1. Introduction
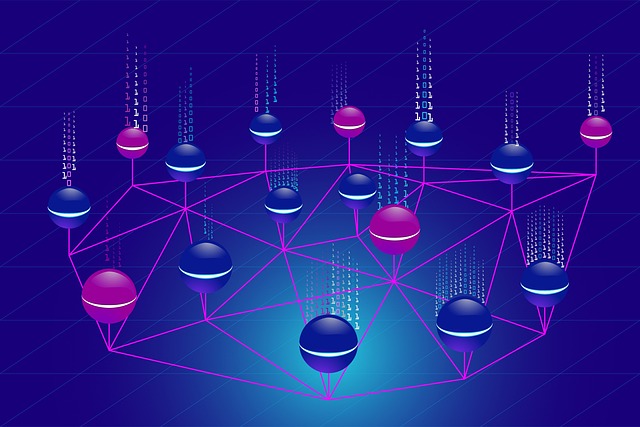
Introduction: Machine Learning (ML) has revolutionized various industries by empowering systems to learn from data and make decisions without explicit programming. In the realm of Customer Relationship Management (CRM), ML plays a pivotal role in enhancing customer interactions, optimizing marketing strategies, and improving overall business performance. By leveraging ML algorithms, businesses can analyze vast amounts of customer data to predict trends, personalize offerings, and streamline processes, ultimately creating a more efficient and tailored CRM experience. This blog post delves into the intuitive ways ML transforms CRM, making it smarter and more effective than ever before.📙
2. Understanding CRM
Systems for managing customer relationships, or CRMs, are crucial tools used by companies to oversee communications and connections with both present and future clients. By organizing and automating many parts of client engagement, these systems assist businesses in increasing sales, streamlining operations, and providing better customer service. Conventional CRM methods usually entail keeping client data, including contact details, purchase history, and preferences, in one single database that sales, marketing, and customer support personnel may readily access. The principal objective is to augment consumer relationships by means of customized communication tactics and data-driven marketing strategies.
To gain a deeper knowledge of CRM systems, keep in mind that these platforms do more than just store customer data; they also let organizations monitor consumer interactions across several channels, including social media, email, phone calls, and website visits. With this comprehensive understanding of the consumer journey, businesses may anticipate future actions and analyze data patterns to provide more individualized experiences. CRM systems can automate processes like as following up with emails, setting up meetings, and sending alerts for potential sales depending on pre-established guidelines established by the company.
The relevance of CRM systems rests in its capacity to concentrate customer data for improved decision-making and strategic planning. With a single, comprehensive view of every customer's preferences and behavior patterns, businesses can more successfully target their marketing campaigns, spot upselling possibilities, predict sales trends, and ultimately forge better bonds with their clientele. To stay ahead of the curve in today's competitive market scenario, where individualized experiences foster customer loyalty, organizations must fully utilize the potential of CRM systems.
3. The Role of Machine Learning in CRM
Because machine learning offers insightful data and predictive analytics, it is essential for improving Customer Relationship Management (CRM) strategies. Machine learning algorithms can find patterns and trends in massive amounts of data from diverse sources that human analysts might miss. By using these data, organizations may better understand the requirements, tastes, and behaviors of their customers and adjust their marketing tactics and customer interactions to increase engagement and conversion rates.
CRM systems may now make judgments in real time based on dynamic shifts in consumer behavior thanks to machine learning. Predictive lead scoring techniques, for instance, use past data to identify leads that have the highest likelihood of becoming clients, enabling sales teams to efficiently allocate their resources. Sentiment analysis tools assess consumer input from various sources using natural language processing algorithms. The information they provide can be used to improve products or services and quickly resolve customer issues.
Considering everything mentioned above, we can say that integrating machine learning into CRM systems transforms the way companies engage with their clientele by facilitating mass customization and promoting data-driven decision-making. In today's competitive market context, businesses can improve operational efficiency, foster stronger customer relationships, and eventually achieve sustainable growth by utilizing machine learning (ML) tools such as sentiment analysis and predictive analytics.
