1. Introduction:
Data science is essential in today's data-driven society for drawing insightful conclusions from massive volumes of data. But in the midst of all the analytics and algorithms, something seems to be lacking: human interaction. Here's when Mr. Holmes enters the picture. To reveal the hidden stories within data sets, we can employ a similar strategy to solve mysteries, just as Sherlock Holmes did with his keen observational abilities and intuition. Through the integration of an investigative mindset into data science methodologies, it becomes possible to reveal more profound meanings and important discoveries that algorithms by themselves might miss. Let's continue exploring "The Missing 'D' in Data Science" while acknowledging Mr. Holmes's astute observation and analysis skills.
2. Understanding Data Science:
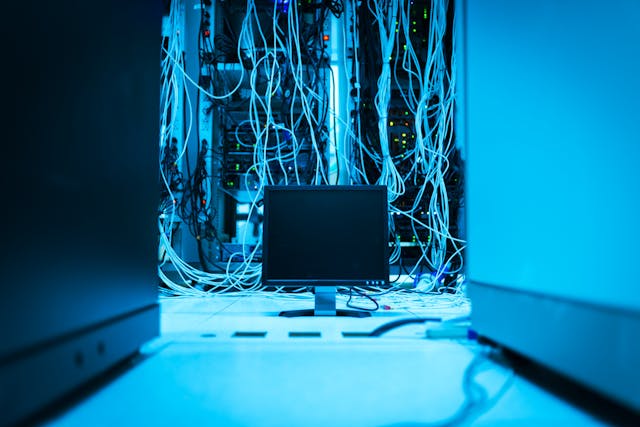
The area of data science is concerned with deriving knowledge and insights from data by combining computer science, statistics, and domain experience. It is essential to many different industries because it enables businesses to make well-informed decisions through data-driven research. The power of data science resides in its capacity to reveal correlations, patterns, and trends that would otherwise go undetected in massive databases.
Applications of data science are many and include personalized suggestions on streaming platforms and predictive analytics in finance. Data scientists are capable of forecasting future trends, comprehending consumer behavior, streamlining procedures, and much more through the use of complex algorithms and machine learning techniques. By using data to drive strategic efforts, this gives organizations a competitive advantage.
In any organization, data provides the basis for problem-solving and decision-making. Through the examination of both past and current data, decision-makers are able to evaluate risks, recognize opportunities, and formulate efficacious plans. Making decisions based on data reduces ambiguity and makes evidence-based decisions more likely to result in positive outcomes. In today's complicated and changing economy, data essentially enables organizations to manage obstacles with certainty and precision.
In the field of data science, Mr. Holmes is the archetypal investigator who, using his astute observational abilities and deductive reasoning, solves riddles concealed within enormous databases. Similar to how Sherlock Holmes deciphers clues to solve crimes, data scientists employ cutting-edge analytical methods to find important insights that spur innovation and industry growth. Adopting a curious and exploratory mindset like to that of Mr. Holmes has the potential to revolutionize our understanding of data science, transforming it from a technical undertaking to an enthralling adventure rife with possibilities.
3. The Missing "D":
The "D" that frequently disappears has more meaning in the field of data science than just being an alphabetical omission. This omitted "D" stands for the deduction component, which is the essential connection between unprocessed data and insightful conclusions. Like a detective piecing together hints to solve a crime, data scientists comb through data to find patterns and trends that help them make wise decisions.💭
There are a lot of similarities between data analysis and detective work. In order to create a coherent story, both professions need assembling evidence, closely examining details, and assembling disparate pieces of information. Essentially, they have the same goal in mind: using reason and logic to make sense of ambiguity and complexity.
Similar to how Sherlock Holmes uses his astute observational abilities and logical reasoning to solve crimes, data scientists use statistical models and algorithms to decipher datasets and extract important information from massive amounts of data. We may comprehend the missing "D"'s importance as the link between data gathering and useful insights by realizing that it is the fundamental component of inference and interpretation in data science.
Data scientists can transcend computing into the art of deduction by adopting the attitude of a sleuth skilled at discovering connections between dots that others might miss. Adopting this comprehensive strategy improves one's capacity for problem-solving while also promoting a deeper comprehension of intricate systems and phenomena that exist behind the surface levels of data sets.
4. Mr. Holmes: The Detective Analogy:
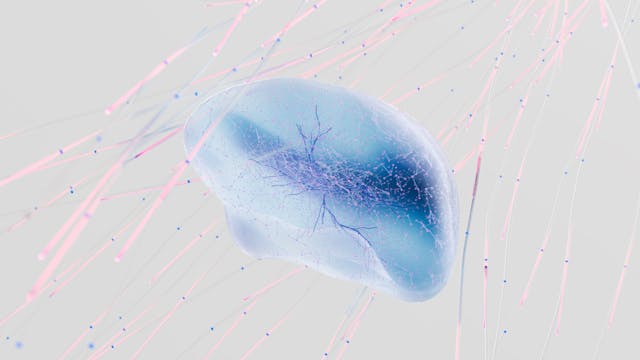
Sherlock Holmes is a shining example of investigative skill and deductive reasoning in the field of data science. Similar to the well-known investigator, data scientists must have an acute sense of detail, the capacity to make connections between seemingly unrelated bits of information, and an unwavering quest for the truth concealed in the data they examine.
