1. Introduction:
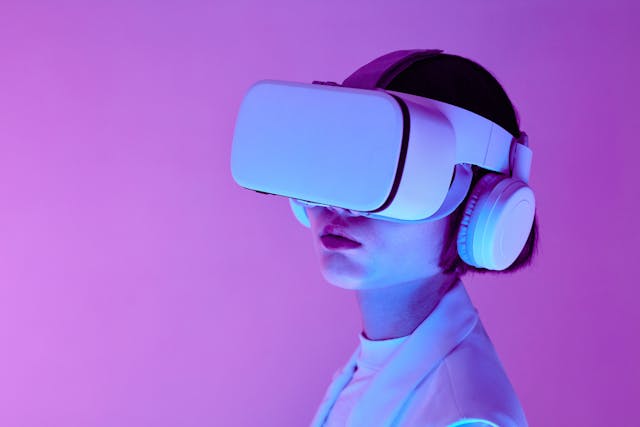
Self-service analytics is a methodology that allows people to access and evaluate data without significantly relying on data scientists or IT specialists. It provides non-technical users with the tools and interfaces they need to explore and extract insights from data. The idea that self-service analytics is a completely independent process is called into question by some constraints, despite the fact that it has gained appeal due to its promise to democratize data.
The notion that users can manage all parts of analytics on their own without assistance, even with the proliferation of self-service analytics solutions, is a little misleading. As it happens, effective data analysis calls on a deep comprehension of data interpretation, context, and potential biases in addition to tool proficiency. This blog article examines the possibility that self-service analytics is not as independent as previously thought and emphasizes the value of technical specialists and business users working together to derive valuable insights from data.
2. Misconceptions about Self-Service Analytics:
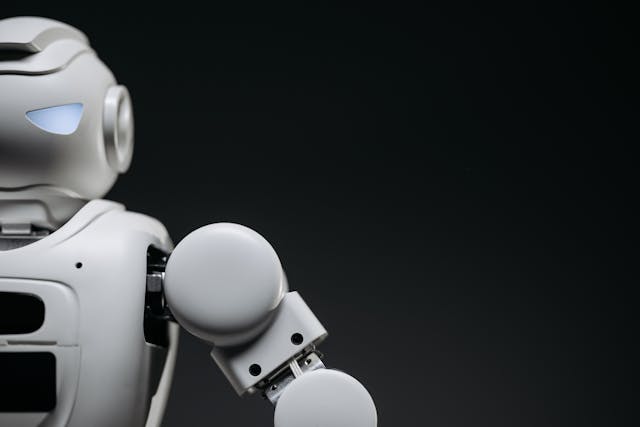
### Misconceptions about Self-Service Analytics
#### Exploring Common Misconceptions
Sometimes people mistake self-service analytics for a miraculous tool that turns any user become an instant data expert. Nevertheless, this idea frequently ignores the significance of context, quality, and data governance. It's critical to realize that self-service does not imply a lack of standards or chaos with regard to data. Without knowing the underlying data structures or biases, users may believe they may obtain accurate insights, which could result in incorrect interpretation and conclusions.
#### Discussing the Limitations of Self-Service Analytics
Self-service analytics has drawbacks even if it provides speed and flexibility in data access. It's possible that users lack the expertise needed to correctly complete complex studies or evaluations. Erroneous conclusions may arise from neglecting appropriate instruction or direction about the interpretation of data. Analytical skills such as critical thinking, subject expertise, and data literacy are crucial yet sometimes overlooked when concentrating only on the self-serve side.
After reviewing the material above, we can draw the conclusion that establishing a more knowledgeable and productive data-driven culture within companies requires first dispelling these myths and understanding the dangers of relying too much on self-service analytics. More insightful insights and well-informed decision-making processes can result from striking a balance between autonomy and supervision, providing users with education and training, and advocating for a holistic approach to analytics.
