1. Introduction to Predictive Analytics
Introduction:
With the use of statistical algorithms, machine learning methods, and historical data, predictive analytics is a potent instrument for projecting future events. Predictive analytics can provide important insights into what is likely to happen next by examining trends in data. Businesses are increasingly using predictive analytics to obtain a competitive edge by making educated decisions ahead of time in today's fast-paced and data-driven world. In this article, we'll look at five ways predictive analytics can help you succeed in both your personal and professional life.
2. Understanding the Basics of Predictive Analytics
Utilizing data, statistical algorithms, and machine learning approaches, predictive analytics determines the probability of future events based on past data. Businesses can anticipate future events and make well-informed decisions by examining historical trends and habits. This gives businesses the ability to foresee developments, act pro-actively, and obtain a competitive advantage in their sector.
Understanding the three main elements of predictive analytics—data collection, data analysis, and predictive modeling—is crucial to understanding the field's fundamentals. Data collecting is the process of compiling pertinent information from a range of sources, including social media, surveys, consumer databases, and more. To extract valuable insights, data analysis involves organizing, processing, and cleansing the data. Statistical algorithms are used in predictive modeling to create models that project future trends or results based on past patterns.
Businesses may improve decision-making procedures in a variety of departments, including marketing, sales, finance, operations, and customer support, by utilizing predictive analytics. Organizations can use it to forecast client behavior, spot possible dangers and opportunities, allocate resources optimally, boost operational effectiveness, and spur overall company growth. To put it simply, knowing the fundamentals of predictive analytics is essential to maximizing its potential for informed decision-making and long-term success.
3. The Impact of Predictive Analytics on Decision Making
Predictive analytics is crucial in the field of decision-making since it offers insightful information derived from data analysis. Organizations are able to make well-informed decisions about a range of operational factors by utilizing both historical and current data. This makes it possible to take a more proactive stance while dealing with possible issues and seizing new chances.
Predictive analytics has a big influence on decision-making since it can more accurately predict future patterns and results. Organizations are able to predict changes in consumer behavior, market fluctuations, and operational hazards by examining patterns and trends within data sets. Decision-makers can take preventative measures that minimize risks and optimize benefits thanks to this foresight.
Decision-makers are empowered by predictive analytics, which provides customized suggestions based on certain scenarios. Organizations may optimize marketing tactics, product recommendations, and consumer segmentation by utilizing machine learning algorithms and sophisticated analytical tools. This degree of personalization guarantees that choices are made in accordance with personal tastes or corporate goals, producing better results.
Optimizing operational efficiency and resource allocation is achieved through the use of predictive analytics into decision-making procedures. Organizations can efficiently distribute resources and streamline procedures by finding workflow bottlenecks or inefficiencies. This optimization increases overall productivity and promotes an ongoing improvement culture inside the company.
By modeling numerous what-if scenarios to evaluate possible outcomes, predictive analytics supports scenario planning and risk management. In uncertain situations, decision-makers can assess various courses of action using predictive models to identify the best path of action. This proactive risk assessment reduces unanticipated costs and directs strategic choices toward advantageous outcomes.
Based on the aforementioned, it can be inferred that predictive analytics has a significant influence on decision-making processes, transforming the way businesses handle intricate problems and prospects. Decision-makers can make well-informed decisions that benefit their companies by utilizing the power of data-driven insights. Not only can the incorporation of predictive analytics into decision-making procedures improve strategic planning, but it also cultivates an innovative and flexible culture in companies that adopt this game-changing technology.
4. Improving Business Strategies with Predictive Analytics
The application of predictive analytics is crucial to advancing corporate initiatives. Through the utilization of sophisticated algorithms and data, businesses are able to make well-informed decisions that lead to success. Market trend forecasting is a crucial method predictive analytics improves business plans. Businesses may more accurately forecast future trends by examining past data and patterns, which gives them the opportunity to modify their tactics in advance. Businesses are able to take advantage of new opportunities and maintain an advantage over competitors because to this foresight. 😏
Marketing activities can be optimized with the use of predictive analytics. Businesses can customize their marketing strategy to target the correct audience with individualized campaigns by researching customer behavior and preferences. Improved ROI results from increased engagement and conversion rates brought forth by this modification. Businesses can focus their resources on high-value clients and increase customer loyalty by using predictive analytics to forecast customer lifetime value.
