1. Introduction
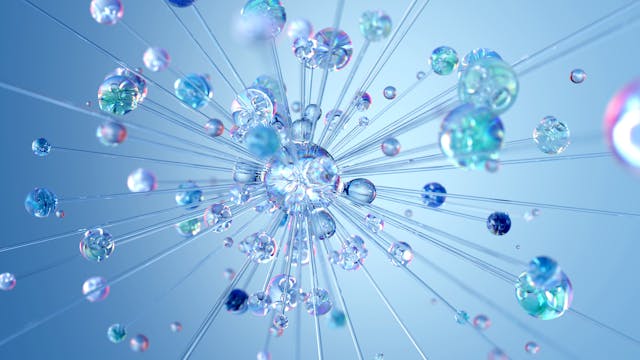
Big data analytics on sites like Twitter is a potent tool for analyzing and forecasting a wide range of phenomena in the era of digital information. Through the utilization of this analytical approach, researchers and health professionals can obtain valuable insights about public health trends, patterns, and feelings by leveraging the massive amount of data that is generated on Twitter every day.
Accurately predicting the spread of diseases is essential for managing them and controlling outbreaks. Experts may track real-time conversations and discussions about illnesses, symptoms, and medical treatments on Twitter by using big data analytics. This knowledge helps them to foresee possible outbreaks, pinpoint high-risk regions, and effectively deploy resources to stop the disease from spreading. Precisely predicting the spread of disease has the potential to prevent deaths and lessen the worldwide effects of pandemics.
2. Understanding Big Data in Disease Monitoring
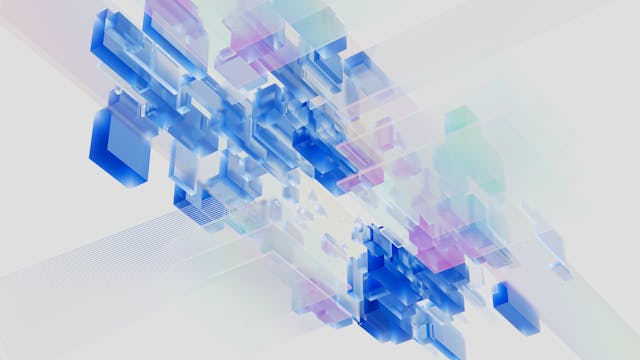
Twitter data can be quite helpful in disease monitoring as it offers up-to-date information on public health trends. Potential patterns of disease propagation can be identified by analyzing tweets about symptoms, outbreaks, and preventive efforts using sophisticated analytics and algorithms. Health officials can more effectively plan for and contain epidemics with the use of this real-time data in addition to more conventional surveillance techniques.
Nevertheless, there are unique difficulties associated with using Twitter data for illness surveillance. A significant challenge is ensuring data dependability and correctness because tweets can be context-free or contain inaccurate information. It is also crucial to respect user privacy and ethical standards when gathering and evaluating such enormous volumes of personal information. Notwithstanding these difficulties, social media data offers enormous potential for forecasting the spread of disease. The rapidity of information collection and analysis presents a potential benefit for early detection and response initiatives, ultimately supporting successful public health interventions.🧐
3. Big Data Analytics Techniques in Disease Prediction
Big data analytics approaches are crucial for efficiently utilizing Twitter data in the field of disease prediction. Algorithms for data mining and machine learning are frequently used to search through enormous volumes of tweets for insightful content. To find trends linked to disease outbreaks, methods like sentiment analysis, network analysis, and clustering are used. These techniques assist in sifting through the noise of social media data in order to find relevant information.
To detect possible disease outbreaks early on, real-time surveillance is crucial. Real-time Twitter data analysis makes it possible to quickly spot patterns and abnormalities that could point to a health emergency. Through the proactive monitoring of Twitter conversations, hashtags, and geotags, public health experts can proactively address emerging hazards prior to their transformation into full-blown epidemics. The efficacy of control and preventative measures can be strongly impacted by the timeliness of these insights.
Proactive and prompt reactions to possible health risks are made possible by integrating big data analytics techniques with Twitter's disease prediction feature. Through the utilisation of real-time monitoring and sophisticated algorithms, public health authorities can augment their capacity to predict, monitor, and alleviate the transmission of illnesses among populations.
