1. Introduction:
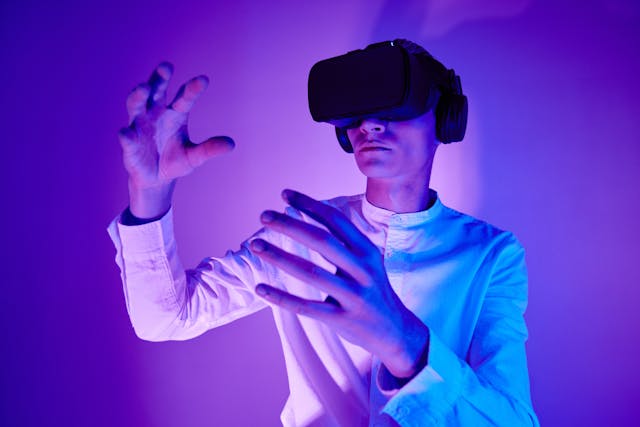
Because cloud computing offers strong resources and tools to effectively analyze and process large amounts of data, it has completely changed the way data scientists work. Data scientists can scale their operations up or down according to their needs thanks to the flexibility of cloud services, which frees them up to concentrate more on drawing conclusions from data than on maintaining infrastructure. The collaboration of data scientists and cloud computing has greatly improved the scalability, speed, and accuracy of data analysis procedures, resulting in more informed decision-making in a variety of businesses.
2. Evolution of Cloud Computing:
The development of cloud computing has revolutionized the field of technology. Its origins can be seen in the timesharing concept of the 1960s, which let several people use a single computer at once. The concept of remote access to computing resources evolved in the 1990s and early 2000s with the advent of grid computing and utility computing.
But the modern era of cloud computing did not really take off until the mid-2000s. Pay-as-you-go cloud services that are scalable and flexible have revolutionized the business, thanks to companies like Microsoft Azure, Google Cloud Platform, and Amazon Web Services (AWS). This period saw a move toward automation, self-service provisioning, and virtualization, which made it possible for companies to grow their operations efficiently and swiftly.
These days, cloud computing is a crucial component of every organization's digital infrastructure. The emergence of technologies such as microservices, containers, and serverless computing has significantly expanded the realm of what is feasible in the cloud. In order to satisfy the changing needs of organizations, cloud providers are always innovating with new services and solutions as data quantities increase dramatically and computational requirements get more complicated.
Through its development, cloud computing has gone from being a cutting-edge idea to becoming a cornerstone of contemporary IT architecture. With its capacity to provide on-demand access to nearly unlimited compute and storage resources, cloud computing has empowered data scientists to solve challenging issues at scale. The continuous progress in cloud technology portends a promising future in which data scientists can take advantage of state-of-the-art instruments and platforms to propel creativity and understanding beyond imagination.🫥
3. Role of Data Scientists in the Cloud:
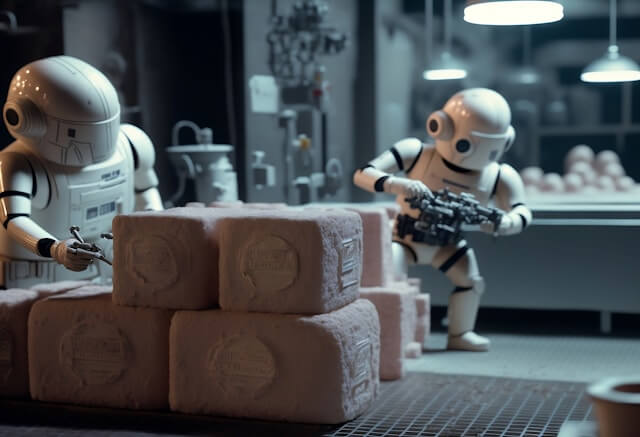
When it comes to utilizing cloud technology for their work, data scientists are essential. Data scientists may quickly and effectively access enormous amounts of data housed on distant computers by utilizing the power of cloud computing. They process, analyze, and visualize data on a scalable infrastructure that adjusts to their computational demands by using cloud-based tools and platforms. As a result, they are able to manage large data sets without being constrained by conventional on-premises systems.