The methodical methodology taken by Sherlock Holmes to unravel riddles can be compared to the fundamental ideas of efficient data analysis. The principles of hypothesis testing, pattern identification, and predictive modeling in data science are strongly aligned with his emphasis on observation, logical reasoning, and inference. Data scientists can gain important insights that help them make well-informed decisions by taking Holmes' lead and carefully weighing all of the available evidence before using it to make meaningful conclusions.
To put it simply, adopting the mindset of Mr. Holmes in the field of data science is viewing each dataset as an intriguing mystery that needs to be solved. Data scientists can improve their analytical skills and solve complicated problems with accuracy and clarity by utilizing deductive reasoning and investigative techniques reminiscent of the great detective himself.
5. Uncovering Clues in Data Analysis:
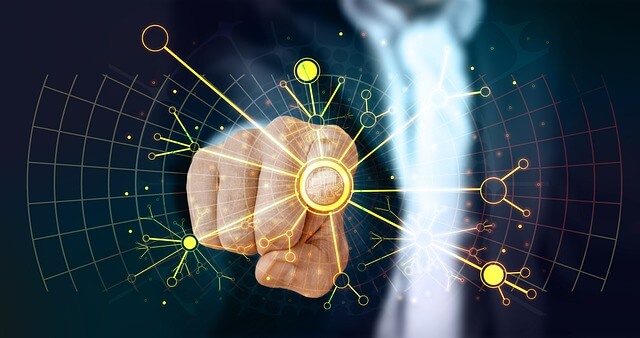
In the field of data analytics, taking cues from the renowned investigator Sherlock Holmes might yield insightful solutions to challenging issues. Similar to how Sherlock Holmes methodically solves puzzles, data scientists can use similar techniques to analyze data and come to insightful conclusions. The importance of being meticulous in every facet of an inquiry is one of the most important lessons Sherlock Holmes gives. Similar to this, when it comes to data analysis, ignoring even the slightest details might result in incorrect interpretations and lost chances for insightful discoveries.
Sherlock Holmes is a famed character in detective fiction because of his acute observational skills and critical thinking. Using a similar perspective when conducting their study might also be beneficial for data scientists. Through in-depth examination of datasets, challenging presumptions, and deciphering data like hints in a case, analysts can find hidden patterns and correlations that might not be immediately obvious. This rigorous process is necessary to extract precise results and make defensible conclusions based on evidence derived from data.
Data scientists can use logic and reasoning to make connections within their datasets, just as Sherlock Holmes uses deductive reasoning to connect seemingly unrelated pieces of information. Through the use of logical frameworks and a methodical investigative methodology, analysts may more efficiently sift through large volumes of data and produce insights that are actionable. Cultivating these Sherlockian traits can help professionals stand out in the fast-paced field of data science by empowering them to tackle intricate analytical problems with clarity and precision.
In summary, the "D" that is absent from data science, which stands for deduction, emphasizes the significance of applying Sherlock Holmes' techniques to data analysis in a methodical manner. Data scientists can uncover deeper levels of significance inside datasets and make more significant business decisions by adopting Holmes' attention to detail, critical thinking abilities, and deductive reasoning methodologies. As Sherlock Holmes deciphers puzzles with systematic accuracy, data scientists can also unravel intricate issues by adopting a detective's attitude in their analytical pursuits.😠
6. Solving the Case: Improving Data Practices:
The significance of thorough data cleaning and preparation must be emphasized in order to enhance data analysis methods. Analysts can produce more dependable insights by guaranteeing the relevance and accuracy of the data. The quality and depth of analytical results can be improved by utilizing machine learning algorithms and sophisticated statistical methodologies. Improving overall data practices also requires interdisciplinary teams to collaborate in order to get a variety of viewpoints and specialized knowledge in managing large, complicated data sets.
Adding aspects of detective work to data science techniques can offer a new way of looking at problems. Data scientists can find patterns and connections inside datasets by following a methodical strategy, much like detectives do when they're collecting clues and connecting the dots to solve a case. Stakeholders can comprehend complex information more easily by using visualization tools to create interactive dashboards that present a story based on data discoveries. Unlocking important insights from data requires adopting a curious attitude and asking the right questions in a manner similar to a detective's investigation procedure.
