1. Introduction to Artificial Intelligence in Manufacturing
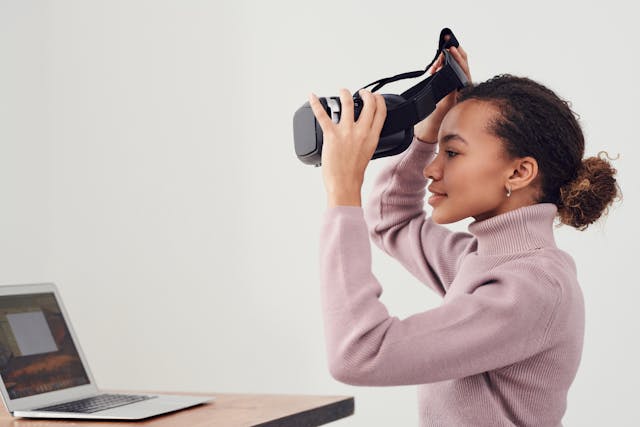
Through the introduction of cutting-edge technologies that improve efficiency, stimulate innovation, and optimize processes, artificial intelligence (AI) has completely transformed the industrial industry. AI is being used in manufacturing for supply chain optimization, autonomous machinery, quality control, and predictive maintenance. Manufacturers can now extract insights from massive amounts of data in real-time, which improves operational efficiency and lowers costs, thanks to the integration of AI.
It is impossible to exaggerate the significance of AI in modern production. Businesses may detect machine faults before they happen by using AI algorithms for predictive analytics. This allows for proactive maintenance, which minimizes downtime and lowers operating expenses. Artificial intelligence (AI)-driven quality control systems detect flaws early in the production process, ensuring constant product quality. By decreasing waste and rework, this improves customer happiness while simultaneously raising overall efficiency.
Judging from everything mentioned above, artificial intelligence (AI) has emerged as a key component of contemporary manufacturing, providing businesses with a plethora of chances to maintain their competitiveness in the ever-evolving market. It is imperative for firms hoping to prosper in the digital era due to its evident influence on decreasing operational costs, enhancing product quality, and stimulating innovation.
2. Applications of AI in Manufacturing
With its many uses, artificial intelligence (AI) is changing the manufacturing scene. The automation of repetitive operations in the industrial process is one important area where artificial intelligence is making waves. Manufacturers may increase efficiency, boost accuracy, and free up human labor for more intricate and strategic jobs by utilizing AI technology like robotic process automation (RPA) and machine learning algorithms.
Predictive maintenance is a key area in which artificial intelligence is used in manufacturing. Manufacturers can maximize production efficiency by anticipating equipment failure and planning preventative maintenance by employing AI-powered predictive analytics. This method helps extend the life of machinery, cut down on unplanned breakdown expenses, and decrease downtime.
AI-powered systems have changed quality control and fault identification. Large volumes of data from cameras and sensors can be analyzed in real-time by machine learning algorithms to find anomalies or flaws in goods during the production process. As a result, producers are able to increase customer happiness, cut waste, and maintain high standards of product quality.
AI integration boosts productivity in industrial processes and creates new opportunities for innovation and efficiency gains in the sector. Adopting AI-driven solutions will be crucial for enterprises hoping to maintain their competitiveness in a market that is changing quickly as technology advances.
3. Enhancing Supply Chain Management through AI
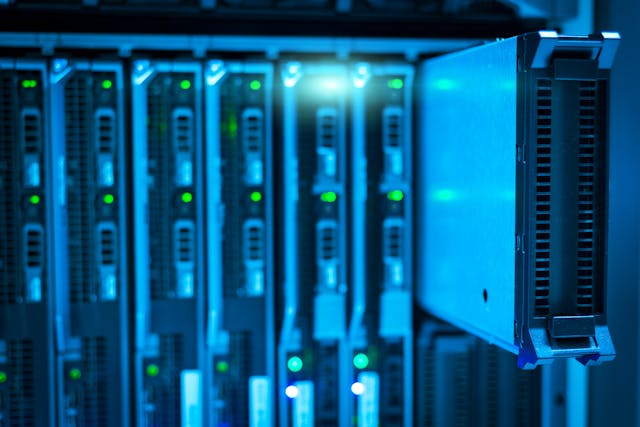
Artificial Intelligence is a key component in manufacturing since it helps to improve supply chain management procedures. Businesses may transform how they handle inventory management and demand forecasting by utilizing AI technology like machine learning and predictive analytics. Businesses can now quickly and accurately analyze massive amounts of data thanks to these cutting-edge solutions, which improves their ability to predict market demands and determine the ideal inventory levels. As a result, producers can lower the cost of surplus inventory while maintaining product availability to satisfy consumer demand.
