1. Introduction
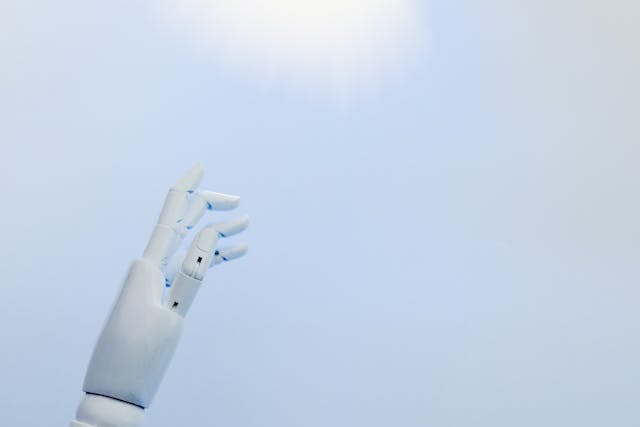
Insurance businesses are increasingly using insurance data analytics to obtain a competitive edge in the market in today's data-driven environment. In order to glean insightful information, insurance data analytics entails gathering, analyzing, and interpreting massive amounts of data produced within the insurance industry. Through the utilisation of sophisticated analytics tools and technology, insurers may enhance customer experiences, reduce risks, increase operational efficiency, make better informed decisions, and stimulate business growth.
It is impossible to exaggerate the significance of using data analytics in the insurance sector. Insurers now have access to a plethora of untapped data due to the exponential increase of data sources, which range from telematics and Internet of Things devices to policyholder information and claims data. Insurers can find hidden patterns, trends, and correlations in this data by using advanced analytical techniques like machine learning, artificial intelligence, and predictive modeling. These techniques can help insurers detect fraud more effectively, assess risk more accurately, understand customer behavior better, optimize pricing strategies, and personalize products and services.
In an increasingly dynamic environment, insurers may remain ahead of the competition by implementing efficient insurance data analytics strategies that convert raw data into actionable insights. Companies that use data analytics to their full potential will have a major competitive advantage in today's fiercely competitive insurance market. These advantages will include faster decision-making, increased underwriting accuracy and efficiency, improved customer engagement and retention rates, and ultimately profitable and long-term growth.
2. The Role of Data Analytics in Insurance
In the insurance industry, data analytics is altering the way insurers evaluate risk, create policies, spot fraud, and improve consumer satisfaction. Through the utilization of data analytics tools and technology, insurance businesses may swiftly and efficiently make well-informed decisions by extracting important insights from massive data sets. By using these insights, insurers can create more individualized products and services by having a deeper understanding of the needs, interests, and behaviors of their clientele.
For insurers, there are many advantages to using data analytics. First, by examining past data patterns and trends to more precisely forecast future hazards, data analytics enables insurers to enhance risk assessment. This guarantees that insurers manage risks well and helps them price their products more competitively. Insurance companies may stop losses and detect possible fraud instances early on by employing sophisticated analytics tools like predictive modeling and machine learning.
By automating manual procedures, cutting paperwork, and expediting the claims processing process, data analytics improves operational efficiency in the insurance industry. By enabling quicker responses and settlements, this not only saves time but also enhances the general customer experience. By utilizing data analytics insights to generate consumer segmentation analysis, insurers can enhance their marketing strategy by implementing focused campaigns.
By adopting data analytics, insurance businesses can make data-driven decisions that enhance risk management, operational effectiveness, customer satisfaction, and profitability, helping them to remain competitive in a sector that is changing quickly.
3. Challenges in Implementing Insurance Data Analytics
Insurance companies must overcome a number of obstacles in order to successfully implement insurance data analytics. The volume and complexity of data that insurers handle is a frequent challenge, therefore having reliable systems and infrastructure in place is crucial to efficiently processing and analyzing this enormous quantity of data. A major problem is integrating several data sources from external and internal channels while maintaining data accuracy and quality.
