1. Introduction
Both Big Data and Small Data are essential for guiding decision-making processes in today's data-driven environment. Large volumes of intricate data gathered from multiple sources are referred to as "big data," and extracting valuable insights from it calls for sophisticated analytics techniques. Conversely, Small Data refers to easier-to-manage datasets that provide targeted insights into consumer trends or behavior. Small Data delivers specific insights into individual habits and preferences, but Big Data offers a wide overview and aids in identifying overarching trends. Businesses must comprehend the significance of utilizing both forms of data in order to make well-informed selections that spur expansion and innovation.
2. The Value of Big Data
Large and complicated data sets that are too big and complex to handle well with conventional data processing tools are referred to as "big data." Web traffic, sensor data, social media data, and so on are a few examples. Businesses employ analytics on big data to find patterns, trends, and correlations in areas including consumer behavior, industry trends, and operational effectiveness. With modern analytics techniques like predictive modeling and machine learning algorithms applied to massive amounts of data, it helps businesses to make better decisions, enhance strategies, and obtain competitive advantages.
3. The Role of Small Data
Small Data are datasets that are sufficiently small to allow for human analysis and interpretation without the need of sophisticated software or algorithms. Little Data is on obtaining insights from limited and particular sources, as contrast to Big Data, which incorporates vast amounts of information evaluated using machine learning and other technologies. Customer feedback forms, individual purchase histories, survey replies, and social media interactions are a few examples of little data.
Small data is significant since it can offer in-depth understandings of personal preferences and habits. Through the analysis of modest yet significant datasets, companies can learn more about their clients personally. This knowledge enables businesses to better customize goods, services, and marketing tactics to the particular requirements and preferences of their target market. Small Data enhances Big Data by providing qualitative context and human-centered viewpoints that assist businesses in making better decisions and forging closer bonds with their clientele.
4. Complementary Nature of Big Data and Small Data
In the field of data analysis, big and little data are sometimes perceived as antagonistic, but in practice, they can complement one another to give a more complete view of the corporate environment. Big data gives businesses the ability to identify broad patterns and insights due to its enormous amount and variety of information. Small data, on the other hand, provides a deeper insight of individual actions or preferences by concentrating on tiny, granular bits of information.
Big data, when combined, can reveal broad patterns and trends that might otherwise be missed when concentrating just on smaller datasets. Subsequently, little data can be employed to explore these patterns more thoroughly, offering context and subtlety that big data alone could overlook. For a retail corporation, big data can show that online sales generally rise during the holiday season. The goods driving this trend might then be identified with precision using small data, as well as client categories who are particularly engaged at this time.
The healthcare sector is one more example of this complimentary nature. Large-scale health trends, like a rise in the frequency of a particular disease, can be found by using big data analysis across populations or geographical areas. However, minute information gleaned from each patient's file can provide accurate insights into how various medications are impacting particular patient populations or identify distinct genetic markers for customized care.
To put it simply, large data sets the stage by exposing broad patterns and trends, and tiny data offers the specific context required to draw meaningful conclusions from those patterns. Businesses and organizations can obtain a more comprehensive understanding of their operations and customers by skillfully combining these two methods, which will result in more focused plans and improved overall results.
5. Use Cases
In the field of data analysis, the utilization of big and little data is essential to offering firms thorough insights. Organizations can find patterns, correlations, and trends within enormous datasets by using big data for macro-level trends research. Businesses can obtain a comprehensive understanding of market dynamics, consumer behavior, and industry trends by utilizing big data analytics solutions. Making strategic decisions about product development, marketing tactics, and budget allocation is aided by this kind of analysis.
However, when it comes to micro-level tailored insights, tiny data really shines. Small data is any qualitative or quantitative information that is collected on a smaller scale, yet it nevertheless contains rich context and comprehensive details about the unique needs, preferences, and behaviors of each consumer. Small data, such purchase history, customer feedback, and encounters with the brand at several touchpoints, can be gathered and analyzed by businesses to create individualized experiences that cater to the unique demands of their clientele.
