1. Introduction
The open-source community is growing quickly in the field of big data analytics. In the big data space, open-source solutions are essential for driving innovation and teamwork. Organizations are using these tools more frequently to get insights from massive amounts of data that help them make better decisions and improve their business plans. Because of the open-source ecosystem's dynamic character, innovative solutions are continuously being developed, stretching the bounds of what is conceivable when it comes to managing and analyzing enormous datasets. This blog article explores the importance of open source within the framework of big data analytics and highlights the multitude of choices that data professionals have in this dynamic environment.
2. Evolution of Big Data Open Source Tools
The way businesses handle and analyze data has changed dramatically as a result of the amazing evolution of big data open source tools. Important resources like Hadoop, Apache Spark, and Apache Kafka have been indispensable to this process. With its distributed file system and MapReduce programming methodology, Hadoop first revolutionized big data processing by enabling the parallel processing of massive datasets across computer clusters.
The real-time analytics and quicker data processing demands of the business led to the emergence of Apache Spark as a more effective Hadoop substitute. Its in-memory computing powers significantly increased interactive queries' and iterative algorithms' processing times. Apache Kafka is a distributed messaging system that facilitates fault-tolerant, high-throughput communication, thereby mitigating the difficulties associated with managing real-time data streams.
In response to shifting industrial demands, these technologies have undergone constant evolution. For example, Hadoop has improved its usability for a wider range of use cases by expanding its ecosystem with projects like Apache Pig for data flow scripting and Apache Hive for SQL querying. To meet a variety of analytical needs, Spark has also introduced libraries such as GraphX for graph processing and MLlib for machine learning. With the growing adoption of cloud technologies and containers by enterprises, these tools have also evolved to function smoothly with contemporary infrastructure configurations.👍
In summary, the development of big data open source technologies is reflective of the changing needs of the industry. These tools have evolved from batch processing to real-time analytics to efficiently handle a wide range of use cases. We may anticipate more big data technologies that will drastically alter data management and analysis in the future as long as technology keeps advancing at a fast rate.
3. Popular Open Source Platforms in Big Data
A number of open-source systems have become prominent participants in the rapidly developing field of big data processing, transforming the way data is handled and examined. In this scenario, Apache Spark, Apache Hadoop, and Apache Kafka are three of the leading players.
The distributed file system of Apache Hadoop is well known for allowing large volumes of data to be processed across clusters of commodity hardware. It is perfect for situations where bulk data processing is required since it performs exceptionally well in batch processing and large-scale data storage. Parallel computing made possible by Hadoop's MapReduce framework makes it possible to analyze structured and unstructured datasets quickly.
Conversely, Apache Spark's quick in-memory data processing has made it more well-known. Compared to conventional disk-based systems like Hadoop, Spark dramatically increases processing speed by utilizing resilient distributed datasets (RDDs). It is a preferred option for real-time analytics and iterative algorithms due to its adaptability, which also extends to machine learning and graph processing workloads.
A high-throughput distributed messaging system for real-time data streaming applications is Apache Kafka. Serving as a barrier between producers and consumers, Kafka ensures fault tolerance and scalability while handling continuous streams of data effectively. Because of its resilience and low latency, it is a good fit for applications like event-driven systems, stream processing pipelines, and log aggregation.
Essentially, these three platforms are essential in forming the big data environment because they provide scalable solutions specific to various parts of data processing, such as real-time streaming and interactive queries with Spark and Kafka, and storage and batch analysis with Hadoop. Their ongoing development is a reflection of the rising need for effective solutions that can leverage big data to spur innovation and new insights across sectors.