Data scientists may easily experiment with alternative models and algorithms thanks to cloud computing. They can deploy machine learning models at any size, execute intricate simulations, and test hypotheses with ease by spinning up virtual computers or containers. Because cloud resources are available on-demand, data scientists may concentrate more on drawing conclusions from data rather than handling infrastructure, which boosts productivity and encourages creativity in their work.
Cloud platforms facilitate collaboration across data science teams, promoting knowledge sharing and speeding up the creation of sophisticated analytical solutions. Regardless matter where they are in the world, data scientists can work together in real time on shared datasets, notebooks, and workflows. In addition to increasing team productivity, this collaborative atmosphere fosters a culture of ongoing learning and development among data scientists.
Furthermore, as I mentioned earlier, the way data scientists approach their work has changed dramatically as a result of the incorporation of cloud computing technology. Their ability to address intricate issues with increased dexterity and sophistication, coupled with the generation of valuable business insights from huge datasets, has been enhanced. The capabilities and influence of data scientists in realizing the full potential of big data analytics across multiple industries will grow along with cloud computing.
4. Benefits and Challenges:
Data scientists can process large amounts of data effectively in a cloud computing environment because of its scalability. To increase productivity, cloud platforms also give users access to cutting-edge resources and tools for machine learning and data analysis. Because team members may work on shared projects in real time from anywhere in the world, collaboration is made easier. Because cloud services do not require significant upfront infrastructure costs, they provide affordable alternatives.
However, when working in the cloud, data scientists face difficulties guaranteeing data security and privacy. Strong security measures must be put in place while handling sensitive data in order to guard against illegal access and data breaches. Consistency and integrity issues can also arise from the complexity of managing various datasets in various cloud settings. When using cloud computing resources for their projects, data scientists constantly have to worry about avoiding latency issues and optimizing performance.
For data scientists operating in a cloud environment, striking a balance between the advantages of scalability and sophisticated tools and the difficulties of data protection and performance optimization is essential. Data scientists may optimize the benefits of cloud computing while protecting sensitive data and guaranteeing smooth operations by proactively tackling these obstacles through strategic planning and implementing best practices. 🤝
5. Real-world Applications:
Empirical implementations offer concrete illustrations of the potent interplay between cloud computing and data science. Netflix is an impressive example of a case study, as it makes use of cloud platforms to assess viewer preferences and customize content suggestions. Using services like Amazon Web Services (AWS), Netflix processes large datasets to improve user experience by making tailored recommendations.
Another notable example is Airbnb, which improves its search algorithm and offers tailored lodging recommendations by using cloud services for data analysis and storage. Because of the cloud's scalability, Airbnb is able to adapt to changing visitor volumes with ease and keep enhancing the services it offers by using data analytics to gain real-time insights.
Cloud computing is used by healthcare institutions such as Mount Sinai to process large volumes of patient data for predictive analytics, which leads to more precise diagnosis and treatment recommendations. Cloud platforms' cost-effectiveness and flexibility enable healthcare providers to fully utilize data science's potential to transform patient care.
These case studies highlight how data scientists' skills are enhanced by the smooth integration of cloud computing across a range of industries, leading to improved productivity, creativity, and results for both end users and enterprises.
6. Future Trends:
As both professions continue to develop, the interaction between data scientists and cloud computing is expected to grow in the future. Data scientists will require increasingly specialized tools and platforms from cloud computing, which will cater to their demands. To manage bigger datasets and more intricate studies, data scientists will depend more and more on the scalability, flexibility, and computational power of cloud solutions.