4. Case Studies: Successful Applications of Twitter Data in Disease Spread Prediction
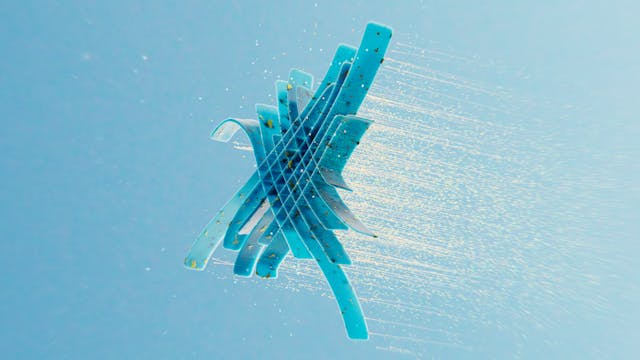
Big data analytics on Twitter has shown to be an effective tool for tracking and forecasting the spread of diseases in recent years. Its significance for foretelling outbreaks and directing public health initiatives is illustrated by a number of fruitful case studies. For example, during the 2016 Zika virus outbreak, scientists used Twitter data analysis to pinpoint areas that might be at risk based on messages regarding symptoms, travel habits, and insect encounters. Early warnings from this strategy helped with resource allocation and focused actions.
Predicting flu epidemics using data from Twitter is another noteworthy example. Through the analysis of tweets referencing flu symptoms or associated phrases, researchers were able to predict influenza patterns before more conventional surveillance techniques. Authorities were able to effectively promote preventative measures and modify the distribution of healthcare resources as a result of this early detection. The incorporation of social media data into models for illness forecasting has improved the predictability and promptness of outcomes, which has in turn resulted in more proactive public health interventions.
These Twitter-based predictive algorithms have a substantial influence on public health measures in addition to predicting. Through the integration of real-time data from social media platforms such as Twitter, health professionals can customize interventions based on targeted geographic regions or demographic groups that are more susceptible. By quickly addressing possible hotspots, this tailored approach not only maximizes resource usage but also boosts the efficacy of mitigation activities.
The way public health officials respond to developing health emergencies has changed dramatically as a result of using big data analytics on Twitter to predict illness transmission. Social media platforms provide enormous volumes of real-time data that can be used to proactively predict outbreaks, distribute resources effectively, and carry out interventions in a timely manner. As technology develops, it will be essential to incorporate these cutting-edge methods into current surveillance systems to improve readiness and reaction tactics for potential disease threats.
5. Ethical Considerations and Challenges
Ethical issues and difficulties need to be taken into account when using big data analytics on Twitter to predict the spread of disease. The use of social media users' personal information for health monitoring raises privacy concerns. There are serious ethical issues that need to be carefully navigated in light of the possible misuse or illegal access to people's private information.
The study of Twitter data is hampered by biases and constraints. Due to the possibility that Twitter users do not accurately or completely represent the community, the data analysis may contain ingrained socioeconomic or demographic biases. Recognizing these constraints is essential to guaranteeing the precision and dependability of predictions about the spread of disease derived from Twitter analytics.🔖
Comprehending and tackling these ethical dilemmas and obstacles is crucial to utilizing big data analytics on Twitter efficiently for forecasting the development of diseases while maintaining confidentiality and minimizing partialities in the examination procedure.
6. Benefits of Proactive Disease Surveillance Using Big Data Analytics on Twitter
Using big data analytics on Twitter to do proactive disease surveillance has major benefits for controlling and stopping the spread of disease. Healthcare authorities can quickly implement preventive measures by using predictive analytics to forecast possible epidemics. This proactive approach effectively stops the spread of illnesses before they worsen by enabling early intervention tactics like focused public health campaigns or more access to healthcare in high-risk locations.
An important advantage of using big data analytics on Twitter for disease prediction is the possible financial savings from early discovery and containment. Health organizations can better spend resources by seeing patterns and trends in social media discussions about symptoms and contagion. By distributing resources strategically, intervention measures are implemented where they are most required, cutting down on waste and optimizing the use of scarce healthcare resources.📍
Essentially, by utilizing big data analytics on Twitter for illness surveillance, medical practitioners are better equipped to anticipate possible health emergencies. Authorities can prevent the spread of diseases by using early prediction capabilities and effective resource allocation based on real-time social media data. This would eventually protect public health and reduce the financial burdens associated with outbreaks.
7. Collaborations Between Health Authorities and Tech Companies
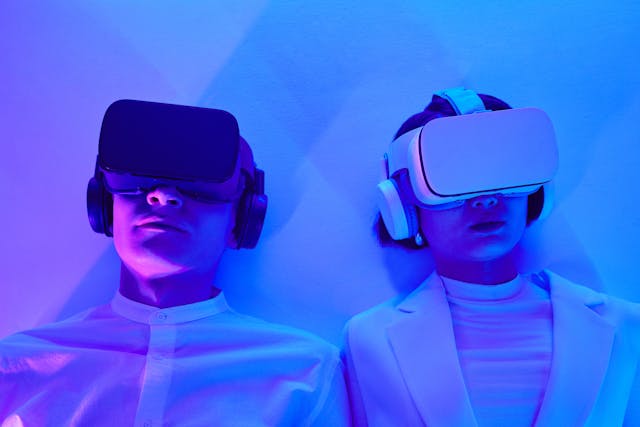
Effective collaboration between health authorities and technology companies is becoming more and more important in order to accurately predict the spread of diseases using big data analytics. Researchers, technology companies, and public health organizations are collaborating to use large datasets from Twitter and other platforms for better illness predictions. Predictive models and tactics can be improved by combining cutting-edge technologies and public health experience thanks to the collaboration between these sectors.
