1. The Importance of Big Data in Fraud Detection
Using big data is crucial when it comes to risk management and fraud detection. The sheer amount, speed, and diversity of data produced in today's digital environment offer a wealth of insightful information that can be used to successfully identify fraudulent activity. Organizations can use big data analytics to sort through enormous volumes of both structured and unstructured data in order to spot trends, abnormalities, and patterns that could point to fraud.
Through the examination of a variety of data sources, including transaction records, user behavior patterns, geolocation data, social media activity, and more, companies are able to generate thorough profiles of authentic users and identify any discrepancies that may indicate fraudulent or suspect conduct. Proactive fraud prevention strategies and more accurate risk assessment are made possible by this holistic viewpoint.
Big data platforms' real-time processing powers enable businesses to stop fraud before it starts or even identify it when it has already occurred. organizations may keep one step ahead of fraudsters and efficiently tackle growing threats by utilizing advanced analytics techniques such as machine learning algorithms, artificial intelligence, and predictive modeling on large data sets. These techniques allow organizations to change their risk management strategies dynamically.
2. Understanding the Role of Machine Learning in Risk Management
Machine learning is essential to risk management because it makes it possible to analyze large datasets in a sophisticated way and find patterns and anomalies that can be signs of impending danger. Machine learning can improve fraud detection by detecting anomalous transactions or behaviors that diverge from conventional patterns thanks to sophisticated algorithms. Machine learning models can detect fraudulent activity more accurately while reducing false positives that could interfere with operations by utilizing big data.
Because machine learning algorithms are able to learn and adapt continuously, risk management systems can advance and remain one step ahead of more sophisticated fraud schemes. By using real-time transaction monitoring and predictive modeling, machine learning enables firms to proactively detect and reduce hazards before they become more serious. By keeping a secure environment for transactions, this proactive strategy not only protects against financial losses but also aids in retaining client trust.
A more comprehensive understanding of hazards across numerous touchpoints within an organization is made possible by machine learning techniques like anomaly detection, clustering, and classification. Businesses can learn more about new risks and weaknesses by combining these technologies with big data analytics. This thorough understanding fortifies overall resistance against changing fraud tactics and facilitates proactive risk mitigation strategies.
Modern risk management relies heavily on machine learning, which uses big data to improve fraud detection, streamline risk assessment procedures, and strengthen defenses against possible attacks. Organizations may cultivate a security-conscious culture and confidently and nimbly navigate the complex world of financial threats by adopting these cutting-edge technology.
3. Leveraging Predictive Analytics for Advanced Fraud Detection
The use of predictive analytics transforms the field of fraud detection. Organizations may proactively detect suspect patterns and behaviors before they develop into full-blown fraud events by utilizing big data and predictive algorithms.
Businesses can use predictive analytics to examine past data and find patterns, irregularities, and any red flags that point to fraudulent activity. These insights enable companies to bolster their defenses against impending dangers by acting proactively.
Because predictive analytics constantly improves and refines detection algorithms, it enables firms to remain ahead of ever-evolving fraud schemes. Businesses may ensure a more resilient and flexible risk management strategy by utilizing machine learning and artificial intelligence (AI) technologies to instantly adjust to new fraud tendencies.
In order to stay one step ahead of fraudsters in today's increasingly digital landscape, it is imperative that predictive analytics be included into fraud detection processes, to paraphrase what I wrote. Organizations may efficiently strengthen their defenses, manage risks, and secure their assets by utilizing big data and sophisticated analytics solutions.
