1. Introduction to Data Maturity Mapping
Knowing where a company stands in terms of data maturity is essential when it comes to utilizing big data. As a compass, data maturity mapping helps businesses evaluate how well they can handle and use data to inform strategic decision-making. Businesses can identify the strengths, shortcomings, and areas for progress in their data operations by classifying several phases of data maturity.
Businesses may see where they are on the data maturity continuum, from simple data gathering to sophisticated predictive analytics and machine learning capabilities, by using data maturity mapping. This procedure entails assessing a number of factors, including organizational alignment with regard to data objectives, analytics tools, quality, and data governance. By using this evaluation, companies can identify weaknesses in their data architecture and procedures that might be preventing them from getting useful insights from their data assets.
Companies may map out a clear course for improving their data capabilities by determining where they are now on the Data Maturity Map. By using this strategic approach, organizations may efficiently allocate resources to move towards greater levels of data maturity and set realistic targets based on their existing degree of maturity. To fully realize the benefits of their data-driven efforts, organizations may make well-informed decisions about technology investments, personnel development, and process enhancements by using data maturity mapping.
2. Importance of Developing a Big Data Strategy
To properly harness the power of data, firms must develop a big data strategy. Businesses can leverage big data's potential to spur innovation, enhance decision-making, and achieve competitive advantages in their particular industries by using an organized strategy. Organizations can better target efforts and achieve quantifiable results by aligning their data projects with business goals through the establishment of explicit objectives.
A clearly defined big data strategy sets instructions for effectively gathering, storing, analyzing, and using data, which aids in the optimization of data management procedures. It helps businesses to improve data quality, expedite workflows, and guarantee compliance with laws protecting privacy and security of personal information. Businesses may maximize the value generated from data while controlling costs connected with data management when they have a strategic plan in place.
An organization's culture of data-driven decision-making is fostered by a strong big data strategy. It equips staff members with the knowledge and abilities necessary to make wise decisions by encouraging them to understand the value of data as a strategic asset. This move toward data-centricity encourages departmental cooperation and empowers groups to work together toward shared goals using solid evidence rather than gut feeling.
For businesses looking to stay relevant and competitive in today's increasingly digital market, where massive amounts of data are generated every second, having a well-crafted Big Data Strategy is a must, not an option. It offers companies a road map for successfully navigating the intricacies of machine learning, artificial intelligence, big data analytics, and other emerging technologies. In the big data era, organizations may position themselves for long-term success and sustainable growth by devoting time and money to creating a strong plan up front.
3. Understanding Data Maturity Levels
Any firm trying to create a successful big data strategy must have a solid understanding of data maturity levels. Through evaluating your company's position on the data maturity spectrum, you can ascertain the necessary measures to advance towards more advanced and valuable data exploitation.
Levels of data maturity often span from simple, ad hoc data utilization to sophisticated, strategic data as a key asset exploitation. At the lowest level, companies might not have a clear data strategy, have erratic data quality, and only occasionally generate insights from their datasets. Organizations start to set up more organized procedures for gathering, storing, and evaluating data as they advance up the maturity ladder.
Implementing tools and technology to automate procedures, enhance data quality, and facilitate improved decision-making is common at intermediate levels of data maturity. At this point, companies may begin to use business intelligence tools or rudimentary analytics to better effectively extract insights from their data.
A strategic strategy where data is viewed as a valuable business asset that influences decision-making at all organizational levels is required for advanced maturity in data usage. All departments have a culture of data-driven decision-making embedded in them, advanced analytics techniques are used for prescriptive and predictive analysis, and data governance frameworks are built.
Organizations can assess their current state of data asset utilization and plan for their future state by comprehending these various data maturity levels. This self-evaluation serves as the basis for creating a roadmap that will improve their capacity to handle big data efficiently and extract the most value possible from it. In the later sections of this series, we will go more deeply into the tactics needed at every step to advance an organization's data maturity.😥
4. Assessing Current Data Practices and Capabilities
It is important to do a thorough assessment of your present data practices and capabilities at this stage of developing your big data strategy. Knowing where you stand with data handling will help you identify areas that want improvement. Start by looking at the ways that data is gathered, kept, processed, and used in your company.