Data scientists will utilize cloud-based AI services for image identification, natural language processing, predictive analytics, and other applications as AI and machine learning technologies develop. This partnership will lead to more efficient deployment procedures, enhanced algorithmic accuracy, and quicker model training. There will be a concentrated push to create safe cloud environments that adhere to strict data protection laws as worries about privacy and security increase.
Serverless computing models, which completely abstract infrastructure administration from data scientists and let them to concentrate on drawing conclusions from data without having to worry about backend upkeep, are likely to become more prevalent. Data scientists will be able to efficiently process real-time data streams closer to the source thanks to the combination of edge computing with cloud services.
Prospective prospects abound for the convergence of data science and cloud computing. Through this relationship, AI-driven decision-making processes will become more innovative, faster time to insights will be possible, and computational resources will be used more effectively. Organizations can maintain a competitive edge by promptly adjusting to these new trends and fully utilizing their data assets by employing cutting-edge cloud technology under the direction of knowledgeable data scientists.
7. Tools and Platforms:
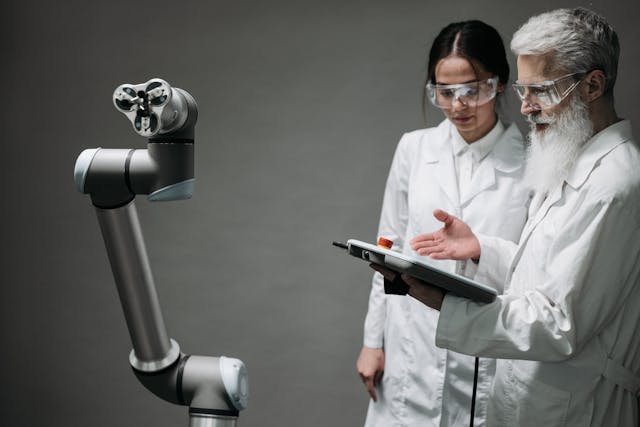
Data scientists process, analyze, and extract insights from massive volumes of data using a range of cloud-based tools and platforms. A popular platform is Amazon Web Services (AWS), which provides services like data warehousing's Amazon Redshift and machine learning model building's Amazon SageMaker. Scalable data processing and analytics technologies from Microsoft Azure include Azure Machine Learning Studio and Azure Databricks. BigQuery and AI Platform are available on Google Cloud Platform for data processing and querying, respectively, and machine learning tasks. Data scientists also frequently choose tools like TensorFlow, Hadoop, Apache Spark, and Jupyter notebooks for different phases of the cloud-based data analysis process. Data scientists can work more effectively with large data sets and intricate algorithms to extract insightful information thanks to these powerful tools and platforms.
8. Security Considerations:
Security issues are critical when it comes to cloud computing and data scientists, particularly when working with sensitive data. To protect sensitive data from possible breaches, unauthorized access, or data loss, cloud data security is essential. Strong security measures, including access restriction, encryption, and frequent audits, are necessary for data scientists to put in place in order to guarantee the integrity and confidentiality of the data they handle. Neglecting data security can have serious repercussions, such as breaking regulations, harming a company's brand, and costing them money. Acknowledging the significance of cloud data security, data scientists may efficiently reduce risks and safeguard confidential data from online attacks.
9. Training and Skill Development:
In order to improve their knowledge of cloud computing, prospective data scientists may find it helpful to enroll in online tutorials and courses. Courses on cloud computing, ranging from fundamentals to advanced topics, can be found on platforms such as Coursera, Udemy, and edX. Practical experience gained from working on projects on websites like GitHub or Kaggle can be quite beneficial. Joining coding communities or collaborating with industry experts on sites like Stack Overflow can help you obtain practical advice and insights. Pursuing certifications such as AWS Certified Machine Learning-Specialty or Google Professional Data Engineer might add legitimacy to your skill set. Attending conferences, webinars, and workshops on a regular basis can also help you stay current on the newest developments in cloud computing technology for data science applications.