4. The Impact of Real-time Data Processing on Risk Mitigation
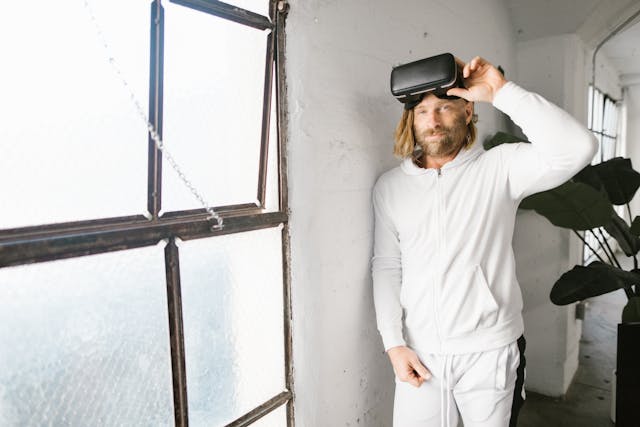
When it comes to fraud detection and risk management, real-time data processing greatly improves risk mitigation. Organizations may quickly identify anomalies and unlawful activity by examining data as it is generated. This enables prompt intervention to avert potential hazards. This quick reaction is essential to stopping fraudulent activity because it reduces the amount of time that bad actors have to take advantage of weaknesses.
Organizations can keep ahead of changing risks and quickly adjust their strategy to counter new threats by having the capacity to interpret real-time data. Businesses are better equipped to proactively manage risks when they have access to timely insights from live data sources, which enable them to make informed decisions quickly. By facilitating quick reactions to possible vulnerabilities, this agile strategy not only increases fraud detection techniques but also fortifies entire risk management frameworks.
Organizations are better able to put preventive measures in place when real-time data processing makes it easier to spot patterns and trends that can point to fraud or other hazards. Businesses can quickly identify suspicious patterns and take steps to minimize possible losses by continuously monitoring transactions and activity in real time. This proactive approach strengthens the organization's resilience in the face of unforeseen hazards in addition to providing protection against fraudulent operations.
5. Case Studies: Successful Implementation of Big Data in Fraud Prevention
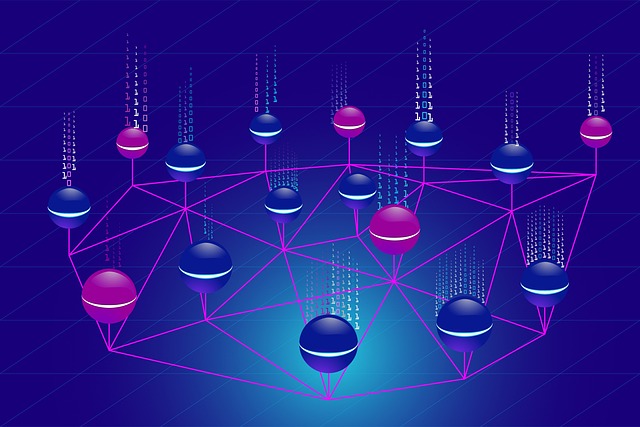
Case studies that demonstrate the effective use of big data in fraud prevention offer important insights into the real-world uses of utilizing data analytics for effective risk management. PayPal is a well-known online payment company that uses big data analytics to instantly identify and stop fraudulent transactions. Through the examination of several data points, including transaction history, customer activity patterns, and device information, PayPal is able to promptly detect and address possible fraud incidents.
American Express, a financial services firm renowned for its proactive approach to fraud detection, is the subject of another noteworthy case study. American Express analyzes millions of transactions every day using big data technologies, which helps the corporation identify odd spending trends or questionable activity that could be signs of fraud. American Express is able to promptly respond to security concerns and safeguard the financial assets of its clients thanks to this proactive monitoring.
Walmart, a massive retailer, has also effectively used big data to combat fraud. Walmart can identify unusual trends that can indicate fraudulent activity like return fraud or unlawful discounts by using sophisticated algorithms to examine a variety of data sets, including sales records, customer information, and inventory details. Walmart improves its ability to protect against financial damages resulting from fraudulent behavior by utilizing big data in this way.
The aforementioned case studies highlight the noteworthy influence that the utilization of big data may have on sophisticated fraud detection and resilient risk management tactics in various sectors. Organizations can reduce risks and protect their reputation by using big data analytics to proactively detect and combat possible attacks, therefore using the abundance of information available.
6. Enhancing Security Measures with Big Data Analytics
By enabling sophisticated fraud detection and effective risk management techniques, the use of big data analytics has completely changed security procedures. Organizations can improve their security measures by using sophisticated algorithms that evaluate massive volumes of data in real-time by using the power of big data. By taking a proactive stance, companies can stop possible fraud efforts by quickly identifying anomalies and suspicious activity.