Analyze the technology and techniques you presently employ for data management. Are they effective? Do they satisfy your requirements? Examine your present infrastructure to see if there are any gaps that could prevent you from utilizing big data to its fullest. Your current data practices' strengths and shortcomings will be determined with the aid of this examination.
Examine the knowledge and abilities of the team members who handle data. Evaluate their level of expertise in using data analytics tools and deciphering big data findings. By identifying any skill shortages, you can make plans for training initiatives or recruitment campaigns to improve the competencies of your workforce.
Finally, assess how well your present data projects are performing in comparison to your predetermined goals and objectives. Assess the effectiveness of your current techniques and whether they are producing the expected results or whether they may be improved. By evaluating these facets of your present data practices and capabilities, you may provide a strong basis for subsequently creating a successful big data strategy.
5. Creating a Roadmap for Data Maturity Improvement
In order to fully utilize big data, enterprises must develop a data maturity improvement strategy. This entails determining the capabilities of the data currently available, establishing improvement targets, and detailing the precise actions needed to achieve these goals. First and foremost, it's critical to carry out a comprehensive evaluation of the organization's current data infrastructure, procedures, and personnel. This assessment will assist in determining the company's data maturity level and the areas that require improvement.
Establishing specific goals is the next stage in creating a strong roadmap once the present situation has been assessed. To guarantee that they can be properly monitored over time and are actionable, these objectives should be SMART (Specific, Measurable, Achievable, Relevant, Time-bound). Every objective, whether it's to raise the organization's overall data maturity level, boost analytics capabilities, or expedite data governance procedures, should work toward this end.👧
The roadmap should include a clear statement of objectives followed by a detailed list of initiatives and actions needed to reach these targets. These could be making investments in new technology, offering staff training, setting up strong data governance structures, or putting best practices for data management into effect. Prioritizing each project according to how it could enhance data maturity and fit into the larger business plan should be done.
Any effective path for improving data maturity must include regular monitoring and review. This enables companies to monitor their progress in relation to predetermined objectives, spot obstacles or bottlenecks early on, and make the required corrections to continue on course. Organizations should make sure they're using big data to promote innovation and competitive advantage by regularly evaluating and improving their approach to developing data maturity.
Developing a plan for improving data maturity entails evaluating current capabilities, establishing targeted efforts, SMART targets, and regularly tracking advancement. Organizations may increase their degree of data maturity and fully utilize big data analytics in all facets of their operations by putting in place a well-thought-out plan that is in line with business objectives and priorities.
6. Implementing Tools and Technologies for Enhanced Data Management
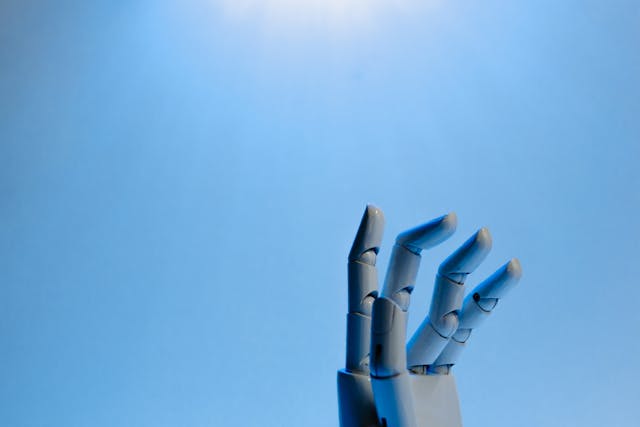
Enhancing data management as part of an organization's big data strategy requires the use of tools and technologies. Businesses need effective ways to store, process, analyze, and derive insights from massive amounts of data because of the exponential growth of data. Big data management can be made more efficient by putting in place tools like data lakes, warehouses, and ETL (Extract, Transform, Load) procedures and business intelligence software.