The utilization of big data analytics on social media sites like Twitter can significantly enhance public health results when interdisciplinary collaboration is employed. A more thorough picture of illness trends is produced by fusing the technological prowess of data analytics businesses with the clinical expertise of health authorities. In the end, this cooperative strategy results in more effective disease control and prevention strategies by enhancing prediction algorithms and enabling prompt responses and interventions.💬
8. Future Directions in Predicting Disease Spread with Big Data Analytics
Future developments in big data analytics techniques and technology may significantly improve the precision and promptness of disease transmission prediction on social media platforms. Using machine learning algorithms to better evaluate large volumes of data in order to spot early warning indications of disease outbreaks is one exciting avenue to pursue. To find pertinent health-related information rapidly among the vast amounts of social media posts, better natural language processing technologies might also be created.
Even with these possible developments, there could be a number of difficulties as big data analytics for predicting the spread of disease on social media sites like Twitter develops further. Ensuring data privacy and security during the collection and analysis of sizable datasets containing private health information is a key challenge. Disease forecasts may become inaccurate as a result of problems with data reliability and quality that arise with increasing data volumes. Another issue that must be carefully considered in order to guarantee justice and equity in illness prediction algorithms is addressing cultural biases ingrained in social media data.📦
9. Implementing Strategies Based on Predictive Analytics
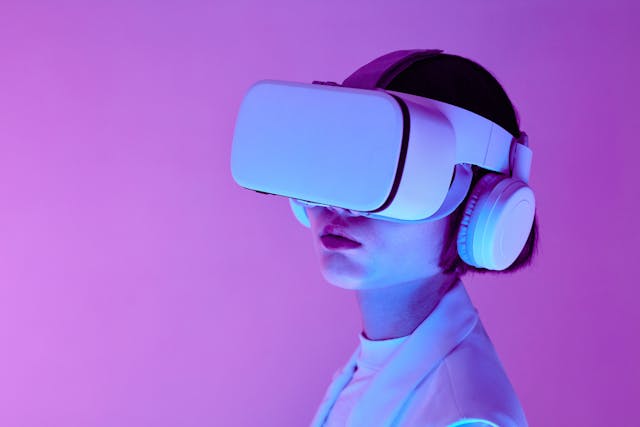
Adopting tactics based on big data-derived predictive analytics has the potential to completely transform the way communities, healthcare providers, and legislators approach illness prevention and control. These stakeholders can proactively carry out targeted interventions like public health campaigns, resource allocation, and vaccination drives by using insights from Twitter analytics to predict the spread of disease. By keeping an eye on important indicators like infection rates, hospital admissions, and death figures, one may assess how well these tactics are working to avoid or lessen the effects of infectious diseases. Decision-makers can more effectively and efficiently maximize their response efforts to stop the spread of diseases by utilizing real-time data and predictive modeling.
Predictive analytics can be used by policymakers to foresee illness outbreaks and adjust policies accordingly. For example, governments can deploy resources to expand healthcare capacity or start educational initiatives encouraging preventive measures like hand cleanliness or mask-wearing if they notice a spike in flu-related tweets in a particular area. By using social media data analysis to identify high-risk individuals, healthcare providers can leverage predictive analytics to give targeted interventions like early screening programs or outreach activities. Additionally, communities can make a significant contribution by using big data analytics insights to embrace preventive practices and assist local health authorities in putting proactive measures into place. A comprehensive approach to disease control can be accomplished by promoting collaboration among many stakeholders on the basis of predictive insights.
Proactive strategies powered by big data analytics are successful when they can quickly and precisely address possible health risks. For instance, examining Twitter data during the COVID-19 pandemic assisted in locating newly emerging infection hotspots and attitudes around vaccination hesitancy or pandemic restrictions. Decision-makers were better able to address public concerns using open communication techniques and allocate resources where they were most needed thanks to the information provided. Stakeholders can adapt their intervention tactics dynamically in response to changing public attitude or emerging trends by tracking shifts in online conversations about health subjects in real-time.
Evaluating pertinent health outcomes against predetermined benchmarks or historical data is necessary to gauge the effectiveness of predictive analytics-informed initiatives. One way to evaluate the success of a social media campaign to raise immunization awareness in a community is to compare vaccination rates before and after the campaign is launched. Comparably, monitoring hospitalization rates after specific interventions based on prediction models are put into place can help determine whether these steps have been helpful in lowering the burden or severity of the disease over time. Refining predictive models and making sure interventions stay in line with changing dynamics of disease transmission require constant observation and feedback loops.