The culture transformation that insurance businesses must undergo in order to adopt a data-driven approach presents another difficulty. Employees need to be educated on the advantages of data analytics through training programs and good communication because they frequently oppose changing from traditional ways. When adopting data analytics efforts, insurers face additional complexity due to regulatory compliance and concerns about data privacy and security.
Insurers have a few options for addressing these issues. Investing in cutting-edge technologies like artificial intelligence (AI) and machine learning can boost productivity, automate tasks, and improve decision-making based on real-time insights. Aligning data analytics activities with business goals requires a well-defined data strategy that includes processes, targets, and key performance indicators.
By working together with outside partners like insurtech firms or data analytics specialists, insurers can acquire the specific skills and resources they need to successfully manage challenging analytical assignments. Employees can better grasp the importance of data analytics in promoting corporate growth and competitiveness by participating in training programs and seminars designed to create a culture of data literacy inside the company.
In an increasingly dynamic industry context, insurers may leverage the potential of insurance data analytics to achieve a competitive edge by proactively addressing these concerns and putting appropriate solutions in place.
4. Types of Data Used in Insurance Analytics
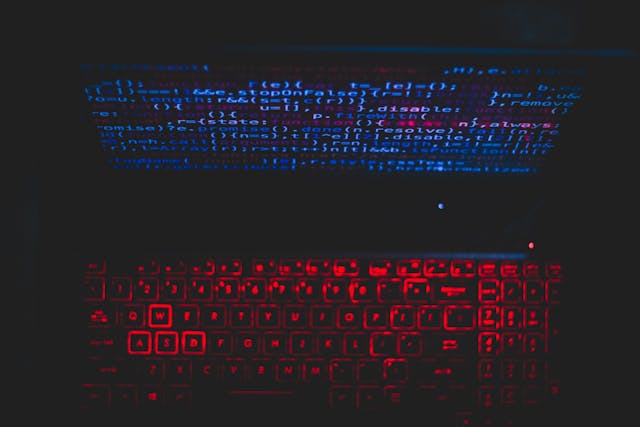
Different kinds of data are essential in insurance analytics for forming business plans and obtaining a competitive advantage in the sector. Big data, unstructured data, and structured data are some of these data kinds.
The term "structured data" describes information that has been arranged in a way that makes it easy for computers to process it. Financial information, claim histories, and policyholder details are usually included. Insurers can precisely analyze risk, optimize processes, and customize client experiences by analyzing structured data.🤓
Non-standardized information from sources like social media posts, emails, contact center transcripts, and photos is referred to as unstructured data. Through the use of text mining, sentiment analysis, and natural language processing techniques, insurers can gain useful insights to improve customer service, identify fraud patterns, and forecast market trends by utilizing unstructured data.
Large amounts of both organized and unstructured data are involved in big data, necessitating the use of sophisticated analytics techniques like predictive modeling and machine learning algorithms. Insurance businesses may better predict future losses, enhance pricing tactics, and create cutting-edge solutions that meet changing customer expectations by utilizing big data analytics.
In today's dynamic market scenario, insurers can remain ahead of the competition, manage risks proactively, and make informed choices more quickly by utilizing a comprehensive approach that integrates structured, unstructured, and big data analytics.
5. Applications of Data Analytics in Insurance
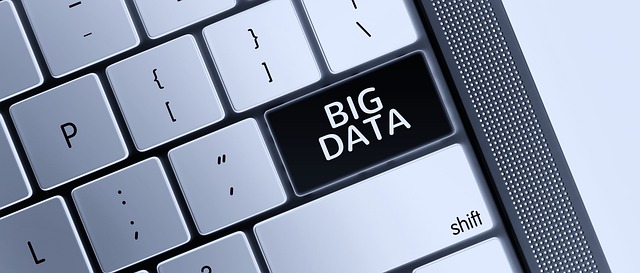
Data analytics is essential to helping businesses in the insurance sector gain a competitive edge. Analytics are used by insurers to enhance their risk assessment and pricing methods. Through the examination of extensive datasets, including past claims, demographic data, and market trends, insurance companies are better equipped to forecast the risks involved in providing coverage for specific individuals or assets. Insurance companies are able to provide more precisely priced insurance because to this greater understanding, which lowers possible losses and boosts profitability.