For the purpose of facilitating easy access to and analysis of structured and ordered data, data warehouses are essential. They offer an organization's central location for combining data from different sources. However, compared to conventional storage systems, data lakes are the best option for storing enormous volumes of unstructured and raw data at a reduced cost. ETL process implementation guarantees effective data cleansing, transformation, and loading into the right systems.
Businesses may effectively see and analyze their data with the use of business intelligence tools. These technologies provide customers with the ability to extract meaningful insights from their big data through dashboards, reports, and analytics features. Businesses may take advantage of actionable insights from their massive datasets to improve decision-making processes, increase operational efficiency, and find new growth prospects by strategically deploying these technologies.
7. Building a Data-Driven Culture within the Organization
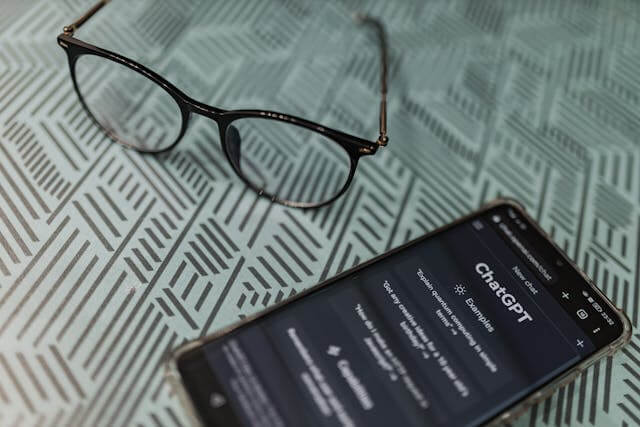
Implementing a big data strategy successfully requires a firm to develop a data-driven culture. Creating an atmosphere where data is respected, used, and trusted at all organizational levels is part of this cultural shift. Organizations should concentrate on crucial elements including staff training, leadership support, and creating effective channels for communicating the value of data in order to do this.
By establishing the tone at the top and exhibiting a dedication to using data to inform decisions, leadership is essential in forming a data-driven culture. Executives must explain the benefits of data to staff members at all levels and adopt data-driven processes themselves. Through setting an exemplary example and integrating data into strategic dialogues, leaders can motivate others to emulate their actions.
Another essential element in creating a culture that is data-driven is employee training. Businesses should fund training initiatives that give staff members the know-how to properly analyze and use data. Employees might feel empowered to use data in their daily operations if they have access to pertinent tools, resources, and training sessions catered to diverse roles within the firm.
Fostering a culture that is driven by data also requires clear communication about the value of data. Companies need to be clear about how data drives business outcomes and impacts decision-making procedures. Employees can gain a better understanding of the importance of integrating data into their work by hearing about success stories that showcase the effects of data-driven initiatives.
All stakeholders in a business must be dedicated to and put forth constant effort toward developing a data-driven culture. Organizations can foster an atmosphere where utilizing big data becomes engrained in the organizational DNA by giving priority to leadership support, allocating funds for employee training, and stressing transparent communication about the importance of data.
8. Measuring and Monitoring Progress in Data Maturity
Measuring and tracking advancement are essential components of every organization's data maturity journey, as they guarantee success. To reliably track developments, precise metrics and key performance indicators (KPIs) must be established. The organization's strategic goals and objectives for data maturity should be in line with these measures.
Making educated decisions is possible when the company regularly evaluates where it is in its data maturity journey. Through regular analysis of these measures, stakeholders may pinpoint areas for development, acknowledge accomplishments, and modify tactics as necessary. By using an iterative method, teams may stay focused on achieving their data maturity targets and foster a culture of continuous progress.
In order to assess data maturity effectively, companies need to take into account both quantitative and qualitative indications. Data initiatives can lead to increases in operational efficiency, adoption of data-driven decision-making procedures, or rates of data correctness. These are examples of quantitative measurements. Surveys and interviews may be used as part of qualitative methods to find out how staff members feel about the company's data culture and capabilities.
Putting in place a strong monitoring system guarantees that the organization as a whole can see and understand the progress made. Providing regular updates on important indicators keeps stakeholders informed and involved in the process of achieving data maturity. All organizational levels can better access and comprehend complex data by using visualizations like scorecards and dashboards.