4. Emerging Trends in Big Data Open Source Ecosystem
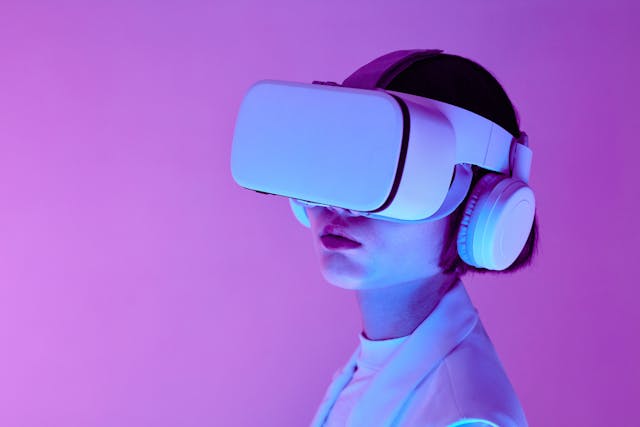
The big data open source ecosystem has expanded significantly in recent years, and new ideas and fashions are appearing quickly. A notable development in today's fast-paced digital environment is the increased emphasis on real-time data processing and analytics, which is motivated by the need for immediate insights. Due to their effectiveness in managing streaming data, technologies like Spark Streaming, Apache Kafka, and Apache Flink are becoming more and more well-known.
The use of AI and machine learning in big data analytics is another noteworthy trend. Large dataset processing and value extraction within enterprises are being revolutionized by open source tools like PyTorch, TensorFlow, and Apache MXNet. Businesses may create complex models for applications like image recognition, natural language processing, and predictive analytics with the help of these tools.
In terms of big data, open source solutions appear to have a bright future. More developments in fields like security, privacy, and data governance should allay growing worries about data protection. The demand for more resilient solutions that can manage the enormous volumes of data created at the edge will probably increase as edge computing and IoT technologies advance.
Taking into account everything mentioned above, we can say that innovation and technological developments are driving a swift evolution of the big data open source scene. Through keeping up with new developments and forecasting trends, companies can leverage open source technology to get valuable insights from their data assets. It is evident that as we progress toward a more connected and data-driven future, there are a plethora of intriguing opportunities for utilizing big data with open source tools.
5. Community Collaboration and Contributions
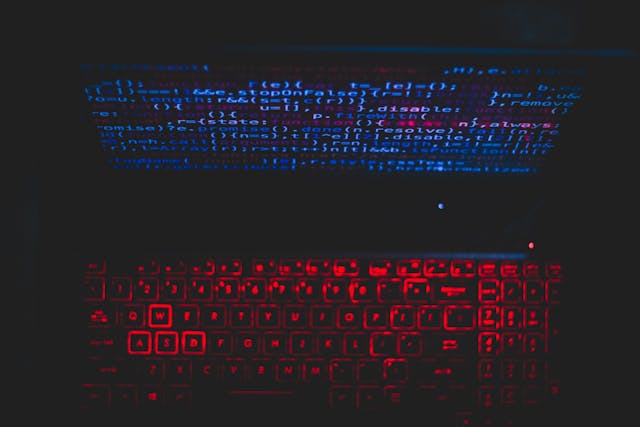
In the big data landscape, community collaboration is essential to the progress of open source initiatives. The combined efforts of developers all across the world who provide their knowledge, time, and resources enable these projects to flourish. People from different backgrounds join together through community collaboration to exchange ideas, work on solutions, and invent as a group. Rapid advancement and ongoing improvement of open source technology are made possible by the strength of many people working toward a common objective.
Across the globe, developers have made countless successful contributions that are prime instances of how community collaboration can spur innovation in the big data open source ecosystem. These contributions, which range from feature additions and bug fixes to completely new project initiatives, demonstrate the commitment and expertise of developers everywhere. These kinds of partnerships not only improve on ongoing projects but also provide doors for new ones that tackle new problems in big data processing, analytics, and storage.
Open source is an inclusive and transparent community that invites developers to engage and contribute regardless of their location or affiliation with an enterprise. This community-driven strategy advances the collective capacities of open source solutions in managing large volumes of data efficiently while encouraging creativity, knowledge exchange, and skill development among members. In order to address the ever-changing demands of today's data-driven world, the big data open source environment is growing and evolving as a result of this cooperative effort.
