1. Introduction
IT security is a field that is rapidly expanding in today's digital world. It is no longer possible to protect against skilled attackers using standard security methods due to the increasing complexity of cyber threats and attacks. As a result, the use of machine learning and sophisticated analytics in IT security procedures has increased significantly. Organizations may more effectively identify, address, and mitigate possible security breaches by utilizing artificial intelligence and data analysis. We will examine the main forces driving this development and how these technologies are changing the cybersecurity scene in this blog article.
2. Evolution of IT Security
The field of information technology security has changed dramatically in the last few decades. In the past, firewalls, antivirus software, and simple encryption methods were the mainstays of security measures used to defend networks and systems against online attacks. But as technology developed, cyberattacks also became more sophisticated. Conventional security approaches found it difficult to stay up with the ever changing strategies used by bad actors.
IT security used to be more reactive than proactive. Rather than anticipating and preventing breaches, organizations frequently found themselves having to respond to them after they happened. This strategy resulted in a never-ending game of cat and mouse between hackers and cybersecurity experts.
Organizations' approaches to defending against cyber attacks have fundamentally changed as a result of the growth of advanced analytics and machine learning in the field of IT security. With the help of these technologies, enormous volumes of data can be monitored and analyzed in real time to find patterns that point to possible hazards. Through the utilization of machine learning algorithms, security systems may continually enhance their threat detection capabilities by adapting and learning from new data points.
The adoption of machine learning and sophisticated analytics signifies a paradigm change in IT security tactics. Organizations can now incorporate proactive security systems that can identify aberrant activity or zero-day attacks based on patterns in data that may not be instantly identifiable using traditional approaches, rather than depending entirely on predetermined rules or signatures to detect known threats.
Organizations can obtain a more comprehensive understanding of the security posture of their IT infrastructures by merging historical data with real-time monitoring and predictive analytics. By taking a comprehensive strategy, threat detection and response capabilities can be improved, which in turn increases overall cybersecurity resilience.
After putting everything above together, we can say that the transition of IT security from conventional techniques to sophisticated analytics and machine learning represents a critical turning point in the fight against cyber attacks. In an ever-evolving digital landscape, firms may better secure their valuable assets and remain ahead of attackers by adopting these cutting-edge solutions.
3. Advanced Analytics in IT Security
Advanced analytics is essential for strengthening defenses against cyberattacks in the field of IT security. The term "advanced analytics" describes the process of analyzing massive amounts of data for patterns and insights using sophisticated methods like machine learning and artificial intelligence. Processing various data sources, including logs, network traffic, and user behavior, is necessary in the context of IT security in order to proactively identify potential threats and vulnerabilities.
There are numerous advantages to using data analytics in IT security. Organizations can improve their threat detection skills by using advanced analytics to spot suspicious activity and anomalies that may go undetected using more conventional techniques. Real-time monitoring, quick incident response, and predictive analysis to foresee potential dangers based on past trends are all made possible by advanced analytics. Security professionals are better able to keep one step ahead of hackers and reduce risks before they become major attacks thanks to this proactive strategy.
There are several uses for data analytics in IT security. Advanced analytics offers cybersecurity experts a full arsenal to protect their organization's digital assets, ranging from identifying vulnerabilities in systems and studying malware behavior to detecting insider threats and advanced persistent attacks. In order to minimize human error and speed up response times to security issues, data analytics can help with automated replies.
A paradigm change towards proactive cybersecurity methods that use data-driven insights to defend against a constantly changing threat landscape is marked by the rise of advanced analytics in IT security. Organizations can enhance their defense mechanisms, optimize security operations, and better protect their vital assets from malevolent actors looking to take advantage of weaknesses in increasingly intricate digital environments by adopting advanced analytics technologies like AI and machine learning.
4. Machine Learning Fundamentals
Machine Learning Fundamentals: In the realm of IT security, machine learning has emerged as a powerful tool to bolster defense mechanisms against a myriad of cyber threats. Fundamental to understanding machine learning in this context is the principle of leveraging algorithms and statistical models to enable machines to learn from and make decisions based on data without explicit programming. By embracing machine learning, organizations can proactively identify patterns and anomalies within vast datasets that signify potential security risks.
Types of Machine Learning Algorithms Commonly Used in Cybersecurity:
Various kinds of machine learning algorithms are essential for strengthening cybersecurity protections. Support Vector Machines (SVM), Random Forest, and Naive Bayes are supervised learning algorithms that are frequently used for classification problems in which the algorithm is trained on labeled data. Algorithms for unsupervised learning, like as clustering and anomaly detection, are essential for spotting unusual network behavior and threats. Systems that employ reinforcement learning algorithms are endowed with the capacity to adjust and improve their security procedures in reaction to past cyber-event results. IT security experts can create strong defense plans that adapt to the ever-evolving threat landscape because to the variety of these algorithms.