7. The Art of Interpretation:
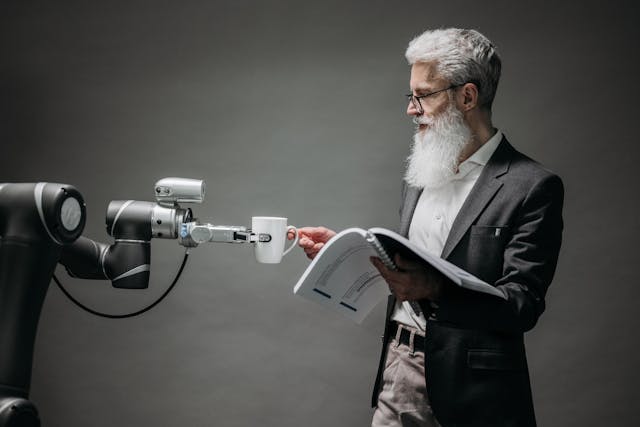
Making sense of complex data sets is a critical function of the art of interpretation in the field of data science. Data scientists frequently have difficulties in recognizing patterns, trends, and linkages in the data as they sift through an abundance of information. Gaining an acute observational and inferential sense is crucial to discovering the insights concealed in the data.
The existence of noise or unimportant information that can obfuscate important patterns is a major problem when evaluating large, complicated data sets. Data scientists must improve their capacity to sift through this clutter and concentrate on finding pertinent signals that lead to useful discoveries. Data analysts who hone their observational abilities are able to identify patterns that are not immediately obvious.
Using statistical methods to confirm ideas, actively searching for patterns across various variables, and visualizing data through graphs and charts can all help create a keen sense of observation. When data scientists approach data analysis with a Sherlock Holmes-like curiosity and critical perspective, they can find important insights that might have gone overlooked otherwise.
8. Avoiding Common Pitfalls:
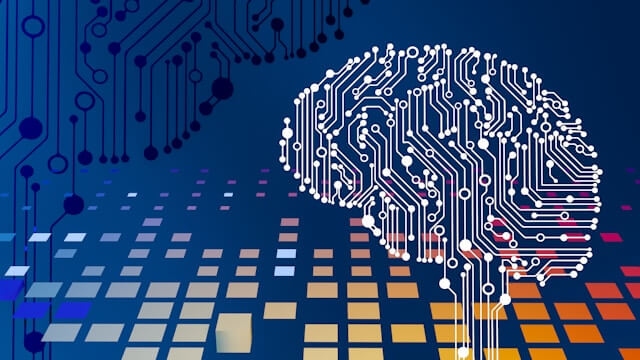
Accurate results in data analysis depend heavily on avoiding frequent errors. Confirmation bias is a frequent error in which researchers inadvertently give more weight to data that supports their assumptions. One can use Sherlock Holmes' strategy of impartially weighing all options to prevent this. Overfitting is another common mistake made by models that fit training data too closely and don't perform well when applied to fresh data. Holmes' emphasis on obtaining all pertinent information can motivate analysts to employ a variety of datasets to create strong models.
Neglecting outliers or anomalies might cause distorted outcomes in data analysis. The meticulous nature of Sherlock Holmes serves as a helpful reminder to thoroughly examine odd data points that may offer insightful information or point out mistakes in the analytical process. Time and resources may be lost if the problem statement is not well defined before analysis begins. Determining specific goals and hypotheses up front directs the analytical process toward more targeted and significant results, just like Holmes does with his case summaries.
Data scientists frequently make the mistake of depending only on automated technologies and failing to critically examine or comprehend their outputs. To appropriately confirm algorithmic conclusions, analysts must apply critical thinking and manual checks, taking a cue from Sherlock Holmes' meticulous methodology. Decision-making procedures based on data insights may be hampered by a failure to effectively communicate results. Analysts may make sure that their findings are useful and that stakeholders understand them by following Holmes' methodical and precise approach to presenting conclusions and supporting data.
Ignoring ethical issues in the gathering and processing of data is a big mistake with potentially dire repercussions. Data scientists should put privacy, fairness, and transparency first at every stage of the analysis process, much as Sherlock Holmes did in his investigations. Analysts can reduce the dangers of biased algorithms or sensitive information misuse while building confidence among users and communities in data-driven projects by implementing ethical principles into their work. 🫥
After reviewing the material above, we can draw the important conclusion that studying Sherlock Holmes' research techniques can help data scientists steer clear of typical mistakes. Data analysts can improve their analytical accuracy and provide more dependable and significant results in an increasingly complicated digital environment by adopting a mentality that is similar to Mr. Holmes's: objectivity, thoroughness, clarity, critical thinking, and ethical responsibility.
