1. Introduction
The use of algorithms has grown in many facets of our life, including the criminal justice system. Predicting crime is one area where algorithms are becoming more and more popular. These algorithms try to predict where and when crimes might occur, as well as who might be involved, by examining enormous volumes of data and patterns. Technology-assisted crime prevention seems like a great notion, but it also brings up significant ethical and societal issues that should be carefully considered. We will examine the complicated realm of using algorithms to predict crime in this blog article, looking at both the possible advantages and disadvantages of this developing field.
2. Historical Background
The practice of predicting crimes began in the late 1800s, when Italian criminologist Cesare Lombroso introduced the theory of phrenology. According to Lombroso, a person's physical characteristics, such as their face features and skull form, may suggest a tendency toward criminal activity. Early attempts to predict crime based on physical traits were made possible by this idea.
Early in the 20th century, scientists started looking into several approaches to forecast criminal behavior. Psychologists such as William Sheldon proposed a connection between somatotypes and criminal tendencies by linking bodily types to personality qualities. This school of thought gave rise to theories like William Marston's DISC theory, which divided people into four behavioral types with varying degrees of criminal propensity: dominance, influence, stability, and conscientiousness.
The mid-20th century saw a shift in attempts toward employing statistical analysis and behavioral profiling to anticipate crime as a result of technological advancements. Early computerized systems, such as the Violent Criminal Apprehension Program (ViCAP), made an effort to connect crimes by comparing victim profiles or modes of operation. With a shift from pseudoscientific to more data-driven methods, these initiatives represented a major advancement in the field of crime prediction.👍
3. Ethical Concerns
There are serious ethical issues with predictive algorithms used to predict crime. The possibility that these algorithms would reinforce current prejudices and discrimination is a significant problem. The algorithm may unfairly target particular neighborhoods or demographics, aggravating socioeconomic inequities, if the previous crime data used to train these algorithms reflects biased policing methods. Increased monitoring and profiling of already marginalized populations may result from this.
The absence of accountability and transparency in the workings of these prediction algorithms raises additional ethical concerns. People may find it difficult to comprehend the reasoning behind a choice or how it may affect them personally due to the intricacy of these systems. Evaluating these algorithms' accuracy, fairness, and possible harm becomes challenging if they are proprietary or hidden from public view.
Using predictive algorithms to anticipate crime carries the potential of becoming a self-fulfilling prophecy. Law enforcement may unintentionally enhance criminality in certain communities by increasing monitoring and intervention in locations designated as "high-risk" by the algorithm. This vicious cycle has the potential to stigmatize communities and fuel a vicious cycle of increased policing, marginalization, and punishment.
Taking into account all of the aforementioned information, we can conclude that although predictive algorithms have the potential to enhance public safety and law enforcement tactics, their development and application must take ethical considerations into serious account. It is important to implement safeguards to reduce bias, maintain accountability, encourage openness, and stop unfavorable outcomes that can endanger marginalized groups. To develop a more just and equitable system of crime prevention and prediction, it is imperative to strike a balance between the advantages of predictive technologies and ethical precepts.
4. Algorithm Development
The process of developing algorithms for crime prediction is multi-step, starting with problem definition and adequate data selection. Typically, this data consists of demographic data, past crime statistics, and other pertinent details. After that, preprocessing is done on the data to clean and format it for analysis.
After the data is prepared, it is used to train different machine learning algorithms, like decision trees, random forests, and neural networks. The algorithms can forecast future criminal activity by using the patterns they discover during training on historical data.
It is essential to assess the algorithm's performance in order to guarantee accuracy and dependability while predicting crimes. In order to evaluate the model's ability to recognize patterns and generate precise predictions based on the input variables, new data must be tested on the model.
The system must be continuously monitored and improved in order to adjust to shifting patterns and raise prediction accuracy over time. Throughout the algorithm development process, ethical concerns like bias, fairness, and privacy are also crucial to guarantee that predictions do not violate individual rights or perpetuate societal imbalances.
5. Predictive Variables
A wide range of characteristics and parameters are employed in crime prediction algorithms to forecast criminal conduct. Demographic data including age, gender, race, socioeconomic status, and educational attainment are frequently included in these variables. Geographic information such as location and neighborhood features are important components of these algorithms.
Predictive variables in crime algorithms also include behavioral elements including social connections, work position, and prior criminal history. Environmental elements that influence possible criminal conduct include community support services, law enforcement presence, and resource accessibility.
Combining these various predictors enables algorithm developers to build models that try to predict the probability that people will commit crimes. To be sure, using such intricate systems for crime prediction raises ethical questions about prejudice and bias.
