1. Introduction
Demand forecasting is more important than ever in today's dynamic economic environment, especially in light of the "new normal" ushered in by recent international events like the COVID-19 pandemic. Demand forecasting is the process of projecting future consumer demand for goods or services. This information is used to help firms plan their production, inventory, and overall business strategy. Accurate and flexible forecasting is crucial for businesses to adapt and prosper in this new paradigm, as customer behavior is changing quickly and unpredictably.
Because machine learning can analyze vast amounts of data fast and effectively, it has become a useful tool in demand forecasting. Machine learning models can identify intricate patterns in past sales data, market trends, seasonality, and outside variables affecting demand by utilizing sophisticated algorithms and technology. Compared to conventional approaches, this allows organizations to provide projections that are more accurate while minimizing human error and time lag. Machine learning offers real-time insights and predictive skills for decision-making, which is a crucial advantage in the face of extraordinary market disruptions and uncertainty.
2. Understanding Demand Forecasting
Demand forecasting, which involves estimating future demand for goods or services, is an essential part of corporate operations. It supports companies in making defensible choices about resource allocation, inventory control, and production. Precise demand forecasting is crucial in the ever-evolving and dynamic business landscape of today.
Demand forecasting presents new complexities and challenges for organizations in the wake of the pandemic. The epidemic has increased uncertainty, which has reduced the accuracy of standard forecasting techniques. Accurate demand prediction is becoming more difficult due to supply chain interruptions, rapid changes in consumer behavior, and unstable economic conditions. To effectively handle these risks, business executives must modify their forecasting models to include new variables and data sources.
Demand forecasting in light of the "new normal" necessitates a flexible strategy that makes use of machine learning techniques to swiftly assess enormous volumes of data. Businesses may increase the precision of their demand projections and react quickly to shifting market conditions by utilizing machine learning algorithms. After the pandemic, creating strong demand forecasting techniques requires finding a balance between real-time insights and historical data analysis.🫡
3. Traditional Methods vs. Machine Learning Approaches
It is clear that traditional forecasting techniques, such as time series analysis and moving averages, rely on past data and established statistical models when compared to machine learning techniques. Although they work well in some situations, they may not be able to capture intricate patterns or abrupt changes in demand brought on by outside variables. However, machine learning techniques like support vector machines, neural networks, and random forests are better at managing non-linear correlations and are more dynamic in their ability to adjust to shifting patterns.
There are several benefits of employing machine learning to forecast demand. Large volumes of data from multiple sources may be analyzed in real-time by machine learning algorithms, which helps organizations identify subtle trends and changes that may go unnoticed by traditional approaches. When fresh data becomes available, machine learning (ML) models can use techniques like supervised learning or deep learning to constantly learn and improve their forecasts. For more accurate forecasts, these models can simultaneously take into account a wide range of factors, including as promotions, seasonality, weather, and even sentiments on social media.
Compared to conventional methodologies, machine learning techniques provide a more adaptable and scalable approach to demand forecasting. They are proficient in managing high-dimensional data and have the ability to spot intricate patterns that influence customer behavior. ML algorithms may be adjusted and tailored to meet certain business requirements, which allows them to be used in a variety of sectors and product categories. Businesses can obtain a competitive edge by optimizing inventory management, raising customer satisfaction through improved service standards, and ultimately raising overall profitability in this new normal of fluctuating market demands by incorporating machine learning into demand forecasting processes.
And as I mentioned above, while traditional forecasting techniques are useful in some situations, they have inherent limits that become obvious when dealing with the uncertainties of the quickly evolving business environment of today. An effective substitute is machine learning, which uses sophisticated algorithms to produce demand forecasts that are more precise and timely. Through the application of machine learning (ML) to demand forecasting in this volatile and unpredictable new normal, organizations may make well-informed decisions that boost productivity, improve competitiveness, and ultimately promote growth in a constantly changing market.