4. Benefits of Integrating ML into CRM
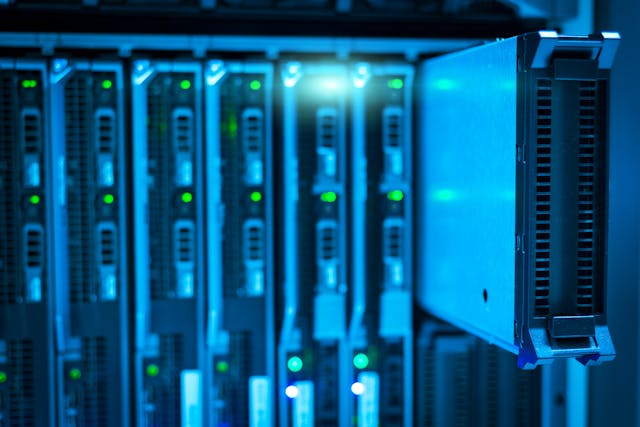
Numerous advantages arise from integrating machine learning (ML) with customer relationship management (CRM) systems, which have the potential to completely transform how companies engage with their clientele. Businesses can obtain deeper insights into their customers and improve their understanding of preferences, behaviors, and trends by utilizing machine learning (ML) algorithms. Higher engagement and conversion rates follow from more individualized marketing strategies made for specific consumers.
Businesses may anticipate demands and offer proactive support by integrating machine learning (ML) into CRM, which improves the overall customer experience. Real-time analysis of large volumes of data allows machine learning algorithms to anticipate patterns in consumer behavior and tailor automated replies. This proactive strategy encourages enduring loyalty in addition to raising consumer happiness.
Through automation and predictive analytics, machine learning (ML) enables businesses to maximize resource usage and streamline processes. Automating repetitive processes allows staff to focus on high-value activities that foster growth and innovation. Examples of these duties include data input, lead scoring, and sales forecasting. A calculated investment that helps companies stay ahead of the curve in the cutthroat market of today is the integration of ML into CRM systems.
5. Implementing ML in CRM
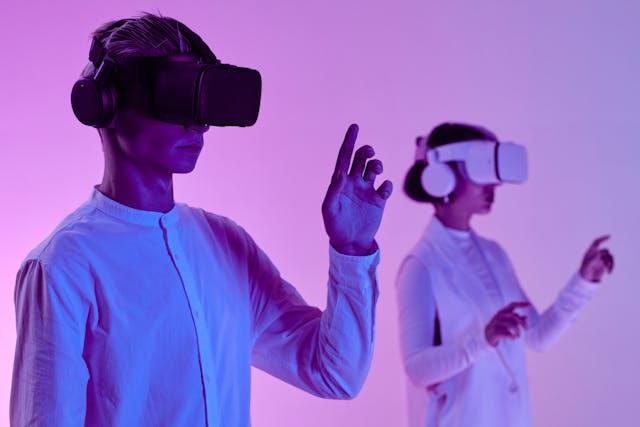
Your customer relationship management (CRM) system can function much more effectively and efficiently if machine learning (ML) is integrated into it. Take the following actions to properly incorporate ML models into current CRM systems:
1. **Data Preparation**: Start by ensuring your data is clean, organized, and relevant. Data quality is crucial for the success of any ML model.
2. **Model Selection**: Based on the type of data you have and the business issues you want to resolve, pick the right machine learning models. Regression, classification, clustering, and recommendation models are popular options.
3. **Training Models**: Train your chosen ML models using historical CRM data. This step involves feeding labeled data into the algorithm to enable it to learn patterns and make predictions.⌨️
4. **Integration with CRM**: Integrate the trained ML models into your CRM system. Ensure seamless communication between the ML algorithms and CRM databases for real-time decision-making.
5. **Validation and Testing**: Make sure the integrated ML models function correctly in real-world situations by subjecting them to extensive testing. To gauge progress, compare their findings with current CRM procedures.
6. **Continuous Learning**: Implement mechanisms for continuous learning, where ML models can adapt and improve over time with new data inputs from CRM interactions.
You can use machine learning to make your CRM system a more intelligent, effective tool that improves customer connections and company outcomes by carefully following these steps.
