1. Introduction: Discuss the increasing relevance of data scientists in today's tech-driven world.
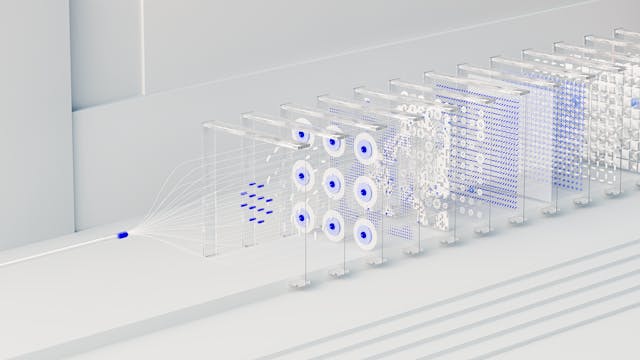
In the technologically advanced world of today, data scientists are becoming increasingly important. As the amount of data created by both individuals and corporations grows exponentially, data scientists are becoming increasingly important in helping to glean useful insights from this massive amount of data. When it comes to sifting through large, complicated data sets to find patterns, trends, and correlations that can guide organizations' decision-making and spur innovation and growth, data scientists are indispensable. The need for qualified data scientists is only going to grow as businesses in all sectors depend more and more on data-driven strategies to remain competitive.
Thanks to technological improvements, businesses can now gather vast volumes of data from a variety of sources, including online transactions, social media, and sensors. But the usefulness of all this data is dependent on the conclusions that may be drawn from it. Data scientists are qualified to sort through this deluge of information, use cutting-edge analytics methods, and convert unprocessed data into meaningful insights that may inform operational and strategic decisions. In addition to giving businesses a competitive edge, their capacity to interpret data facilitates a deeper understanding of client preferences, market trends, and operational inefficiencies.
The machine learning models and algorithms that drive cutting-edge solutions like recommendation systems, predictive analytics, fraud detection systems, and autonomous decision-making processes are created in large part thanks to the work of data scientists. These smart solutions increase product offers, streamline corporate procedures, and personalize user experiences in addition to increasing operational efficiency. Data scientists are becoming more and more important as businesses look to use automation and artificial intelligence to improve consumer experiences and streamline operations.
Furthermore, as I mentioned previously, data scientists are becoming more and more relevant in today's tech-driven world, which emphasizes how crucial it is for businesses to use data to spur innovation and success. Businesses will need the skills of data scientists to unlock actionable insights that can guide strategic decision-making as they continue to navigate an increasingly complex digital landscape marked by massive amounts of information, rapid technological advancements, and changing consumer behaviors. Businesses must embrace this big data era by realizing the need of experienced data scientists in converting unstructured data into insightful knowledge that drives long-term success.
2. The Role of Data Scientists: Explore the key responsibilities and functions of data scientists in various industries.
In today's data-driven world, data scientists are essential to a variety of sectors. Large-scale data collection, analysis, and interpretation are among their main duties in order to support organizations in making defensible judgments. Predictive modeling is a tool used by data scientists in finance to evaluate risks and identify fraud. Patient data is analyzed in the healthcare industry to enhance treatment results and optimize workflow. Data scientists are used by retail organizations to forecast consumer behavior and optimize pricing tactics.
The machine learning algorithms that drive recommendation systems in streaming and e-commerce platforms are developed in large part by data scientists. They concentrate on vehicle predictive maintenance and route optimization in the transportation sector. Data scientists make a substantial contribution to marketing as well by seeing patterns, developing models for customer segmentation, and refining advertising strategies using data insights. Their influence cuts across many industries, leveraging the potential of data interpretation and analysis to stimulate efficiency and innovation.
3. Impact of Data Scientists on Business: Highlight how data scientists contribute to decision-making, innovation, and competitive advantage for businesses.
In order to support businesses in making well-informed decisions, data scientists are essential. They offer insightful analysis of intricate data sets, assisting firms in making strategic decisions that might boost productivity and profitability. Their capacity to identify important patterns and trends in data enables businesses to make more accurate and confident data-driven decisions.