6. Accuracy vs. Bias
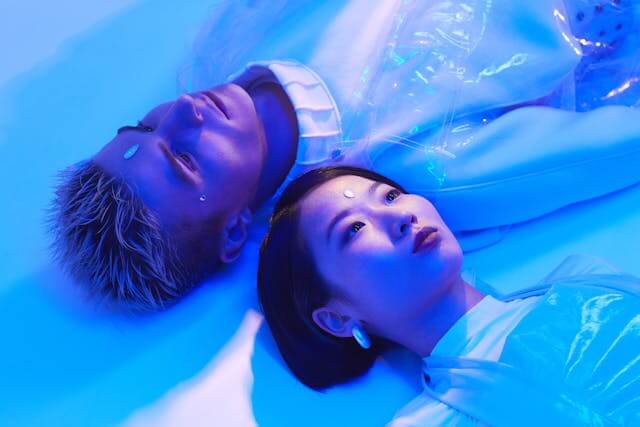
Predictive algorithm debates frequently center on how to strike a careful balance between bias and accuracy. On the one hand, these algorithms' ability to recognize possible threats and stop crimes depends heavily on their accuracy. But sometimes, in the process of pursuing accuracy, biases can seep into the system.
Predictive algorithms may be biased due to a number of factors, including erroneous assumptions made by the algorithm itself or past data reflecting underlying social prejudices. Unchecked biases have the potential to continue injustice and discrimination, especially against marginalized communities that are already disproportionately impacted by structural injustices.
Finding the right balance between bias and accuracy necessitates a careful analysis of both the algorithms and the data that feeds them. Making sure that prediction models are not exacerbating inequities or perpetuating preconceptions requires ongoing attention. We cannot create more moral and efficient algorithms for forecasting crime while maintaining the values of justice and fairness unless we confront prejudice head-on.
7. Real-world Applications
More and more components of the criminal justice system are incorporating predictive algorithms. Pretrial risk assessment is a prominent use case where algorithms are used to evaluate data and forecast the probability of a defendant committing a crime or evading justice if freed before to trial. In an effort to increase efficiency and equity, judges make judgments on bail and release conditions based on these assessments.
The use of predictive algorithms in sentencing recommendations is another important use. To forecast the chance of recidivism, these algorithms examine variables like demographics, the type of incident, and prior criminal history. Judges may find that this information adds context to their choices regarding punishment and may even help identify offenders who would benefit from alternative rehabilitative programs.
8. Impact on Society
There are serious ethical questions about how employing algorithms to anticipate crime would affect society. Supporters claim that these algorithms can make it easier for law enforcement to allocate resources and even help prevent crimes, but detractors warn against the possibility of bias and discrimination that these systems may inevitably contain.
The use of algorithms to anticipate social inequality is a significant source of concern. These algorithms are likely to repeat and even worsen systemic prejudices within the criminal justice system if they are trained on historical data reflecting such biases. This could result in people being erroneously classified as high-risk or certain populations being unfairly singled out for attention due to circumstances beyond their control.
The presumption of innocence and due process are two essential justice concepts that could be compromised by relying solely on algorithmic predictions. The use of opaque algorithms to inform sentencing and policing decisions raises concerns about accountability, transparency, and the right of individuals to contest or appeal these rulings.
Self-fulfilling prophecies provide a risk in which people who are expected to commit crimes are subjected to harsher treatment or heightened surveillance, which may encourage them to commit crimes. This may set off a negative feedback cycle that widens the gaps in trust and exacerbates tensions between communities and law enforcement.
As previously stated, algorithms can help law enforcement combat crime, but it is important to use caution when using them and fully comprehend the effects they will have on society. Maintaining individual rights and civil freedoms while utilizing technology for public safety is a difficult task that calls for constant discussion, supervision, and ethical concerns.
9. Legal Implications
Regarding algorithmic crime prediction's legal ramifications, there are a number of significant issues and difficulties that must be resolved. The possibility of bias in these algorithms, which can provide biased results, is an important consideration. There's a chance that the algorithms' ingrained historical data biases will cause some groups to be unfairly singled out or disadvantaged.
Accountability and openness are two more important factors. Comprehending the decision-making process of these algorithms can provide difficulties, particularly when intricate mathematical models are involved. Concerns concerning due process and people's ability to contest or challenge results that could have an impact on them are raised by this lack of openness.
When personal data is used in crime prediction algorithms, privacy and data protection issues surface. Predictive policing requires the collection and storage of data in a secure manner, with explicit policies governing who may access and utilize the data.