Developing predictive models that can spot trends suggestive of fraudulent activity is a major advantage of using big data for security measures. With the help of fresh data inputs, these models are able to learn and adjust continuously, increasing in accuracy over time. Organizations can successfully minimize risks before major damage occurs by spotting abnormalities and patterns early on.
Organizations may do thorough risk assessments thanks to big data analytics, which analyzes past data, market patterns, and outside variables that could affect security flaws. This comprehensive approach offers insightful information about possible risks and aids in the proactive strategy development of firms to effectively counter them. Businesses may fortify their defenses and safeguard themselves against changing cybersecurity threats by keeping ahead of emerging hazards.
In light of everything mentioned above, we can draw the conclusion that, in the rapidly changing digital environment of today, utilizing big data for sophisticated fraud detection and strong risk management is essential. Organizations may improve their fraud detection skills, strengthen their security measures, and more effectively manage risks proactively by integrating cutting-edge technologies and analytical tools. By strategically utilizing big data analytics, businesses can demonstrate their commitment to upholding strong security standards across all activities, which not only helps protect sensitive data but also builds customer trust.💱
7. Best Practices for Utilizing Big Data for Fraud Detection
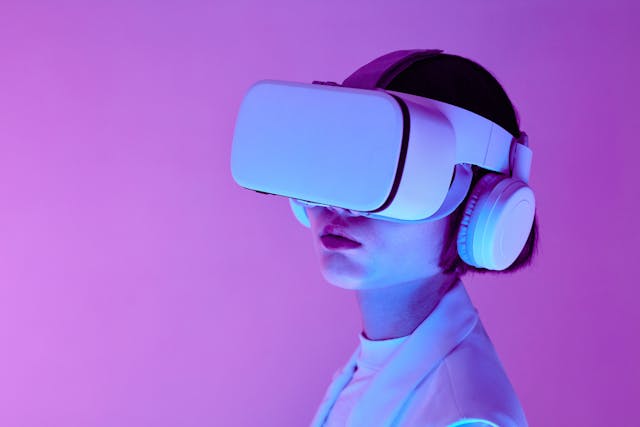
Implementing best practices when utilizing big data for fraud detection is crucial for the success and effectiveness of any system. Here are some key strategies to consider:
1. **Data Quality Assurance:** Ensure your data sources are reliable, accurate, and consistent. Implement processes for data cleansing, validation, and normalization to maintain high-quality data.
2. **Real-time Monitoring:** Leverage real-time analytics to detect anomalies and suspicious activities as they occur. This allows for quick response and mitigation of potential risks.
3. **Algorithms for Machine Learning:** Utilize sophisticated machine learning methods, such support vector machines, neural networks, or random forests, to evaluate vast amounts of data and spot trends that point to fraudulent activity.
4. **Feature Engineering:** Develop relevant features based on the unique characteristics of your data that can improve the accuracy and efficiency of fraud detection models.
5. **Behavioral Analysis:** Utilize behavioral analytics to create profiles of normal user behavior and detect deviations that may signify fraudulent activity.💡
6. **Collaboration and Integration:** Foster collaboration between teams handling fraud detection, risk management, IT security, and data analytics to ensure a holistic approach to combating fraud.
7. **Ongoing Enhancement:** To keep ahead of changing threats, assess and update your fraud detection models on a regular basis based on fresh data insights, new patterns in fraud schemes, and input from real instances.
You may improve the accuracy of detecting fraudulent activity, reduce false positives, and guarantee a strong risk management framework in your company by implementing these best practices into your big data analytics approach for fraud detection.
8. Addressing Emerging Threats through Big Data Analytics
Remaining ahead of developing risks is essential to preserving security and confidence in the fields of risk management and fraud detection. Organizations can proactively address these issues before they worsen by utilizing big data analytics. Real-time detection of abnormalities and patterns suggestive of possible dangers is possible through the utilization of sophisticated algorithms and machine learning models on extensive datasets. This makes it possible for institutions to respond quickly and efficiently in order to reduce risks and safeguard their assets.