To elucidate this further, consider a retail corporation that leverages big data to study sales patterns across multiple geographies and demographics in order to comprehend the whole market demand for different products. On a larger scale, this macro-level analysis aids in the optimization of price and inventory levels. Simultaneously, by utilizing little data from individual customer interactions and transactions, the business is able to tailor messaging, offers, and suggestions to each client's preferences and purchasing habits.
Furthermore, as I mentioned previously, tiny data offers individualized insights that help companies improve customer experiences on a micro level, while big data offers insightful information about larger trends and patterns that influence strategic decision-making at a macro level. Organizations can develop deeper relationships with individual customers and gain a more comprehensive picture of their target markets by skillfully utilizing both big data and small data analytics methodologies. In today's competitive business environment, the integration of big data for macro-level trends analysis and small data for micro-level individualized insights enables firms to make well-informed decisions that promote growth and client loyalty.
6. Benefits of Utilizing Both
There are several advantages to using both big and little data, and these advantages can have a tremendous influence on how a firm operates. Organisations can augment their decision-making proficiencies by amalgamating the extensive discernments afforded by big data with the intricate subtleties supplied by small data. Big data makes it possible to analyze information at a macro level, uncovering patterns and trends in massive datasets, whereas small data provides more granular, tailored insights that give these conclusions more context.
Better consumer comprehension and satisfaction result from the combination of big and little data. Big data can highlight broad patterns in consumer behavior, tastes, and market dynamics; tiny data, on the other hand, focuses on specific encounters and feedback. With this all-encompassing perspective, companies may better customize their goods and services to match the unique demands and expectations of their clients. Stronger brand loyalty and increased consumer satisfaction rates are possible outcomes of this strategy.
7. Challenges in Incorporating Both Types of Data
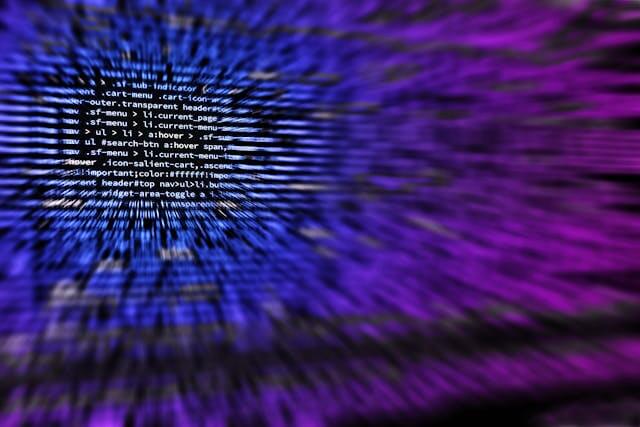
Organizations must successfully handle the problems posed by incorporating both Big Data and Small Data. Big Data can be intimidating in terms of handling the sheer number and variety of information. For businesses to effectively sort through and make sense of this enormous volume of data, they must invest in reliable storage systems and analytics technologies. Making educated decisions in the face of such diversity requires ensuring data relevance and quality.
However, even though Small Data might first appear easier to handle, it can still be difficult to guarantee its authenticity and dependability. Organizations that collect tiny data need to be extremely aware of the techniques employed in data collection because even minor biases or mistakes can have serious repercussions. Maintaining data integrity in small data sets requires the implementation of validation and verification methods.
Integrating these kinds of data successfully necessitates a thorough strategy that tackles these difficulties head-on. Organizations may fully realize the benefits of data-driven insights throughout their operations by utilizing sophisticated analytics techniques for Big Data and strict quality control procedures for Small Data. The combination of the precision of Small Data and the depth of Big Data can provide a comprehensive perspective that successfully informs strategic decision-making.
