1. Introduction:
Introduction: Discovery Analytics is a dynamic approach to data analysis that focuses on exploration and experimentation rather than traditional reporting. It involves delving into datasets to uncover hidden patterns, relationships, and insights that can drive innovation and decision-making. Unlike conventional analytics where specific questions are posed and answered, discovery analytics thrives on curiosity and the willingness to explore data without a predefined agenda.
The advancement of data analysis techniques is greatly dependent on research and development, particularly in the current big data era. Organizations may stay ahead of the curve and develop innovative techniques, algorithms, and tools for gleaning insightful information from complicated datasets by allocating resources to research and development. Through constant research, companies may see new trends, adjust to shifting market conditions, and seize opportunities that might otherwise go undiscovered. Within the field of discovery analytics, research and development (R&D) acts as a stimulant to convert unprocessed data into meaningful insights that inform strategic choices.
2. The Essence of Discovery Analytics:
Within the field of data analysis, discovery analytics relies on the spirit of experimentation and inquiry. It explores unknown territory and goes beyond normal ways to provide hidden truths that might not be visible using more conventional approaches. Through the embrace of curiosity and an open-minded approach to data analysis, discovery analytics facilitates innovation and problem-solving.
Analysts can overcome preconceptions and ingrained tendencies by means of inquiry. They are free to explore databases at their leisure, allowing the information to lead them to fresh insights. Trial and error is a common part of this process, as analysts try out several theories and approaches to see which produces the best insights. This kind of experimentation fosters creativity in problem-solving, empowering analysts to think creatively and develop original answers to challenging problems.
Environments that embrace discovery analytics are ones that foster innovation. Organizations can seize fresh chances for expansion and improvement by fostering an environment of innovation and exploration. This kind of data analysis provides novel insights that may inspire ground-breaking concepts or uncover unrealized potential in already-in-use procedures. Through the utilization of discovery analytics, companies may maintain their competitive edge and adaptability in a constantly changing environment.
3. Tools and Techniques in Discovery Analytics:
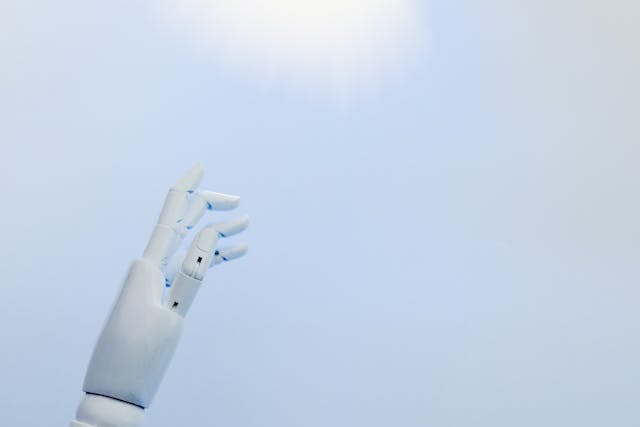
A range of tools are used in the field of discovery analytics to unearth insights from data. These resources include both basic spreadsheets and sophisticated data mining software. Pattern and trend extraction from big datasets is a typical use for data mining technologies like RapidMiner, KNIME, and Weka. By helping to detect significant patterns in the data, pattern recognition tools let researchers draw conclusions from their results that will guide their future actions. Users may visually depict complex data sets with the use of visualization tools like Tableau, Power BI, and D3.js, which facilitates simpler interpretation and comprehension of the available information. Through research and development activities across numerous industries, these technologies play a critical role in fostering innovation and advancement by converting raw data into actionable insights.
One important tool in Discovery Analytics is data mining, which uses algorithms to examine big databases and find trends, connections, and abnormalities. Utilizing methods like association rule mining, regression, clustering, and classification, researchers can obtain insightful information that helps guide decision-making. Finding patterns or structures in data that can be used to forecast future trends or comprehend the underlying relationships between variables is the main goal of pattern recognition. This method is especially useful in areas like natural language processing, picture identification, and fraud detection.