Big data's capacity to evaluate enormous volumes of data from various sources is a key benefit when it comes to fraud detection. This all-encompassing strategy decreases false positives and improves threat detection accuracy by providing a more thorough understanding of prospective threats. Organizations can remain proactive in their risk management strategies and adjust to changing risks by regularly improving algorithms based on fresh data inputs.
Organizations can improve their prediction skills to recognize new dangers by utilizing big data analytics. Predictive models can identify possible hazards before they manifest by examining past trends and patterns in addition to the data that is now available. With this kind of foresight, businesses can effectively resist impending risks by deploying specific security measures or modifying their risk management policies, for example.
9. Integrating AI and Big Data for Proactive Risk Management
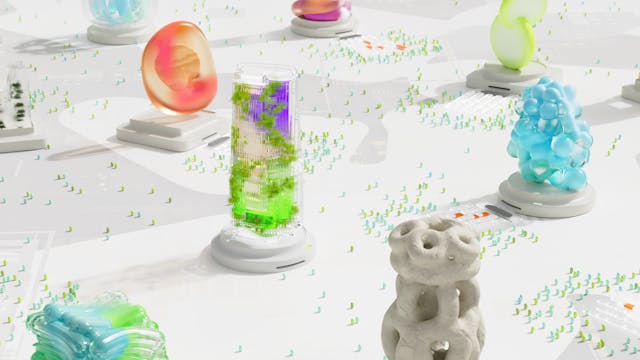
Organizations can employ proactive risk management strategies that improve fraud detection and risk reduction by integrating AI with big data. AI systems are able to identify trends and abnormalities in real-time by utilizing the massive quantity of data that is accessible. This allows for the early detection of any problems before they become more serious. By taking a proactive stance, companies may minimize the effects of fraudulent activity and keep ahead of developing threats and weaknesses.
Risk management solutions driven by AI are able to quickly identify any suspicious activity by continuously monitoring transactions, consumer behaviors, and market trends. Through the integration of AI's capacity for pattern recognition and predictive analytics with Big Data processing capability, enterprises can create resilient risk models that dynamically adjust to changing risks. Through this integration, automated decision-making processes based on data-driven insights are made possible, which speeds up response times and simplifies operations for efficiently managing fraud risks.
Organizations may generate a complete view of risk across several business areas and channels by integrating AI and Big Data. Companies can obtain a comprehensive understanding of their risk environment and more effectively discover interrelated vulnerabilities by combining data from several sources into a single platform. By improving situational awareness, this integrated strategy enables firms to proactively address specific areas of concern through the implementation of targeted mitigation solutions.
To sum up what I've written so far, companies have a revolutionary chance to improve their risk management procedures through the integration of AI and big data. Businesses may increase overall resilience against emerging threats, improve decision-making processes, and expand their fraud detection capabilities by leveraging the power of machine learning and advanced analytics on massive datasets. To succeed in proactive risk management in an increasingly digital environment and to stay ahead of the constantly changing threat landscape of today, it is imperative to embrace this synergy between AI and Big Data.
10. Challenges and Opportunities in Implementing Big Data Solutions for Fraud Detection
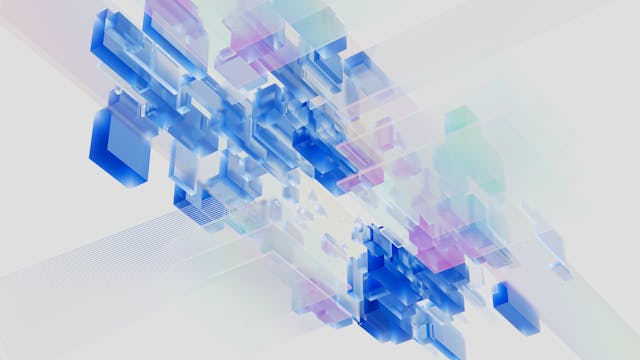
In today's dynamic corporate market, implementing big data solutions for fraud detection brings with it a number of opportunities as well as problems. Managing the enormous amount, velocity, and diversity of data produced by contemporary digital transactions is one of the main issues. In order to handle this enormous volume of data, advanced infrastructure that can process and analyze data in real-time and quickly identify irregularities and possible fraud tendencies is needed.