6. Challenges and Opportunities Ahead
The big data open source community is facing a number of difficulties as it grows quickly. One key challenge is the necessity for constant maintenance and support for various open source projects. It gets harder to maintain compatibility, bug fixes, and regular updates with so many platforms and tools accessible.
One of the biggest challenges that still exists with open source solutions is interoperability. It can be intimidating to construct extensive data pipelines by seamlessly integrating several technologies. The absence of uniformity among various instruments frequently results in problems with data coherence, format conversions, and system coherence in general.
Conversely, this expansion offers a wealth of chances to improve big data technologies. Better cooperation across various open source communities can result in creative fixes for the present issues with analytics, storage, and data processing. Through collaborations and knowledge-sharing programs, the ecosystem can benefit from cumulative experience.
Opportunities for growth are presented by developments in areas like cloud scalability, real-time analytics capabilities, and machine learning integration. Acknowledging new developments like AI-powered automation and decentralized processing architectures might help big data technologies advance in terms of performance and efficiency.
7. Case Studies: Real-world Applications
Case studies that demonstrate how open source tools have been successfully used in various industries provide compelling illustrations of the expanding impact of big data technologies. Companies in a variety of industries are using these tools to improve decision-making, spur innovation, and obtain insightful business information. For example, a retail business may use open-source analytics tools to examine consumer buying trends and refine inventory control plans using forecast information.
Big data technology have the potential to completely transform the healthcare sector by providing predictive analytics that can tailor treatment plans or predict disease outbreaks. Hospitals can use data-driven decision-making to optimize operations, cut expenses, and improve patient outcomes by utilizing open-source technologies. Similar to this, financial institutions can use big data tools to identify fraudulent activity, better manage risks, and precisely customize financial services to match the needs of their clients.
By enabling customized learning experiences catered to the requirements of each individual student, the use of open source software in the educational sector has the potential to revolutionize traditional teaching approaches. Teachers can spot patterns in big datasets produced by online learning platforms, modify their curricula accordingly, and increase student engagement and success rates. Incorporating big data technologies into education not only enhances student performance but also assists organizations in making strategic choices that will support their long-term expansion and advancement.
These case studies highlight how open source tools may revolutionize a variety of sectors. Organizations may achieve unprecedented levels of innovation, efficiency, and competitiveness in today's dynamic business market by leveraging the potential of big data technologies.😀
8. Comparison between Commercial vs Open Source Solutions
It's critical to evaluate the advantages and disadvantages of both commercial and open-source solutions when deciding which to utilize for big data requirements. Better security features, specialized support, and cutting-edge functionality are frequently included with commercial software. It may not provide the same degree of customisation as open-source alternatives, though, and it can be expensive. Conversely, open-source software usually comes with a huge developer community, is readily adaptable, and is free to use. Yet, in comparison to commercial products, it could be lacking in certain sophisticated features and thorough support.
Consider aspects like cost, necessary features, scalability, security requirements, and maintenance resource availability when choosing the best solution for your needs. A commercial solution can be appropriate if you value first-rate assistance above all else and are prepared to shell out money for sophisticated functionality. On the other hand, an open-source solution can be more appropriate if customisation is essential and you have internal experience to run the system efficiently. Making an educated choice that best meets your big data goals will come from knowing your unique needs and assessing how well each type of software fits them.
9. Security and Privacy Considerations
As the open source big data landscape is growing quickly, security and privacy concerns become more and more important. Potential data susceptibility as it flows through several systems and networks is one of the primary issues with big data processing using open source solutions. Cyberattacks, illegal access, and data breaches are all more likely when massive amounts of data are processed and stored.