Predictive analytics powered by AI is revolutionizing manufacturing by optimizing distribution procedures. Businesses may enhance the efficiency and cost-effectiveness of their supply chains by optimizing them with the use of historical data and current insights. Manufacturers can reduce risks and improve overall operational performance by using predictive analytics to foresee future bottlenecks or disruptions in distribution networks and take proactive measures to alleviate these issues. In summary, artificial intelligence (AI) enables manufacturing companies to quickly adjust to shifting market conditions and keep a competitive advantage in the current fast-paced business environment.
Careful planning and strategic execution are necessary when implementing AI for supply chain management. In order to choose the optimal integration strategy, businesses wishing to implement AI technology should first evaluate their current infrastructure and data capabilities. Working with supply chain optimization consulting firms or AI specialists can offer insightful advice and valuable insights during the implementation phase. To optimize the benefits of these new technologies inside the supply chain ecosystem, companies must invest in training staff on how to use them.
Creating an environment that values innovation and ongoing development is essential to using AI successfully in manufacturing supply chains. An organization can break down its silos and move smoothly toward an AI-driven supply chain model by promoting cross-departmental collaboration and knowledge sharing. Accurately assessing the effects of AI integration requires ongoing observation of key performance metrics pertaining to supply chain operations. Businesses can find areas for additional optimization and adjust their AI strategies by gathering input from stakeholders at various organizational levels.
Considering everything mentioned above, we can draw the conclusion that manufacturers looking to improve operational efficiency and competitiveness can gain greatly from incorporating artificial intelligence into supply chain management procedures. In today's increasingly complicated global marketplace, organizations can unlock new prospects for development and sustainability by utilizing AI-powered demand forecasting tools and predictive analytics to optimize distribution networks. Manufacturing organizations may remain ahead of the curve and create long-lasting value throughout their whole supply chain ecosystem by embracing innovation and making smart investments in AI projects.
4. Implementing Robotics and Cobots in Manufacturing
Robotics and cobots combined with AI algorithms improve productivity and efficiency in production. Simplified processes and increased accuracy are made possible by the combination of intelligent algorithm-equipped collaborative robots, or cobots, with robots. On assembly lines, robotics may greatly boost output while preserving uniformity in quality. In a similar vein, using cobots to assist human workers with dangerous or repetitive activities can improve safety. These technologies lead to a more efficient workforce overall by streamlining production processes and freeing up human resources for more intricate and cognitive work.
Robotics has many advantages in material handling and assembly lines. Robots are perfect for repeated jobs requiring accuracy and consistency, such as assembling, welding, painting, or packing. Manufacturers can outperform hand labor in terms of accuracy and speed by automating certain operations. Robots in material handling reduce the danger of injuries from manual lifting operations by quickly and easily moving large loads throughout the production process. Robots are able to work continuously, without pauses or downtime, guaranteeing uninterrupted production plans that optimize output.
Manufacturing processes may now be more flexible and adaptive thanks to the integration of AI and robotics. Manufacturers can dynamically optimize production workflows by using AI algorithms to monitor and modify robot behavior in real-time based on data analytics. This flexibility makes it possible to quickly adjust the manufacturing process to unanticipated disturbances or shifting needs. Artificial intelligence (AI)-powered predictive maintenance helps avert unplanned malfunctions by seeing possible problems before they become expensive ones. Manufacturers may increase their efficiency, production, and competitiveness in the fast-paced market of today by utilizing robotics and AI technologies.