Using big data analytics on Twitter and other platforms can revolutionize the way we anticipate, stop, and treat infectious diseases. Through the implementation of proactive measures informed by insights obtained from the analysis of social media data, stakeholders from all sectors can work together more successfully to combat the spread of illness on a local and global level. The effectiveness of intervention measures targeted at lessening the burden of infectious diseases on society as a whole will be further improved by the continuous improvement of predictive models through real-world feedback loops.
10. Public Awareness and Education Initiatives
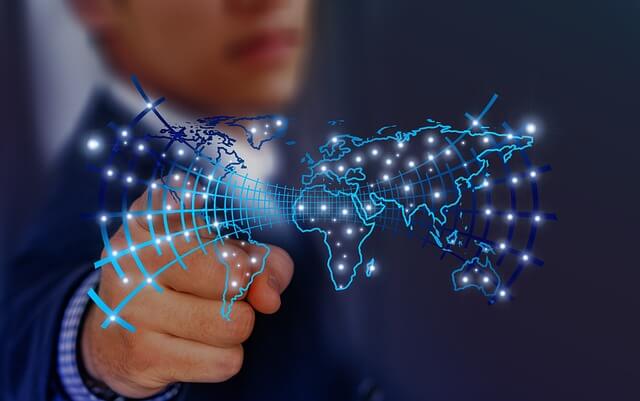
Proactively, one way to raise public knowledge and comprehension of disease surveillance using social media data would be to make educational infographics or short video explainers that show how big data analytics on Twitter and other platforms can forecast the spread of disease. The general people can understand difficult subjects in easily consumable formats thanks to these pictures.
Organizing webinars and workshops, either in-person or virtually, is another smart way to interact with the community. Topics including the value of early detection using social media data, privacy precautions taken while examining public posts, and how individuals might support this group effort could be covered in these sessions.
Establishing clear communication channels is essential to improving transparency and communication on the use of big data analytics for illness prediction. Establishing a specialized website or social media presence with the express purpose of disseminating information about current and planned research, upcoming initiatives, and significant discoveries helps foster public trust.
Surveys and focus groups with community stakeholders might yield important information on misconceptions or worries about the application of big data analytics to disease spread prediction. Open and honest discussion of these issues during scheduled town hall meetings or Q&A sessions can help the initiative become more transparent and accountable.
11. Recommendations for Stakeholders
In order to foresee the spread of diseases on Twitter, stakeholders are advised to work together through government agencies, non-governmental organizations, healthcare providers, and social media platforms. Encouraging communication and information exchange between these sectors may improve the precision and promptness of disease outbreak forecasts. Prioritizing data transparency and access will help stakeholders make sure that important insights are used to their fullest potential.
Stakeholders can help optimize disease prediction models through big data analytics by providing researchers and analysts with pertinent data sets. Stakeholders can facilitate the implementation of more sophisticated predictive modeling approaches by giving them access to complete and up-to-date data from social media platforms like Twitter. Encouraging the public to participate in Twitter discussions about health could produce better data sources for analysis, which would ultimately improve the accuracy of disease spread forecasts.
Through the implementation of these guidelines and the promotion of a cooperative strategy for utilizing big data analytics on Twitter, stakeholders can greatly improve their capacity to anticipate the spread of diseases and effectively address them. This proactive approach not only helps with public health initiatives but also demonstrates how technology and digital platforms can be used to improve global health outcomes.
12. Conclusion: Harnessing the Power of Big Data Analytics on Twitter for Public Health
The aforementioned information leads us to the conclusion that by gathering real-time insights from online interactions, big data analytics on Twitter has demonstrated significant potential in predicting the spread of disease. Researchers and public health officials can obtain useful information to predict outbreaks and carry out prompt interventions by examining trends, emotions, and geospatial data. This strategy provides an efficient way to track population health dynamics in a proactive manner.
In order to improve global health security going forward, it is critical to support additional research and innovation in the use of big data analytics. Constant research into new techniques, software, and instruments can improve the predictive power of using Twitter data analysis for illness surveillance. To fully leverage the potential of big data to protect public health globally, partnerships between data scientists, healthcare practitioners, and technology specialists are essential.
By utilizing big data analytics on Twitter and other platforms, we can increase our preparedness to respond more quickly to new health risks and epidemics. Adopting state-of-the-art techniques and technologies will improve our knowledge of how diseases spread while opening the door to future developments in prevention and response tactics. Let's keep investigating the enormous potential that big data possesses to revolutionize public health procedures for the benefit of global civilization.