1. Introduction
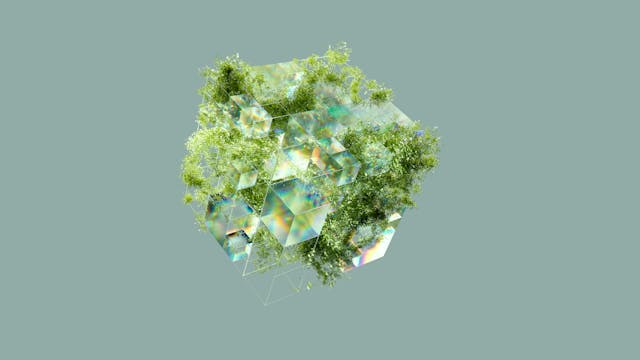
**Introduction:** In the realm of data analysis, a longstanding debate has revolved around the importance of data quantity versus data quality. Some argue that having more data is always better, while others stress the criticality of ensuring high-quality, accurate data. However, the dichotomy between data quantity and data quality is not as stark as it may seem. This article delves into how these two aspects can complement each other rather than compete, shedding light on the ways in which organizations can leverage both to drive valuable insights.
**Thesis Statement:**
The purpose of this essay is to demonstrate how, in the field of data analysis, quantity and quality of data are not mutually exclusive but rather work in harmony. Through an analysis of the ways in which organizations can balance the acquisition of vast amounts of data with the preservation of its correctness and pertinence, we discover a sophisticated methodology that optimizes the possibility of extracting practical knowledge from extensive datasets.
2. Importance of Data Quantity
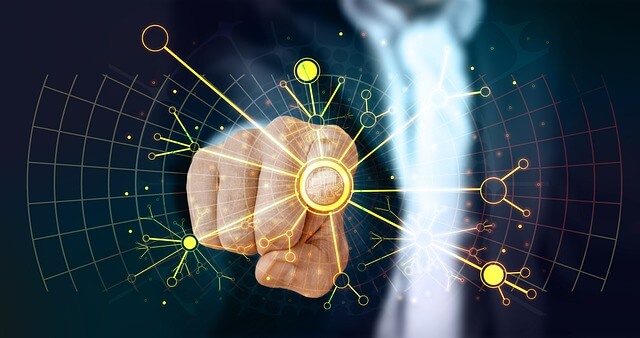
In many different businesses, the amount of data is crucial to improving insights and decision-making processes. Organizations can have a deeper understanding of market trends, consumer behavior, and other critical business elements by utilizing a larger volume of data. Businesses that have access to larger datasets are better able to spot patterns, trends, and correlations that could have gone missed in smaller datasets. As a result, their activities are better understood, and more intelligent strategic planning is possible.
The significance of data quantity in the big data era cannot be emphasized. Large volumes of consumer data are used by sectors like e-commerce to enhance overall customer experiences, optimize pricing methods, and personalize recommendations. Online retailers, for example, customize product offerings and promotions to individual preferences based on browsing history, purchasing histories, and demographic data; this leads to increased consumer satisfaction and conversion rates.
In a similar vein, healthcare providers use big datasets from wearable technology, medical imaging systems, genomics research, and electronic health records to enhance patient outcomes. Healthcare practitioners can foresee possible health hazards, spot disease trends, tailor treatment regimens, and improve the efficiency of healthcare delivery overall by analyzing vast amounts of patient data.
Big data analytics is used in the financial services industry for a variety of purposes, including customer segmentation, algorithmic trading methods, risk assessment, and fraud detection. In order to quickly identify suspicious activity and stop fraudulent transactions, banks analyze transactional data in real time. Big data algorithms are used by investment firms to spot market trends early and make data-driven investment decisions that improve portfolio performance.
Predictive maintenance techniques are used in industries such as manufacturing, where sensor data from machines is used to predict equipment faults before they happen. This proactive strategy maximizes production efficiency, lowers maintenance costs, and reduces downtime. Manufacturers can maintain continuous operations and improve overall equipment effectiveness (OEE) by combining real-time sensor readings from factory floor equipment with massive volumes of past operational data. π
Summarizing the above, we can conclude thatπ
data quantity empowers organizations across diverse sectors to uncover valuable insights,
optimize processes,
and drive innovation.
By effectively harnessing the vast amounts of available data,π
companies can stay ahead in today's competitive landscape
and unlock new opportunities for growth
and transformation.πΈ
Ultimately,
combining both ample quantities of relevant data
with high-quality standards
is key to achieving success
in this age of information abundance,
wherein every byte counts towards making meaningful business decisions that pave the way for future success.