3. Complexity of Data Analysis:
Data analysis requires a sophisticated grasp of datasets, statistical techniques, and subject expertise. It is not just about crunching numbers. It is impossible to overestimate how difficult data analysis activities can be, as they call for more than just rudimentary technical knowledge. Accurate result interpretation and sound conclusion drawing are prerequisites for meaningful analysis. The likelihood of reading facts incorrectly or coming to poor conclusions increases dramatically in the absence of this knowledge.
In order to guarantee proper data analysis and deduction of insights, expertise is essential. Expert analysts are able to spot trends, correlations, and anomalies that an inexperienced eye could miss. They can use a variety of statistical methods to extract useful information from complicated datasets and find hidden trends. This degree of proficiency is necessary to convert unprocessed data into insightful knowledge that can influence an organization's strategic decision-making.
Today's increasing amount and diversity of data makes specialist skills in data analysis more important than ever. It takes a strong grasp of statistical ideas and procedures in addition to technical expertise with tools like software applications and programming languages to analyze big and diverse datasets. This combination of abilities is essential for addressing the inherent difficulties presented by contemporary data sources and guaranteeing precise and trustworthy analytical results.
Although self-service analytics tools provide consumers some freedom to explore data, the real benefit of data analysis is the skill needed to successfully negotiate its complexity. Organizations can more fully understand the importance of skilled individuals in maximizing the potential of their data assets by recognizing the breadth of knowledge and expertise required for meaningful analysis.
4. Importance of Data Literacy:
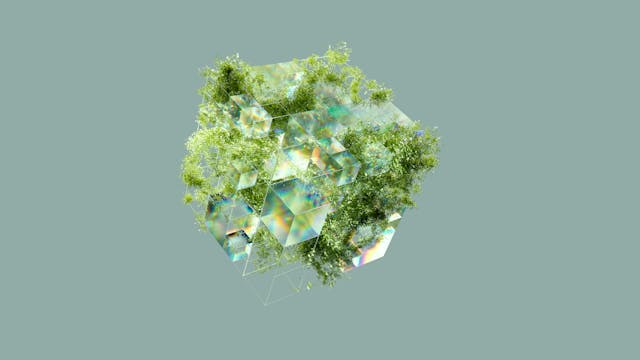
The foundation of successful self-service analytics is data literacy. In order to fully utilize self-service capabilities and the power of data, users need to be proficient in handling and comprehending data. Proficiency in data interpretation, analysis, and insight extraction is essential for successfully navigating the intricate realm of analytics.
People who lack sufficient data literacy skills could find it difficult to make efficient use of self-service analytics solutions. Incompetence can cause facts to be interpreted incorrectly, lead to incorrect inferences, and ultimately impede decision-making processes. Users could become overwhelmed by the abundance of information at their disposal and be unable to derive practical conclusions or meaningful insights if they lack a strong foundation in data literacy.
Thus, if companies want to fully utilize self-service analytics, they must invest in helping users develop their data literacy abilities. Businesses may enable their employees to confidently explore and analyze data on their own, spurring innovation and well-informed decision-making at all organizational levels, by offering training and resources to improve data literacy skills. Encouraging a culture of data literacy is essential to realizing the full potential of self-service analytics.
From everything mentioned above, it is clear that success depends on factors other than technology, even though self-service analytics tools have the ability to completely transform the way businesses use their data assets. It is impossible to exaggerate the significance of data literacy; it is a vital component that supports the efficacy and influence of self-service analytics programs. Organizations may fully utilize self-service analytics and foster sustainable growth and innovation in the data-driven landscape of today by acknowledging the crucial role that data literacy plays and actively fostering its development within teams.
5. Role of Data Governance:
In the field of analytics, data governance is essential since it guarantees the security, quality, and integrity of data. By outlining rules, roles, and duties pertaining to data usage, it offers businesses a foundation for efficiently managing their data assets. Data governance becomes even more crucial in the setting of self-service analytics. It establishes guidelines for data access and usage, ensuring adherence to laws and norms while enabling users to freely investigate insights.
Self-service analytics programs are impacted by effective data governance, which establishes rules for data access, use, and sharing. Users can trust the data they are studying because it sets standards for data quality and consistency throughout the company. Organizations can make sure that self-service analytics processes comply with corporate policies and legal requirements by implementing governance mechanisms including data classification, lineage tracking, and access controls.
