant learning and practice.
5. Tip 3: Prioritize Data Quality and Cleaning
Tip 3: Prioritize Data Quality and Cleaning
For reliable analytical results, data quality is essential. Reliable insights are built on a foundation of clean, high-quality data. Making sure your data is error-, inconsistency-, and accuracy-free is essential to getting trustworthy results from your research.
To get the data ready for analysis, there are multiple processes in the data cleaning process. This entails eliminating duplicates, dealing with null values, fixing mistakes, and standardizing forms. Early resolution of these problems paves the path for more efficient and accurate analysis procedures.😀
Best practices include thoroughly inspecting the dataset for abnormalities, outliers, and inconsistencies in order to guarantee data quality prior to analysis. Using automated techniques, profiling data, and putting validation criteria into practice can all assist find problems and fix them quickly. Transparency and reproducibility in your analysis also depend on routinely auditing and recording your data cleaning procedures.
6. Implementing Best Practices for Efficient Data Analysis
It is essential to summarize the most important lessons learned from the previous advice in order to use best practices for effective data analysis. Analysts can efficiently expedite their processes by employing proper tools, understanding the significance of structuring data, and ensuring data quality.
To improve analytical skills, practicing consistently is required. Frequent participation in data analysis assignments enhances one's skills and promotes a more profound comprehension of various datasets and analytical methodologies. Constant practice makes decision-making and efficiency in data analysis better.
It is advised that aspiring data analysts look for more materials and educational opportunities to broaden their knowledge. Attending industry events, workshops, or online courses can offer analysts important insights and keep them up to date on the newest developments in big data analysis trends and technology. Devoting time to continuous education programs enhances one's professional development and data analysis proficiency.
7. Conclusion
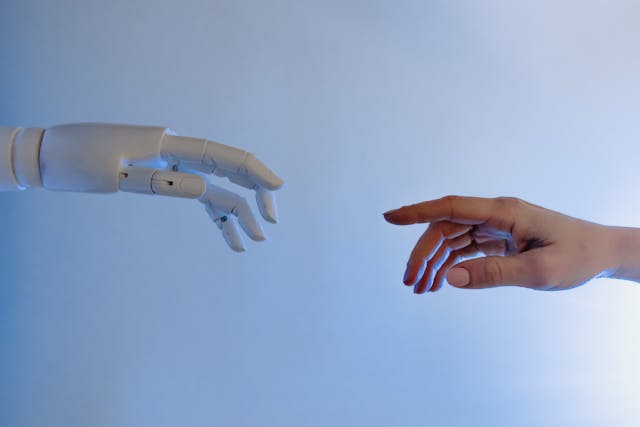
We can infer from the foregoing that effective big data analysis is essential for producing insightful findings and wise decision-making. Both novices and seasoned pros can improve their data analysis abilities by adhering to the three suggestions provided in this article: setting clear objectives, choosing relevant tools, and streamlining procedures. These pointers enhance the precision and dependability of outcomes while streamlining the analytical procedure.
Using these techniques in your upcoming analytical project can help you understand data more effectively and solve problems more quickly. The quality and efficiency of your analyses can be greatly improved by implementing these suggestions into your workflow, regardless of how much expertise you have with big data analysis. Keep in mind that with continued use and improvement of these methods, your analytical skills will get even sharper over time. Therefore, don't be afraid to use these pointers in your next projects to maximize the benefits of your data analysis work.