1. Introduction:
Big data is a key component of the digital age that is revolutionizing industries and influencing decision-making. Large complicated data collections that need sophisticated analytics to find patterns, trends, and insights are referred to as "big data." Although the term "big data" implies a large amount of data, it may be deceptive because big data refers to enormous volumes of information rather than physical size. This begs an intriguing question: Given its virtual character, why is large data not referred to as small data? Let's investigate this linguistic disparity and learn more about its underlying causes.👋
2. Understanding Big Data:
Big data is the term used to describe extraordinarily vast and intricate data sets that are too big and complex to handle efficiently using conventional data processing tools. Big data is defined by four primary features: volume (the total amount of data), variety (the types of data), velocity (the rate at which the data is created and processed), and veracity (the precision and dependability of the data).
This is different in a few ways from conventional datasets. While traditional datasets are often structured and kept in databases, big data typically consists of unstructured or semi-structured material including text, photos, videos, and social media posts. While traditional datasets may frequently be managed using more conventional methods, the sheer volume of big data necessitates the use of specialist tools and technologies like distributed computing and cloud storage for storage, processing, and analysis.
Big data is produced at astonishing rates by a variety of sources, including mobile devices, social networking sites, sensors, and more. To obtain significant insights from this fast-moving stream of data, analysis must be done in real-time or very close to it. Traditional datasets, on the other hand, are typically static or updated on a regular basis.
Big data's veracity component emphasizes how difficult it is to guarantee accurate and trustworthy information within these enormous datasets. Conventional datasets frequently feature pre-established schemas that support the preservation of information consistency and quality assurance. On the other hand, because of its many sources and formats, big data could not have well-defined structures or standards, necessitating the use of sophisticated validation and cleaning methods to provide reliability.
3. Historical Context:
The phrase "big data" began to acquire popularity in the early 2000s, coinciding with a dramatic change in data analysis and administration. Traditional small-scale datasets were replaced in this era by the handling of huge volumes of data, which presented both new opportunities and challenges for businesses and researchers. Our ability to gather, store, and analyze massive volumes of data increased with technology, necessitating the development of specialized tools and methods to draw conclusions from this flood of data.
The introduction of cloud computing and Hadoop technologies allowed enterprises to store and handle data on a never-before-seen scale. To characterize this paradigm shift toward handling datasets so large and intricate that conventional techniques proved insufficient, the phrase "big data" was coined. This change not only required new infrastructure, but it also altered how we viewed innovation, decision-making, and problem-solving in a variety of industries.
The term "big data"'s historical background highlights a pivotal time when the emphasis changed from controlling scarcity to utilizing excess. Businesses started using advanced analytics techniques like machine learning and artificial intelligence to extract meaningful insights as they realized the value hidden within their data troves. Predictive analytics, tailored recommendations, focused marketing campaigns, and other applications that have completely changed the way businesses function in the digital age were made possible by this evolution.
4. Scale Comparison:
Scale is a critical factor to take into account when comparing huge data to tiny data. Usually, little data involves datasets that are simple to handle with conventional data processing methods. These datasets are really straightforward and only need a little amount of processing and storage power. Big data, on the other hand, deals with enormous amounts of data that are more than standard databases can handle.
Let's look at an example to show this disparity in scope: examining client comments for a nearby retailer. In this case, small data would be a few hundred feedback entries that might be examined with an Excel or similar program. The dataset is fairly manageable and requires little computing work to extract insights from.
However, the volume and complexity increase dramatically if we scale up to big data for a worldwide e-commerce platform that analyzes millions of transactions per day across user behavior. To process this volume of data efficiently, sophisticated analytics tools and methods including distributed computing systems and machine learning algorithms are needed.
When we compare the resources required to process big data and tiny data efficiently, we can see the significant differences between the two types of data. Big data requires distributed systems like Hadoop or Spark to be deployed over numerous servers in order to manage the sheer volume and velocity of the data generated, whereas small data may be examined on a single workstation with limited resources.
5. Perception and Marketing:
Our perception of ideas like big data is heavily influenced by perception. The phrase "big data" connotes enormous amounts of information with a wealth of possible insights, and it also naturally conveys an air of importance and creativity. This label is associated with advanced technology and analytics capabilities, drawing interest from businesses that want to use it to make informed decisions.
However, there could be a number of reasons why the phrase "small data" hasn't taken off as much. To begin with, it's not as grandiose as "big data." The term "small" may unintentionally minimize its importance, causing some people to undervalue its contribution to decision-making. Big data has been the main marketing emphasis, and businesses have been investing in big data analytics tools and technologies.
Big data's attraction is its capacity to process enormous amounts of data quickly from a variety of sources. Numerous sectors are drawn to this scalability and complexity as they seek to utilize the abundance of data for possibilities and insights. On the other hand, datasets that are easier for people or smaller businesses to handle in terms of size and breadth are typically referred to as tiny data.
Taking into account everything mentioned above, we can say that even if big data has dominated conversations about analytics and technological innovation, it makes sense to acknowledge the significance of tiny data. Both types of data are essential to decision-making processes, and depending on the situation and goals at hand, each offers a special set of benefits. Organizations can more successfully customize their analytical processes to get relevant insights by recognizing the importance of both large and small datasets.