Data governance assists companies in finding a balance between reducing the risks associated with improper or illegal use of information and empowering business users to exploit data independently. Governance frameworks facilitate improved collaboration amongst various teams involved in analytics initiatives by encouraging clarity regarding ownership, terminology, and data sources. This cooperative strategy promotes a culture of accuracy and accountability in self-service analytics initiatives.
Self-service analytics efforts that are successful are founded on a foundation of data governance. Organizations can enable users to leverage analytics while reducing risks by establishing explicit policies and procedures that foster trust in data assets. Adopting strong data governance policies is about more than simply compliance; it's about promoting a data-driven culture where safe and trustworthy information is essential for making well-informed decisions.
6. Challenges in Implementation:
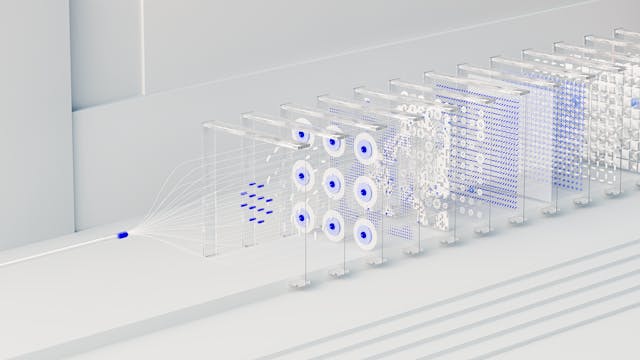
Self-service analytics tool implementation is not without its difficulties. Making sure the tools are simple enough for non-technical individuals to use and navigate is a common challenge. Many users could find it difficult to fully utilize these technologies without the right guidance or assistance, which could result in underuse and frustration.
Concerns about data security and governance provide major implementation challenges. To protect sensitive data, organizations need to set up strict procedures that provide users the freedom to carry out their own analysis. It can be difficult to strike a balance between these demands, and doing so calls for a strong structure that complies with best practices and legal requirements.
The requirement for continual maintenance and upgrades is another crucial element affecting the success of deployment. Self-service analytics technologies need to evolve along with data sources and organizational needs in order to stay relevant and valuable. These tools run the danger of becoming antiquated or unreliable over time if there isn't a dedicated team for upkeep and ongoing development.
Self-service analytics tool adoption success is largely dependent on organizational culture. Adoption rates might be hampered by resistance to change, a lack of support from important stakeholders, or a general distaste for data-driven decision-making. Organizations must make investments in change management techniques that support an empowering and data-literate culture at all levels in order to overcome these obstacles.
Although self-service analytics can provide users with valuable insights, there are a number of obstacles that must be carefully considered before implementing it successfully. Businesses can optimize the advantages of self-service analytics tools and achieve significant results across their operations by addressing usability difficulties, guaranteeing data governance compliance, preserving tool relevance through upgrades, and cultivating a supportive company culture.
7. Balancing Autonomy and Control:
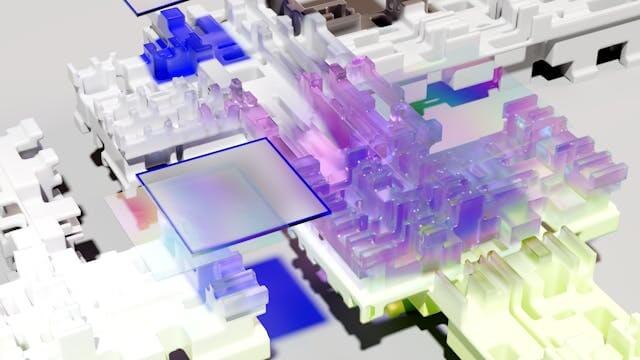
It's critical to strike a balance between control and autonomy while using self-service analytics. Allowing users to freely examine data can encourage creativity and new ideas, but it also raises questions about the security and veracity of the data. In self-service environments, it's critical to strike a balance between giving users the freedom to evaluate data on their own and keeping control over its confidentiality and quality.
