1. Introduction
Introduction: In today's data-driven world, the quality of data plays a pivotal role in the success of businesses. Data is no longer just an asset but the lifeblood that fuels decision-making processes across organizations. Poor data quality can lead to inaccurate insights, flawed strategies, and ultimately hinder competitiveness in the market. To ensure that data-driven decisions are reliable and effective, measuring return on investment (ROI) on data quality becomes crucial in assessing the value derived from efforts to maintain and improve data accuracy, completeness, consistency, and reliability.
Assessing the return on investment (ROI) of improving data quality means figuring out how those investments affect the organization's observable gains. It entails examining the ways in which enhanced data quality benefits areas such as overall business performance, cost reduction, revenue generation, customer happiness, operational efficiency, and risk mitigation. By recognizing the return on investment (ROI) associated with data quality, organizations can effectively allocate resources towards efforts that yield notable enhancements in data reliability and utility. Organizations can justify investments in procedures, tools, and resources devoted to upholding high-quality data standards by calculating the benefits of strong data quality practices.
Stay tuned for the upcoming sections, where we will go deeper into best practices and various approaches for calculating ROI on data quality. These sections will equip your organization with insights that can be put to use right away, helping to drive business success through improved data management practices.
2. Understanding Data Quality
Understanding Data Quality
The measurement of data accuracy, consistency, and dependability is known as data quality. It is essential to corporate operations and decision-making processes because better results and more dependable insights come from high-quality data. In essence, data quality makes sure that the information being used is reliable and suitable for a range of studies and strategic projects.
Data quality can be impacted by various variables. These can include inconsistent data across databases or systems, outdated or missing information, problems with data processing methods, a lack of standard formats for data storage, and human error during data entering. Lower data quality levels can be caused by a variety of factors, including duplicate data entry, inadequate data governance procedures, and poor system integration. Gaining an understanding of these common variables is essential to enhancing the overall quality of data in a system or organization.đ
3. Key Metrics for Measuring Data Quality ROI
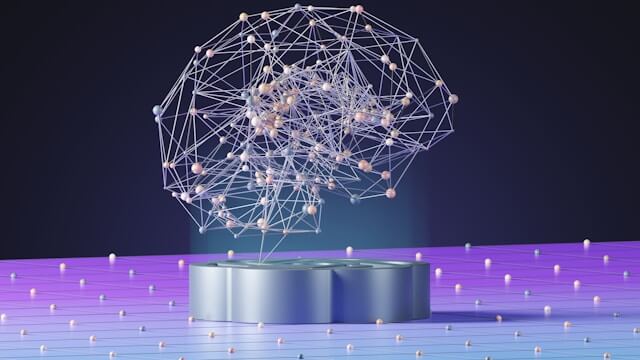
Key Metrics for Measuring Data Quality ROI
When assessing the ROI of data quality programs, it's critical to concentrate on important indicators that have a direct bearing on the total value derived from data. Among the most important metrics to monitor is accuracy, which guarantees the dependability and credibility of judgments based on data. Inaccurate information can result in expensive mistakes and misplaced tactics, which eventually hurt the bottom line.
A crucial indicator for assessing the return on investment in data quality is completeness. A large dataset guarantees that the conclusions extracted from it are more thorough and true to the world they are trying to depict. Inadequate data can lead to erroneous conclusions or overlooked opportunities, which prevents businesses from optimizing their operational effectiveness or seeing possible expansion chances.
Maintaining data uniformity across various systems and sources within an organization is mostly dependent on consistency. Redundancies, ambiguities, or contradicting information can result from inconsistencies, which can impede decision-making and undermine corporate objectives. Businesses may increase the efficiency of their operations and the efficacy of their plans by upholding consistency in the quality of their data.
A crucial factor in determining the return on investment of data quality initiatives is timeliness. The usefulness and efficiency of data in facilitating prompt decision-making are directly impacted by its relevance and currency. Information that is out of date or delayed might lead to lost opportunities or reactive rather than proactive reactions to consumer demands or industry developments. In today's fast-paced business climate, firms may maintain agility and responsiveness by ensuring that data is current and easily accessible.
