1. Introduction
The introduction of big data utility has changed the game in the rapidly changing commercial lending sector. In this sense, "big data utility" refers to the methodical application of large and intricate datasets to improve lending operations. These datasets are gathered from a variety of sources, including market trends, customer transactions, financial records, and more.
Big data has completely changed the way lenders operate by giving them access to previously hard-to-get or hard-to-analyze information about market patterns, borrower behavior, and credit risks. Lenders are now able to reduce risks, expedite procedures, make better decisions, and ultimately increase their bottom line thanks to this revolution. The capacity to promptly and precisely collect and evaluate vast quantities of data has provided lenders with a competitive advantage in the current hectic commercial landscape.
2. Historical Overview of Commercial Lending
The commercial lending sector has historically made significant use of conventional underwriting and decision-making techniques. The main criteria used by lenders to evaluate loan applications are physical assets, credit reports, and previous financial statements. For many small enterprises, this resulted in drawn-out approval procedures and restricted access to loans.
Lenders had a hard time correctly estimating credit risk prior to the big data era. Lender expenses soared and default rates were frequently high as a result of incomplete information. Large volumes of data were difficult to swiftly assess due to manual processes, which caused delays in loan approvals and possibly cost-prohibitive opportunities.
The conventional method of commercial lending was laborious, ineffective, and deficient in the breadth of knowledge required to make wise choices. Big data utility was going to have a revolutionary effect on commercial lending practices, and the sector was ready for change.
3. Emergence of Big Data in Commercial Lending
The use of big data in commercial lending has brought about a substantial change in the way financial institutions assess and appraise possible borrowers. In the past, banks used historical financial data and conventional credit scores to determine risk. However, lenders may now provide a more complete view of a borrower's creditworthiness thanks to the abundance of data that is currently available, including transactional, social media, and other alternative sources.
The need for better decision-making processes through the integration of a larger range of data points gave rise to big data analytics in the lending industry. Lenders are now able to use real-time data and static models to assess risk more accurately thanks to this progress. Financial institutions may now swiftly and effectively process and analyze enormous volumes of data by utilizing cutting-edge algorithms and machine learning approaches.
The commercial lending industry is witnessing a revolution in risk assessment and borrower evaluation through the application of big data. Lenders are better able to decide who to lend to and on what terms if they have access to real-time data on consumer behavior, market trends, and economic indicators. Lenders may now better forecast creditworthiness and defaults by using big data to uncover previously unseen patterns and trends.
Taking into account everything mentioned above, we can say that the use of big data analytics has completely changed the commercial lending market by giving lenders more detailed understanding of the risks that could be involved with a borrower. We may anticipate more advancements in this area as technology develops, which will further improve overall decision-making accuracy and speed lending procedures.📜
4. Benefits of Big Data Utilization
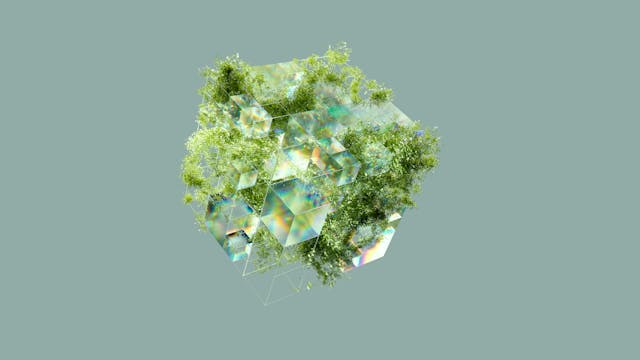
Big data utilization in the commercial lending sector has revolutionized old procedures and brought forth several benefits. A notable benefit is the enhancement of credit decision-making procedures. Lenders can make more informed lending decisions and lower risk exposure by evaluating a borrower's creditworthiness through the analysis of massive volumes of data from several sources.
Lending institutions' operating expenses have decreased as a result of the application of big data analytics. Lenders may optimize operations, reduce human labor, and eventually save operating costs by using algorithms and machine learning models to automate procedures like loan origination, underwriting, and risk assessment.