Setting precise rules for data preparation, validation, and interpretation is necessary to ensure data accuracy. Enhancing data literacy and giving users access to dependable, current data sources can improve the correctness of analytical outcomes. Self-service users' analysis can remain accurate by implementing data governance procedures like validation procedures, documentation guidelines, and quality control checks.
Self-service analytics also needs to pay attention to security, which is another important factor. Protecting sensitive data from misuse or unauthorized access can be achieved by putting strong security measures in place, such as role-based access rules, encryption methods, and monitoring systems. Enhancing the security posture of self-service environments can be achieved by teaching users about appropriate procedures for managing sensitive data and identifying possible security threats.🎛
In self-service analytics, striking a balance between user autonomy and control is a dynamic process that necessitates ongoing assessment and modification. Through fostering cooperation between IT specialists and business users, institutions can create structures that facilitate both creativity and adherence to data regulations. In self-service analytics efforts, user empowerment and organizational oversight can live together if good data management techniques are emphasized along with experimentation and exploration.
8. The Human Element in Analytics:
Automation cannot fully replace the vital role that humans play in analytics. Self-service analytics tools are convenient, but context, creativity, and critical thinking are all enhanced by human skill in data analysis. Only humans are capable of correctly interpreting data, applying subject knowledge, and posing the relevant questions.
A cooperative strategy blends human intuition with technological strength. It promotes collaboration by putting data analysts and business users together to examine data, corroborate findings, and extract insightful knowledge. When technology and human intelligence work together, creative solutions and more accurate results are frequently produced that would go unnoticed in a self-service paradigm alone.
A fully self-service model, on the other hand, runs the danger of misinterpreting results owing to a lack of human monitoring or ignoring crucial subtleties in the data. Analyses that are sound, pertinent to the business environment, and in line with organizational objectives are guaranteed by human intervention. Organizations may use technology and human expertise to achieve corporate success and make better decisions by embracing the human element in analytics.
9. Leveraging Automation in Analytics:
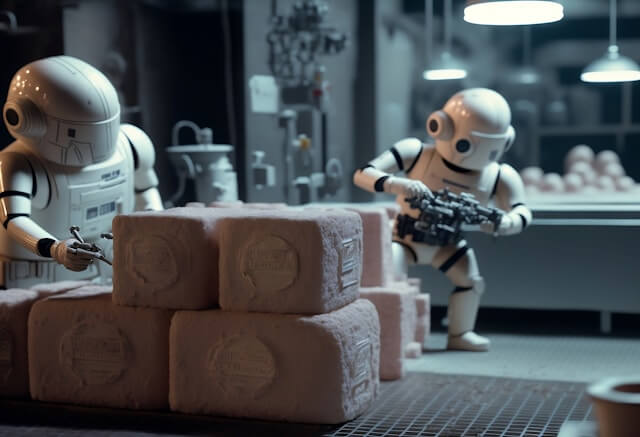
Utilizing automation in analytics streamlines procedures and minimizes manual labor, revolutionizing data analysis. Workflows for data analysis benefit from automation's smooth integration, which increases productivity while maintaining quality requirements. Routine processes like data cleansing, preprocessing, and visualization can be automated to free up analysts' time to concentrate more on gaining insights and making wise choices. This change guarantees accuracy and consistency across analyzes while also speeding up the analytics process. Organizations may now derive meaningful insights from data at scale without sacrificing quality thanks to automation.
10. Future Trends in Analytics:
As far as analytics trends go, we are seeing a big move in the direction of automated and collaborative procedures. Advanced technologies like augmented analytics, which use AI and machine learning to improve data analysis skills, are replacing traditional self-service analytics methods in the sector. As a result of this transformation, a new era in which people may engage with data in more natural ways and rely on intelligent systems to help generate insights while lowering the need for manual involvement is emerging.
The distinction between guided and self-service analytics is becoming more hazy due to emerging trends like embedded analytics, which give customers access to pre-built analytical features in the everyday apps they use. The incorporation of analytics into diverse business tools streamlines the acquisition of insights derived from data, eliminating the need for users to go between distinct platforms or user interfaces. Consequently, companies may enable a larger user base to easily make decisions based on data.