Making sure sensitive data is secure and private while using it to detect fraud presents another difficulty. Businesses must manage intricate regulatory frameworks—like the CCPA and GDPR—to comply with data protection rules and obtain insightful knowledge from customer data. For the purpose of protecting data integrity and confidentiality, it is imperative to implement strong encryption algorithms and access controls.
Notwithstanding the obstacles, employing big data solutions for fraud detection presents noteworthy prospects for establishments to augment their risk mitigation tactics. Businesses may more accurately and effectively identify fraudulent activity by utilizing machine learning algorithms and advanced analytics. Quick action in reaction to questionable activities is made possible by real-time monitoring features, which reduce financial losses and brand harm.
Big data solutions give businesses a complete picture of consumer interactions across all channels, making it possible to spot fraudulent tendencies that would go undetected with conventional techniques. Organizations can obtain meaningful insights into potential hazards and vulnerabilities inside their operations by combining different data sources into a single platform.
Based on everything mentioned above, we can draw the conclusion that although using big data solutions for fraud detection presents a number of security and data handling issues, it also offers businesses great chances to improve their risk management procedures. In today's increasingly digital environment, businesses may proactively combat growing dangers and safeguard their assets from fraudulent actions by adopting cutting-edge technologies and best practices in analytics.
11. Regulatory Compliance and Ethical Considerations in Big Data Applications for Risk Management
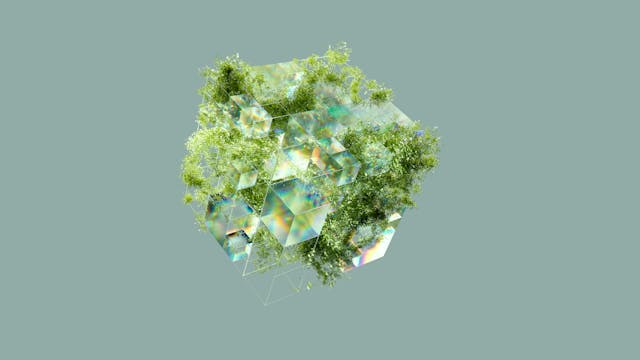
When using big data for risk management, ethical and regulatory compliance are crucial factors to take into account. Businesses need to make sure that their data practices comply with applicable laws and regulations, like the CCPA, GDPR, and industry-specific rules like HIPAA. Regulators and consumers alike depend on transparency in data collection, processing, and use. To reduce the danger of data breaches and non-compliance fines, it is imperative to use strong security measures to protect sensitive data.
Using big data for risk management and fraud detection might provide ethical challenges. Establishing explicit policies on data usage, permission, and privacy protection is essential for businesses. It's critical to strike a balance between protecting people's right to privacy and utilizing big data for commercial insights. To maintain ethical standards in their data processes, organizations should think about putting encryption tools, data minimization tactics, and anonymization procedures into effect.
One of the most important ethical factors in big data applications for risk management is the prevention of discrimination. Algorithmic decision-making procedures have the potential to unintentionally reinforce biases if they are not closely observed and addressed. Organizations can mitigate the risk of biased outcomes in their risk management methods by implementing fairness-aware algorithms, regularly undertaking bias evaluations, and supporting diversity in dataset gathering.
Based on the aforementioned information, we may draw the conclusion that ethical and regulatory compliance are essential to the responsible use of big data for sophisticated fraud detection and effective risk management. Organizations can reap the benefits of big data analytics while maintaining integrity and confidence in their operations by giving priority to openness, security, privacy protection, bias mitigation, and fairness in their data processes.