6. Case Studies
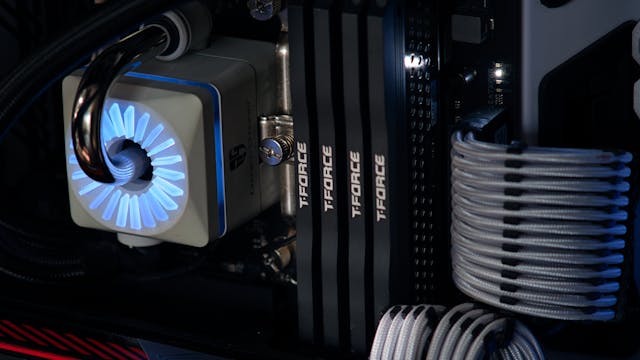
Machine learning is proving to be a game-changer in the field of customer relationship management (CRM), completely changing how organizations interact and manage their clientele. Businesses may now delve deeper into client data and obtain important insights that were previously elusive by utilizing ML algorithms.
Case studies provide an insight into the real-world effects of ML integration with CRM systems. One such instance is a retail business that effectively predicted customer preferences using predictive analytics. Sales and customer satisfaction significantly increased as a result of the company's ability to personalize recommendations through analysis of shopping trends and behaviors.
An additional interesting case study comes from a telecom company that predicted customer attrition rates using machine learning algorithms. They were able to take proactive measures with retention techniques by identifying key indicators that indicated clients were likely to leave through advanced data analysis. They were able to significantly lower turnover rates by 15% as a result, which increased revenue and improved client loyalty.
These real-world instances demonstrate the revolutionary potential of machine learning in CRM software, demonstrating its capacity to propel corporate expansion and cultivate enduring client connections. Businesses may open up new avenues for more in-depth customer awareness and impactful strategy customization by adopting machine learning (ML) technologies.
7. Overcoming Challenges
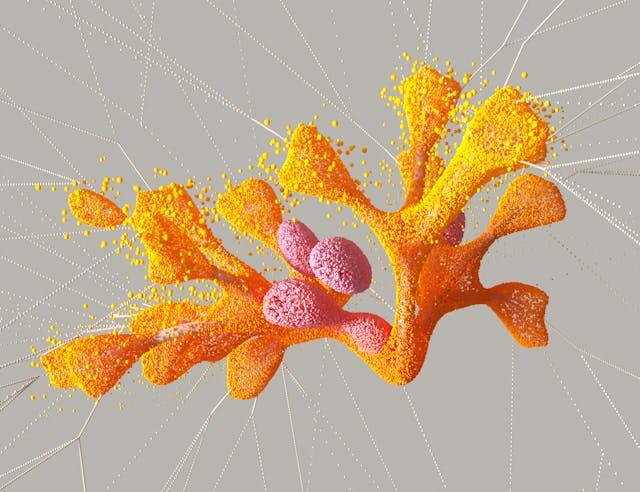
Businesses frequently run into problems with data quality and model interpretability when integrating machine learning into CRM systems. Inaccurate insights and forecasts can result from missing or inconsistent data, which can cause data quality issues. To properly handle these problems, it is imperative to set up strong data governance procedures and make investments in data cleansing methods.
Another typical challenge when implementing machine learning in CRM is model interpretability. Building trust and making defensible decisions based on predictions requires an understanding of how a model makes its predictions. Techniques such as feature importance analysis, SHAP values, and model-agnostic methods like LIME can assist explain the inner workings of sophisticated machine learning models.
Businesses may successfully leverage the power of AI-driven CRM solutions by overcoming these obstacles with proactive data management strategies and interpretable machine learning models and approaches. This guarantees precise insights, better decision-making procedures, and superior client experiences—all of which promote the expansion and prosperity of businesses.
8. Future Trends in ML-Driven CRM
Significant progress in ML-driven CRM is anticipated in the future. The incorporation of augmented analytics, which provides deeper insights and predictive capabilities, into CRM systems is one important trend to keep an eye on. Natural language processing (NLP) advances will make it possible for chatbots and voice assistants to provide more natural-feeling customer interactions.
The advent of Explainable AI (XAI) in CRM systems is another noteworthy advancement. This technology offers transparency into the decision-making process of AI models, which is essential for upholding consumer trust. Marketing strategies based on real-time feedback can be optimized and personalized recommendations improved with the use of reinforcement learning algorithms.💡
We may anticipate a rise in the use of big data analytics and machine learning in CRM as long as data remains a key component of company success. Large volumes of consumer data can now be meaningfully analyzed by businesses, resulting in better customer interaction and more focused marketing strategies. All things considered, ML-driven CRM has a promising future that will be marked by increased efficiency, satisfaction from customers, and customisation.