Organizations that use continuous monitoring are better able to recognize patterns or deviations that need to be addressed since they can track trends throughout time. Organizations can proactively address obstacles and seize chances for future growth in their data maturity roadmap by devising a regular review cycle for progress against established KPIs.
Tracking and measuring the advancement of data maturity is essential to the success of a company. Organizations may effectively sail their data maturity journey by building robust monitoring systems, defining clear KPIs that are aligned with strategic goals, routinely measuring progress using quantitative and qualitative indicators, and promoting a culture of continuous development. Watch this space for our upcoming blog article, where we'll dive even further into the latter phases of creating a thorough big data strategy—incorporating knowledge from cutting-edge analytics tools!
9. Best Practices for Sustaining Data Maturity Growth
Sustaining data maturity growth requires a commitment to continuous improvement. Here are some best practices to ensure your organization's data maturity keeps advancing:
1. **Empower Data Literacy**: Invest in training programs to enhance data literacy across the organization, ensuring everyone understands the value and importance of data-driven decision-making.
2. **Cultural Change**: Encourage staff members at all levels to use data in their daily work, creating a collaborative atmosphere that revolves on insights.
3. **Data Governance**: Establish robust data governance policies to ensure data quality, security, and compliance are maintained as the volume and complexity of data grow within the organization.
4. **Agile Approach**: Embrace agile methodologies for managing data projects, enabling quick iterations, adaptability to changes, and efficient collaboration between different teams.
5. **Performance Metrics**: Define key performance indicators (KPIs) that measure the effectiveness of your data initiatives, providing insights into areas that need improvement or optimization.
6. **Innovative Technologies**: Use tools like artificial intelligence (AI) and machine learning to improve your data processing skills. Keep up with new developments in the field of big data and analytics.
7. **Data Integration**: Ensure seamless integration of various data sources within the organization to avoid silos and enable comprehensive analysis for better decision-making.
8. **Feedback Loop**: Create a feedback loop mechanism where insights derived from data analysis lead to actionable outcomes that drive further improvements in your data strategies.
Organizations can continue on their path to greater data maturity by adhering to these best practices, which will open up new avenues for innovation and growth through the thoughtful application of big data analytics.
10. Case Studies: Successful Implementation of Data Maturity Strategies
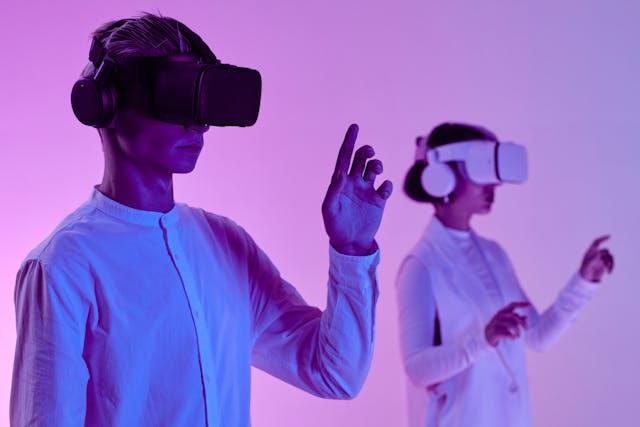
We examine real-world case studies that demonstrate the effective application of data maturity strategies in this last installment of our Big Data Strategy series. These case studies serve as evidence of the importance and effects of creating a thorough data maturity map for organizations.
Case Study 1: Retail Industry
A prominent retail company put its consumer analytics-focused data maturity plan into practice. Through the examination of consumer purchasing patterns, demographic information, and industry developments, the business enhanced its range of products and advertising strategies. As a result, sales income rose, customer happiness rose, and inventory control got better. The company's operations and competitive position in the market were revolutionized by the methodical utilization of data.
Case Study 2: Healthcare Sector
Utilizing data maturity practices, a sizable hospital network enhanced patient care results. By combining real-time patient monitoring devices with electronic health records (EHR), healthcare providers were able to obtain important insights into patient status and treatment effectiveness. Proactive healthcare interventions, a decrease in medical errors, and an improvement in patient satisfaction rates were the outcomes of this.