The legality of employing predictive algorithms to make choices that have a big impact on people's lives is under scrutiny. To make sure that these instruments are applied properly and ethically within the confines of the current legal frameworks, the legal system may need to change and adapt.
Maintaining fundamental rights and justice principles while utilizing technology for public safety is a difficult balance that must be struck when navigating the legal terrain of algorithmic crime prediction.
10. Future Trends
We can expect increasingly complex and precise algorithms for crime prediction in the future. Large volumes of data from many sources, including geolocation data, social media activity, and even biometric information, will probably be added to machine learning models to improve them. The combination of these data sets has the potential to increase forecast accuracy and perhaps initiate proactive measures to stop crimes before they happen.
Artificial intelligence developments could make it possible for prediction algorithms to take into account intricate socioeconomic variables like access to healthcare, education quality, and poverty levels when predicting criminal behavior. These comprehensive models have the potential to provide a more thorough knowledge of the underlying causes of crime and assist policymakers in effectively customizing remedies.
Predictive algorithms may be used for crime prevention in a way that is more accountable and transparent. Stricter regulations on algorithm development and application in law enforcement may be imposed by regulatory organizations in response to growing public awareness of issues like bias and privacy concerns. Ethical considerations and innovation must coexist in harmony for predictive police technology to develop responsibly.🖱
11. Case Studies
A closer examination of the potential effects of algorithmic predictions on people or communities can be found in case studies. These algorithms have frequently come under fire for feeding prejudice and upholding discrimination. The story of Robert Williams, a Black man who was falsely detained because of face recognition technology, serves as an illustration of the risks associated with depending too heavily on faulty algorithms that unfairly single out particular demographics.
In the context of predictive policing, localities such as Chicago have expressed apprehension regarding algorithms that purport to anticipate crime hotspots, but could ultimately result in excessive police and surveillance in underprivileged communities. The real-world effects of such tactics are illustrated by the tale of Sam, a young Latino guy who was stopped by police enforcement on multiple occasions not because of actual criminal behavior but rather because of projections based on statistics.
These case studies highlight how crucial it is to consider the moral ramifications of applying algorithms to decision-making processes in law enforcement and other fields. Technology can be a very useful tool for increasing productivity and effectiveness, but its possible effects on social justice and civil rights must be carefully considered before implementing it. To make sure that these tools advance justice and equity for all, it is critical that we place a high priority on fairness, openness, and accountability as we negotiate the tricky intersection of algorithms and crime prediction.
12. Conclusion
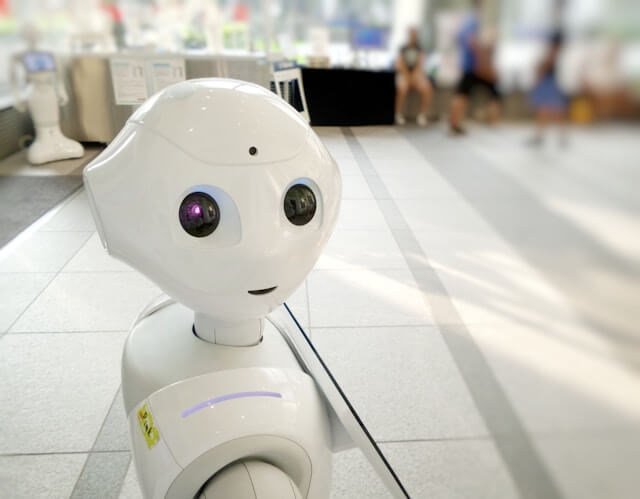
From the foregoing, it is clear that there are moral questions about bias, accuracy, and privacy when algorithms are used to predict crime. These algorithms have the potential to violate civil freedoms and maintain current societal imbalances, even though they can help law enforcement prevent crime. To reduce biases and prevent discriminatory practices, it is crucial to maintain these systems' accountability, openness, and ongoing monitoring.
In terms of algorithmic crime prediction, technological developments that maximize predictive accuracy and reduce bias are probably in store. It will be essential to work together with data scientists, legislators, ethicists, and communities to create frameworks that give justice and fairness first priority in algorithmic decision-making. Raising public knowledge of the drawbacks and hazards associated with algorithmic crime prediction can result in better-informed debates and regulations surrounding its application.
The safeguarding of individual rights and freedoms must be balanced with the possible advantages of algorithmic crime prediction as we negotiate this dynamic terrain of technology and law enforcement procedures. Through a critical and equitable lens, we can tackle this intricate problem and work toward developing more equitable and just systems that handle crime while respecting core human values.