1. Introduction
Introduction: In the realm of finance, fraud constitutes any deceptive or unlawful act carried out to gain an unfair financial advantage. This can include activities such as identity theft, credit card fraud, and money laundering. Detecting and preventing fraud is crucial for maintaining the integrity of financial systems and safeguarding individuals and businesses from substantial monetary losses. In recent years, Artificial Intelligence (AI) has emerged as a powerful tool in combatting fraud by leveraging advanced algorithms to analyze vast amounts of data quickly and accurately.
By sifting through massive datasets to find trends, abnormalities, and suspicious behavior that may signal fraudulent transactions, artificial intelligence (AI) plays a critical role in detecting potential fraudulent activity. Artificial intelligence (AI) systems may identify anomalies instantly and generate alerts for additional inquiry by employing machine learning techniques like anomaly detection. AI algorithms can adapt and develop over time by continuously learning from fresh data and strengthening their analytical powers to stay ahead of evolving fraud strategies.
By reducing false positives and improving speed and accuracy of spotting suspicious activity, artificial intelligence (AI) in fraud detection helps businesses save time and money. Artificial intelligence (AI)-driven fraud detection systems can work around the clock, without getting tired or biased, and they can keep an eye out for fraudulent attempts in a variety of financial processes. Financial institutions can bolster their defenses against sophisticated fraud schemes and shield their assets and clients from financial harm caused by fraudulent acts by utilizing AI technology.
2. Understanding Fraudulent Activities
Comprehending the diverse forms of fraudulent actions is crucial for effectively countering fraud. Identity theft, credit card fraud, insurance fraud, and phishing scams are a few of the common forms of fraud that occur nowadays. There are serious risks associated with these activities for people, companies, and even governments.
To trick systems and carry out their crimes, fraudsters use a wide range of techniques. Typical strategies include impersonating someone using a stolen or false identity, credit card theft via payment system vulnerabilities, insurance fraud involving the manipulation of insurance claims for financial benefit, and phishing scams that use misleading emails or websites to collect sensitive information.
Organizations can proactively deploy preventative measures and effectively detect and deter fraudulent actions by leveraging artificial intelligence capabilities and recognizing the many types of fraud and the strategies employed by fraudsters to trick systems. Artificial intelligence (AI) technology offers sophisticated features like anomaly identification, pattern identification, and real-time monitoring that strengthen protection against many types of fraudulent activity.
3. Role of AI in Fraud Detection
By using complex algorithms to examine patterns in huge datasets and identify fraudulent activity early on, artificial intelligence plays a critical role in fraud detection. These artificial intelligence systems are capable of sorting through enormous volumes of data, spotting irregularities and questionable activity that could point to possible fraud cases. Artificial intelligence (AI) technologies contribute to improved fraud detection processes by continuously learning from new data and adjusting to emerging schemes.
The use of machine learning models to identify irregularities in financial transactions is one example of how AI has been successfully applied to fraud prevention. These algorithms have the ability to promptly identify anomalous patterns that could indicate fraudulent activity, such as sudden huge withdrawals, odd purchase locations, or abnormal spending behaviors. Algorithms for natural language processing are used to examine text data, like emails or messages, in order to spot indications of phishing attempts or other fraudulent communications.
Through the analysis of consumer activity patterns, AI-powered solutions have also proven successful in e-commerce platforms in identifying account takeovers and payment fraud. Sophisticated artificial intelligence algorithms are able to quickly identify differences between user profiles and transaction information, triggering security professionals to look into the matter further and stop possible losses brought on by fraudulent activity. The ongoing progress in artificial intelligence technology presents considerable potential for enhancing fraud detection techniques and mitigating vulnerabilities in diverse sectors.
4. Machine Learning Techniques for Fraud Prevention
Machine learning techniques are essential for detecting and stopping fraudulent actions when using artificial intelligence for fraud prevention. Two main methods are frequently employed in the field of fraud detection: supervised and unsupervised learning for anomaly detection.
Through labeled data training, supervised learning enables the model to identify trends in previous fraud cases and apply those insights to fresh data. Conversely, unsupervised learning operates without labeled data and finds abnormalities or outliers in the dataset that could indicate dishonest activity.💿
Because they can visually depict decision rules that categorize data into distinct groups based on attributes like transaction amounts, frequency, location, etc., decision trees are widely used tools in fraud analysis. These decision-making trees simplify complicated processes by reducing them to a set of straightforward questions, which facilitates understanding of the steps taken to arrive at a given conclusion.
Because neural networks can recognize intricate patterns and correlations in data, they are another effective tool in the fight against fraud. Their network of interconnected layers of information-processing nodes is similar to that of the human brain. Because neural networks analyze multiple features at once, they are particularly good at identifying minor trends that could point to fraudulent activity.
After putting everything above together, we can say that when applied to fraud investigation using machine learning approaches, decision trees and neural networks both have special benefits. Decision trees are perfect for understanding decision-making processes because they offer interpretability and transparency. Neural networks, on the other hand, are particularly good at identifying complex patterns in large datasets, which makes it possible to detect fraudulent activity more precisely. Organizations may strengthen their efforts to prevent fraud and safeguard themselves against monetary losses and harm to their reputations brought on by dishonest behavior by making effective use of these technologies.