Because it makes it possible for researchers to portray complex data in a way that is simple to understand and evaluate, visualization is essential to discovery analytics. By utilizing interactive charts, graphs, dashboards, heat maps, and other visual aids for data visualization, researchers can get more profound understanding of patterns and connections that might not be instantly discernible by merely examining numerical values. Real-time data analysis from various perspectives is made possible by visualization tools, which promote a deeper comprehension of the underlying relationships and patterns in the dataset. Researchers can more effectively communicate their findings to stakeholders and make more informed decisions based on reliable data produced from their study by integrating visualization tools into their analytical processes.
To sum up everything I've written thus far, a key component of discovery analytics is the integration of analytical tools like data mining software with methods like pattern recognition and visualization. With the use of these tools, researchers may sift through enormous volumes of data in order to find hidden insights that spur innovation and breakthroughs in a variety of fields. Organizations can obtain a competitive edge by using these tools and techniques successfully during research and development processes to make decisions based on thorough analysis of their data assets. It's not about hacking; rather, it's about adopting R&D procedures supported by advanced analytics tools in order to make significant discoveries that influence the direction of industries all over the world.
4. Ethical Considerations in Discovery Analytics:
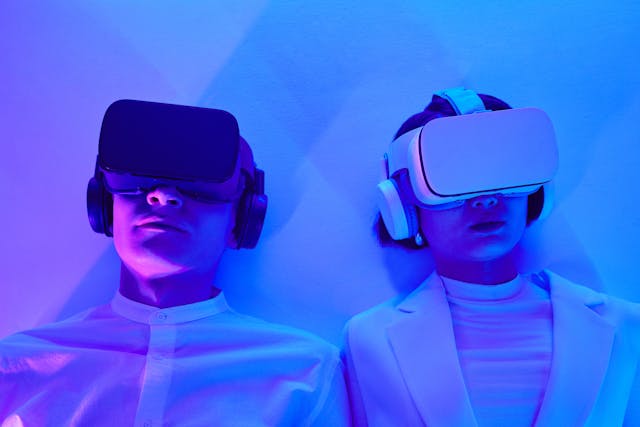
In the field of discovery analytics, examining data necessitates a deep understanding of the ethical implications and concerns. Researchers have to walk a tightrope between accountability and innovation as they mine datasets for insights. Particularly, privacy is a basic concern that calls for strict safeguards to protect private data from misuse or unauthorized access.
If bias is allowed to go unchecked, it can subtly infiltrate analytics research, skewing outcomes and maintaining inequality. It is the responsibility of researchers to guard against biases caused by bad algorithms, insufficient data sampling, or preconceived beliefs. A key component of ethical analytics is transparency, which necessitates open communication regarding methods, conclusions, and any potential drawbacks.
In addition to maintaining the integrity of their work, researchers can foster stakeholder trust by addressing these ethical aspects of data exploration. In today's data-driven environment, incorporating a moral compass into discovery analytics not only improves research quality but also promotes accountability and societal benefit.
5. Case Studies in Discovery Analytics:
Many real-world examples highlight the significant influence of analytics research and development in the field of discovery analytics. In one such case, a sizable retail company used sophisticated data analysis methods to find latent patterns in the purchasing habits of its customers. They were able to manage inventory levels and promotions by discovering a correlation between weather patterns and product sales by thoroughly analyzing their records. The company's revenue rose and significant cost reductions were achieved through the deployment of discovery analytics.
In a different case study related to the healthcare sector, a top hospital improved patient outcomes and resource allocation by utilizing data mining and predictive modeling. They created a tailored medicine strategy that greatly increased recovery rates for some illnesses through extensive analysis of patient information and treatment plans. In addition to providing patients with individualized treatment, this creative application of discovery analytics increased operational effectiveness in the hospital environment.