One of the most important aspects of company strategy risk management is predictive analytics. Through the examination of many elements and metrics, including market dynamics and internal functions, enterprises can detect possible hazards prior to their exacerbation. Businesses may efficiently minimize risks and make educated decisions to safeguard their assets and reputation by using this proactive approach.
Increasing operational efficiency is a key advantage of using predictive analytics into corporate plans. Businesses can increase productivity by streamlining operations, allocating resources more effectively, and optimizing procedures by utilizing data insights. Businesses can improve overall performance by making focused interventions after identifying bottlenecks and inefficiencies.
Taking into account everything mentioned above, we can say that using predictive analytics in business plans enables organizations to make more informed decisions using data-driven insights. Predictive analytics provides a competitive edge that propels success in today's fast-paced corporate environment, from predicting market trends to improving marketing efforts and successfully managing risks. Adopting this technology helps companies stay ahead of the curve, creative, and adaptable in a market that is becoming more and more competitive.
5. Enhancing Customer Experience through Predictive Analytics
Predictive analytics is a great tool for organizations trying to stay ahead in the current competitive market when it comes to improving the customer experience. Businesses can obtain important insights into the behavior, interests, and trends of their customers by utilizing predictive analytics. This makes it possible for companies to customize their goods and services to match the unique requirements of their clients, which eventually boosts client happiness and loyalty.
Using predictive analytics to personalize marketing initiatives is one strategy to improve customer experience. Businesses can forecast future actions and adjust marketing strategies by studying historical behavior and preferences. Showing clients items or services that are relevant to their needs strengthens the relationship with them and raises the possibility that they will convert.
Businesses can anticipate client wants before they materialize with the aid of predictive analytics. Businesses can proactively give answers or recommendations to customers by evaluating data on past purchases, browsing habits, and other interactions. Customers are delighted by this proactive attitude, which also gradually fosters loyalty and confidence.
The customer journey can be optimized with the use of predictive analytics. Businesses can find areas for customer experience enhancement or pain points by evaluating data on touchpoints across several channels. This enables businesses to implement focused adjustments that improve the client experience overall, resulting in higher satisfaction and retention rates.
Businesses may identify at-risk clients before they leave by using predictive analytics. Through the analysis of multiple variables, including feedback scores, interaction frequency, and usage habits, businesses may identify high-risk clients and take proactive measures to keep them around. Long-term, this focused retention approach improves client connections while simultaneously conserving resources.
Based on the aforementioned, it is evident that organizations hoping to prosper in the current competitive environment must use predictive analytics to improve customer experience. Businesses can create meaningful experiences that encourage customer pleasure and loyalty by personalizing marketing efforts, anticipating customer needs, improving the customer journey, and early identification of at-risk clients.
6. Implementing Predictive Analytics in Healthcare for Better Patient Outcomes
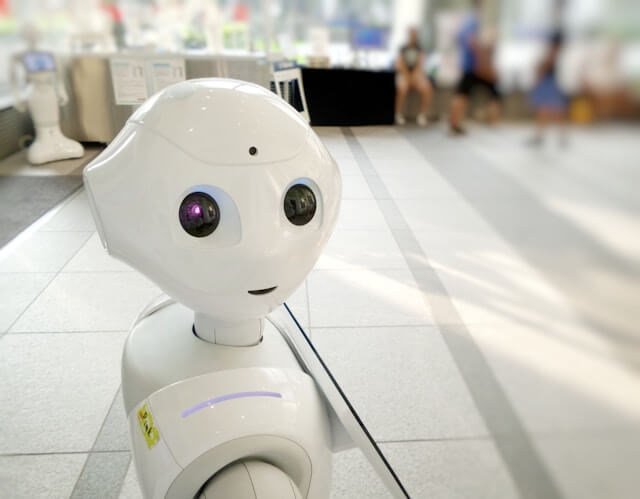
Patient care and results are being revolutionized in the healthcare industry by the use of predictive analytics. Healthcare professionals can foresee possible health issues before they worsen, resulting in more proactive and individualized patient care, by utilizing sophisticated algorithms and historical data. The capacity to recognize high-risk patients and take early action to avert problems or readmissions to the hospital is a significant advantage. This proactive strategy not only lowers healthcare expenditures dramatically but also improves patient outcomes.