5. Real-world Applications of AI in Fraud Prevention
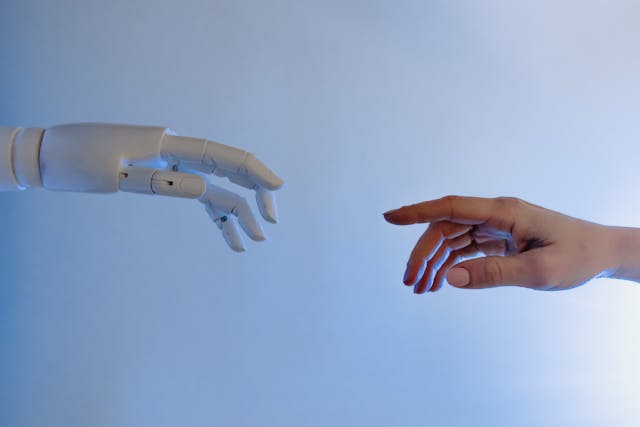
Artificial intelligence (AI) has shown to be remarkably effective at preventing fraud in a variety of businesses. Case studies demonstrate how important AI is in stopping fraudulent activity. Financial firms, for example, use AI systems to scan large datasets for transaction abnormalities and immediately identify possible fraud. By taking a proactive posture, financial fraud has been drastically decreased, saving billions of dollars yearly.
AI-based fraud detection solutions are also very beneficial to the retail and e-commerce industries. These industries are able to quickly identify and stop fraudulent transactions before they happen by using machine learning algorithms to recognize patterns suggestive of fraudulent conduct. This protects companies from monetary damages and, by guaranteeing safe transactions, builds consumer trust.
The insurance industry has experienced significant changes as a result of AI-driven fraud prevention technologies. By utilizing AI algorithms to evaluate claims for authenticity and identify any indications of fraudulent behavior, insurers may now expedite the claims processing process. In the end, this lowers the risks connected with false claims and improves operating efficiency, which saves money for policyholders and insurers alike.
The use of AI in fraud protection has completely changed how businesses fight fraudulent activity, as I said above. By embracing the power of artificial intelligence technologies, organizations across multiple sectors may proactively detect and minimize risks connected with fraud, therefore preserving their operations and boosting confidence among stakeholders. It is anticipated that as AI develops, its ability to stop fraud will become progressively more crucial in protecting companies from ever changing online threats.❗️
6. Challenges and Limitations of Using AI for Fraud Detection
There are obstacles and restrictions when using AI to detect fraud, especially when it comes to moral issues. The application of AI gives rise to worries about invasions of privacy, particularly when personal data is heavily utilized for individual profiling. There are concerns that an excessive dependence on AI systems may result in a reduction in human supervision and accountability for fraud detection procedures. There are concerns around accountability and fairness when it comes to detecting fraudulent activity because AI systems' decision-making process is opaque.
The efficiency of fraud detection attempts can be considerably impacted by biases found in AI algorithms. Due to unbalanced training data or incorrect model assumptions, biases may unintentionally find their way into the algorithms, resulting in discriminating practices in the identification of fraud. In order to avoid some groups being unfairly singled out or shut out due to erroneous algorithmic evaluations, it is imperative to address these biases.
To reduce these obstacles and limits, it is crucial to prioritize fairness, openness, and accountability when employing AI for fraud detection. AI system biases can be found and fixed with the use of techniques like algorithmic audits, bias detection tools, and the generation of different datasets. Encouraging multidisciplinary cooperation amongst technologists, ethicists, and lawyers helps guarantee that ethical considerations are sufficiently taken into account throughout the creation and application of AI-powered fraud detection systems.
7. Impact of AI on Future Fraud Prevention Strategies
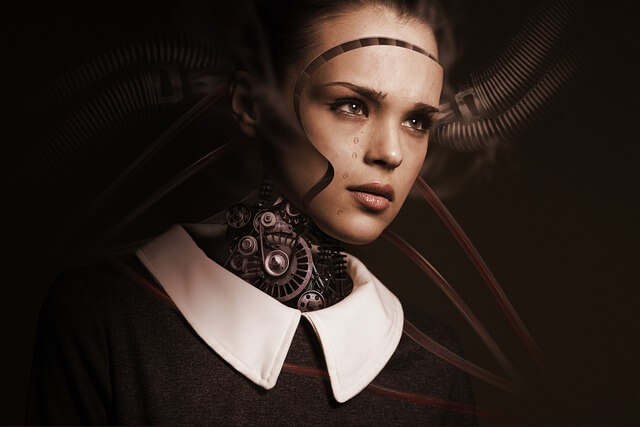
It's expected that artificial intelligence will have a big influence on future fraud prevention tactics. The future of fraud protection is expected to be shaped by advances in AI, which will improve real-time fraud detection, strengthen risk analysis skills, and allow for more accurate threat identification. In order to adapt to new types of fraud and learn from data patterns that point to fraudulent activity, machine learning algorithms will keep developing.
Using deep learning techniques for more accurate detection, natural language processing for text-based data analysis (emails, chat logs), and anomaly detection algorithms to spot outliers in behavior that might point to fraudulent activity are some of the emerging trends in AI-based anti-fraud measures. Stronger fraud prevention efforts will be largely dependent on the integration of AI with other technologies, such as blockchain for secure transactions and biometric authentication for improved identity verification.
Organizations will need to make investments in strong AI-powered solutions as these technologies develop in order to keep up with increasingly cunning scammers. Working together, industry participants, regulators, and AI specialists will be crucial to fostering innovation and creating practical plans that can foresee and stop fraud in the future. The use of AI to proactively detect threats, secure sensitive data, and protect financial transactions in a constantly changing digital environment is the key to the future of fraud prevention.