One of the biggest investment firms in the financial industry employed exploratory data analysis to spot new market trends and investment opportunities before rivals did. Through the application of state-of-the-art algorithms to enormous amounts of financial data, they were able to make strategic judgments that generated significant returns for their clients and obtain insightful knowledge regarding market movements. This success story serves as an example of how discovery analytics can give an advantage in a market that is always changing.
These case studies demonstrate the various industries in which discovery analytics is applied, as well as its revolutionary power in fostering creativity, enhancing decision-making procedures, and producing measurable outcomes. Organizations may stay ahead of the curve in today's data-driven world and uncover hidden jewels within their data landscapes by adopting research and development in analytics.
6. Overcoming Challenges in RandD Analytics:
Many obstacles may appear in the field of R&D analytics, impeding the advancement of creativity and learning. The enormous volume of data that needs to be sorted through in order to extract insightful information is one recurrent barrier. Using sophisticated data processing methods, such data mining software or machine learning algorithms, can help solve this difficulty by streamlining the analysis process and identifying important patterns in the data.
Ensuring data accuracy and reliability is a major difficulty in R&D analytics. Large datasets are utilized for research and testing, and mistakes or inconsistencies in the data can provide findings that are not accurate. Maintaining data integrity and raising the standard of analytic outputs can be accomplished by putting strict data validation procedures into place and routinely checking the data.
Barriers to collaboration inside or between departments or research teams can limit the advancement of R&D analytics. Building an environment of open communication and knowledge exchange within the company is crucial to overcoming this obstacle. Fostering cross-functional cooperation and putting collaborative tools into place can help researchers communicate more easily and work together more effectively.
R&D analytics faces a constant struggle in keeping up with quickly changing industry trends and technological advancements. Effectively adjusting to new technology and approaches can be facilitated for researchers in an organization by fostering a culture of ongoing learning and professional growth. Maintaining a leading position in innovation can be achieved by researchers through the provision of resources and training programs. ๐
To sum up everything I've written thus far, companies may effectively improve their research skills, spur innovation, and make ground-breaking discoveries by identifying these typical R&D analytics roadblocks and putting effective tactics in place to overcome them.๐
7. Future Trends in Discovery Analytics:
Future developments in the field of Discovery Analytics seem likely, thanks to the use of cutting-edge technology like artificial intelligence and machine learning. By enabling more complex data exploration and analysis procedures, these technologies have the potential to completely transform RandD analytics.
Researchers will be able to concentrate on higher-level decision-making and innovation by using AI to automate tasks like pattern identification, anomaly detection, and trend forecasting. Algorithms for machine learning will enable analysts to extract meaningful information from large, complicated datasets, resulting in more precise forecasts and insightful discoveries.
Researchers will find it simpler to work intuitively with data thanks to emerging trends like augmented analytics, which blends natural language processing with data analytics. The development of explainable AI will improve analytical processes' transparency and boost confidence in insights derived from AI.
By combining big data technology with Discovery Analytics, businesses will be able to effectively handle enormous volumes of both structured and unstructured data. Through this combination, extensive data investigation will be made easier, revealing hidden patterns and connections that may lead to ground-breaking discoveries in R&D.
The future of RandD analytics is undoubtedly exciting, as I mentioned above, with cutting-edge technology positioned to completely change the nature of innovation and discovery. Through the use of artificial intelligence, machine learning, augmented analytics, and big data technologies, scholars can unleash novel avenues for data investigation and drive research and development endeavors to previously unheard-of levels.
8. Collaborative Approaches to Discovery Analytics:
Effective use of discovery analytics within businesses requires collaborative approaches. Promoting collaboration and joint endeavors can greatly improve research and development (R&D) analytics. Experts from different departments can contribute their ideas, leading to a more comprehensive knowledge of the data being studied when they are brought together. This kind of cross-functional cooperation promotes creativity and innovation in addition to knowledge exchange.