4. Data Preparation for Demand Forecasting
Accurate demand forecasting requires careful data preparation, especially in the post-COVID era that we currently inhabit. To obtain accurate forecasts, it is imperative to ensure the use of dependable data sources, effective collection techniques, and comprehensive preprocessing. Businesses in the new normal need to modify their strategies to take changing market trends and customer behavior into account.
Accurately reflecting the current market conditions requires gathering data from a variety of sources while preparing for demand forecasting. Sales information, consumer demographics, social media analytics, economic indicators, and more might be included in this. A comprehensive view that can result in more intelligent predictions is provided by combining several data points.
To guarantee the quality of the dataset used for forecasting, preprocessing methods include data cleansing, addressing missing values, normalization, and encoding categorical variables are essential. Machine learning models can function at their best and produce more accurate forecasts if these problems are resolved early on in the process.🎛
In order to effectively capture the distinct dynamics of the market in the post-COVID age, feature selection and engineering methodologies are essential. Companies must determine the pertinent characteristics that affect demand while taking into account fresh elements impacted by shifting customer tastes and outside variables like disruptions in the supply chain or unstable economic conditions. The precision and applicability of demand projections will increase when these strategies are adjusted to handle post-COVID trends.
5. Machine Learning Models for Demand Forecasting
Demand forecasting relies heavily on machine learning, which provides businesses navigating the uncertainty of the new normal with precise predictions. Because of their effectiveness, popular machine learning models like Random Forest, LSTM, and ARIMA have been utilized extensively for demand forecasting. Because of its skill with time-series data and seasonal patterns, ARIMA (AutoRegressive Integrated Moving Average) is a useful tool for forecasting future demand based on past trends. However, time-series data and LSTM (Long Short-Term Memory) models work well with sequential data because they can capture long-term relationships in variations in demand. By using ensemble learning approaches, Random Forest algorithms are highly effective in managing huge datasets with numerous attributes, resulting in reliable forecasts.
Businesses can add new variables to these models that reflect the shifting environment and help them meet the challenges of the new normal. Demand patterns can be greatly impacted by variables including societal trends, economic indicators, and even international occurrences like pandemics. Organizations can improve the precision of their predictions and better equip themselves to handle abrupt changes in consumer behavior by including these factors into the current models. Forecasts must be regularly adjusted to take changing conditions into account in order to stay accurate and relevant in dynamic market situations.
Real-time data streams can provide machine learning models with current insights into demand patterns. As IoT devices and sensors become more widely available, organizations may take advantage of this abundance of real-time data to quickly modify their forecasts. Through the integration of historical data with real-time information streams, machine learning models are better equipped to identify abrupt shifts or irregularities in demand trends, allowing companies to react quickly to changes in the market. This flexibility is essential for managing uncertainty and maintaining competitiveness in the quickly evolving corporate environment of today.
To put it simply, using machine learning models to forecast demand gives companies the flexibility to react quickly to shifting market conditions while also producing precise forecasts. Businesses can acquire a competitive edge in predicting customer requests and optimizing their operations by knowing the advantages of each model and tailoring them to meet certain needs resulting from the new normal. Using machine learning to demand forecasting will be crucial to promoting business resilience and growth in these uncertain times as we continue to navigate through unprecedented times.
6. Evaluation Metrics for Forecasting Accuracy
When it comes to demand forecasting, assessing forecast accuracy is essential to figuring out how well machine learning models work. Mean Absolute Error (MAE), Root Mean Squared Error (RMSE), and Mean Absolute Percentage Error (MAPE) are three important metrics that stand out in this review procedure.
Without taking into account the direction of the errors, MAE sheds light on the average magnitude of the errors. The root mean square error (RMSE) assigns greater weight to significant errors by accounting for both their magnitude and direction. The prediction accuracy is assessed by MAPE as a percentage, which facilitates interpretation in terms of relative performance.
Selecting the appropriate metric is contingent upon the particular demands and objectives of the organization. For example, MAE may be favored if it is necessary to have an impartial perspective on errors, regardless of their direction. However, RMSE may be more appropriate if greater errors are thought to be more serious and should be penalized accordingly. Selecting an evaluation metric that fits the organization's demand forecasting goals and objectives requires an understanding of these subtleties.