Case Study 3: Financial Services
A data maturity roadmap with an emphasis on risk management and fraud detection was put into place by a global financial institution. Through the utilization of machine learning and predictive analytics on extensive transaction datasets, the organization was able to detect questionable actions instantly. This proactive strategy improved regulatory compliance while reducing financial risks and protecting consumer assets.
Case Study 4: Manufacturing Industry
A multinational manufacturing company implemented data-driven strategies to optimize its manufacturing workflows. The company collected real-time operational data from IoT sensors put in manufacturing facilities in order to correctly estimate maintenance needs and optimize equipment performance. As a result, manufacturing efficiency rose, downtime was minimized, and total operating expenses were greatly decreased.
These case studies highlight how applying customized data maturity techniques in line with business objectives can have a revolutionary effect. Businesses may cultivate a data-driven culture, build scalable infrastructure, invest in people development, and use advanced analytics technologies to successfully transition into data-driven organizations. Organizations can fully utilize the power of big data when they have a well-defined data maturity map, which also positions them as leaders in their field, ready for long-term success in a highly competitive environment.
As we come to the end of our investigation into Big Data Strategy and its critical role in organizational performance using a Data Maturity Map methodology, we hope that these case studies have given you insightful knowledge on how strategic data management principles are actually applied in a variety of industries. It is now imperative for businesses looking to prosper in the current digital economy to embrace innovation through data-driven decision-making. Keep checking back for more thought-provoking articles about new developments influencing big data and analytics in the future!
11. Challenges in Achieving Higher Data Maturity Levels
Businesses face a number of obstacles on their path to greater data maturity levels, which may impede their development. Lack of executive buy-in and support, inadequate data quality and governance procedures, compartmentalized data management systems, a lack of resources and experience in data analytics, and difficulty integrating diverse data sources are some typical obstacles.
In the absence of robust backing from upper management, efforts aimed at enhancing data maturity could have difficulties in gaining momentum or obtaining the essential resources for their triumph. Decisions based on inaccurate information and erroneous insights might result from poor data governance and quality. Finding relevant analysis at the corporate level is difficult when siloed data management systems provide fragmented, inconsistent, or incomplete data sets across departments.
For companies looking to improve their data maturity, a major obstacle is a lack of resources and experience in data analytics. To solve this issue, it may be necessary to make investments in professional development initiatives, hire qualified personnel, or form alliances with outside specialists. It can be difficult and time-consuming to integrate different data sources from different systems within an organization if the required tactics and resources are not in place.
A comprehensive strategy that tackles organizational culture, talent development, technology infrastructure, and strategic alignment with business goals is needed to overcome these obstacles. Through proactive identification of these challenges and implementation of focused solutions, companies can more skillfully traverse the path towards increased data maturity.
12. Conclusion: The Future of Big Data Strategy and Continuous Improvement
Organizations must have a precise and well-defined data maturity map in order to successfully traverse the difficulties of big data. It acts as a compass to help people navigate the many phases of data maturity, from simple data administration to sophisticated analytics and the creation of insights.
In today's data-driven environment, continual improvement is essential for businesses to remain relevant and competitive as they progress toward utilizing the potential of big data. Through consistent evaluation of their data maturity levels and strategy iterations, companies may adjust to evolving market dynamics, technological breakthroughs, and customer inclinations.
Agility, creativity, and a never-ending quest for excellence in data management and analytics are key components of the big data strategy of the future. Using data-driven insights to acquire a competitive edge and open up new opportunities will require firms to embrace emerging technologies like automation, machine learning, and artificial intelligence.
In summary, the creation of a strong big data strategy backed by an extensive data maturity map is not a one-time event; rather, it is a continual process of progress that will help businesses prosper in the age of digital transformation. Businesses can fully utilize big data to drive growth, improve decision-making, and create sustainable competitive advantages in today's and tomorrow's dynamic business environment by investing in their data capabilities and fostering a culture of innovation and learning.