10. Collaborative Opportunities:
A possible path for innovation and expansion in the data science profession is collaboration between cloud service providers and data scientists. When data scientists collaborate, they can take advantage of the sophisticated tools and scalable infrastructure that cloud platforms provide, which helps them analyze big datasets more quickly and derive insightful information. Cloud service providers can provide customized solutions to meet certain industry difficulties by leveraging data scientists' knowledge.📣
Joint research initiatives, in which data scientists collaborate closely with cloud professionals to address challenging issues needing advanced analytic techniques and substantial computational resources, are one way to promote collaboration. These interdisciplinary teams can push the limits of data-driven decision-making and predictive modeling by integrating their distinct skill sets.
The creation of new cloud-based tools and services aimed at streamlining the data science workflow is another area where collaboration opportunities exist. In order to enable cloud service providers better customize their solutions to fit the demands of data professionals working in a variety of domains, including banking, healthcare, and e-commerce, data scientists can offer insightful feedback on usability and functionality.
To sum up what I mentioned, the key to fostering innovation and realizing the full potential of big data analytics is to investigate cooperation prospects between cloud service providers and data scientists. Together, these two teams may leverage cutting-edge technologies to address challenging issues, find hidden patterns in data, and eventually propel corporate success in a digital environment that is changing quickly.
11. Best Practices:
To maximize effectiveness and performance, data scientists must follow best practices while utilizing cloud computing resources. The following recommendations will help to strengthen the current relationship between data scientists and cloud computing:
1. **Right-sizing Resources**: Tailor your cloud resources to match the workload's requirements. Avoid over-provisioning to prevent unnecessary costs.
2. **Automation**: Use automation tools like scripts or configuration management systems to streamline repetitive tasks, ensuring consistency and efficiency across your processes.
3. **Data Encryption**: Implement strong encryption methods to secure sensitive data both in transit and at rest in the cloud environment.
4. **Cost Monitoring**: Regularly monitor your cloud expenses and utilize cost management tools to optimize spending based on usage patterns.
5. **Scalability**: Leverage the scalability of cloud services to adjust resource allocation based on workload demands, ensuring optimal performance during peak times.
6. **Backup and Recovery**: Establish robust backup and recovery strategies to safeguard against data loss or system failures, utilizing cloud storage options for resilience.
7. **Collaboration Tools**: Utilize collaborative tools available in the cloud for seamless teamwork among data scientists, enabling efficient sharing of insights and results.
8. **Security Policies**: Define clear security policies tailored to your organization's needs, incorporating best practices for access control, identity management, and compliance standards.
9. **Performance Monitoring**: Implement tools for real-time monitoring of system performance, resource utilization, and application behavior to identify bottlenecks or areas of improvement promptly.
10. **Continuous Learning**: Stay updated with emerging trends and advancements in cloud technologies relevant to data science through continuous learning and upskilling initiatives.
Data scientists may drive innovation and insights in their projects by utilizing these best practices to fully utilize cloud computing resources for their analytical workflows in an efficient and effective manner.
12. Conclusion:
We can infer from the foregoing that cloud computing and data scientists have a symbiotic relationship that is still developing and growing. Scalable infrastructure, data analysis tools, and storage capacities are all made possible by cloud computing, which is essential for data scientists managing massive amounts of data effectively. This frees data scientists from the burden of maintaining infrastructure so they may concentrate on drawing conclusions and adding value from the data.
Data scientists can quickly and affordably experiment with various algorithms, models, and datasets thanks to the flexibility and agility provided by cloud platforms. By giving data science teams a centralized platform to share code, findings, and best practices, the cloud also promotes teamwork.
As technology in both cloud computing and data science evolve, this synergy is only projected to grow stronger. Through data-driven insights, data scientists will continue to innovate and propel corporate success by utilizing the cloud's potential. Organizations may remain competitive in the rapidly evolving digital ecosystem of today by adopting this continuing relationship between cloud computing and data science.