5. Applications of Machine Learning in Threat Detection
Because machine learning helps systems recognize trends and abnormalities that can point to cyber dangers, it is essential to improving threat detection skills in IT security. Through real-time analysis of large datasets, machine learning algorithms are able to identify potentially suspicious activity that conventional rule-based systems could overlook. By taking a proactive stance, businesses can keep ahead of emerging cyberthreats and react quickly to possible security breaches.
Anomaly detection algorithms have been successfully applied in the real world to threat detection, allowing for the identification of anomalous network behavior that may be a sign of a breach. These algorithms are able to identify patterns that deviate from the usual, such as sudden increases in network traffic or unwanted attempts to get access, which may indicate a security risk. An further instance involves employing machine learning models to examine user conduct and find deviations from customary usage patterns, which aids in identifying hacked accounts or insider threats. 🙏
Email content, sender behavior, and metadata are analyzed by machine learning for red flags suggesting malicious intent, which is then used in email security to identify phishing attempts. Organizations may automatically screen questionable emails and shield staff members from phishing scams thanks to these sophisticated analytics tools. Endpoint protection solutions employ machine learning algorithms to continuously scan devices for indications of malware activity or compromise, strengthening organizational networks' overall security posture.
Machine learning is used in the field of threat intelligence to go through massive amounts of data from numerous sources in order to find new threats and weaknesses. Organizations can effectively defend against potential attack vectors by using predictive analytics to anticipate them and take preemptive measures. Sophisticated threat hunting platforms powered by machine learning algorithms allow security analysts to quickly evaluate and address problems they find before they become major cyber catastrophes.
The manner in which enterprises protect their digital assets from sophisticated cyber threats has been transformed by the incorporation of machine learning into IT security operations. By embracing the power of sophisticated analytics and artificial intelligence, businesses can strengthen their threat detection skills and mitigate risks more effectively in today's dynamic cybersecurity world.
6. Challenges and Limitations
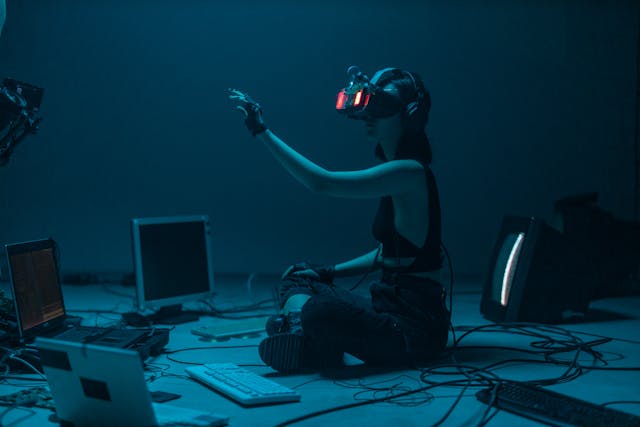
There are various obstacles to overcome when integrating machine learning and advanced analytics into IT security. The dynamic nature of cyber threats poses a significant challenge, necessitating the quick adaptation of models in order to successfully identify novel trends. Another issue is the lack of qualified individuals with experience in both data science and cybersecurity, which is problematic because these intricate systems require specialized skills to build and maintain.
When using machine learning and advanced analytics in IT security, data quality is still a major challenge. The caliber of the input data has a significant impact on the precision and dependability of the insights produced by these technologies. The efficacy of security measures may be jeopardized in the absence of clear, pertinent, and current data, perhaps resulting in false positives or negatives that could endanger companies.
When advanced analytics and machine learning are implemented in IT security, scalability problems may also occur. Conventional infrastructure may find it difficult to effectively meet processing requirements as data quantities increase dramatically. Requiring systems to be scalable to manage substantial data volumes without compromising performance is crucial for deriving significant insights from security analytics.
Another drawback of using advanced analytics and machine learning in IT security is interpretability. Experts in cybersecurity may find it difficult to evaluate or explain outcomes from models developed using complex algorithms, which makes it harder for them to comprehend why particular decisions are made. Developing trust in automated security systems requires clear procedures for interpreting model outcomes.
7. Future Trends
A number of new trends are anticipated to impact advanced analytics and machine learning in the future of IT security. The growing adoption of AI-driven security solutions, which are capable of real-time threat adaptation and learning, is one significant development. Requiring defenses to become more dynamic and adaptive will be essential to fending off more complex cyberattacks.