By forecasting how certain patients may react to particular therapy or medications, predictive analytics in healthcare can also improve treatment regimens. Healthcare professionals can better meet each patient's individual needs by customizing therapies based on the patient's medical history, genetics, lifestyle choices, and other factors. This increases the likelihood of positive results. In order to make sure that personnel, supplies, and prescription drugs are available when and where they are most required, predictive analytics can assist healthcare institutions allocate resources as efficiently as possible.
Predictive analytics in healthcare enables more precise disease outbreak and public health trend forecasting. Public health professionals can more accurately anticipate and be ready for health emergencies by evaluating data from multiple sources, including social media, electronic health records, and environmental factors. By being proactive, epidemics can be contained more quickly and their negative effects on communities are lessened.
Predictive analytics is a useful tool in healthcare that helps both individual patients and advances medical research and development. Researchers can find patterns and correlations through the analysis of massive amounts of data from many sources, which could result in the development of novel treatments or interventions. This data-driven strategy quickens the rate of medical innovation and, in the end, raises the standard of treatment that patients everywhere can get.
Healthcare practitioners can transition to a more patient-centered approach that emphasizes preventive, individualized care, and better results by utilizing predictive analytics. By incorporating data-driven insights into clinical decision-making, healthcare delivery is revolutionized and the groundwork for a better future for all is laid.
7. Overcoming Challenges in Adopting Predictive Analytics
Businesses may face difficulties when implementing predictive analytics, but getting past these issues is necessary to realize the full benefits of this technology. The need for qualified staff, interaction with current systems, problems with data quality, opposition to change, and expensive implementation expenses are a few typical obstacles. Companies can spend money on expert hiring or training to close the skill gap. Making changes to current systems in order to integrate predictive analytics may be necessary, but doing so can result in better decision-making. Reliability and accuracy of data are essential for predictive models to work well.
Predictive analytics adoption may be hampered by organizational resistance to change. This problem can be solved by integrating staff members in the process and teaching them about the advantages of this technology. Although there are financial risks, investing in predictive analytics can pay off in the long run by increasing revenue and improving efficiency. Businesses can use predictive analytics to achieve a competitive edge in today's data-driven world by proactively tackling these difficulties.
8. Key Benefits of Using Predictive Analytics in Finance and Investment
The application of predictive analytics is essential for improving decision-making in the investment and finance industries. Risk management is a major advantage. Businesses can implement proactive risk mitigation methods by using predictive analytics to better predict market swings and evaluate possible risks before they arise.
Predictive modeling is a major benefit for portfolio management. Predictive analytics can assist in optimizing investment portfolios by avoiding potential losses and identifying profitable possibilities based on trends and patterns by using historical data and sophisticated algorithms.
In the financial sector, predictive analytics helps with fraud detection and prevention. Financial institutions can mitigate the risks of fraudulent conduct and protect assets by swiftly flagging suspicious behaviors based on real-time analysis of transactional data.
By analyzing behavioral patterns and preferences, predictive analytics improves consumer insights and allows for the customization of individualized financial offers. By properly meeting each customer's unique needs, this not only increases customer satisfaction but also retention rates.
Finally, the ability to accurately foresee financial trends is essential for making well-informed investment decisions. Through data-driven insights, predictive analytics gives professionals the capacity to more accurately estimate market moves, allowing them to take advantage of profitable opportunities while limiting risks related to uncertainty.
9. The Role of Machine Learning in Powering Predictive Analytics
Machine learning is a key component of predictive analytics, enabling precise forecasts and insights. Large data sets are combed through by machine learning algorithms to find patterns and relationships that may be difficult for people to see. Machine learning can predict future trends with high accuracy by using past data to train models.