Strong security measures must be put in place in a big data environment to safeguard sensitive data. Protecting data from unwanted access can be aided by encrypting it while it is in transit and at rest. It is important to implement access control measures to guarantee that only individuals with the proper authorization can access or modify sensitive data. Frequent security audits and monitoring can assist in quickly identifying any irregularities or possible breaches so that appropriate action can be taken.
Before keeping or processing personally identifiable information, it might be anonymized or pseudonymized to provide an additional degree of security. To quickly fix any vulnerabilities, it's critical to keep up with the most recent security risks and upgrades in the big data open source community. Organizations can reduce the risks involved in handling sensitive data processing and improve the security of their big data environments by adhering to these best practices and exercising caution.
10. The Role of Artificial Intelligence and Machine Learning
Analytics have been completely transformed by the integration of big data with artificial intelligence (AI) and machine learning (ML) using open-source platforms. Large-scale datasets may be seamlessly integrated with AI/ML algorithms thanks to open-source tools like TensorFlow and Apache Spark, which improve analytical insights. Businesses may now effectively extract significant patterns and trends from large, complex data sets by combining AI/ML with big data.
Big data analytics skills have been greatly enhanced by recent developments in AI algorithms. Large-scale dataset processing has become faster and more precise thanks to innovations like deep learning and neural networks. Reward systems like reinforcement learning are improving decision-making based on information from large-scale data analysis. These developments are accelerating innovation and creating competitive advantage across a range of industries by stretching the bounds of what businesses can accomplish with their data.
11. Future Prospects: Innovations on the Horizon
Open-source big data tools have a bright future ahead of them, full of continuing progress and maybe game-changing discoveries. Upcoming developments in technology may include better machine learning algorithms that can handle large volumes of data more quickly, improving accuracy and providing organizations with insights more quickly. Advances in natural language processing (NLP) could provide more opportunities for the extraction of useful information from a variety of data types by improving the analysis of unstructured data sources like text, audio, and images.
The integration of big data analytics with cutting-edge technologies like artificial intelligence (AI) and the Internet of Things (IoT) is another field that is expected to see significant growth. This convergence may lead to increasingly linked and intelligent systems that may produce real-time insights from several sources. It is anticipated that the deployment of serverless computing models and cloud-native architectures will simplify data processing operations, enabling enterprises to expand their analytics capabilities without sacrificing flexibility or performance.
Looking ahead, big data analytics is probably going to be greatly influenced by developments in edge computing and decentralized data processing. Edge computing systems can handle large datasets more efficiently and with less delay by moving computation closer to the data source. Blockchain technology advancements could bring about new approaches to safely exchange and store data while preserving openness and privacy, which is important in an era of growing cybersecurity risks and data restrictions.
From everything mentioned above, it is clear that the worldwide IT community is always innovating and working together to shape the rapidly changing environment of open-source big data technologies. Through predicting future developments and investigating possible innovations in big data analytics, we can get ready for a time when data-driven insights will be easier to obtain, more potent, and more revolutionary than before. It will be crucial for businesses to adopt these changes if they want to remain competitive in a world where data is becoming more and more important.
12. Conclusion
To sum up everything I mentioned above, an ever-expanding community of developers and organizations is driving the rapid expansion of the big data open-source ecosystem. The emergence of open-source technologies like Spark, Kafka, and Apache Hadoop has drastically changed how businesses handle and examine data. In addition to offering affordable solutions, this expansion encourages industry players to collaborate and innovate.
It is impossible to exaggerate the importance of ongoing innovation and cooperation within this group. Adopting open-source tools facilitates greater flexibility, quicker development cycles, and easy access to a wealth of resources. The big data community makes sure that companies can keep up with the rate of technological development and remain competitive in today's data-driven world by cooperating to improve current technologies and develop new ones.
It is obvious that the big data open-source landscape will keep changing quickly as we move toward the future. Organizations may set themselves up for success in an increasingly data-centric environment by participating in open-source initiatives, adopting new technologies, and remaining engaged in the community.