Numerous exemplary cases demonstrate the benefits of utilizing big data in business lending. For example, banks that use sophisticated analytics tools have reported higher acceptance rates and quicker loan approval times as a result of better risk management. Big data analytics has allowed fintech companies to offer more individualized loan solutions that are catered to the demands of specific borrowers, which has increased consumer satisfaction and given them a competitive edge in the market. 😹
5. Challenges and Risks Associated with Big Data Adoption
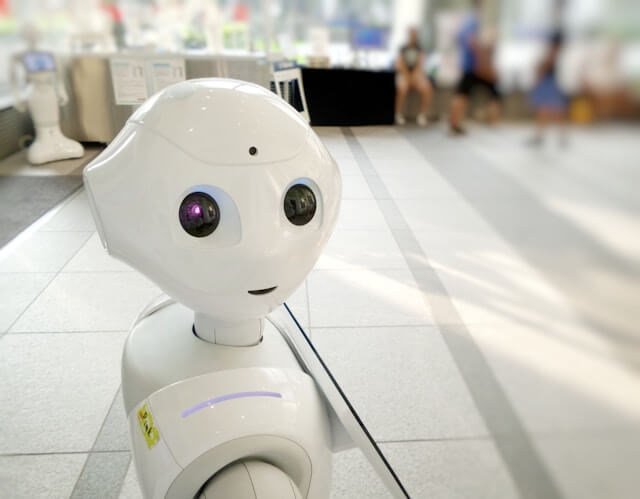
Many dangers and concerns have surfaced as the commercial lending sector begins to adopt big data usefulness; they should be carefully considered. Data privacy is a big worry since so much private information is gathered, which can lead to moral conundrums and legal problems if not handled properly. Maintaining privacy protection while utilizing client data for insights is still a difficult balance to strike.
Another crucial issue to deal with when implementing big data in commercial lending is algorithm bias. Unfair practices in loan approvals or interest rates can arise from biases in previous data or in algorithms themselves, which can lead to discriminatory outcomes. To guarantee fair and impartial decision-making procedures, lenders must actively monitor and reduce biases.
Big data adoption in the lending industry is greatly influenced by legislative barriers in addition to ethical considerations. Complying with regulations like GDPR and HIPAA makes data handling procedures more complicated, necessitating that lenders make investments in reliable systems and procedures to satisfy legal requirements. Businesses hoping to exploit the benefits of big data analytics in lending operations have a significant barrier in navigating these restrictions while pursuing innovation. 😜
The ethical application of big data technology in the commercial lending sector and its sustained expansion depend on addressing concerns about algorithm bias, data privacy, and regulatory compliance. Lenders can leverage big data while preserving transparency and confidence with both customers and regulators by identifying these issues and taking proactive steps to address them.
6. Role of Artificial Intelligence and Machine Learning
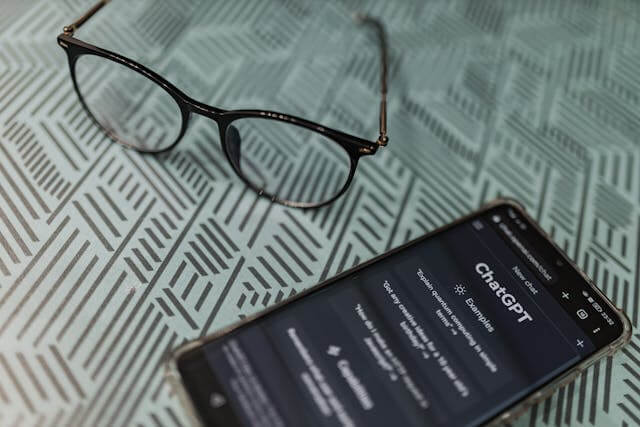
By utilizing big data analytics, artificial intelligence and machine learning have completely changed the commercial lending sector. Large volumes of data combined with AI technologies have made it possible for lenders to reduce risks, expedite procedures, and make better decisions.
Improving loan performance prediction is a major function of predictive modeling, a significant application of AI in lending. Predictive models are more accurate than traditional approaches in predicting the risk of default or late payments by evaluating patterns and trends in past data. This knowledge helps lenders make better decisions and minimize possible losses by helping them evaluate risks more skillfully.
Lenders may more precisely evaluate creditworthiness, comprehend the financial behavior of their clients, and customize loan terms with AI-driven predictive modeling. This degree of customization raises the profitability and overall performance of loan portfolios in addition to increasing customer happiness.
The amalgamation of Artificial Intelligence and Machine Learning with big data analytics has revolutionized the commercial lending domain by providing sophisticated instruments for evaluating risk, making decisions, and fostering client interaction. In the fast-paced world of commercial lending, predictive modeling is a particularly potent tool that improves loan performance prediction and ultimately propels success.