As I mentioned earlier, companies may more accurately assess the return on their expenditures in improving data quality by concentrating on measures like correctness, completeness, consistency, and timeliness. For organizations willing to prioritize data quality as a strategic priority, these core indicators not only increase data dependability and usability but also help to improve decision-making processes and eventually drive greater return on investment.
4. Methods for Calculating ROI on Data Quality
There are several ways to assess the return on investment (ROI) associated with enhancing data integrity. One strategy is to take into account cost reductions that come from fewer mistakes and more operational effectiveness. When a business invests in cleaning up its client database, for example, precise addresses might result in lower shipping expenses, which can save a lot of money over time.
Evaluating the revenue gain attributed to improved data quality is an additional approach. Businesses can increase conversion rates and customer retention by utilizing precise and current data for focused marketing initiatives. For instance, a store may find previously overlooked trends that influence better product offerings and price strategies, ultimately increasing revenue, by more accurately evaluating sales data.
The computation of ROI on data quality also heavily weighs risk mitigation. Enhanced data quality can assist companies in spotting possible errors, fraud, and compliance concerns before they become expensive ones. By eliminating the possibility of insuring high-risk clients, an insurance company can minimize its exposure to claims and financial losses by employing high-quality data for underwriting choices.
Through the comparison of these several strategiesâcost reduction, revenue growth, and risk reductionâbusinesses may acquire a thorough grasp of the financial implications of investing in data quality. Every approach offers a different perspective on the concrete advantages that result from maintaining correct, trustworthy data throughout all processes.
5. Best Practices for Improving Data Quality
Improving data quality is crucial for making informed business decisions and achieving accurate ROI calculations. Here are some actionable steps to enhance data quality effectively:
1. Identify Data Quality Standards: Clearly define what, inside your company, qualifies as high-quality data. This will function as a benchmark for evaluating and enhancing the quality of data.
2. Implement Data Quality Tools: Invest in tools like data profiling, cleansing, and monitoring software to identify errors, inconsistencies, and duplications in your datasets.
3. Conduct Regular Data Audits: Schedule routine audits to assess the current state of your data quality. Identify areas for improvement and track progress over time.
4. Educate and Train Staff: Give instruction on the best ways to enter data and the significance of keeping high-quality data. Make sure workers are aware of their responsibilities for maintaining data quality standards.
5. Enforce Data Governance Policies: Establish clear data governance policies that outline roles, responsibilities, and procedures for managing data quality throughout its lifecycle.
6. Foster Stakeholder Collaboration: Involve stakeholders from different departments in data quality initiatives to gain diverse perspectives and ensure alignment with business objectives.
7. Monitor Data Quality Metrics: Define key performance indicators (KPIs) related to data quality and regularly monitor them to track improvements and demonstrate ROI on data quality efforts.
By adhering to these best practices and stressing the value of stakeholder collaboration and data governance, companies may improve their data quality management procedures dramatically and make more informed decisions based on dependable data sources.
6. Tools and Technologies for Enhancing Data Quality
Using a variety of tools and technologies is essential when it comes to improving the quality of your data because it guarantees accuracy and dependability. With the use of data profiling tools, you may learn more about the composition and caliber of your data, better comprehending its features and spotting possible problems. Cleaning tools assist in identifying and fixing erroneous or corrupted records, guaranteeing that your data is error-free and consistent. Monitoring tools are essential for tracking how your data evolves over time and helping you to consistently uphold high standards.
Because technology increases speed, decreases human error, and automates manual operations, it plays a major role in simplifying data quality processes. Through the utilization of cutting-edge technology like artificial intelligence and machine learning algorithms, entities can further improve their data quality procedures. Large datasets contain patterns, anomalies, and trends that could be missed by manual review. These tools can find them. This increases the overall efficacy and accuracy of data quality metrics while also saving time.
