1. Introduction to Cybersecurity and AI
Cybersecurity is essential in today's digital world to protect our private data from online attacks. The increasing complexity of cyberattacks presents many obstacles for enterprises trying to safeguard their systems and data. With ransomware, phishing, malware, and insider threats among other things, the cybersecurity landscape is always changing and new solutions are needed to remain ahead of the bad guys.
Artificial Intelligence (AI) has become a potent instrument for strengthening cybersecurity protocols. By utilizing machine learning and artificial intelligence algorithms, businesses may strengthen their defenses and proactively identify and address possible security breaches. Real-time threat analysis, anomaly detection, and predictive modeling are made possible by AI, which gives cybersecurity experts the ability to see weaknesses before attackers do.
Enhancing threat detection, incident response times, and overall security posture are all improved by using AI into cybersecurity procedures. Organizations may increase risk mitigation, expedite repetitive security activities, and free up staff for more strategic endeavors by utilizing AI-driven automation. In this blog article, we'll look at how companies may use AI to improve cybersecurity assurance and fortify their defenses against ever changing cyberthreats.
2. Understanding the Role of AI in Cybersecurity
AI is essential for improving cybersecurity measures since it provides cutting-edge tools for identifying and averting online threats. AI systems can evaluate enormous volumes of data in real-time to spot trends, abnormalities, and possible threats that could be overlooked by conventional security procedures by utilizing machine learning algorithms. Artificial intelligence-driven systems, for example, have the ability to quickly identify suspicious activity or attempts at malware penetration by monitoring system logs, network traffic, and user behavior.
Threat detection is a well-known use of AI in cybersecurity. Artificial intelligence (AI) systems can detect odd behavior that may be a sign of a cyberattack immediately by continuously analyzing network data and monitoring system activities. These technologies use previous data to learn from and adjust their detection methodologies to detect sophisticated threats such as insider threats or zero-day exploits. In order to proactively strengthen defenses against possible breaches, artificial intelligence (AI) can automate the process of finding vulnerabilities in a system or application before hackers use them.
Threat prevention is a crucial area of cybersecurity where AI shines. By using predictive analytics to foresee potential attack vectors and vulnerabilities within an organization's infrastructure, AI-driven systems may proactively fight against cyber attacks. Artificial intelligence (AI) systems are able to classify typical patterns of behavior and quickly identify deviations that may indicate a security issue. These techniques include behavioral analysis and anomaly detection. Organizations can use these data to develop proactive security solutions that reduce risks before they become major security incidents.
Organizations can successfully strengthen their defenses against evolving cyber threats by incorporating AI technology into cybersecurity operations. In today's quickly changing threat landscape, artificial intelligence (AI) is essential for protecting digital assets and upholding a strong cybersecurity posture, from strengthening preventive measures to improving threat detection methods.
3. Implementing Machine Learning for Cyber Threat Detection
The cybersecurity industry has undergone a revolution with the application of machine learning for cyber threat identification. Algorithms for machine learning are essential for recognizing and successfully countering online threats. These algorithms have a high degree of accuracy when it comes to pattern recognition, anomaly identification, and data analysis. Organizations may detect possible dangers in real-time and stay one step ahead of cyber attackers by utilizing machine learning.
There are numerous advantages of employing machine learning models for real-time threat detection. These models outperform conventional rule-based techniques because they can continuously learn from fresh data and adjust to changing threats. Additionally, automated threat detection made possible by machine learning can drastically cut down on response times and human error when detecting and controlling security breaches. The capacity of machine learning algorithms to concurrently examine several data sources improves an organization's overall cybersecurity posture.
As previously said, there are unmatched benefits to using machine learning for cyber threat identification when it comes to protecting digital assets from advanced cyberattacks. Organizations may improve their cybersecurity skills and better defend themselves in the constantly changing threat landscape of today by utilizing machine learning algorithms.
4. Enhancing Incident Response with AI Technology
Cybersecurity procedures are being revolutionized by the use of AI technologies to improve incident response. Artificial Intelligence (AI) solutions have the potential to greatly improve incident response speed and accuracy, allowing firms to identify and address hazards instantly. Artificial intelligence (AI) can swiftly evaluate large amounts of data, spot patterns, and find abnormalities that human analysts would miss by utilizing machine learning techniques. As a result, threats are identified and contained more quickly, which eventually lessens the effect of cyberattacks.