The merging of data analytics with cybersecurity is another emerging trend. Organizations will have enhanced capabilities to identify irregularities, anticipate possible dangers, and take proactive measures to reduce risks before they worsen by merging the strength of sophisticated analytics with strong security protocols.
Artificial Intelligence and machine learning will find further applications in IT security as cyber attacks get more sophisticated and widespread. Based on past data patterns, predictive analytics will be crucial in predicting possible dangers, and artificial intelligence (AI) algorithms will improve threat detection by spotting minute clues that more conventional techniques would miss.
These technologies are expected to become increasingly more self-sufficient and intelligent in the future, allowing security systems to quickly adjust to shifting threat environments. It is probable that automation technologies and machine learning models will work together to detect and address security incidents instantly, cutting down on response times and minimizing possible harm.
Advanced analytics and machine learning have the potential to significantly improve cybersecurity postures across industries as cyber threats become more sophisticated. Organizations may strengthen their defenses against changing threats and efficiently preserve their digital assets by utilizing these technologies to their full potential and keeping up with new developments.💬
8. Case Studies
The use of case studies is essential for demonstrating the observable advantages of machine learning and advanced analytics in supporting IT security protocols. We can gain a better understanding of how these technologies are changing the cybersecurity landscape by delving into certain real-world cases.
A financial institution that used machine learning algorithms to identify unusual activity within its network is the subject of one interesting case study. By real-time analysis of massive volumes of data, the system identified suspicious patterns that suggested a possible cyberattack. Consequently, the firm successfully prevented a slew of intricate attacks by acting proactively, protecting confidential client data and avoiding monetary losses.
In a different case, a major e-commerce platform improved its fraud detection skills by using advanced analytics. The business was able to quickly and accurately identify fraudulent transactions by using anomaly detection and predictive modeling. By guaranteeing safe online transactions, this proactive strategy not only reduced revenue loss from fraudulent activity but also strengthened customer loyalty and trust.
These case studies highlight the critical role that machine learning and advanced analytics play in strengthening IT security defenses against changing cyberthreats. Organizations may efficiently protect their digital assets and stay one step ahead of criminal actors by utilizing data-driven insights and predictive algorithms.
9. Ethical Considerations
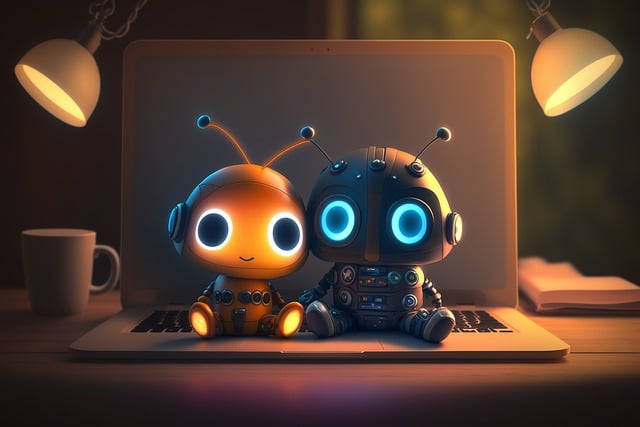
It's critical to address the ethical issues that arise with the implementation of advanced analytics and machine learning in IT security. Privacy is one of the main issues. Large-scale data collection and analysis raises concerns about how private data is gathered, kept safe, and used to improve security measures without violating people's right to privacy.
Algorithmic bias presents yet another important ethical dilemma. The quality of machine learning models is contingent upon the quality of the training data. Biased or inadequate data might produce discriminating results, particularly in delicate domains like security. It is imperative to acknowledge and address biases within these systems to guarantee equitable treatment for every person.
Building trust in machine learning and advanced analytics technologies used for IT security requires transparency. Users need to be aware of how these technologies work, what kinds of data they evaluate, and how judgments are formed in light of their results. Lack of openness can make stakeholders fearful and skeptical, which reduces these instruments' usefulness.
It takes a multidisciplinary strategy combining ethicists, legislators, and legal experts in addition to engineers to address these ethical challenges. Establishing rules and laws that support the ethical application of machine learning and advanced analytics while maintaining the highest standards of privacy, equity, and openness in IT security procedures will require teamwork.
10. Implementing Advanced Analytics Solutions
To optimize the benefits of implementing advanced analytics solutions in IT security, a strategic approach is necessary. The following are doable actions that businesses wishing to implement these cutting-edge technologies can take:
1. **Evaluate Your Existing Security Framework:** It's critical to evaluate your present security infrastructure before deploying sophisticated analytics. Recognize the gaps in your current setup in terms of data sources, workflows, and technologies, and how advanced analytics might assist close them.