9. Harnessing Technology for Data Insights:
Using technology effectively is essential in the field of data science to extract valuable insights from massive amounts of data. Technological tools are essential for improving data visualization and interpretation, which helps consumers comprehend and access complicated datasets more easily. Analysts can produce dynamic, lifelike data visualizations by using programs like Tableau, Power BI, or Python libraries like Matplotlib and Seaborn. This will help stakeholders spot patterns, trends, and outliers more quickly.
It is crucial to remember the role that human intuition plays in the data analysis process even as technology breakthroughs continue to transform the area of data science. Deeper understanding of complicated datasets and more precise forecasts can result from the interaction of human intuition and machine learning algorithms. Human analysts provide a distinct viewpoint that robots cannot duplicate. Their topic expertise, critical thinking abilities, and contextual awareness are crucial for correctly evaluating data.
Organizations may fully utilize their data assets by fusing human creativity and intuition with the analytical powers of robots. With the help of this hybrid technique, data outputs can be interpreted more nuancedly, improving strategic planning and decision-making. Using both technology tools and human knowledge will be essential as we advance in the big data era to provide meaningful insights that spur innovation and success in the data science sector.
10. Ethical Considerations in Data Science:
Ethical considerations are vital in data science because they guarantee the responsible handling of sensitive data. When working with data that could have an impact on specific people or society as a whole, data scientists frequently encounter moral conundrums. The moral code and acute sense of ethics associated with Sherlock Holmes can serve as an inspiration for data science ethical frameworks.
Finding a balance between protecting people's privacy and using data for study is a recurrent ethical conundrum in the field of data science. Data scientists have to walk a tightrope in order to handle sensitive data in an appropriate and moral manner. By taking inspiration from Sherlock Holmes's regard for privacy and personal space, data scientists might apply comparable values to their job.
In data science, accountability and transparency are crucial components of ethics. When working with data that potentially have important ramifications, data scientists should be open and honest about their procedures, presumptions, and possible biases. Data scientists might use Sherlock Holmes' dedication to justice and truth as a model to maintain accountability and openness in their work.
Data scientists might aim to make more informed and responsible decisions when presented with moral conundrums by adding Sherlock Holmes' moral code into ethical frameworks. High ethical standards in data science practice can be upheld by following fundamental pillars such as respect for privacy, accountability, transparency, and truthfulness.
11. Conclusion:
After reviewing the main points, we can say that we have looked at the importance of the "D" that is absent from data science, which is the essential component of deduction. Data scientists can gain from adopting a similar attitude in their analytical process, much as Sherlock Holmes tackles investigations with a rigorous attention to detail and sharp observation.
Through the application of deductive reasoning in data analysis, experts can discover deeper insights that lead to better informed decisions rather than just surface connections. Adopting a holistic strategy that incorporates deductive reasoning makes it possible to comprehend complicated datasets more thoroughly and facilitates the successful resolution of challenging issues.
Enter our inner Sherlock Holmes as we explore the world of data science. We may uncover hidden patterns, make precise inferences, and fully utilize the power of data if we hone our logical abilities and dig past the apparent. Recall that the "D" that is missing in the field of data science could hold the key to opening up new avenues for research and creativity.
12. Call to Action:
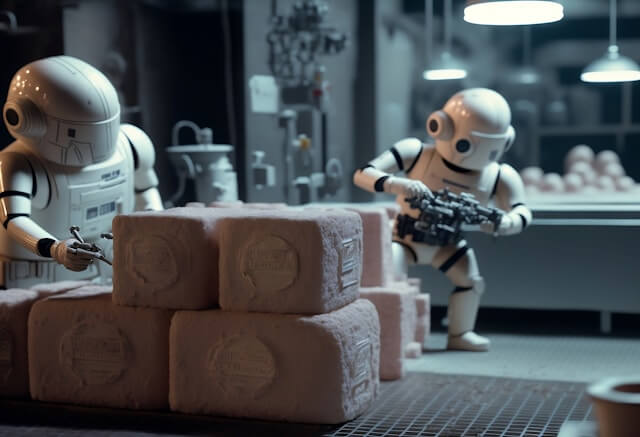
And as I mentioned above, as you take a step back and look at your existing data practices, remember the "D" that is lacking from data science—the importance of detective work and curiosity—something Mr. Holmes would surely emphasize. Adopt an attitude of constant improvement when solving data puzzles and looking for more profound understandings.
Let's explore in a way that goes beyond conventional data analysis techniques. Integrate contemporary analytics methods with the spirit of detective work to find hidden patterns, anomalies, and narratives in the data. Adopting multidisciplinary methods exposes us to fresh viewpoints and techniques that have the potential to completely transform the way we handle data analysis.
Seize this chance to push your team and yourself to approach complicated data challenges with the investigative mindset. Encourage critical thinking, curiosity, and meticulousness at every stage of the data analysis procedure. Let's explore the field of data science together, equipped not only with algorithms but also with the astute observational abilities of a master investigator such as Sherlock Holmes.