1. Introduction
For efficient decision-making and operations, data quality is critical in information-centric businesses. It speaks to the precision, consistency, dependability, and completeness of the data that these businesses maintain and use. Relying on reliable and pertinent information is ensured by maintaining good data quality.
Good data quality has a direct impact on many organizational aspects, including daily operations and strategic planning. Better insights from reliable data result in well-informed decisions that can provide a competitive advantage in the marketplace. Inconsistent or erroneous data may lead to poor analysis and misplaced tactics, which may result in losses of money or harm to the organization's reputation. Setting data quality as a top priority is essential to maintaining organizational efficacy and efficiency.
2. Understanding Data Quality
Businesses that rely heavily on information must comprehend data quality. The condition of qualitative and quantitative information kept in databases or information systems is referred to as data quality. It includes a number of elements, such as timeliness, correctness, consistency, reliability, and completeness. While completeness refers to whether all necessary data is accessible, accuracy measures how well data replicates real-world objects or events. While reliability denotes the dependability of data sources, consistency makes sure that data is consistent across systems and prevents contradictions. The relevance of data at a certain moment is highlighted by timeliness.
Data quality in companies can be influenced by a number of things. These encompass procedures related to gathering, preserving, altering, and obtaining data. Inaccuracies or inconsistencies in the data can result from human mistake during manual entry or processing. Over time, old technological infrastructure or poor maintenance practices might deteriorate data quality. A lack of standard operating procedures or poorly defined business standards might potentially negatively affect the quality of the data. To maintain high-quality data in businesses, external influences like shifting customer expectations or regulatory requirements must be taken into account.
3. Impacts of Poor Data Quality
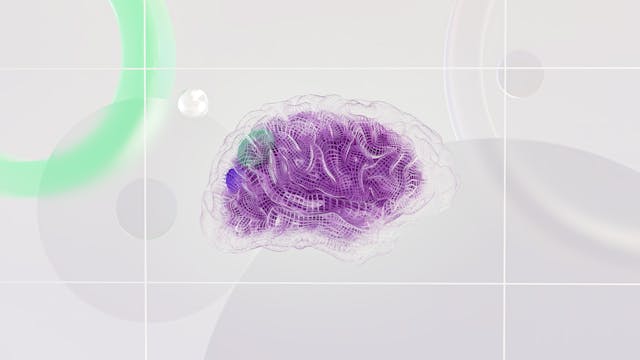
Impacts of Poor Data Quality:
Organizations can be significantly impacted by poor data quality, especially in contexts that are heavily dependent on information. The negative impact of poor data quality on the decision-making process is one of the main effects. Decision-makers may make poor decisions as a result of inaccurate or insufficient data, which could have an adverse effect on the organization's overall performance. It is difficult to precisely evaluate risks, spot opportunities, or predict trends in the absence of trustworthy data.
Poor data quality has an impact on more than just decision-making procedures. Making judgments based on faulty information can have a negative impact on business operations. Inaccurate data can lead to inefficiencies, increased expenses, and missed possibilities for efficiency. For instance, inaccurate customer data may lead to ineffective marketing initiatives or insufficient resource allocation, both of which can negatively impact the competitiveness and profitability of the company.
Customer interactions may be directly impacted by poor data quality. In the modern world, when customized experiences and focused marketing tactics are essential, incomplete client data might result in misdirected communication initiatives. Customers may become irate and lose faith in the company if promotions are sent to the wrong audience groups or with inaccurate contact information. This may eventually lead to a decline in client satisfaction, a rise in churn rates, and a loss of revenue.
To sum up what I've written so far, companies that rely significantly on information for their operations must maintain high standards of data quality. By recognizing the ramifications of poor data quality on decision-making processes and its effects on business operations and customer relationships, organizations may prioritize data quality management measures to prevent against these harmful outcomes.
4. Ensuring Data Quality Standards
Ensuring good data quality standards is critical for information-centric firms to operate efficiently. Such standards must be established and upheld, which calls for careful planning including all facets of data management. Ensuring data accuracy, completeness, and consistency can be facilitated by routine audits and cleansing procedures. Errors can be kept out of the system entirely by implementing data validation rules at the time of entry.
Using cutting-edge tools and technologies is crucial for ongoing monitoring and data quality improvement in addition to these tactics. By analyzing datasets to find anomalies or discrepancies, data profiling tools can reveal possible areas for improvement. By centralizing important company data, master data management systems may guarantee that reliable and consistent data is used in a variety of applications.
