1. Introduction
Delivering outstanding customer service is essential in today's cutthroat business environment to keep clients and guarantee long-term success. In order to develop long-term relationships with customers, increase brand loyalty, and propel corporate expansion, customer service is essential. Data science has become a potent instrument for improving the entire customer service experience as businesses work to meet and beyond customer expectations.
Through the utilization of data science methodologies and tools, enterprises may extract significant knowledge from enormous volumes of customer information. Businesses can better understand client preferences, behaviors, and problem spots with the use of these insights. By using this information, businesses can improve customer happiness by tailoring interactions, anticipating requirements, and offering proactive help.
Next time we will discuss how predictive analytics can optimize response times...
2. Understanding Customer Needs
Providing outstanding customer service requires a thorough understanding of the demands of the client. Through the use of data analytics, data science can be crucial in this regard by providing important insights into the preferences and behavior of customers. Businesses can gain a better understanding of what their consumers want, when they want it, and how they prefer to interact with the company by examining trends, patterns, and past data.
Using data analysis to personalize client interactions is one of the most effective ways to improve customer service. Effective use of customer data allows companies to customize offerings, communications, and support services to each customer's preferences and requirements. Customers get a more interesting and fulfilling experience as a result of this customisation, which eventually boosts customer happiness and loyalty.
Businesses are able to make more educated decisions and provide more individualized service to their clients by utilizing data science to better understand customer needs. This strengthens the bonds between companies and their customers while also enhancing the general customer experience.🖍
3. Predictive Analytics for Anticipating Customer Needs
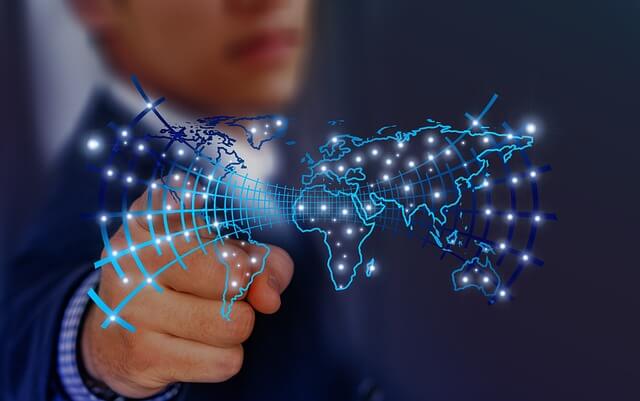
By using previous data analysis to forecast future patterns, predictive analytics is a potent tool for predicting client needs. Businesses can forecast client behavior and preferences by utilizing sophisticated algorithms, which allows them to customize their offerings. Predictive analytics, for example, can spot trends in consumer behavior when it comes to purchases, which enables businesses to foresee when a customer might require a specific good or service before the customer even knows it.
Predictive analytics has been successfully applied to proactive customer service in e-commerce platforms, where it has been used to provide individualized recommendations based on browsing and purchase history. By making recommendations for products based on user preferences, Amazon's recommendation engine serves as a shining example of how predictive analytics may improve the customer experience. Businesses may greatly increase customer happiness and boost revenue by correctly predicting what potential customers would be interested in.
Predictive analytics, based on network analysis and user activity patterns, is used by telecom providers to anticipate possible service interruptions. These businesses may guarantee continuous service for their clients by proactively resolving such problems before they arise, which raises client happiness and loyalty. Predictive analytics, in its whole, enables companies to anticipate and surpass customer expectations while staying ahead of customer needs.
4. Improving Response Times with Data Science
Using data science to speed up reaction times can revolutionize customer service. Businesses can expedite and improve the speed at which they respond to consumer requests by utilizing data algorithms. This makes it possible to handle client issues more effectively, which raises customer happiness and loyalty.
Data-driven techniques have significantly shortened response times, as demonstrated by case studies. For example, companies can forecast demand peaks and adjust resource allocation to reduce wait times by examining historical data on customer interactions. Proactive actions like these improve the consumer experience in general and help to create a favorable brand perception.📅
Through the utilization of data science methodologies like machine learning and predictive analytics, organizations can acquire significant understanding of consumer behavior trends. They can respond to inquiries and complaints more quickly because they can anticipate client needs and adjust their responses accordingly, thanks to this knowledge. Data science may improve response times, which benefits customers and increases operational efficiency and profitability.
5. Enhancing Customer Experience Through Sentiment Analysis
Sentiment analysis is a potent tool for improving the client experience. Businesses may now measure customer satisfaction and feedback more precisely than ever before by utilizing this data science technique. Sentiment analysis is the technique of identifying the feelings, viewpoints, and attitudes included in text data, such as customer reviews, social media posts, or polls, by utilizing machine learning and natural language processing algorithms.