6. Technological Advances:
Big data and tiny data are distinguished mostly by technological advancements. One of the key characteristics of big data is its capacity for efficient processing of enormous volumes of information. This ability has been made possible by significant improvements in processing power, which have made it possible to analyze enormous datasets that were previously insurmountable.
These advances in technology have opened the door for the discipline of big data analytics, which uses advanced tools and algorithms to mine vast and complicated datasets for insightful information. Big data analytics' ascent has transformed numerous businesses by offering fresh viewpoints on consumer behavior, industry trends, and operational effectiveness.
Essentially, big data thrives on cutting-edge technology that can process and analyze enormous volumes of information quickly and effectively, whereas small data depends on conventional data processing techniques appropriate for manageable datasets. This distinction highlights how technology advancements have influenced the development of data analytics and the potential they provide for growth and innovation across a range of industries.
7. Industry Applications:
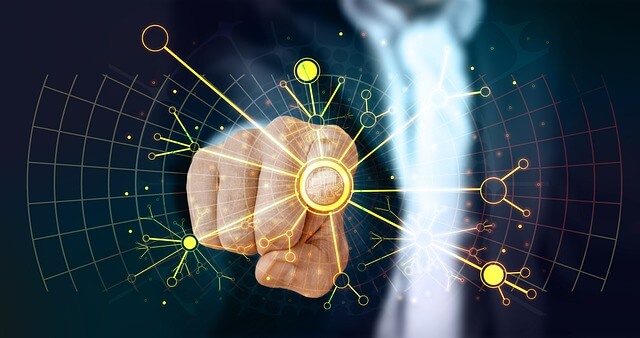
Big data is essential for producing insights, facilitating educated decision-making, and stimulating innovation in a variety of industries, including retail, healthcare, finance, and more. Large volumes of consumer data are analyzed by retailers in order to improve customer experiences and tailor marketing campaigns. Big data is used by healthcare practitioners to enhance patient outcomes via personalized treatment and predictive analytics. Big data is used by financial firms for algorithmic trading, risk assessment, and fraud detection.
When comparing the effects of managing small datasets to solving big data problems, it becomes clear how much complexity and scale change. Although small datasets can be handled using conventional tools and techniques, their size limits limit the insights they can provide. On the other hand, scalability problems with huge data need the use of cutting-edge technology for analysis, like machine learning techniques and cloud computing. Big data gives firms the ability to make strategic decisions based on thorough analytics rather than tiny samples by exposing patterns and trends that were previously unreachable with smaller datasets.
8. Semantic Considerations:
The preference for "Big Data" versus "Small Data" can be understood through an analysis of the semantics of naming conventions in technology and analytics. The immense volume, velocity, and variety of data being generated today led to the coining of the phrase "Big Data". It illustrates the difficulties and possibilities involved in managing such big databases.
If the industry had chosen "Small data" instead, it might have suggested a concentration on datasets that are easier to handle or less complicated. This might have caused one to undervalue the possibility of using smaller-scale data sources to get meaningful insights. The phrase "Big Data" drives firms to build sophisticated analytics capabilities by serving as a reminder of the scope and complexity of today's data landscapes.
We explore how linguistic decisions can influence our knowledge and approach to technologies by examining if labeling it "Small Data" would have changed perceptions. "Small Data" may have focused on specificity or simplicity, but "Big Data" prioritizes volume and complexity. This, however, might have minimized the importance of even small datasets in influencing corporate choices or stimulating innovation.
Beyond simple labels, the language employed in technology domains such as big data analytics matters in terms of how we conceptualize and apply data-driven insights to traverse the increasingly data-rich surroundings of today. The choice to label it as "Big Data," with its associations of complexity and scale, emphasizes the revolutionary power that these enormous databases can have when properly examined and understood.
9. Cognitive Bias and Perception:
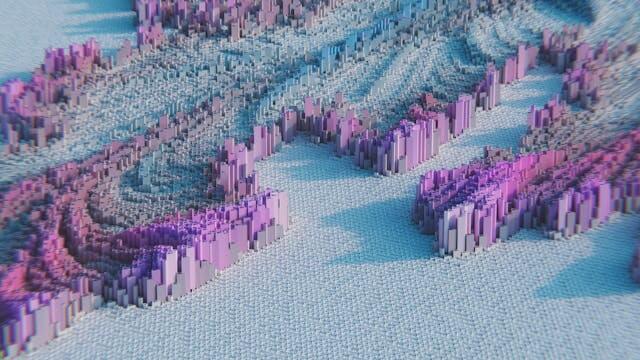
Our tendency to assign more significance to larger amounts, such big data, than to smaller data is mostly due to cognitive biases. The "anchoring bias," in which the magnitude of huge data—the initial piece of information encountered—becomes the comparison's anchor, making lesser datasets appear less meaningful in light of the larger dataset.