2. **Establish Clearly Defining Objectives:** Clearly state what you hope to accomplish with advanced analytics. Whether your goal is to reduce false positives, improve incident response, or improve threat detection, having specific goals can help you plan your implementation strategy.
3. **Select the Appropriate Tools and Technologies:** Make sure the analytics tools and technologies you choose are compatible with your IT infrastructure and goals. These could be predictive analytics software, data visualization tools, or machine learning algorithms.
4. Construct Data Pipelines: To guarantee that data is continuously flowing to your analytics platform from a variety of sources, build strong data pipelines. For advanced analytics solutions to be effective, data quality is essential.
5. **Train Your Team** Educate your IT security staff on the proper use and interpretation of insights produced by sophisticated analytics tools. Giving your staff the tools they need to succeed in implementation is essential.
6. **Implement Use Cases:** To begin, start small by putting use cases into practice that highlight the benefits of advanced analytics in resolving certain security issues. As you become more comfortable with the technology, gradually go into more complicated scenarios.
7. **Performance Monitoring:** Keep an eye on how well your advanced analytics solutions are doing in relation to predetermined metrics and key performance indicators (KPIs). Review results frequently, and adjust as necessary to maximize outcomes.
8. **Integrate with Existing Systems:** Make sure that your current security systems and workflows are seamlessly integrated with advanced analytics solutions. Optimizing efficiency and effectiveness requires compatibility and interoperability.
9. **Remain Current:** Machine learning and advanced analytics are fields that are always changing. To maintain a strong and resilient IT security posture, keep up with emerging trends, technologies, and best practices.
10. Promote Collaboration inside enterprise: To take use of varied experience in cooperatively implementing successful advanced analytics solutions, promote collaboration amongst IT security teams, data analysts, and decision-makers inside the enterprise.🔖
11. The Human Element
Despite advancements in analytics and machine learning, the human element is still indispensable in the field of IT security. Although these technologies are very good at analyzing large volumes of data and seeing trends that point to potential dangers, human cybersecurity specialists also bring intuition, creativity, and critical thinking to the table. Their knowledge and proficiency enhance automated technologies by offering context, sophisticated comprehension, and tactical insights that computers would miss.
The foundation of every effective cybersecurity defensive plan is human expertise. They are able to decipher confusing signals, foresee changing dangers, and come to well-informed conclusions by fusing data analysis with practical experience. Experts may refine algorithms, evaluate findings, and modify methods to successfully address emerging difficulties by collaborating with sophisticated analytics and machine learning systems. By working together, we can combine the best aspects of technology and human nature to build a more robust defense against more complex cyberattacks.
When it comes to ethical issues in cybersecurity measures, human specialists are vital. They are in charge of making sure that the application of machine learning and advanced analytics tools complies with industry rules, ethical norms, and the law. In order to avoid biases in data analysis or decision-making processes that could have serious repercussions for people or organizations, human oversight is crucial. Because of this, human experts serve as gatekeepers in cybersecurity operations to maintain accountability and integrity when automated technologies might fail.
The cultivation of a proactive and adaptive security posture in the current digital ecosystem is contingent upon the synergy of human skills and technology capabilities. Organizations may create a robust defense mechanism that successfully reduces risks and promotes innovation in cybersecurity practices by recognizing the distinct capabilities that each brings to the table: human cognitive thinking and machine computational efficiency. Amidst the rapid evolution of IT security landscapes propelled by advanced analytics and machine learning technologies, the human element remains an invaluable asset.
12. Conclusion
The emergence of advanced analytics and machine learning in IT security, as I mentioned above, signifies a significant change in the way businesses defend against cyberattacks. We have looked at how these technologies allow for faster incident reaction times, proactive threat detection, and anomaly detection that is not possible with human capabilities. Security procedures have been transformed by their capacity to evaluate enormous volumes of data in real-time.
Advanced analytics and machine learning have enormous revolutionary promise for protecting digital assets. Businesses can successfully improve overall cybersecurity posture, detect trends suggestive of assaults, and stay ahead of quickly emerging cyber threats by utilizing these solutions. In an increasingly complex digital ecosystem, the key to reducing risks and controlling vulnerabilities lies in the combination of human experience with machine-powered skills.
As we look to the future, it is obvious that sophisticated analytics and machine learning will continue to play a critical role in bolstering IT security measures. It is now imperative for businesses to adopt these advancements if they want to safeguard sensitive data and keep their consumers' trust. The development of these tools heralds a new era in cybersecurity, one in which proactive defense tactics are not just advised but also necessary to fend off contemporary attacks.😀