These changing tendencies have a significant impact on the idea of self-service analytics. Although users can already independently examine data thanks to typical self-service technologies, the focus of the data access landscape going forward is on democratizing data access even more through automated insights and integrated analytics. Organizations may establish ecosystems where data flows effortlessly between departments, facilitating knowledge exchange and collaboration, and stimulating innovation and well-informed decision-making at all levels by utilizing technological and design advancements.
Based on everything mentioned above, we may draw the conclusion that, as the analytics sector develops further, the idea of self-service analytics will come to represent more than just empowering individuals; rather, it will also involve establishing an intelligence-driven, data-driven culture within enterprises. In order to facilitate improved decision-making processes at scale, the upcoming trends in analytics are altering how firms approach data analysis by placing a strong emphasis on accessibility, automation, and integration.
11. Case Studies and Examples:
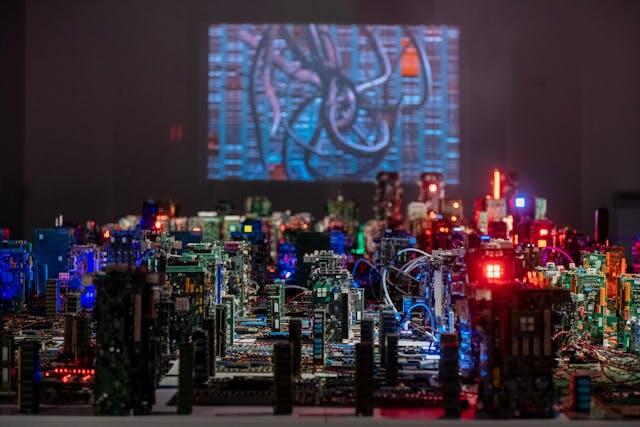
Case studies and actual situations offer insightful information about the difficulties and solutions associated with self-service analytics. One prevalent issue is consumers' lack of data literacy, which can cause results to be misinterpreted or conclusions to be drawn incorrectly. Businesses such as Company X encountered this problem and overcame it by offering in-depth training courses on data analysis techniques and tools.
Self-service analytics solutions provide security vulnerabilities, which is another common challenge. For example, Company Y found it difficult to protect data privacy when allowing access to confidential information throughout divisions. They overcame this challenge by putting strong user authentication procedures in place and protecting data integrity using encryption.
A few essential components are needed for the successful deployment of self-service analytics. Organizations must prioritize investing in strong data governance structures in order to guarantee data compliance, quality, and consistency. Aligning analytics aims with overall company objectives requires open lines of communication between IT specialists and business users. Finally, in order to track the effectiveness of self-service analytics projects and make the required adjustments in real-time, systems for continuous monitoring and feedback should be built.
In today's data-driven environment, businesses may overcome self-service analytics hurdles and take advantage of its potential to drive informed decision-making and sustainable growth by studying these case studies and following the guidelines for effective implementation.
12. Conclusion:
To sum up what I mentioned, it's critical to recognize that, even if self-service analytics greatly benefits users by enabling them to autonomously study data, total autonomy might not always be possible. The intricacies involved in interpreting and analyzing data frequently call for certain knowledge and abilities beyond what a self-service model can offer. It is vital to stress the necessity of a balanced approach in data analysis approaches.
Organizations can leverage user empowerment and maintain accuracy and dependability in their insights by acknowledging the constraints of full self-service analytics and promoting a cooperative effort between users and data experts. A culture of data-driven innovation can be fostered and better informed decision-making processes can result from combining the advantages of expert guidance with user-driven discovery.
The key to achieving successful analytics results is finding a happy medium between professional engagement and self-service capabilities. Through the strategic integration of these methodologies, firms can optimize their data assets and achieve significant commercial results. To truly unleash the power of data analysis, it is not necessary to choose between two extremes; rather, the key is to discover a way to combine independence and expertise in a harmonious way.