7. Impact on Customer Experience
Big data utility has brought in a new era of individualized customer experiences in the commercial lending sector. Thanks to sophisticated data analysis, lenders can now customize their offers for specific customers, resulting in more advantageous and relevant interactions. This degree of customization raises the possibility of favorable loan outcomes in addition to improving customer happiness.
Through the use of big data insights, lenders are moving toward customer-centric strategies. Institutions are now concentrating on comprehending and satisfying the individual demands of each borrower rather than providing conventional one-size-fits-all solutions. This change makes it possible for lenders to provide clients with terms, prices, and services that are precisely tailored to their individual needs and preferences.
Big data has a significant effect on the commercial loan customer experience. Through customized solutions, it has completely changed the way lenders interact with borrowers, establishing stronger bonds and trust. Prioritizing customer-centric practices powered by big data insights will be essential for the sector to stay competitive and fulfill the ever-evolving demands of today's borrowers as it continues to evolve in this digital age.
8. Evolution of Credit Scoring Models
Conventional credit scoring techniques used to mostly rely on little amounts of information, like payment history and outstanding obligations. These methods frequently missed important details on a borrower's creditworthiness. On the other hand, contemporary data-driven models use big data analytics to evaluate a greater number of variables, such as usage of social media, online transactions, and even utility bill payments.
Through the incorporation of big data, FICO scores, one of the most used credit scoring systems worldwide, have experienced substantial improvements. FICO scores now provide a more complete picture of a person's credit risk profile by combining data from sources other than financial records. Due to this advancement, lenders are now able to provide credit to those who might not have been given it by old scoring algorithms and make more accurate lending judgments.
The lending process is now more accurate and efficient thanks to the incorporation of big data into credit scoring models. The time it takes for a loan application to be approved or denied can be shortened by lenders using a wider range of data points to swiftly determine an applicant's creditworthiness. Borrowers profit from quicker access to funds and more individualized loan proposals that are catered to their unique financial circumstances as a result.
In the commercial lending sector, the development of credit scoring models powered by big data utility represents a major advancement. Lenders may increase the number of borrowers who have access to loans while also making better selections by embracing sophisticated analytics and utilizing a variety of datasets. This change heralds a paradigm shift toward more equitable and effective lending procedures that eventually serve the interests of lenders and borrowers alike.😼
9. Future Trends in Commercial Lending Industry
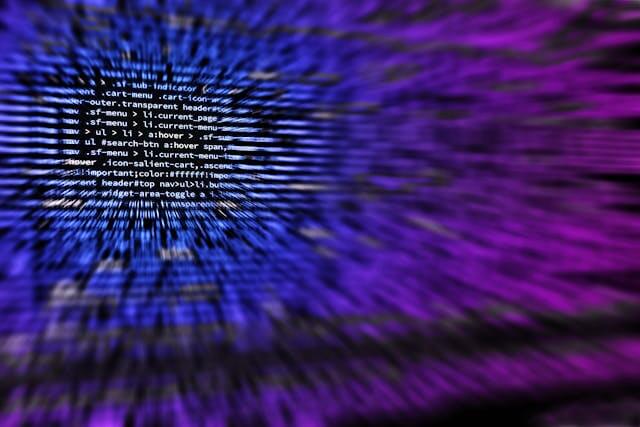
A number of intriguing phenomena are expected to drastically alter the commercial lending landscape as we look to the future of this sector. The use of blockchain technology in smart contract integration is one important development that lies ahead. Lenders can boost the efficiency and trust of agreement enforcement and transaction processing by utilizing the transparent and secure characteristics of blockchain technology. This shift to smart contracts enabled by blockchain improves security while streamlining intricate procedures, cutting down on paperwork and expediting deal closings.
A fascinating trend that has the potential to completely transform commercial lending is the use of augmented reality (AR) in property appraisals, in conjunction to blockchain advancements. Through the use of augmented reality (AR) technology, lenders may do real-time virtual property inspections, improving speed and accuracy in assessing the quality and worth of assets. This innovative usage of augmented reality enhances risk assessment for lenders and gives borrowers a more engaging experience, increasing transparency and efficiency throughout the financing process.