When it comes to using data analysis to drive innovation, cross-functional teams are essential. Combining different skill sets and areas of knowledge enables a thorough approach to data exploration, resulting in more reliable conclusions and tactics. When people from various backgrounds collaborate on analytics initiatives, their distinct perspectives can inspire fresh concepts and solutions. Through these cooperative efforts, companies are able to make well-informed decisions that are grounded in a comprehensive analysis of facts from various perspectives.
When it comes to discovery analytics, teamwork is crucial in the fast-paced business environment of today. The full potential of data analysis within an organization can be realized by establishing a culture that encourages collaboration and interdisciplinary interaction. Through the recognition of the advantages of cross-functional teams in fostering innovation via data analysis, businesses may maintain a competitive edge and guarantee that their research and development endeavors produce significant discoveries that drive expansion and prosperity.
9. The Intersection of Data Science and RandD:
Traditional processes in research and development (R&D) are being revolutionized by the integration of data science methodologies. Data science improves decision-making processes and spurs innovation by providing R&D activities with an organized and analytical framework. Through the use of data analytics techniques, scholars can glean important insights from intricate datasets, identifying patterns and trends that could have gone missed otherwise.๐คจ
It's amazing how well modern analytics techniques and conventional research methods work together. While experimentation and hypothesis-driven research are important components of traditional R&D, data science offers a data-centric viewpoint that enhances existing methods. Researchers might find novel directions for investigation in addition to more effectively validating preexisting ideas by using sophisticated analytics approaches like machine learning and predictive modeling.
Combining machine learning techniques, statistical analysis, and data visualization, data science provides a methodical approach to research and development. With the use of these technologies, researchers can efficiently examine vast amounts of data, spot patterns, and come to well-informed conclusions based on facts rather than gut feeling. Organizations can expedite the creation of new goods or solutions and streamline their innovation pipeline by incorporating data science into their R&D procedures.
The implementation of data-driven research and development approaches has the capacity to decrease expenses linked to exploratory experimentation. Researchers can prioritize experiments based on expected results, optimize resource allocation, and reduce risks related to unclear factors by utilizing predictive analytics and historical data. This proactive strategy raises the probability of successful outcomes while also improving the efficiency of R&D activities.
Taking into account everything mentioned above, we can say that the nexus of data science and R&D signifies a paradigm change in the way that research is carried out and creativity is encouraged. Through the integration of contemporary analytics methodologies with established research frameworks, institutions can seize novel prospects for expansion and exploration. The mutually beneficial association between data science approaches and research and development endeavors creates the foundation for enhanced operational efficiency, well-informed choices, and ground-breaking breakthroughs that propel advancements throughout various sectors.
10. Key Metrics for Success in Discovery Analytics:
To successfully measure the performance and impact of R&D analytics activities, it is imperative to consider the Key Metrics for Success in Discovery Analytics. Establishing unambiguous performance metrics allows firms to precisely monitor their development and results. Determining success levels and areas for growth is made easier by establishing benchmarks based on these data. Businesses can assess the success of their discovery analytics efforts and make well-informed decisions for subsequent projects by comparing results to predetermined targets. Setting up important indicators is necessary to optimize the return on R&D expenditures and guarantee ongoing innovation in a company.๐ฃ
11. Implementing a Culture of Exploration:
Establishing a culture of exploration is crucial to creating an environment at work that encourages experimentation, curiosity, and lifelong learning. Organizations can implement tactics like setting aside time for research projects, hosting hackathons or innovation challenges, promoting cross-departmental cooperation, and praising and rewarding creative thinking to foster this culture. ๐ญ Establishing environments where staff members are comfortable testing theories, asking questions, and freely exchanging ideas is essential to fostering creativity.
Emphasizing the significance of establishing an atmosphere that fosters innovation in companies is imperative. Establishing trust amongst staff members, encouraging a growth mentality that views failure as a necessary component of learning, and offering opportunities for professional development are all part of this. It is the responsibility of leaders to set an example by being open to trying new things and making errors. Employees may better grasp the motivation for their exploratory efforts and maintain their motivation to innovate by setting clear goals and objectives that are in line with the organization's vision.