8. Tools and Technologies for Handling Both
Tools like Hadoop and Spark are essential for effectively managing massive volumes of data in the big data environment. Massive datasets may be processed and stored across computer clusters thanks to Hadoop's distributed file system and processing framework. Conversely, Spark offers a quick and versatile cluster computing system that, thanks to its in-memory computing capabilities, allows for real-time data processing.🤩
Even while these tools are necessary to manage large-scale data analytics, efficiently gathering tiny data is just as crucial to obtaining insightful knowledge. Techniques including feedback forms, observational studies, interviews, surveys, and interviews can assist in gathering small amounts of both qualitative and quantitative data. The limited data that has been gathered can be further refined to support well-informed decision-making by utilizing strategies like sentiment analysis, trend tracking, and consumer profiling.
To achieve thorough insights that support strategic decision-making processes across enterprises and industries, it is essential to strike a balance between big data management technologies and efficient small data collection techniques. By combining these strategies, a comprehensive understanding of data-driven insights that can spur innovation, increase operational effectiveness, and improve overall business performance is ensured.
9. Ethical Considerations
**9. Ethical Considerations**🥰
**Privacy issues when managing huge datasets:** Privacy is a fundamental ethical consideration when it comes to large data. If massive volumes of data are gathered and managed carelessly, there may be breaches of personal information. Businesses and organizations must place a high priority on data security measures in order to safeguard people's right to privacy and stop illegal access.
a. **Maintaining confidentiality and permission with little personal data:** Despite the fact that tiny data may not seem as private as huge data, it is still crucial to guarantee consent and secrecy while working with personal data. No matter the volume of data shared, individuals should have the choice to consent or not and should be fully informed about how their information will be used. Maintaining confidence between data collectors and persons disclosing personal information requires protecting the privacy of tiny datasets.
10. Future Trends
The growing sophistication of big data technology is one trend that is evident when we look to the future of data analytics. Big data analytics is expected to continue to advance quickly, becoming ever more potent and adaptable. This progress could include more integration with artificial intelligence and machine learning systems, better algorithms for handling large volumes of data, and increased real-time analysis capabilities.
However, the use of tiny data is expected to advance in a promising way in the future. Big data analytics can benefit from the depth and specificity that tiny data gives, but little data also offers useful insights on a larger scale. In order to obtain actionable insights and make wise decisions, companies may innovate in the next years in how they use small data sources including individual preferences, consumer feedback, and trends in niche markets. In an increasingly competitive world, businesses can uncover new potential for development and innovation by skillfully integrating the strengths of big data and small data.
11. Conclusion
Based on everything mentioned above, we can say that companies looking to gain deep operational insights must leverage both Big Data and Small Data. Small Data gives precise, nuanced information that can reveal particular client wants and preferences, whereas Big Data offers significant trends and patterns on a larger scale. Businesses can obtain a comprehensive insight of their clients, markets, and operations by merging these two forms of data. To remain competitive and adaptable in today's changing business environment, companies must include both Big Data analytics and Small Data observations into their decision-making processes.
12.Resources and Further Reading
Resources and Further Reading
- Viktor Mayer-Schönberger and Kenneth Cukier's book "Big Data: A Revolution That Will Transform How We Live, Work, and Think" offers a perceptive look at the effects of big data on numerous facets of our existence.
- "Small Data: The Tiny Clues That Uncover Huge Trends" by Martin Lindstrom offers a fresh perspective on the power of small data in understanding consumer behavior and preferences.
Foster Provost and Tom Fawcett's "Data Science for Business: What You Need to Know about Data Mining and Data-Analytic Thinking" is a useful manual that explains how to use both big and little data to effectively inform business choices.
- Tom Davenport's essay in the Harvard Business Review titled "You Don't Have to Be a Big Company to Use Big Data" explores the myth that big data analytics is just beneficial for giant firms and clarifies how small businesses may also leverage its potential.
- The McKinsey & Company paper "Beyond big data: Why business leaders should embrace new paradigms of decision-making" provides insightful viewpoints and case examples for anyone seeking further information on combining big and small data methods.