5. Data Analytics for Process Optimization
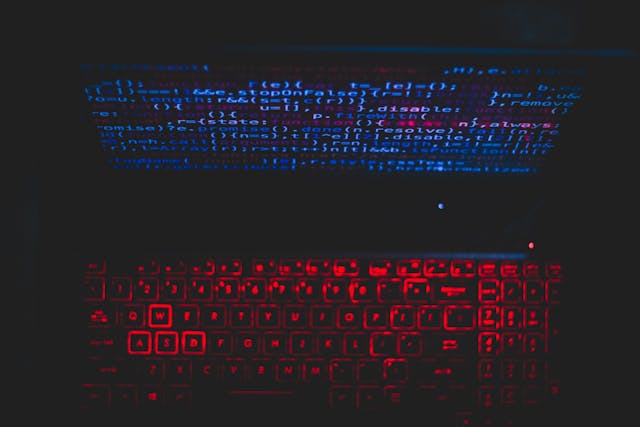
Using big data analytics has become essential for process optimization in the manufacturing sector. Utilizing the enormous volumes of data produced during manufacturing, firms can find insights that result in notable gains in productivity and quality. In this procedure, real-time monitoring and analysis are essential because they allow for proactive decision-making based on current facts. Manufacturers may estimate maintenance needs, find bottlenecks, and improve workflows with this strategy to increase efficiency.
In order to use data analytics for process optimization, cutting-edge technological solutions that can efficiently manage large datasets must be integrated. By applying predictive modeling methods and machine learning algorithms, manufacturers can gain insightful knowledge from unprocessed data, which helps them make well-informed decisions. Manufacturers can identify irregularities early on and take swift corrective action by continuously monitoring critical indicators in real time, such as production line efficiency or equipment performance data.
A data-driven culture must be adopted by the company in order to successfully apply data analytics in manufacturing. This means providing employees with the necessary training to properly interpret and use analytical tools, encouraging cross-departmental collaboration to exchange insights obtained from data analysis, and setting up clear channels of communication to enable prompt resolution of new problems. Manufacturers can keep up with the rapidly changing market conditions and streamline operations while cutting costs by implementing data analytics into their daily operations.
Considering everything mentioned above, we can draw the conclusion that data analytics has enormous potential to revolutionize industrial operations by promoting proactive decision-making and real-time process optimization. Manufacturers can reap the benefits of enhanced productivity, better quality assurance, and smarter resource allocation by adopting big data analytics. Businesses can discover new avenues for growth and innovation in the dynamic manufacturing sector by implementing the appropriate IT infrastructure and demonstrating a dedication to fostering a data-centric mindset throughout the entire organization.
6. Overcoming Challenges in Adopting AI in Manufacturing
Artificial intelligence use in manufacturing has a unique set of obstacles that companies must overcome. Data security and privacy are two of the main issues that are frequently raised, along with making sure that the system is compatible with current ones and workforce training.
When applying AI solutions, privacy and data security are crucial concerns. Manufacturers deal with private information that needs to be protected against online attacks and security lapses. Employing access controls, encryption, and safe data storage strategies should be a top priority for businesses looking to allay these worries. To guarantee the protection of their data during the AI implementation process, they should also think about collaborating with cybersecurity professionals.
Making sure AI is compatible with current systems is essential to its seamless incorporation into production processes. AI applications must integrate with the present infrastructure without generating any hiccups or delays. The secret to optimizing the advantages of new AI technology is to give workers thorough training programs on how to use them. Businesses can enable workers to adopt AI tools and use them to improve productivity and efficiency in industrial processes by providing sufficient training and support.
7. Case Studies: Successful Implementations of AI in Manufacturing
Several notable applications of artificial intelligence in the manufacturing sector are notable examples of creativity and effectiveness. General Electric (GE) is one business that has adopted AI to streamline its production procedures. Through the use of machine learning algorithms, GE was able to precisely forecast the need for equipment repair, which minimized downtime and resulted in significant cost savings.
Another interesting case study is the one about BMW, a car company well-known for its dedication to technological innovation. BMW improved quality control on its manufacturing lines by implementing AI-powered technologies. BMW was able to identify flaws earlier in the manufacturing process with more accuracy thanks to image recognition technology and data analytics, which enhanced product quality and decreased rework.
Leading industrial automation company in the world, Siemens, used AI to improve supply chain efficiency. Siemens enhanced operational efficiency and decreased storage costs by optimizing inventory levels and distribution networks through the use of AI algorithms for demand forecasting and inventory management.