Fraud detection is a crucial use of data analytics in the insurance industry. The use of sophisticated analytics tools by insurers, such as anomaly detection and predictive modeling, allows them to spot unusual trends that might point to fraud. By taking a proactive stance, insurance companies can reduce the losses they incur from fraudulent claims and safeguard honest consumers from higher premiums brought on by fraudulent activity.
Insurers can improve their consumer segmentation efforts with the use of data analytics. Insurance businesses can design tailored offers and marketing campaigns by evaluating client data, including demographics, preferences, and habits. Insurance companies may better serve the distinct demands of various client segments thanks to this segmentation, which raises customer satisfaction and retention rates.
Insurance businesses can make educated decisions, streamline operations, and maintain a competitive edge in a market that is constantly changing by incorporating data analytics into several facets of their business operations.
6. Tools and Technologies for Insurance Data Analytics
Using cutting-edge tools and technologies for data analytics is crucial to gaining a competitive edge in the ever-changing insurance market. Insurance data analysis involves the use of a variety of sophisticated tools, including business intelligence platforms, Tableau and Power BI for data visualization, and predictive modeling software. With the aid of these technologies, insurers can now make well-informed choices based on thorough analyses drawn from enormous volumes of data.
Insurance businesses can improve risk assessment, expedite underwriting, and customize client service by implementing cutting edge technologies into their operations. With the use of predictive analytics tools, insurers can proactively manage risks and avert future losses by seeing trends and patterns. Platforms for business intelligence offer real-time dashboards and reports that speed up strategic planning and decision-making. By providing interactive representations of complicated data sets, data visualization technologies facilitate stakeholder understanding of important performance measures and trends.
There are several benefits to incorporating cutting-edge technology into insurance operations. First off, by thoroughly examining a variety of risk variables, these tools enable insurers to increase pricing precision. This helps in the design of products that are specifically designed to meet the demands of customers and results in more accurate underwriting choices. Second, with anomaly detection algorithms and machine learning models, insurers can quickly identify fraudulent activity thanks to improved data analytics capabilities. Businesses can drastically lower financial losses by quickly identifying false claims.
By using targeted marketing techniques and individualized services, insurance data analytics using cutting edge technology improves the client experience. Insurance companies are able to recognize consumer preferences, anticipate needs, and provide customized solutions in a proactive manner by applying predictive modeling and segmentation approaches. This builds enduring relationships with policyholders while also raising client satisfaction. By automating repetitive duties like processing claims or renewing policies, the use of cutting-edge technologies simplifies operations inside insurance companies.
Furthermore, as I mentioned above, using cutting-edge techniques and technology for insurance data analytics is essential to being competitive in the market. Insurance companies may increase operational effectiveness, successfully reduce risk, and provide better customer experiences by utilizing predictive modeling software, business intelligence platforms, and data visualization tools. Modern technology not only streamlines internal operations but also opens up new avenues for innovation and expansion in the insurance industry.🤴
7. Case Studies: Successful Implementation of Insurance Data Analytics
Case Studies: Successful Implementation of Insurance Data Analytics
Insightful demonstrations of real-world applications provide insurers with a competitive advantage in the market. Company X is one example of this, as it used predictive analytics to more precisely evaluate policyholder risk profiles. They may customize insurance policies to meet specific needs by examining past data and trends, which would raise consumer satisfaction and retention rates.
Company Y is another noteworthy case study that uses data analytics to detect fraud. They were able to minimize potential costs from fraudulent activity and quickly identify suspicious claims by using sophisticated algorithms and machine learning models. By taking a proactive stance, the business not only protected its profits but also improved its standing with customers.
These excellent case studies offer valuable lessons that highlight the need of making a substantial investment in data analytics skills. In order to stay ahead of the competition in a fiercely competitive market, insurers can no longer afford to rely only on conventional approaches. Instead, they must embrace technology innovations. Having a thorough understanding of data management and interpretation is essential to making profitable and growth-oriented business decisions. Insurance firms may effectively handle digital transformation and maintain their competitive advantage in a constantly changing market by taking a cue from these examples.