Predictive analytics is made possible by machine learning, which continuously learns from new data inputs, adjusts to shifting trends, and gradually increases prediction accuracy. Regression analysis, decision trees, neural networks, and clustering are just a few of the methods that machine learning algorithms use to find patterns hidden in datasets and produce forecasts that help with strategic decision-making.
Complex, nonlinear interactions within data sets that standard statistical methods could miss can be handled by machine learning algorithms. Rather than depending just on human intuition or crude analytics, this capacity enables organizations to make better educated decisions based on a holistic perspective of their data landscape.
Businesses can improve forecasting accuracy, streamline operations, spot growth possibilities, proactively reduce risks, and gain a competitive edge in today's data-driven industry by utilizing machine learning in predictive analytics. Organizations may efficiently extract meaningful insights from their data and make better business decisions across a range of industries by integrating machine learning with predictive analytics.
10. Real-life Examples of Successful Predictive Analytics Implementation
Sure! Here are some paragraphs for the blog post section on real-life examples of successful predictive analytics implementation:
1. **Retail Industry**: Predictive analytics has been successfully applied by retailers such as Amazon and Walmart to forecast customer demand, optimize pricing tactics, and personalize recommendations. These organizations are able to predict consumer behavior through the analysis of prior purchase habits and external factors such as weather and economic trends. This allows them to create more focused marketing campaigns and boost sales.
2. **Healthcare Sector**: By enhancing patient outcomes and cutting expenses, predictive analytics has completely transformed the healthcare industry. For example, institutions such as Memorial Sloan Kettering Cancer Center employ data-driven models to forecast the possibility of readmission or the risk of surgical complications for their patients. This makes it possible for medical professionals to act quickly, customize care, and improve the standard of care overall.
3. **Financial Services**: Predictive analytics is used by banks and other financial organizations to better identify fraud, evaluate credit risks, and customize products for clients. Companies can quickly identify suspicious activity, stop fraudulent transactions, and uphold client trust by using machine learning algorithms that evaluate transactional data in real time.
4. **Transportation Industry**: Predictive analytics is used by Uber and Lyft, among other companies, to better plan routes, estimate fares based on changes in demand, and reduce wait times for passengers. Through the processing of vast quantities of data on user locations, traffic patterns, and past ride requests, these businesses guarantee effective operations and raise customer satisfaction levels.
5. **Manufacturing Sector**: The use of predictive analytics is essential in foretelling equipment malfunctions in manufacturing facilities before they happen. Manufacturers may schedule preventive maintenance work proactively, decrease downtime, increase production efficiency, and ultimately save money by analyzing historical maintenance data and real-time monitoring of machinery performance metrics.
These actual cases demonstrate the wide range of industries in which predictive analytics is being applied as well as how it is revolutionizing decision-making. Businesses that leverage the potential of predictive analytics stand to gain a competitive edge in today's data-driven world. This includes improving customer experiences, increasing operational efficiencies, and successfully reducing risks.
11. Ensuring Data Privacy and Security in Predictive Analytics Applications
Maintaining client trust and complying with regulations require predictive analytics technologies to ensure data privacy and security. Sensitive data can be protected using encryption techniques all the way through the data analysis process. By putting access controls in place, the danger of unauthorized access is decreased because only authorized workers are able to touch and view sensitive data. Frequent monitoring and audits assist in quickly identifying any anomalies or breaches, enabling timely risk mitigation.
It is possible to preserve individual identities while yet deriving insightful information from datasets by using anonymization techniques. Businesses can efficiently use predictive analytics while adhering to privacy rules by eliminating personally identifiable information. It's also critical to keep up with changing compliance requirements and data protection laws so that security measures may be adjusted appropriately and data integrity can be protected in a proactive manner.
Apart from the technical safeguards mentioned earlier, it is crucial to have explicit policies and processes for the management and storage of data in predictive analytics systems. Regular training sessions on data privacy best practices for staff members promote an awareness and accountability culture within the company. Businesses may show their dedication to safeguarding consumer information while utilizing predictive analytics to make well-informed decisions by integrating privacy considerations into every phase of the data analytics lifecycle.