A new era of innovation in the commercial financing sector is being ushered in by the combination of augmented reality for property appraisals and smart contracts driven by blockchain. These developments have the potential to improve consumer experiences, lower expenses, minimize risks, and boost operational efficiency. We may anticipate a more flexible, data-driven approach to financing that is advantageous to lenders and borrowers alike as we adopt these game-changing technologies.
10. Regulatory Environment Adaptations
The incorporation of big data has resulted in a dramatic shift in compliance norms within the commercial lending industry. Regulators have had to adjust as financial firms use massive amounts of data to evaluate credit risks and make lending choices in order to guarantee that these procedures are open, equitable, and comply with current legal requirements. Regulatory authorities have been forced by this change to review and revise their standards in order to stay up with the rapidly changing field of data-driven decision-making.
Going forward, it is anticipated that the regulatory landscape around commercial lending will continue to have a substantial impact on future practices. A greater emphasis on algorithmic transparency, privacy protection, and data security is expected from regulators as financial institutions increasingly rely on machine learning and advanced analytics in their lending operations. More supervision and governance structures that are especially designed to handle the complications brought about by the use of big data in commercial lending may be advocated.
Regulating organizations will be essential in ensuring that lenders follow moral standards, reduce risks related to data usage, and treat borrowers fairly as big data continues to transform the commercial lending sector. We may work toward a balanced regulatory framework that encourages innovation while protecting against potential hazards in this new era of data-driven decision-making by promoting collaboration between industry stakeholders and regulators.
11. Case Studies: Successful Applications of Big Data Utility
Case studies provide fascinating insights into the ways in which big data has transformed the commercial lending sector. A notable illustration of this is Wells Fargo, a well-known banking company that used big data analytics to optimize its loan processing system. Wells Fargo improved its risk assessment capabilities through real-time analysis of massive volumes of consumer data, which led to faster loan approvals and more individualized customer care. This intervention allowed the bank to efficiently offer customized financial solutions by improving decision-making accuracy and saving time.
Lending Club, a peer-to-peer lending platform that transformed the lending and borrowing experience by utilizing big data, is another fascinating case study. Lending Club matches borrowers with potential investors accurately by using algorithms that examine a variety of data factors, including income levels, credit histories, and internet behavior. Thanks to improved risk prediction models driven by big data analytics, this strategy has drastically cut default rates and the amount of time typically spent on underwriting procedures.
Santander Bank is a prime example of how big data has transformed conventional lending methods. Santander Bank was able to expedite the loan approval process by quickly seeing patterns in customer behavior and financial trends by utilizing sophisticated analytical tools on massive datasets. As a result, resources were allocated more effectively, decisions were made with greater accuracy, and customer satisfaction levels were eventually raised. The bank's operational efficiency was revolutionized by the integration of big data utility, which also proved crucial in preserving its competitive advantage in the ever-changing financial services industry.
To encapsulate my earlier writing, these case examples demonstrate how big data has revolutionized commercial lending operations. These real-world examples highlight how exploiting big data utility may generate significant benefits for lending industry organizations, from enhanced decision-making at Santander Bank to accurate borrower-investor matching at Lending Club and quicker loan processing at Wells Fargo. Financial institutions that want to remain competitive in today's market must embrace big data analytics as technology continues to grow at a rapid pace. ðŸ¤
12. Conclusion:
To sum up, the commercial lending business has undergone a revolution thanks to the integration of big data utilities, which have increased risk assessment, sped up decision-making, and improved customer experiences. Through the use of machine learning algorithms and advanced analytics to large datasets, lenders are now able to minimize risks and make better informed loan decisions.
There is a noticeable trend in the commercial lending space right now toward data-driven approaches that put accuracy and efficiency first. Lenders may now operate more efficiently, eliminate manual procedures, and more accurately discover profitable prospects thanks to the use of big data. It has made it easier to create cutting-edge loan products that are suited to the different demands of companies in a range of industries.
Looking ahead, as big data technology continues to progress, the future of commercial lending looks bright. Lenders are anticipated to make further investments in data integrity and security measures to protect sensitive information in light of changing regulatory frameworks and growing cybersecurity concerns. Predictive analytics and artificial intelligence combined are expected to improve credit risk assessment models and increase lending solution customization.
The utilization of big data has transformed the commercial lending landscape by furnishing lenders with timely and actionable information that enable them to promptly adjust to market dynamics. Maintaining a competitive edge in the ever changing business environment will be essential for long-term growth as the sector embraces digital transformation and data-driven decision-making becomes the standard.