These case studies demonstrate how adopting artificial intelligence may benefit manufacturers in real ways, from greater production and efficiency to significant cost reductions. Manufacturing businesses can set the stage for a more competitive, agile, and sustainable future by leveraging the lessons learned from these successful implementations and customizing AI solutions to meet their unique requirements.
8. Regulatory Frameworks and Compliance Considerations
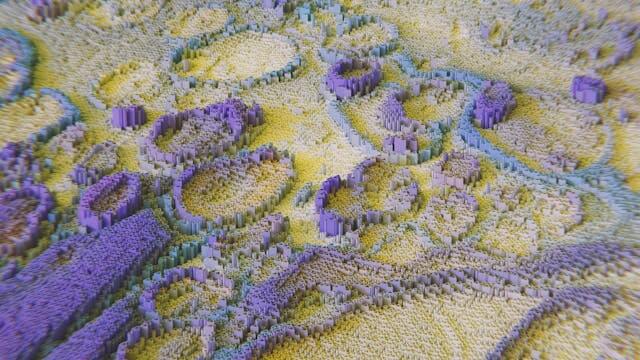
It is crucial to comprehend the legal frameworks and compliance issues when it comes to integrating AI technologies in manufacturing. The employment of artificial intelligence in manufacturing processes can have complicated legal ramifications since these technologies frequently collide with concerns about liability, data privacy, and intellectual property rights. To ensure that AI is used in this industry in an ethical and responsible manner, it is imperative to comply with regulatory regulations.
Manufacturers are required to abide by current rules and regulations, such as those pertaining to product liability, industry-specific standards for information security management like ISO 27001, and data protection laws like GDPR. To reduce risks and maintain regulatory compliance, AI decision-making procedures must be transparent and accountable. Establishing unambiguous policies for data management, model training, and validation can assist businesses in complying with regulatory requirements.
Manufacturers should proactively engage with relevant authorities to be aware about shifting regulatory environments affecting AI technologies, as this will help to promote confidence among stakeholders and regulators. Working with attorneys that specialize in technology law can give you important insights into the particular compliance issues that AI in manufacturing raises. Companies can efficiently negotiate the legal challenges connected with AI implementation by preserving ethical standards and keeping a close eye on developing rules and guidelines.
9. Future Trends: The Evolution of AI in Manufacturing Industry
The integration of edge computing and IoT with AI applications is a crucial topic of focus when examining the future developments of AI in the manufacturing sector. Because of this convergence, real-time data processing is possible right at the source, which speeds up decision-making and improves factory floor operating effectiveness. Through the application of edge computing, manufacturers can enhance dependability, minimize latency, and maximize resource consumption by processing data in closer proximity to its source.
Anticipating how artificial intelligence (AI) will continue to transform processes across multiple industries is crucial to predicting future advances that will influence the manufacturing industry's future. Artificial Intelligence (AI)-driven solutions have the potential to significantly increase efficiency and innovation in industrial operations, ranging from supply chain optimization and autonomous robotics to predictive maintenance and quality control. We may anticipate higher automation levels, better product customization possibilities, and more overall competitiveness for manufacturers adopting these cutting-edge technologies as AI algorithms grow more complicated and capable of tackling difficult tasks.
10. Conclusion: Harnessing the Power of Artificial Intelligence in Manufacturing
Taking into account everything mentioned above, we can say that incorporating artificial intelligence (AI) into manufacturing has many advantages, including higher productivity, better decision-making, preventative maintenance, and better product quality. But issues like exorbitant implementation costs, worries about data security, and the necessity for a highly qualified workforce continue to be major obstacles. Despite these challenges, manufacturers may take use of a wealth of options provided by AI to drive innovation, streamline operations, and maintain competitiveness in the current fast-paced market.
It is recommended that manufacturers adopt AI technologies in order to transform their business processes and open up new opportunities. Businesses may optimize production processes, cut downtime, and eventually increase profitability by utilizing AI-driven technologies like robotics process automation, automated quality control systems, and predictive analytics. In the ever-changing manufacturing market, embracing innovation through intelligent technologies not only increases efficiency but also creates the foundation for long-term prosperity.