Additionally, as I mentioned earlier, improving data quality successfully requires the correct combination of tools and technology. Through the integration of data profiling, cleansing, and monitoring tools with advanced technologies such as artificial intelligence and machine learning, organizations may optimize their workflows while upholding rigorous standards of precision and dependability in their data. Adopting these tools and technology guarantees that decisions are founded on reliable and accurate information in addition to increasing operational efficiency.
7. Case Studies on Successful Data Quality Initiatives
When it comes to data quality programs, real-world success stories are a great way for firms to improve their return on investment. One such instance is a global retailer that, following the simplification of its product data quality procedures, witnessed a notable rise in customer satisfaction and retention rates. The organization decreased errors in its product catalog, which resulted in fewer returns and increased customer trust. This was accomplished by putting in place rigorous data governance procedures and automated data validation technologies.
In a similar vein, a financial services company that invested in improving data quality saw significant returns on its investment. The organization greatly increased the accuracy of its compliance filings and financial reports by integrating data profiling tools and putting in place a thorough data cleansing program. As a result, operational expenses for correcting errors by hand were decreased, and regulatory confidence was raised.
An additional noteworthy case study centers on a healthcare provider that experienced significant advantages by giving priority to data quality activities. The company implemented a master data management system and enforced uniform data input procedures throughout departments, resulting in notable enhancements to patient care coordination and billing accuracy. This improved overall patient outcomes in addition to reducing billing discrepancies and saving money.
These effective implementations highlight how important it is to have a solid execution strategy and strategic planning in order to get significant return on investment from data quality projects. Businesses that place a high priority on upholding data integrity requirements can benefit from increased customer satisfaction, operational efficiencies, and competitive advantages. Businesses can gain important insights on best practices for maximizing the impact of their own data quality strategy by carefully examining these case studies.
8. Challenges in Measuring Data Quality ROI
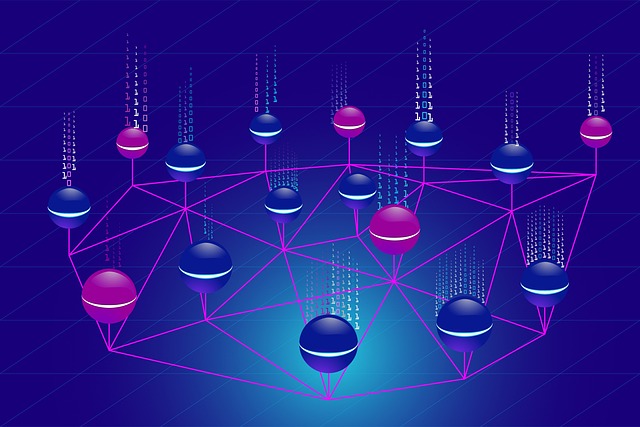
For organizations hoping to make well-informed business decisions, calculating the Return on Investment (ROI) of data quality projects is essential. Nevertheless, a number of obstacles may prevent this procedure. A prevalent challenge is the absence of agreement on the metrics to be employed in assessing enhancements to data quality. If there isn't a consensus on what constitutes success, it becomes difficult to compare outcomes amongst teams or projects.
The inadequacy of resource allocation presents another difficulty in calculating ROI for data quality. Sufficient resourcesâtools, knowledge, and timeâare necessary to precisely monitor the results of data quality initiatives. Inadequate resources may result in assessments that are not completed or in delays obtaining the data required for analysis.
Organizations can begin addressing these issues head-on by bringing in important stakeholders early on to develop a common understanding of the measures that are most important for determining the return on investment (ROI) of data quality. This alignment eliminates uncertainty during the review process and guarantees that everyone is working toward the same objectives.
Investing in reliable technology and solutions for data quality can simplify the assessment process and offer more precise information about how well improvement projects are working. ROI calculations can be more reliable when automation is used to ensure consistent data gathering procedures and lower the risk of human mistake.
Lastly, companies should place a high priority on providing teams with the tools they need to measure and maximize the return on investment from data qualityâbe it budgetary support, specialized staff, or training initiatives. Businesses can overcome resource limitations and carry out more comprehensive evaluations of their data quality investments by providing staff with the appropriate tools and training.