Businesses may keep a close eye on their consumers' feelings in real time by putting sentiment analysis technologies into practice. This makes it possible for them to quickly spot patterns, trends, and possible problems, allowing for proactive reactions to both favorable and unfavorable comments. Businesses can improve the entire customer experience by customizing their products and services to better suit the needs and preferences of their customers by understanding their sentiments.
Sentiment research examines how consumers react to promotional messaging and new product introductions, which helps determine how effective marketing campaigns and customer service initiatives are. This priceless information aids companies in making data-driven decisions that boost client happiness and loyalty. By using sentiment research, businesses may develop more individualized client interactions and establish enduring bonds based on mutual respect and understanding.
6. Data-Driven Decision Making for Service Improvements
Making decisions based on data is an effective way to improve customer service. Businesses can improve their products or services by gaining important insights by utilizing data science approaches. By examining consumer preferences, feedback, and behavior, businesses may make well-informed decisions that have a direct effect on customer satisfaction.
Putting data-driven initiatives into practice affects consumer happiness over the long run in addition to solving immediate issues. Through ongoing analysis and responsive action, companies can identify trends, anticipate patterns, and proactively improve their services to address changing client demands. This proactive strategy shows a dedication to comprehending and satisfying client expectations throughout time.🖋
By seeing opportunities and weaknesses in service delivery that could otherwise go overlooked, data-driven decision making promotes continual improvement. Businesses may continuously provide improved client experiences by optimizing their processes and services and keeping an eye on key performance indicators and metrics obtained from data analysis.
To put it simply, firms are enabled to maximize their services by incorporating data science into decision-making processes and using real-time insights and trends to guide their decisions. Long-term improvements that lead to increased consumer happiness and loyalty are the outcome of this, not just quick solutions.
7. Leveraging Machine Learning for Customer Support Automation
The way organizations engage with clients is revolutionized when they use machine learning to automate customer service. Routine customer support jobs can be automated to save time and resources by using advanced algorithms. Chatbots and virtual assistants driven by AI are leading this change, providing round-the-clock assistance and prompt answers to client questions. By offering prompt, individualized help, these intelligent technologies not only increase overall satisfaction but also improve service efficiency.
Chatbots can learn from client interactions thanks to machine learning algorithms, which gradually improve their accuracy and replies. Customers are guaranteed to receive timely and relevant assistance because to this adaptive learning process, which also increases customer loyalty and speeds up issue resolution. Businesses may manage more questions at once with AI-driven automation, cutting down on wait times and improving the clientele's experience.
Beyond only increasing productivity, machine learning has many advantages in customer service. AI-powered chatbots can proactively handle typical issues before they arise by analyzing massive quantities of data to find trends and patterns in client requests. In addition to streamlining processes, this predictive power helps companies better anticipate the demands of their clients, building stronger bonds and enduring loyalty. Businesses can achieve sustainable growth by streamlining resources and improving customer service to new heights by leveraging machine learning. 😺
8. Case Studies: Real-world Applications of Data Science in Customer Service
Case studies are an effective way to demonstrate the advantages that data science can offer customer service. One prominent example is Amazon, which pioneered the use of data analytics to customize customer experiences. By using advanced algorithms to examine consumer behavior and interests, Amazon is able to deliver personalized product recommendations that increase customer satisfaction and increase sales.
Netflix, a business well-known for its data-driven approach to content suggestion, is another fascinating case study. Netflix enhances audience engagement and retention by optimizing its platform through the use of machine learning algorithms that anticipate viewer preferences through previous interactions.
Data science was used by telecom behemoth T-Mobile to transform its customer support processes. T-Mobile reduced customer complaints and enhanced service quality by using predictive analytics to foresee possible network problems and take proactive measures to resolve them. This led to higher customer satisfaction levels.
The aforementioned case studies showcase how forward-thinking businesses utilize data science tools and methodologies to revolutionize their customer service approaches, resulting in improved client connections and increased corporate expansion.
9. Challenges and Ethical Considerations in Data Science for Customer Service
Organizations must overcome a number of obstacles and ethical dilemmas when applying data science to customer service. Data privacy problems represent a significant barrier. Ensuring the protection of sensitive information becomes increasingly important as firms gather and examine massive volumes of client data. Retaining client trust requires transparent data governance procedures and adherence to laws such as GDPR.