Data scientists play a key role in the innovative development of novel goods and solutions. They aid in the development of novel tools, models, and algorithms that have the power to transform entire sectors of the economy and propel technical breakthroughs. Their knowledge helps companies remain ahead of the curve by using data to efficiently innovate and adjust to shifting market conditions.
Data scientists use advanced analytics to find opportunities and hazards that are hidden from view, giving businesses a competitive advantage. By forecasting consumer inclinations, market trends, and corporate results, they assist firms in identifying opportunities for expansion and improvement while averting possible risks. By taking a proactive stance driven by data insights, companies can surpass their rivals and hold top spots in their respective sectors.
Data scientists have a huge impact on business. These experts are vital assets for any company hoping to prosper in the digital era, from improving decision-making procedures to encouraging innovation and creating competitive advantage. In today's data-driven world, businesses that leverage data science can achieve substantial advantages in terms of productivity, creativity, and competitiveness in the market. As such, hiring data scientists is essential to long-term success.
4. Skills Required to Become a Data Scientist: Outline the essential technical and soft skills needed to excel in this field.
Mixing hard and soft abilities is essential for success as a data scientist. Technical talents include knowledge of statistical modeling and machine learning methods, as well as proficiency in programming languages like Python, R, and SQL. Another essential skill is familiarity with data processing and visualization programs like Tableau, Matplotlib, and Pandas. Proficiency in mathematics and statistics is necessary for conducting effective analysis of intricate datasets.
Soft skills are just as important to a data scientist's success as technical expertise. To effectively communicate complex findings to stakeholders who are not technical, one must possess effective communication skills. The ability to solve problems facilitates the handling of complex data difficulties, and accuracy in reporting and analysis is ensured by attention to detail. Innovative methods for data exploration and interpretation are fostered by creativity.
In order to match their analysis with business goals, a data scientist should have a deep understanding of business strategy and the organization they work for. Since the field of data science is expanding quickly and requires professionals to constantly learn new skills and update their skill set in order to remain relevant, adaptability is another essential soft talent.
Based on the aforementioned, it can be inferred that an effective data scientist should possess a blend of technical expertise and strong soft skills, such as problem-solving, communication, creativity, business acumen, and adaptability. People can succeed in this exciting and demanding area by learning these interpersonal skills as well as the technical components of data analysis.
5. Challenges Faced by Data Scientists: Identify common obstacles encountered by data scientists and strategies to overcome them.
Data scientists face several challenges in their roles, including:
1. **Data Quality**: Analysis and modeling can be less successful when dealing with data that is erroneous, inconsistent, or incomplete. Data scientists should give data cleaning and preparation procedures top priority in order to overcome this obstacle.🖐
2. **Lack of Resources**: The data science process may lag if appropriate tools, technologies, or processing capacity are not available. It can be necessary for data scientists to push for improved resources inside their company or look into different approaches.
3. **Interpreting Results**: It might be difficult to explain complicated findings to stakeholders who might not be technical experts in a way that is clear and understood. Simplifying their findings and employing visualizations to improve understanding should be the main priorities for data scientists.
4. **Ethical Concerns**: A vital aspect of data science is making sure that data is used sensibly and ethically. Data scientists have to deal with concerns like algorithmic bias, user privacy, and GDPR compliance. 🤩
By recognizing these challenges and putting measures in place to overcome them, data scientists can improve their capacity for problem-solving and make more meaningful contributions to the success of their businesses.
6. Data Science Tools and Technologies: Review popular tools, software, and programming languages used by data scientists for analysis and visualization.
To glean insights from data and provide visuals that might facilitate decision-making, data scientists employ a variety of techniques and technologies. Programming languages like Python and R, which are strong and adaptable for statistical modeling, data analysis, and manipulation, are some of their most well-liked tools. Jupyter Notebooks and other software programs offer an interactive environment for writing code and creating data visualizations.