Time is saved and less manual intervention is required when mistakes within datasets are found and corrected more quickly thanks to automated data quality methods. These technologies frequently offer features like duplication detection, reference data validation, and anomaly detection to maintain high-quality data standards effectively. The effectiveness of these technologies can be further improved by collaborative platforms that facilitate cross-functional teams working together on data quality projects.
Through the application of strong tactics bolstered by state-of-the-art instruments and technologies, institutions can create a culture of superior data quality standards that serve as the foundation for their decision-making procedures and propel overall business prosperity.
5. Benefits of High Data Quality
In order to succeed in the data-driven world of today, information-centric enterprises must prioritize high data quality. Improving decision-making processes based on precise data analysis is one of the main advantages of upholding high standards for data quality. Organizations are better able to make decisions that propel corporate growth and success when they can rely on clear, trustworthy data.
Significant cost savings and increased operational efficiency are the results of high data quality. Organizations can minimize errors resulting from substandard data and streamline their operations by guaranteeing that their data is precise, consistent, and up to date. In addition to increasing overall productivity, this optimization helps firms avoid wasting money on fixing problems brought on by inaccurate data.
Organizations that prioritize information and want to achieve a competitive advantage in the data-driven world of today should consider making strategic investments to uphold high standards of data quality. By doing this, these firms can enjoy increased operational efficiency that results in significant cost savings as well as improved decision-making processes based on precise data analysis. High data quality is essential for success and should always be the first concern for any company hoping to prosper in the digital era.
6. Case Studies on Data Quality Successes
Prioritizing data quality has paid off handsomely for a number of companies. For example, Company X experienced a significant decrease in operating expenses following the implementation of a data quality management program. They were able to reduce errors brought about by inconsistent information and streamline internal operations by guaranteeing correct and dependable data throughout their systems. Better decision-making, more efficiency, and higher earnings for the business were the outcomes of this.
Another success story is Organization Y, which saw a significant increase in customer satisfaction after making significant improvements to the quality of its data. They achieved this by standardizing and cleaning their customer data, which allowed them to offer individualized services based on client requirements. Better consumer involvement, higher levels of loyalty, and more repeat business resulted from this. Organization Y obtained a competitive advantage in the market and improved their reputation by investing in data quality initiatives.
Those aiming to enhance their data quality procedures might learn a great deal from these firms' experiences. First of all, it emphasizes how crucial leadership support and executive buy-in are for data quality projects. It might be difficult to devote resources and implement significant changes throughout the firm without support from the top. These case studies highlight the necessity of continual oversight and upkeep of data quality procedures. Data quality is a continuous endeavor that necessitates constant evaluation and improvement in order to guarantee ongoing success.
Organizations can get important insights into how to use high-quality data to improve decision-making and overall company performance by looking at these successful stories and comprehending the fundamental ideas that drove their data quality projects.
7. Overcoming Challenges in Maintaining Data Quality
Information-centric enterprises need to maintain data quality, but they frequently encounter challenges in doing so. Typical problems include inconsistent data from manual entry mistakes, duplicate records from non-standardized processes, and insufficient data validation techniques that provide erroneous information. Using automated data validation tools to reduce errors, well-defined data governance standards to guarantee correctness and consistency, and frequent data audits to find and fix inconsistencies are some ways that businesses can get beyond these obstacles.
Investing in training programs to inform staff members on the value of data quality and best methods for sustaining it is one way to tackle these issues practically. In order to enable timely corrective action, organizations can also install data quality monitoring systems that identify inconsistencies in real-time. To ensure a holistic approach to maintaining high-quality data, departments can coordinate their efforts by forming a cross-functional team to supervise data quality activities. Through proactive resolution of these issues, firms can improve their decision-making procedures and obtain a competitive advantage in the current data-driven environment.