7. Incorporating External Factors into Forecasting Models
Prediction accuracy can be greatly increased by adding external factors, such as economic data, into demand forecasting models. Businesses can better understand the larger market trends influencing their sales by incorporating variables like GDP growth, unemployment rates, or consumer confidence indexes. Incorporating lagged values to account for time delays in impact, feature engineering to create new input variables based on economic indicators, and using techniques like regression analysis to quantify the relationships between these factors and demand fluctuations are some strategies to effectively integrate these variables into machine learning models. Utilizing sophisticated algorithms such as XGBoost or LSTM networks can facilitate the identification of intricate interdependencies and nonlinear correlations between external factors and demand signals.👣
8. Real-World Applications and Case Studies
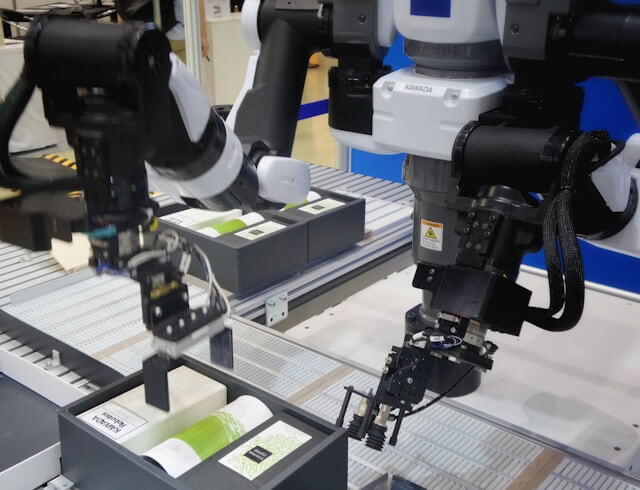
Machine learning has transformed demand forecasting in a variety of industries through practical applications. One such instance is the precise consumer demand prediction made possible by a top e-commerce corporation using machine learning algorithms. The organization drastically reduced stockouts and optimized inventory management by examining previous data and detecting patterns.
Another example is a meal delivery service that used machine learning algorithms to predict demand at peak times. Their forecasts were boosted by the addition of meteorological data and marketing initiatives, which improved resource allocation and raised customer satisfaction.
The observable advantages of using ML in demand forecasting are illustrated by these case studies. The results include lower operating expenses, better overall efficiency, and a higher degree of accuracy in forecasting variations in demand. The key takeaways from these implementations are the necessity of cross-functional cooperation, high-quality data inputs, and ongoing model improvement for the effective integration of ML techniques into current forecasting systems.
9. Challenges and Best Practices in Implementing ML for Demand Forecasting
Businesses have a distinct set of obstacles when implementing machine learning for demand forecasting. Data availability and quality are a prevalent problem. Accurate forecasting depends on the cleanliness, relevance, and timeliness of the data used to train models. To do this, it might be necessary to combine data from several sources and handle missing or inconsistent values.
Choosing the appropriate model parameters and algorithm for a given forecasting task presents another difficulty. Selecting the best algorithm can be difficult due to the abundance of options. It takes experience and experimentation to optimize the model's hyperparameters for best results.
In an organization, managing expectations can be difficult. It's possible that machine learning models won't always instantly beat more conventional forecasting techniques; they're not a panacea. Setting reasonable expectations and deadlines for the application of machine learning-based demand forecasting is crucial.😃
A number of recommended practices can be used to get around these obstacles and increase the likelihood that machine learning for demand forecasting will be implemented successfully. First of all, laying down the project's goals and key performance indicators in advance can help stakeholders understand what is expected of them. This clarity guarantees that efforts are directed toward accomplishing particular corporate objectives.
To create reliable forecasting models, feature engineering and data preparation are essential investments. Machine learning algorithms can more effectively identify patterns and trends in demand behavior by preprocessing and converting unstructured data into insightful characteristics.
Long-term success requires building a feedback loop for models so that they may be continuously assessed and enhanced. Model modification and adaption to shifting market conditions are made possible by routinely comparing the accuracy of forecasts with actual results.