3. Significance of Data Quality
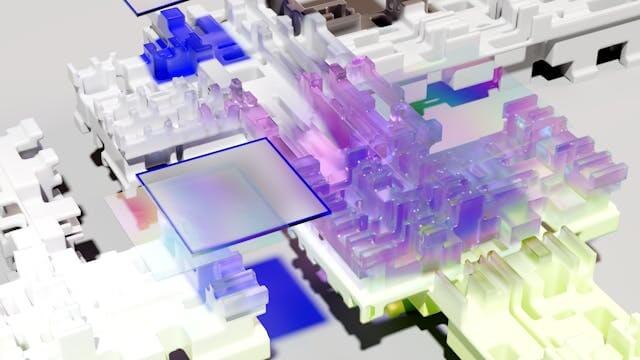
Any process that uses data to drive decision-making must prioritize data quality. Decisions made based on insufficient or inaccurate facts can have a big impact on how things turn out. Data is used by decision-makers to offer information and direction. Decisions based on inaccurate data could lead to inefficiencies, lost opportunities, or even monetary losses. For a company to succeed, data accuracy and completeness must be guaranteed.
Decision-making procedures benefit from the use of relevant and reliable data since it offers dependable information that is in line with the goals at hand. Decisions made using trustworthy data are guaranteed to be founded on facts rather than conjecture or speculation. Relevant information is more applicable and helpful because it directly relates to the particular context of the decision. Organizations can boost their trust in decision outcomes and achieve better results by placing a higher priority on data quality.
Maintaining high-quality data improves overall operational effectiveness and efficiency in addition to improving decision-making. Because of the dependability and applicability of their information assets, organizations that place a high priority on data quality typically see improvements in performance across a range of functions. This dedication to quality guarantees that choices are educated and in line with company objectives, which improves results over time.π
4. Challenges in Balancing Quantity and Quality
In today's data-driven society, striking a balance between quantity and quality of data poses issues. The trade-off problem between accuracy and volume is one of the main obstacles. Businesses are frequently under pressure to obtain a lot of data fast, but this has the potential to erode the data's dependability and accuracy. Achieving a balance between obtaining copious amounts of data and guaranteeing its precision is crucial for arriving at well-informed conclusions.
Handling soiled data and inherent biases inside datasets presents a considerable additional barrier. Inaccuracies, inconsistencies, or duplicates in data might lower the overall quality of the available information. Inadvertent biases in datasets can distort analysis and conclusions. Maintaining both quantity and quality in data gathering systems requires early recognition of these problems and the application of techniques to eliminate and mitigate biases.
Navigating these obstacles successfully calls for a thorough strategy that places equal emphasis on quantity and quality. Organizations can leverage data power without compromising its integrity by using strong data gathering techniques, upholding stringent data hygiene procedures, and conducting frequent audits to identify biases. In today's increasingly complicated digital ecosystem, realizing that data quantity and quality are not mutually incompatible creates potential for more precise insights and well-informed decision-making.
5. Strategies for Improving Data Quality Without Sacrificing Quantity
To improve data quality without sacrificing quantity, data validation procedures must be put in place. Organizations can guarantee that the data obtained satisfies predetermined standards and is error- and consistency-free by putting in place rigorous validation procedures. To quickly identify problems, this may entail implementing automated checks at different phases of data entry. Maintaining high-quality data while gathering vast amounts of it can be facilitated by establishing explicit criteria for data formatting and carrying out frequent audits.
Artificial Intelligence (AI) is one of the cutting edge technologies that may greatly enhance error detection without compromising the volume of data being handled. Large volumes of data can be quickly analyzed by AI-powered systems, which can spot anomalies or discrepancies that a manual examination could miss. Organizations can improve data quality efficiency and expedite mistake detection procedures by utilizing machine learning techniques. By using AI-driven solutions, dataset accuracy is increased and scalability is made possible for efficiently managing massive amounts of data.
To summarise, there are effective ways to improve data quality without compromising quantity. These ways include using sophisticated AI technology and implementing strong data validation processes. Through a commitment to upholding dependable and precise datasets and effectively managing substantial volumes of data, establishments can fully utilize their data assets to facilitate well-informed decision-making and attain commercial triumph.
6. Techniques for Maximizing Data Quantity While Maintaining Quality
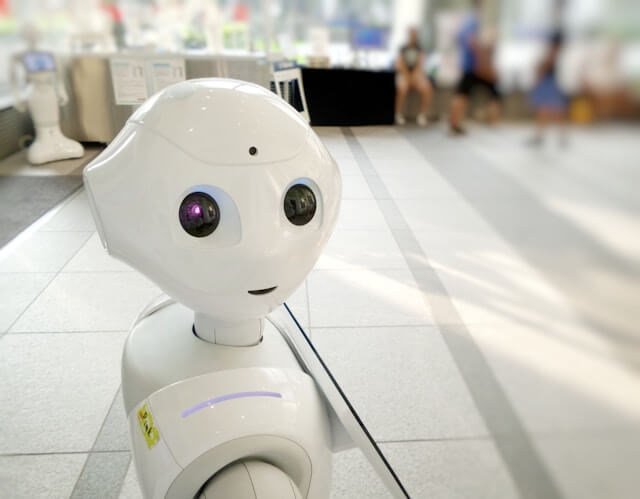
Finding the right balance between quantity and quality is essential for producing insightful findings in the field of data science. Data augmentation strategies are a useful tool for practitioners looking to maximize data quantity without compromising quality. Through techniques such as noise addition, duplication, and transformation, researchers can grow their datasets without sacrificing accuracy or dependability.