9. Future Trends in Data Quality Management
As we look ahead to future trends in data quality management, two key innovations stand out: AI-driven data quality solutions and blockchain technology for maintaining data integrity.
Data quality is being revolutionized by AI, which provides automated procedures for mass data enrichment, validation, and cleansing. These artificial intelligence (AI)-powered solutions guarantee that businesses always have access to high-quality data by quickly identifying trends and abnormalities.
Data validation and archiving are made visible and safe via blockchain technology. Blockchain increases network trust in data consistency and accuracy by generating an unchangeable transaction ledger. There is a lot of promise in this technology to guarantee data integrity across different businesses.
With these new developments, calculating ROI on data quality will have important future ramifications. Workflows can be streamlined, errors can be decreased, and decision-making processes can be optimized using AI-driven solutions, producing more accurate insights and better business results. In the meantime, the tamper-proof characteristics of blockchain improve data security and reliability while lowering the expenses related to mistakes or fraudulent activity.
Businesses that use these cutting-edge technologies may expect improved customer experiences, increased efficiency, and improved risk managementâall of which result in a measurable return on investment for their investments in preserving high-quality data assets.
10. Integrating Data Quality into Business Strategy
Ensuring that data projects lead to the achievement of overall company objectives requires integrating data quality into business strategy. Businesses can increase operational efficiency, customer happiness, and decision-making processes by coordinating data quality initiatives with specific business goals. An company may guarantee that the information it generates and uses is accurate, dependable, and actionable by proactively integrating data quality.
Businesses should first clearly define their main business objectives and determine how data contributes to the achievement of these objectives in order to effectively include data quality considerations into their strategic planning processes. Involving important stakeholders from different departments is crucial to understanding their data needs and issues. It is essential to create a thorough data quality plan that details procedures for gathering, validating, storing, and using data. The data will stay in line with strategy goals if quality indicators are routinely monitored and assessed against predetermined benchmarks.
It is imperative to establish unambiguous accountability for data quality inside the company. It can be easier to assign duties for upholding and enhancing data quality standards by creating positions like Data Stewards or Data Quality Managers. Investing in training initiatives that increase departmental understanding of the value of high-quality data can also support the development of an organizational data governance culture. By incorporating dependable and superior data assets, strategic planning that takes data quality into account not only improves decision-making but also lays the groundwork for long-term corporate success.
11. Continuous Improvement Strategies for Sustaining Data Quality ROI
After installation, maintaining data quality ROI requires constant improvement. Companies can accomplish this by putting measures into place such as frequent data audits, setting up KPIs for data quality, holding training sessions, and making use of automated systems for monitoring. To make sure that efforts to improve data quality are successful and pertinent over time, it is critical to emphasize the need of establishing a continuous improvement culture inside the company. Accepting criticism, promoting departmental cooperation, and routinely reviewing and improving procedures are essential elements of this culture that can support continuous success in preserving high-quality data.
12. Conclusion
Businesses must measure the return on investment (ROI) on data quality to make sure they are basing their decisions on reliable information. We have covered the significance of data quality in promoting business success in this blog post, as well as how it affects a number of different areas, including decision-making, customer relations, and overall operational efficiency. We emphasized the importance of correctness, completeness, consistency, and relevancy as critical metrics for evaluating the quality of data. Through the methodical use of measures such as data profiling, cleansing, and governance, businesses can raise the quality of their data.
Businesses must make calculating the return on investment (ROI) from data quality a top priority as part of their overall business plan. Investing in data quality projects increases revenue growth through focused marketing campaigns and improved customer insights, in addition to improving operational efficiency. Organizations may present a strong argument for ongoing investment in upholding high-quality data standards by quantifying the impact of increased data quality on critical performance indicators including revenue creation, cost savings, and customer satisfaction.đ
As you proceed with your business plans, keep in mind that sound data serves as the cornerstone for wise decision-making. Adopt a mindset that prioritizes data integrity and makes constant investments to track and enhance the return on investment from high-quality data. By doing this, you'll set up your company for long-term success in the increasingly data-driven business environment of today.đ