8. Regulatory Compliance and Data Quality
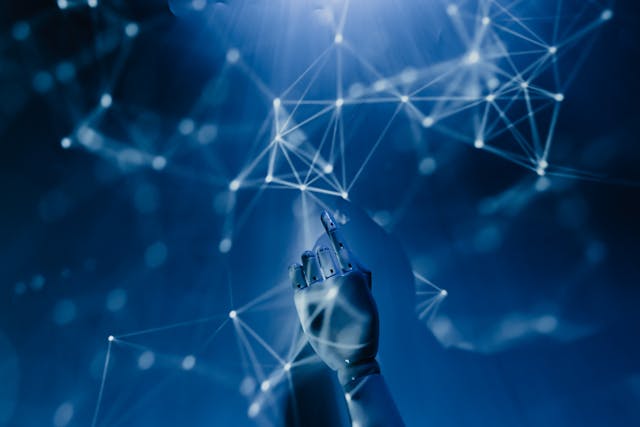
Regulatory compliance and data quality are tightly interwoven in today's information-centric enterprises. Standards are set by regulatory organizations to guarantee the security, accuracy, and dependability of data. Maintaining high-quality data is frequently required by compliance standards in order to safeguard consumer privacy, stop fraud, and maintain transparency. Respecting these rules reduces risks and builds confidence among interested parties.
For firms to successfully meet their regulatory duties, maintaining high-quality data is essential. Businesses can reduce any legal problems and fines by assuring data completeness, correctness, and consistency. Adequate data quality controls protect sensitive information from misuse or unauthorized access and assist firms in demonstrating compliance with regulations like HIPAA and GDPR. Investing in strong data quality procedures improves operational effectiveness and credibility while also preventing non-compliance consequences.
Based on the aforementioned evidence, we may infer that in information-centric firms, the emphasis on data quality is driven by regulatory compliance. Maintaining regulatory compliance necessitates a proactive approach to ongoing management and improvement of data quality. Setting data integrity as a top priority not only protects against legal threats but also establishes confidence with authorities and customers alike. Organizations that place a high priority on data quality will not only be able to comply with the expanding regulations pertaining to data in this period, but they will also be able to strategically employ high-quality data to achieve a competitive advantage.
9. Future Trends in Data Quality Management
Emerging technologies like blockchain, AI, and machine learning are having a bigger influence on data quality standards in the constantly changing field of data quality management. These technologies are transforming how corporations handle data, ensuring accuracy, dependability, and security in an era where data is the new currency. Large datasets can be analyzed by AI and ML algorithms at rates faster than humans can, which makes it easier to spot patterns and irregularities that could point to problems with the quality of the data.
When it comes to data quality management in the future, we should expect a move toward more automated procedures for improving and monitoring data quality. Organizations will be able to take proactive action by using predictive analytics to identify any problems with data quality before they become more serious. Blockchain technology advancements present viable ways to build unchangeable audit trails that improve data records' transparency and credibility.
In order to guarantee greater standards of data quality across industries, the future landscape of data quality management is probably going to witness the confluence of several cutting-edge technologies. Frameworks for data governance will get stronger in order to accommodate the growing amount and complexity of data being produced. Investing in tools and technology that support improved data quality will become more important as long as firms continue to realize how important it is for compliance and decision-making.
Furthermore, as I mentioned previously, emphasizing data quality is non-negotiable as information-centric enterprises navigate through an era marked by exponential growth in data output and consumption. Through the adoption of cutting-edge technology and keeping abreast of developments in data quality management, companies may fully leverage their data assets and minimize the risks associated with incomplete or erroneous information. The people who recognize that maintaining the integrity and dependability of data is essential for long-term success in a world where insights gleaned from it will lead the way.
10. Training and Education for Data Quality Improvement
In information-centric businesses, education and training are essential for enhancing data quality. Employees are better able to comprehend the value of preserving high-quality data when training programs are implemented that concentrate on appropriate data management. Topics include data entry best practices, data validation strategies, and mistake detection and correction approaches can all be covered in these seminars.
Organizations can empower their staff to take responsibility for the data they deal with by offering training on data quality improvement. Workers who understand the fundamentals of data management are more likely to be meticulous, follow data quality guidelines, and take proactive measures to resolve problems as they emerge. Everyone will come to respect precise and trustworthy data as a result, which is crucial for making wise business decisions.
Employees are kept abreast of the newest developments in data quality management through ongoing education programs. Frequent seminars or training sessions can present fresh methods and instruments for improving data quality, giving staff members the know-how to constantly raise the standard and accuracy of data. Putting money into staff training shows that the company is dedicated to upholding strict guidelines for data quality across the board.