Tools like Pandas in Python provide effective ways to handle big datasets with simplicity when it comes to data cleaning and transformation activities. To successfully explain findings, data scientists can generate informative charts and graphs with the use of visualization frameworks like Matplotlib and Seaborn. Interactive dashboards for a more engaging data exploration experience are made possible by technologies like Tableau or Power BI for more complex visualization requirements.
Tools for machine learning, such TensorFlow and Scikit-learn, are crucial for creating algorithms and prediction models that can find patterns in datasets. These libraries enable data scientists to create sophisticated solutions suited to particular analytical problems by offering a broad range of methods, from straightforward linear regression to intricate neural networks.
Technologies like Spark and Apache Hadoop enable data scientists to process large amounts of data effectively in the field of big data. These frameworks facilitate distributed computing among machine clusters, allowing for large-scale parallel processing of activities like modeling, data preprocessing, and analysis.
The field of data science has a wide range of techniques and technologies that are always changing to suit the demands of more difficult analytical problems. Data scientists can navigate complex datasets with creativity and precision and derive important insights that influence decisions in a variety of industries when they have a solid understanding of these technologies.
7. Ethical Considerations in Data Science: Address ethical dilemmas related to data collection, privacy, bias, and transparency in the field of data science.
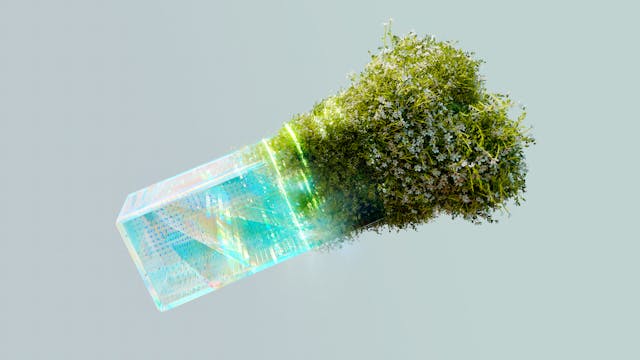
Data science decisions on data collecting, privacy, bias, and transparency are heavily influenced by ethical considerations. It is the responsibility of data scientists to resolve these moral conundrums so that their work maintains the greatest standards of honesty and respect for people's rights.
Data scientists need to be careful to get consent while collecting data, especially when handling private or sensitive information. Maintaining confidence between businesses and their stakeholders depends on protecting people's privacy and making sure that data is anonymized and used appropriately.
Another major ethical challenge in data analysis is dealing with bias. Data scientists must be conscious of any potential biases in datasets that can support unfair treatment or discrimination. Promoting justice and equity in decision-making requires carefully examining algorithms and systemic mechanisms to mitigate these biases.
In order to promote trust in data science approaches, transparency is essential. Data scientists should be transparent about their methods, data sources, and any restrictions or unknowns around their research. By being open and honest about their work, they can guarantee accountability and give stakeholders the ability to judge the reliability of their conclusions.
To put it succinctly, ethical considerations are fundamental to how data scientists do their business. Data scientists may significantly improve society while defending individual rights and advancing social good by adhering to the values of respect, integrity, fairness, and transparency.
8. Future Trends in Data Science: Predict upcoming trends, technologies, and advancements that will shape the future of data science.
It is anticipated that data science will keep developing quickly in the future in response to advances in technology. The growing use of AI and machine learning algorithms, which facilitate faster data processing and decision-making, is one significant trend. The combination of big data analytics and the Internet of Things (IoT) is another new trend that is gaining traction. This combination enables real-time insights from a large array of linked devices.📑
The development of deep learning and natural language processing (NLP) is also anticipated to have a big impact on data science in the future. These tools will improve data scientists' ability to glean insightful information from unstructured data sources like text, audio, and video. As corporations gather and examine more sensitive data, ethical concerns about data security and privacy will become increasingly important.
Interdisciplinary cooperation between data scientists, subject matter experts, and business stakeholders will be more crucial as data science develops. Proficiency in translating technical insights into strategic business decisions and successfully communicating findings are critical competencies for individuals working in this industry. Data science has a bright future ahead of it, one that might spur innovation across industries and open up new avenues for expansion and advancement.