12. Future Trends in Big Data Utilization for Enhanced Security and Risk Mitigation
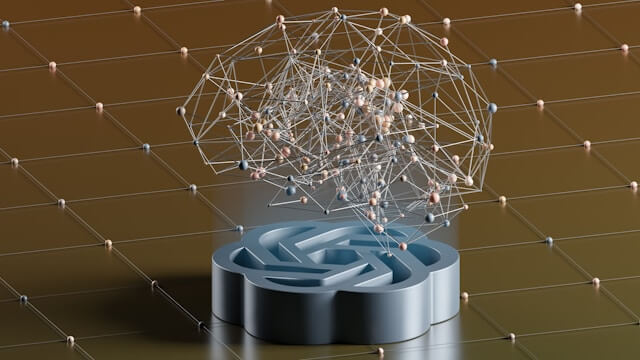
Using big data is becoming more and more important in the changing security and risk management landscape for fraud detection and prevention. Looking ahead, a number of big data use trends have the potential to completely transform security approaches and improve risk mitigation initiatives.
1. **AI-Powered Fraud Detection**: Algorithms based on artificial intelligence (AI) will be essential to improving fraud detection skills. Massive volumes of data may be sorted through in real time by machine learning models, which can then be used to spot suspicious trends and abnormalities that might point to possible fraud. AI will be used in fraud prevention in increasingly complex and efficient ways as it develops.
2. **Behavioral Biometrics**: As a potent deterrent to fraud, using behavioral biometrics for authentication is becoming more and more popular. Through the analysis of distinct patterns in user behavior, such as mouse movements, touchscreen motions, and typing speed, businesses can generate profiles that aid in the differentiation of authentic users from fraudulent ones. Traditional techniques frequently lack an additional degree of protection, but this type of biometric authentication does.
3. **Blockchain for Transparency**: Financial transaction traceability and transparency can be improved by using blockchain technology into security frameworks. Blockchain guarantees that all transactions are recorded immutably, lowering the possibility of fraud or manipulation. Data is stored in decentralized ledgers that are safe and impenetrable.
4. **Difficulties with Quantum Computing**: For cybersecurity professionals, quantum computing offers both potential and difficulties with its promise of exponentially faster processing rates. Although quantum computing can improve encryption techniques, it also presents a risk to established security measures since it may be able to decipher private information that was thought to be secure. Organizations will need to modify their security protocols as quantum computing develops in order to remain ahead of potential threats.
5. **Real-Time Data Monitoring**: By using real-time data monitoring solutions, businesses may now identify fraudulent activity before it happens, as opposed to after it has already happened. Through constant examination of incoming data streams for irregularities or questionable conduct, enterprises can proactively address risks prior to their escalation and mitigate possible harm.
6. **Collaborative Threat Intelligence Sharing**: It's becoming more and more important for industry peers to share collaborative threat intelligence in a time when cyber threats cross corporate borders. Organizations may bolster their defenses against cyberattacks and stay ahead of hostile actors by combining anonymized data on emerging risks and attack vectors.
7. **Predictive Analytics for Risk Management**: By predicting possible hazards based on past data patterns and trend analysis, predictive analytics will continue to be essential to risk management. Through the application of predictive models driven by big data insights, companies can anticipate vulnerabilities and take proactive steps to address hazards before they arise.
8. **Regulatory Compliance Automation**: By effectively monitoring and reporting conformity to industry norms and regulations, automation technologies powered by big data analytics will streamline regulatory compliance operations. Organizations may guarantee operational effectiveness while lowering the possibility of fines or penalties for non-compliance by utilizing automated compliance solutions.
9. **Cloud-Based Security Solutions**: Using cloud-based security solutions allows for the flexible and scalable deployment of strong cybersecurity defenses across a range of digital contexts. Cloud platforms with sophisticated threat detection features give enterprises the flexibility they need to successfully defend their systems against constantly changing cyberthreats.
10. **Integration of Cyber Insurance**: Given the rapid evolution of cyber risks, it is anticipated that businesses looking for all-encompassing protection against possible financial losses from cyber disasters or breaches will increasingly integrate cyber insurance plans with big data analytics.
Organizations may actively protect their assets against fraudulent activity and unanticipated hazards while remaining resilient in the face of changing threats by adopting these future trends in big data use for increased security and risk mitigation techniques.