The way we identify and understand various data sizes is also greatly influenced by human perception. Because of the way that our minds are wired, greater quantities frequently suggest greater value or significance. This intrinsic bias affects both the way we label and understand the data. Simply because it connotes grandeur and size and fits nicely with our preconceived views of worth and influence, the word "big data" itself has significant weight.
When it comes to data, terminology plays a multifaceted role in influencing attitudes and guiding conversations in addition to providing precise descriptions of information. The terminology we use, such as big data versus tiny data, can unintentionally affect how we prioritize and handle various datasets. Regardless of the volume of the data, we can aim for more impartial evaluations by being aware of the cognitive biases at work and how perception affects our opinions.
10. Evolution of Data Terminology:
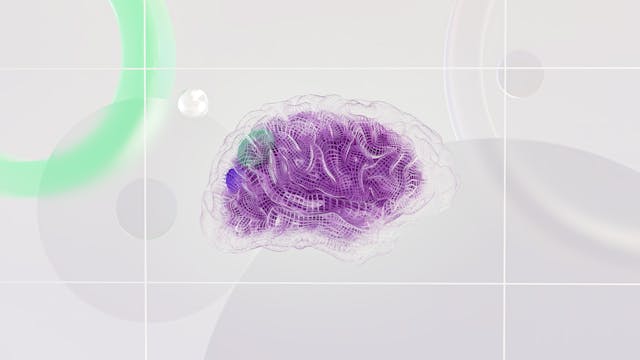
The jargon used in the data sector has changed throughout time to reflect the complexity and expansion of data. The transition from "small data" to "big data" represents this change. As technology developed, so did our capacity to gather, store, and process previously unthinkable volumes of data.
When datasets were smaller and easier to handle, the term "small data" was appropriate. However, we reached a time when conventional approaches were inadequate to handle the enormous volume of information generated every day due to the development of powerful computing systems and storage technologies.
To reflect the diversity of data sizes we face today, other terminology that might more accurately capture different dataset scales could be investigated. Phrases such as "rich data," "dense data," or even classifications like "tiered data" according to volume and complexity could offer a more sophisticated perspective on the various dataset scales that are accessible.
The intricacies of data and how they affect our analytical and decision-making processes can be more effectively communicated if we regularly review and modify the language we use to describe it. The rapid improvements in technology that drive us to explore and use data in ever-new ways are reflected in the growth of language.
11. Environmental Impact:
One important but frequently disregarded component of big data is its environmental impact. Processing large datasets requires a significant amount of energy compared to smaller datasets. Environmental issues are exacerbated by the bigger carbon footprints that result from this increased energy consumption. The demand for sustainable big data handling strategies grows as individuals and organizations struggle to manage ever-increasing volumes of information.
Examining the energy usage associated with handling large datasets highlights a significant difference from smaller datasets. Big data processing necessitates sophisticated algorithms and enormous storage capabilities, which dramatically increase energy consumption. Due to rising carbon emissions, this increase in energy consumption not only raises operating expenses but also presents environmental problems. In today's digital world, it is crucial to comprehend and minimize the environmental effects of processing large amounts of data.
The growing amount of data that businesses manage creates questions about big data operations' sustainability. The continual energy consumption that comes with storing, processing, and maintaining large databases exacerbates the environmental effects. Businesses must address the environmental costs of their efforts as they attempt to extract the insights hidden within massive data sets. Through the implementation of environmentally conscious tactics and efficient resource management, businesses may address the sustainability issues brought about by the rapid expansion of big data.
The growing environmental impact of large data processing operations calls for a paradigm change toward sustainable methods. Handling massive datasets has a negative environmental impact that can be mitigated by investing in renewable energy sources, implementing energy-efficient technologies, and optimizing workloads to use less resources. Educating stakeholders about sustainable data management techniques encourages a culture of accountability for reducing the environmental harm that big data operations cause.
It is imperative that we prioritize sustainability in tandem with technical breakthroughs as we traverse the complex ecosystem of big data analytics to secure a more environmentally friendly future. We create a more sustainable digital landscape by using ecologically responsible methods while processing and organizing massive amounts of data. We can exploit the power of big data while preserving our world for future generations by working together to cut energy use and adopt eco-friendly practices.♌️
12. The Future Outlook:
Looking ahead, automation, AI-driven decision-making, and more efficient procedures for managing massive volumes of data seem to be the main focuses of data management in the future. We may anticipate that advances in machine learning algorithms and predictive analytics will become increasingly important as big data continues to grow in quantity and complexity. This change is probably going to result in the creation of even more advanced data processing and interpretation technologies.
Regarding the evolution of terminology, we may witness a convergence towards a more cohesive method of characterizing datasets by their unique features instead of their sheer volume. When data quality and relevance become more important than just quantity, ideas like "smart data" and "context-aware data" may become more popular.
Since technology is always changing, it is reasonable to believe that new paradigms will also appear to deal with the ever-growing field of digital information analysis and storage. The development of edge computing and decentralized data processing, which would enable quicker access to real-time insights without an undue dependence on centralized servers, is one possible path. An additional potential is a greater focus on the moral issues related to data gathering and use, which could result in frameworks that provide privacy and consent top priority when utilizing big data analytics.