Maintaining data integrity is largely dependent on effective metadata management. Maintaining accurate records of the data's source, processing steps, and transformations helps guarantee its correctness and traceability. Good metadata management promotes reproducibility and confidence in the analysis's conclusions in addition to increasing transparency.
Organizations can enhance their datasets while maintaining their quality standards by employing data augmentation tactics and rigorous metadata policies. This balanced combination of quantity and quality enhances the overall effectiveness of analytical efforts and supports data-driven decision-making procedures.
7. Real-world Examples of Successful Integration of Data Quantity and Quality
The beneficial relationship between data quantity and quality for companies is illustrated by real-world instances. Netflix is a noteworthy example of how these two elements are successfully combined to improve user experience. Netflix creates suggestions that are relevant and accurate while analyzing large amounts of viewing data to keep viewers interested. Its success has been greatly attributed to this well-rounded strategy.
Amazon is another excellent illustration; it was a leader in the use of big data. Amazon's advanced algorithms optimize product recommendations and customized marketing tactics by taking into account a vast amount of user data as well as precise product details. Amazon is now able to provide a flawless purchasing experience by utilizing both the accuracy and depth of data thanks to this integration.
These examples highlight crucial best practices include making an investment in cutting-edge analytics technologies, establishing a culture that prioritizes both quantity and quality, and preserving data integrity through reliable procedures. By emphasizing a comprehensive approach to data management, organizations may learn a lot from these successful integrations and ultimately drive innovation and competitiveness in today's data-driven market.
8. Future Trends in Data Management
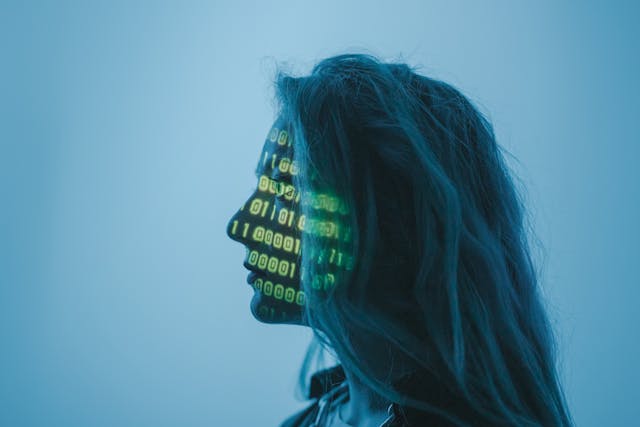
a. New technologies are changing the big data analytics scene by fostering efficiency and creativity in the processing of enormous volumes of data. The methods used for gathering, processing, and storing data are being completely transformed by technologies like blockchain, edge computing, and quantum computing. By processing data at or close to the point of data generation, edge computing lowers latency and accelerates insights. With the capacity to solve complicated problems tenfold faster than conventional computers, quantum computing opens up new avenues for the analysis of large datasets. Blockchain technology generates tamper-proof records of data exchanges, ensuring safe and transparent transactions.β¨οΈ
b. In the digital age, optimizing data quantity and quality requires the interaction of artificial intelligence (AI), machine learning (ML), and automation. AI algorithms are capable of efficiently extracting insights from vast amounts of unstructured data sets by analyzing big datasets significantly more rapidly and precisely than any person has ever been able to. ML models have the capacity to continuously learn from fresh data inputs, enhancing their predictive power over time. Automation tools increase operational efficiencies and decrease manual error in data management procedures. When combined, these technologies increase the amount of data that is available while simultaneously improving its quality by using cutting-edge analysis methods.
In conclusion, the landscape of big data analytics is being significantly shaped by emerging technologies like edge computing, quantum computing, blockchain, artificial intelligence (AI), machine learning (ML), and automation. This is because these technologies will become more and more prevalent as we delve into the future trends of data management. We may fully utilize quantitative measurements through higher volume and qualitative metrics through enhanced correctness and relevance of our datasets by adopting these advances sensibly and carefully incorporating them into our data strategy. In the years to come, these developments will surely propel exceptional progress throughout sectors worldwide by facilitating the synergy between data quantity and quality.
9.Conclusion
To sum up what I've written thus far, it is critical to realize that in the field of data analytics, data quantity and quality are not mutually incompatible. Both factors work in tandem to help gather insightful information and facilitate thoughtful decision-making. Organizations that possess vast amounts of data may be able to identify patterns and trends that would not be visible in smaller datasets. The conclusions drawn, although, could be false or deceptive if the correct quality steps aren't taken to guarantee the accuracy, relevance, and dependability of this data.
In order to properly capitalize on the synergy between data quantity and quality, a comprehensive approach is necessary. This entails putting in place strict quality control procedures in addition to dependable data collection techniques to guarantee an adequate amount of data. Organizations can improve the breadth and precision of their analysis, resulting in more trustworthy insights and strategic decision-making, by adopting both elements at the same time.
Businesses can realize the full value of their data assets and obtain a competitive advantage in today's data-driven environment by realizing how interrelated data quantity and quality are. It is not a matter of favoring one above the other; rather, it is about realizing their mutually beneficial relationship and using it to propel success and creativity.