Establishing a culture of data excellence in information-centric enterprises requires training and education for improving data quality. Organizations may improve decision-making processes, boost operational efficiency, and ultimately gain a competitive edge in today's data-driven business landscape by equipping staff with the knowledge and skills required to handle and maintain high-quality data successfully.
11. Continuous Monitoring and Maintenance Practices
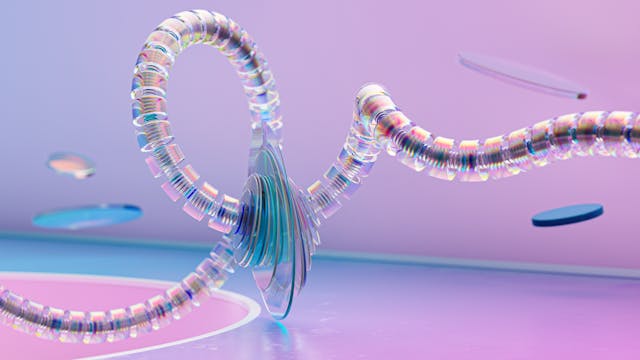
In information-centric companies, maintaining the long-term accuracy of data requires regular maintenance and monitoring procedures. Businesses can quickly find and fix any discrepancies or errors by conducting routine checks and reviews. By being proactive, you may stop possible problems from getting worse and influencing important business choices in addition to maintaining the quality of your data. Additionally, ongoing monitoring guarantees that an organization's data is accurate and up-to-date over time, allowing it to adjust to changing requirements.
In order to create a solid foundation for continuous monitoring, automated procedures that systematically verify data input, storage, and output must be set up. These systems may consist of dashboards, tools for ensuring data quality, or alerts that indicate anomalies or departures from established guidelines. Organizations can optimize their data management procedures and effectively handle any discrepancies that may emerge by utilizing technology for ongoing observation. By taking this proactive approach, you can reduce the possibility of later running into serious problems with the quality of your data, save time and money, and reduce the risks connected with inaccurate or out-of-date information.
A culture of accountability for data quality is fostered inside the company through ongoing monitoring. Every employee becomes a defender of information integrity when they recognize the value of routine audits and take an active role in keeping correct data records. All organizational divisions and levels have a commitment to maintaining high standards of data quality as a result of this shared duty. Businesses can proactively resolve possible mistakes before they negatively impact operations or decision-making processes by instituting this mentality throughout the whole organization.
Based on the aforementioned evidence, we may infer that procedures for ongoing observation and maintenance are essential to maintaining the dependability and correctness of data in information-centric enterprises. Through the implementation of methodical checks, the utilization of technology for automation, and the cultivation of an accountable culture, organizations can protect their data assets against mistakes or gradual degradation. By giving stakeholders timely access to reliable information, continuous supervision improves decision-making skills while also ensuring operational efficiency. Setting a high priority on data quality through ongoing monitoring is essential for businesses hoping to prosper in the fast-paced digital environment of today, where successful operations depend on accurate information.
12. Conclusion:
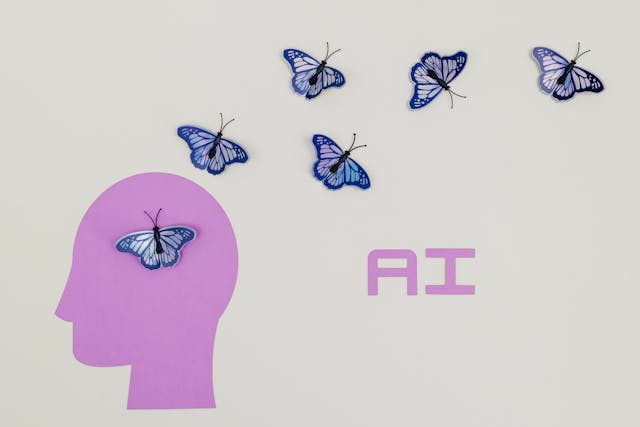
To summarize, information-centric firms must prioritize data quality since it affects decision-making, operational effectiveness, and overall business success. Accurate insights are ensured, errors are minimized, and strategic planning procedures are supported by trustworthy data. Upholding high-quality data is crucial for the organization's culture of credibility and trust as well as for complying with legal requirements.
Long-term benefits of investing in data quality management for an organization include better decision-making, more customer satisfaction, and sustainable growth. Understanding the value of preserving high-quality data as a core asset will help firms position themselves competitively in today's data-driven environment and confidently lead innovation.