Successful implementation also requires cooperation between domain experts, business stakeholders, and data scientists. Bringing together different viewpoints promotes innovation in the development of successful forecasting solutions suited to particular business needs and allows for a more thorough grasp of demand drivers.
From all of the above, we can draw the conclusion that, even though putting machine learning to use for demand forecasting is not without its challenges, these can be significantly reduced in today's new normal business environment by implementing best practices like maintaining data quality, choosing suitable algorithms, controlling expectations, clearly defining objectives, investing in data preprocessing, setting up feedback loops for model evaluation, and encouraging team collaboration.
10. Ethical Considerations in Demand Forecasting with Machine Learning
Ethical issues are crucial when using AI for demand forecasting. Potential biases in the training data may cause distorted predictions that support discrimination or other injustices. Recognizing the dangers of forecasting models perpetuating preexisting biases is critical. It is crucial to have openness in data sources and model decision-making in order to reduce bias and guarantee equitable procedures. Any inadvertent biases in the algorithms can be found and addressed with the use of regular bias evaluations and diverse participation in the teams. Promoting ethical standards in demand forecasting using machine learning can also be greatly aided by putting fairness criteria into place and carrying out routine audits. Organizations can work toward more equitable and dependable forecasting outcomes that are advantageous to all involved parties by proactively recognizing and correcting biases.📱
11. Future Trends in Machine Learning for Demand Forecasting
Future demand forecasting projections will be increasingly precise thanks to the influence of developing technologies. Deep learning and neural networks, two examples of cutting-edge machine learning algorithms, are completely changing how companies predict demand. Large-scale data analysis is done using these technologies to find trends and insights that conventional approaches could miss. Consequently, businesses are better equipped to decide on resource allocation, production scheduling, and inventory levels.
We can see patterns in the future that will change demand forecasting techniques. Combining machine learning with Internet of Things (IoT) gadgets is one such trend. Businesses may quickly understand consumer behavior and market trends by utilizing real-time data from linked devices. Because of this connectivity, proactive modifications to inventory control and supply chains can be made in response to real demand signals.
The incorporation of artificial intelligence (AI) and predictive analytics into demand forecasting systems is another emerging trend. In addition to forecasting future demand, AI-powered systems can provide prescriptive recommendations for the best places to position products, price products, and run promotions. Businesses may remain competitive in ever-evolving marketplaces by adopting a proactive strategy that allows them to adapt swiftly to shifting consumer demands and market dynamics.
In summary, there are a lot of intriguing prospects for demand forecasting with machine learning in the future. Businesses can improve forecasting accuracy, streamline processes, and eventually obtain a competitive edge in the new normal of global marketplaces by adopting innovative technology and trends in this area.
12. Conclusion
As I mentioned before, this blog has emphasized how important it is to use machine learning techniques for demand forecasting in the dynamic business environment of today. We looked at how conventional forecasting methods might not be able to keep up with the changes and volatility that come with the "new normal." Businesses are able to make more accurate forecasts and proactive decisions by utilizing machine learning models, which provide a more dynamic and data-driven approach to identifying intricate patterns and trends inside volatile markets.
Algorithms for machine learning offer the adaptability required to examine enormous volumes of data, spot obscure patterns, and modify projections instantly when circumstances alter. Businesses can improve their agility in reacting to unforeseen interruptions or shifts in consumer behavior by utilizing these cutting-edge strategies. Beyond just improving prediction accuracy, machine learning plays a significant role in demand forecasting by enabling businesses to manage inventories more efficiently, streamline processes, and ultimately increase customer happiness by making more educated decisions.
Adopting machine learning for demand forecasting becomes not only beneficial but also necessary for maintaining competitiveness as we manage uncertainties in global markets, customer preferences, and supply chains. By utilizing the potential of data-driven insights, companies that proactively adopt these technologies put themselves in a position to succeed amid uncertainty. Integrating machine learning into demand forecasting processes becomes a strategic need for resilience and sustainable growth in the new normal, where adaptation is the key to success.