8. Regulatory Considerations in Insurance Data Analytics
Comprehending and respecting regulatory standards is essential when utilizing insurance data analytics. A complicated network of laws that control data collecting, storage, and analysis must be negotiated by insurance companies. These rules are in place to preserve industry standards, guarantee fair pricing and underwriting procedures, and safeguard consumer privacy. There are serious repercussions for breaking these rules, including fines, legal action, and reputational harm.
The insurance industry's use of analytical strategies is greatly impacted by regulations. Data analytics frequently entails handling enormous volumes of private policyholder data. As a result, insurers need to pay close attention to data security policies, consent procedures for using data, and analytic process openness. One of the biggest challenges facing insurance businesses operating in highly regulated environments is finding a balance between using advanced analytics to get business insights and protecting the privacy of consumer data.
Summarizing the above, we may conclude that regulatory concerns play a crucial role in influencing how insurance businesses utilize the power of data analytics to obtain a competitive edge. Insurers can reduce risks and gain regulator and consumer trust by anticipating regulatory compliance needs and incorporating them into their analytic strategies. In order to prosper in an increasingly data-driven market, insurers will need to adopt a culture of ethical data use and compliance.
9. Future Trends in Insurance Data Analytics
Data analytics is essential for guiding strategic decision-making and obtaining a competitive edge in the ever-changing insurance market. A number of significant themes are anticipated to influence how insurance data analytics develop in the future.
The growing use of machine learning and artificial intelligence (AI) is one possible development in the near future. These innovations have the potential to completely change the way insurers handle and evaluate massive volumes of data, resulting in more precise risk evaluations, customized pricing schemes, and improved fraud detection capabilities.
It is expected that predictive analytics will advance even further, giving insurers more agility and precision in trend forecasting. Businesses can proactively detect emerging hazards, enhance customer experiences, and streamline operational efficiencies by utilizing real-time data sources and sophisticated modeling approaches.
Insurance companies will need to make investments in reliable data management systems and analytical tools as data sources continue to diversify beyond conventional structured data sets to incorporate unstructured data from social media, Internet of Things devices, and other sources. For insurers hoping to glean valuable insights and spur innovation in their offerings, this flood of varied data kinds offers both obstacles and possibilities.
In the future, data analytics and cutting-edge technologies like blockchain and quantum computing should intersect, creating new opportunities for safe data exchange, expedited claims processing, and sophisticated risk analysis. In an increasingly competitive market setting, insurers may maintain a competitive edge by adopting these state-of-the-art tools and practices.
Based on the aforementioned information, we can infer that insurance data analytics has a bright future ahead of it for those in the business who are prepared to accept change and make use of cutting-edge tools. Through agility, proactivity, and constant adjustment of their analytical approaches to changing market conditions, insurers may not only prosper in the current digital era but also lead the way toward a more robust and customer-focused insurance ecosystem.
10. Risk Management through Predictive Modeling
In today's ever-changing insurance market, advanced algorithms that enable predictive modeling are essential for helping insurers manage risks. Insurance companies may more accurately predict future hazards by using both historical and current data, which makes proactive risk management techniques possible. When evaluating and managing risks, insurers may make better decisions by using these sophisticated analytical tools to find patterns, trends, and anomalies in their data.🔷
With machine learning algorithms, insurers can use predictive modeling to improve their risk management procedures by quickly and reliably analyzing large amounts of data. This makes it possible for insurers to pinpoint high-risk persons or locations that need extra care, resulting in a more focused approach to risk reduction. Insurers can more effectively manage resources and establish suitable pricing strategies to minimize financial losses by forecasting the frequency and severity of possible claims.🖋
Insurance companies can enhance their fraud detection skills by using predictive modeling to spot suspicious activity or out-of-the-ordinary tendencies. Insurers can avoid financial losses and protect the integrity of their business by identifying possibly fraudulent claims early on by using anomaly detection algorithms. Predictive models can help insurers proactively modify their risk management strategies by helping to spot emerging hazards based on shifting market dynamics or client habits.