The possible bias in the algorithms utilized in customer support procedures is another important problem. Biases within these algorithms can lead to unfair treatment of specific client groups or erroneous insights obtained from the data. It is imperative for businesses to adopt strategies like algorithmic audits and diversified data sets in order to address bias and guarantee fair outcomes for all clients.
There are moral conundrums with transparency and consent when using data science in customer service. It is important to inform customers about the ways in which their data is gathered, utilized, and shared. Giving consumers control over their data and unambiguous opt-in alternatives strengthens their bond with the company. In addition to adhering to moral principles, this openness fosters enduring consumer loyalty and trust.
10. Tips for Implementing Data Science Strategies in Customer Service
Your company's customer service strategy can be completely changed by incorporating data science techniques. The following advice can assist you in effectively incorporating data science to improve customer service:
1. **Establish Specific Goals**: Clearly state your goals for utilizing data science in customer service. Having clear goals can help you focus your efforts whether they be on anticipating client needs, personalizing encounters, or speeding up response times.
2. **Collect Relevant Data**: Make sure you compile high-quality, pertinent data from a variety of sources, including social media, website analytics, feedback forms, and consumer contacts. The caliber of the data you gather has a major impact on how accurate your analysis is.
3. **Use Advanced Analytics techniques**: To extract insightful information from sizable datasets, make use of advanced analytics techniques such as predictive modeling and machine learning algorithms. You may improve consumer experiences by using these technologies to anticipate trends, identify patterns, and make data-driven decisions.
4. **Empower Your Team**: Provide training to your customer care representatives on how to properly analyze and apply data. Urge them to use the insights gleaned from data analysis to tailor communications, foresee requirements, and offer prompt solutions.
5. **Monitor Performance Regularly**: Keep an eye on how well your data science customer service tactics are working. Examine important data like response times, customer satisfaction ratings, and resolution rates to gauge the success of your efforts and make the required improvements.
Through adherence to these guidelines and optimal methodologies, enterprises can leverage the potential of data science to enhance their customer service benchmarks and foster enduring expansion in the current competitive market environment.
11. Future Trends: The Evolution of Data Science in Customer Service
Data science is still essential to improving client experiences in the dynamic field of customer service. Prospective trends for the future appear poised to completely transform the way companies engage with their clientele. The incorporation of AI-powered customisation is one such trend. Businesses can provide customized experiences for every consumer by utilizing artificial intelligence algorithms, which are based on their individual interests and habits. Higher levels of client loyalty and relationships are fostered by this level of individualized service.
Augmented reality support for customer service is a new and intriguing concept. Imagine being able to use augmented reality (AR) technology to give customers real-time visual assistance so they can solve problems or have an immersive experience with items. By giving customers self-service skills, this not only improves their experience but also lessens the load on conventional support channels.
These new developments in data science are only the tip of the iceberg in terms of customer service's future. Businesses can stay ahead of the curve and provide remarkable customer experiences that differentiate them in a crowded market by embracing technology like AI-powered personalization and augmented reality support.
12. Conclusion: Summarizing the key takeaways on how data science can revolutionize the way businesses deliver exceptional customer service experiences.
Taking into account everything mentioned above, we can say that data science has changed the game when it comes to improving customer service for companies. Businesses may use data analytics to automate tasks efficiently, predict trends with accuracy, improve operational efficiencies, personalize customer experiences, and eventually increase customer happiness and loyalty.
The most important lesson is that companies may now better understand their customers thanks to data science. Businesses may anticipate client demands, customize services to individual tastes, and proactively handle concerns by analyzing large amounts of data. This customized strategy builds lasting relationships with clients in addition to improving the overall customer experience.
By finding inefficiencies and streamlining procedures, data science helps firms run more efficiently. Increased productivity, cost savings, and quicker customer response times are the results of this. Organizations may make data-driven decisions that promote growth and competitiveness in today's changing market environment by utilizing predictive analytics.
Accurately predicting trends through data analysis helps organizations remain ahead of the curve and respond proactively to shifting consumer wants or market conditions. Businesses now have the advantage they need to consistently innovate and provide solutions that are pertinent to and appealing to their target market.
Data science-powered automation can greatly increase handling mundane activities and client interactions' efficiency. Businesses can reallocate resources to more vital areas that need human intervention by automating procedures like query management, personalized suggestions, or feedback collecting.
The way organizations engage with their consumers is essentially revolutionized by the use of data science into customer service operations. It offers insightful information that promotes wise decision-making, makes individualized interactions possible at scale, maximizes operational workflow efficiency, predicts future trends for proactive planning, and automates operations to increase responsiveness. Adopting data science is not merely a fad; it is a must for any company hoping to prosper in the fiercely competitive market of today by continuously providing great customer experiences.