9. Ethical Considerations
The use of artificial intelligence (AI) and machine learning (ML) technology in customer relationship management (CRM) has completely changed how companies interact with their clientele. But when businesses use these technologies more extensively to improve customer relations and streamline data processing, it becomes critical to address the ethical issues raised by these developments.
Transparency is a crucial ethical factor when utilizing AI and ML in CRM systems. When using AI to communicate with consumers or make decisions that could affect their experience, businesses need to make sure that customers are informed. Transparency fosters a sense of control over consumers' information by establishing confidence and enabling them to understand how their data is being used.
Data privacy is another important ethical consideration. Because AI and ML algorithms depend so heavily on massive volumes of customer data to function properly, there is a serious risk that privacy laws will be broken if this data is not managed securely. Protecting client data, adhering to laws like GDPR, and implementing strong security measures are top priorities for businesses looking to keep sensitive data protected from abuse or unauthorised access.
In CRM applications, bias in AI algorithms presents a significant ethical conundrum. Biased training data can result in biased practices in customer encounters when these algorithms are developed. In order to maintain equity and avoid discrimination on the basis of socioeconomic class, gender, or race, businesses must actively detect and address biases in their AI systems.
Additionally, as I mentioned above, there are significant ethical issues that are raised by the use of AI and ML in CRM systems, even if these technologies have a lot to offer organizations and consumers alike. Organizations can navigate the ethical complexities associated with advanced technologies intelligently and ethically by putting a priority on transparency, protecting data privacy, and addressing algorithmic bias. This will ensure a more responsible approach to developing smarter CRM solutions for long-term growth and positive customer relationships.
10. Training and Development
Upskilling teams to successfully use machine learning capabilities in CRM operations requires training and development. First and foremost, businesses ought to give top priority to offering thorough training courses that concentrate on the foundations of machine learning and how it applies to customer relationship management. Workshops, online classes, or hiring outside specialists for specific training sessions are a few examples of this.
Fostering a culture of continual learning within the organization is crucial. Encouraging staff members to stay up to date on machine learning tools and trends guarantees that they can use these technologies to improve CRM efforts. Additionally, businesses might set up mentorship programs wherein seasoned data scientists can assist team members in using machine learning methods with CRM data.
Gaining practical experience is crucial for understanding the principles of machine learning. Opportunities to work on actual projects where team members may put their expertise to use in a real-world context should be provided. Developing a culture of support that allows staff members to freely test out new concepts and models is essential to fostering innovation in CRM through machine learning.
To sum up everything I've written so far, investing in team development and training is essential to maximizing the application of machine learning in CRM operations. Organizations can stay ahead of the curve in this quickly changing technological landscape by providing employees with the resources and skills they need.
11. Tools and Resources
To optimize CRM strategies with machine learning approaches, a strong toolkit and resources are needed. Fortunately, companies wishing to use ML for more intelligent CRM procedures have a plethora of solutions at their disposal. TensorFlow, scikit-learn, and Amazon SageMaker are a few well-liked tools. These systems have strong capabilities for creating and implementing ML models that are suited to CRM requirements.
CRM platforms such as Salesforce, HubSpot, and Microsoft Dynamics offer integrated capabilities that utilize machine learning algorithms for better customer insights and predictive analytics, in addition to solutions specially created for this purpose. The process of integrating ML into current CRM operations can be streamlined by taking advantage of these built-in features.
Professionals can also rely on online resources like blogs, whitepapers, webinars, and online courses provided by top tech companies and academic institutions to stay up to date with the newest trends and developments in machine learning for customer relationship management. Communities such as Kaggle and GitHub offer a forum for cooperation and exchange of machine learning solutions customized for CRM problems, thus expanding the toolkit and expertise available to professionals. By interacting with these tools, companies may continuously improve the way they apply machine learning to their customer relationship management strategy.
12. Conclusion
To put it succinctly, incorporating machine learning into CRM procedures has the potential to completely transform the way companies engage with their clientele. Businesses are able to accelerate corporate growth, optimize operations, and personalize consumer experiences by utilizing predictive insights and data analysis. Organizations may improve sales and marketing tactics, anticipate consumer demands, and build stronger client relationships with machine learning's transformative potential. Using this cutting-edge technology opens the door to more intelligent CRM solutions that provide better customer satisfaction and can adjust to shifting market conditions. It's clear that using machine intelligence to make customer interactions more effective, tailored, and engaging across all touchpoints is where CRM is headed.