Predictive modeling using sophisticated algorithms, in short, gives insurers the ability to stay ahead of risks by offering insightful information gleaned from data analysis. Insurers can improve fraud detection systems, strengthen risk management procedures, allocate resources more efficiently, and gain a competitive advantage in an ever-complex insurance market by adopting these cutting-edge technology.
11. Customer Experience Enhancement through Personalized Offerings
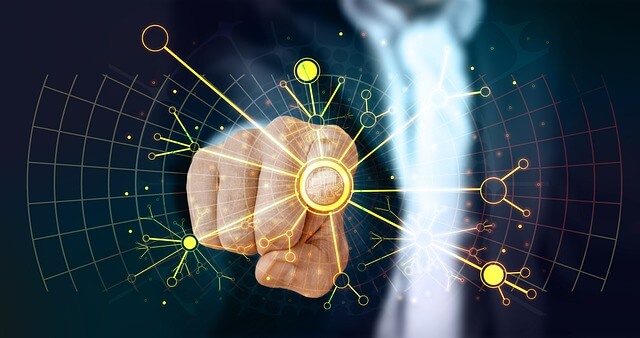
Remaining competitive in the ever-changing insurance market requires utilizing data analytics to improve client experience. Insurance firms may greatly increase overall customer satisfaction by customizing products and services based on consumer profiling.
The field of auto insurance is one interesting use case that highlights the value of tailored offerings. Insurance companies can examine specific driving patterns recorded by telematics devices using sophisticated data analytics. Insurers not only promote safer driving practices but also strengthen their bonds with their clients by using this data to tailor insurance policies that reward safe drivers with reduced premiums or extra incentives.
Health insurance is another area where tailored offerings have shown to be successful. The use of data analytics by insurers to evaluate a policyholder's health risks and preferences allows them to customize coverage packages and wellness initiatives to match individual needs. By offering individualized care, this proactive strategy not only increases customer happiness but also reduces the likelihood of expensive claims by encouraging healthier lifestyle choices.
Insurance firms can get a competitive advantage in the market by adopting individualized products based on consumer profile. This will strengthen client loyalty, boost retention rates, and eventually increase profitability through better customer satisfaction metrics.
12. Conclusion:
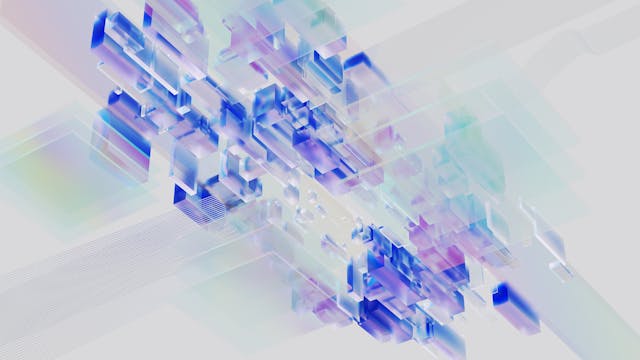
This blog post has demonstrated the critical role that insurance data analytics plays in obtaining a competitive edge in the market, as I mentioned above. We looked at how data analytics may help businesses expand by streamlining operations, enhancing risk assessment, and improving customer satisfaction. Insurance firms can make smart judgments, automate procedures, and outperform rivals by utilizing advanced analytics tools and methodologies.
In order to prosper in the data-driven world of today, insurers must embrace insurance data analytics. Large databases can be mined for insightful information, patterns and trends may be found, future outcomes can be predicted, and consumer offerings can be made more tailored. Through the utilization of data, insurance companies can enhance their pricing tactics, more efficiently reduce risks, and develop customized solutions that cater to changing customer demands.
To put it simply, insurers must invest in insurance data analytics if they hope to succeed and survive in a fiercely competitive industry. Adopting data analytics can provide a company a strategic edge by opening up new avenues, increasing operational effectiveness, and ultimately providing more value to stakeholders and customers. The insurance sector will thrive in the future if it can use data to innovate, set itself apart, and pave the path for a more customer-focused and flexible approach.