Case studies offer concrete illustrations of how AI has changed incident response within enterprises. An eminent financial institution, for example, incorporated threat detection systems driven by AI, resulting in a 60% reduction in incident reaction times. Through automatic risk-level-based alert prioritization, the technology allowed security personnel to quickly concentrate on the most serious threats. During an incident, a different organization effectively employed AI chatbots to facilitate communication, promoting prompt teamwork and enhancing overall response effectiveness. These success stories show how intelligent automation and analysis powered by AI not only increases the efficacy of incident response, but also speeds it up.
5. Utilizing Natural Language Processing (NLP) in Cybersecurity
NLP is essential for enhancing cybersecurity efforts since it makes it possible to analyze security records and messages. Organizations can gain important insights from unstructured data sources like incident reports, threat intelligence feeds, and communication logs by applying natural language processing (NLP) approaches. NLP algorithms assist in finding patterns, abnormalities, and possible dangers that are concealed inside enormous volumes of text data that would be difficult for people to manually examine.
Sentiment analysis, which uses NLP to analyze the context and tone of messages within an organization's network, is one useful use of NLP in cybersecurity. Through the use of NLP algorithms, employee conversations via emails or chat messages can be monitored, and any suspicious or malevolent intent conveyed in the language used can be flagged. This proactive strategy aids in the early detection and prevention of threats prior to the occurrence of a cyberattack.
Another example is the automatic classification and ranking of security alerts produced by monitoring systems through the use of natural language processing (NLP). Organizations may quickly identify significant occurrences that need immediate attention by using natural language processing (NLP) techniques to analyze the content of security alerts. This allows security teams to respond to possible risks effectively in real-time.
By examining email contents for questionable patterns or language suggestive of phishing assaults, natural language processing (NLP) can help identify phishing efforts. Organizations can greatly improve their email security posture by utilizing NLP algorithms to identify similar traits in incoming communications and training machine learning models on historical phishing emails.
As I mentioned above, using natural language processing to cybersecurity improves threat detection capabilities and lets businesses automate time-consuming processes like message categorization and log analysis. Businesses can increase overall operational efficiency and strengthen their defenses against emerging cyber threats by integrating NLP technologies into their cybersecurity toolkits.
6. Securing IoT Devices with AI-driven Solutions
Because of its inherent weaknesses, IoT device security is critical in the field of cybersecurity. These gadgets, which range from industrial sensors to smart home appliances, are frequently weakly secured and open to intrusions. Weak default passwords, unprotected communication, and infrequent security updates are prevalent problems.đź‘
AI-powered technologies present a viable way to improve IoT device security. Large volumes of data may be analyzed by machine learning algorithms, which can then be used to spot anomalies in device behavior and early warning signs of possible risks. AI can also reduce the window of exposure to cyber hazards by automating the process of patching vulnerabilities and immediately upgrading device software.
IoT devices can gain real-time monitoring of developing cyber risks globally by utilizing AI for threat intelligence. By taking a proactive stance, organizations can remain ahead of possible threats and modify their security protocols as needed. To ensure a strong defense against changing cybersecurity threats, AI-driven solutions must be incorporated into IoT security strategy.
7. The Future of AI and Cybersecurity Assurance
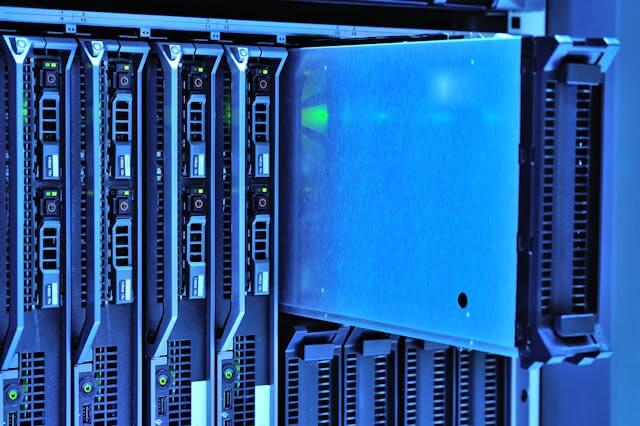
AI in cybersecurity has a bright future ahead of it, with developments predicted to completely change the cyber defense environment. According to predictions, AI will be essential to threat identification, response automation, and predictive analysis—all of which will improve an organization's security posture. AI algorithms are expected to perform better than conventional techniques in detecting and thwarting emerging threats as they advance in sophistication.
However, there are difficulties and ethical issues with using AI into cybersecurity procedures. When using AI for security, concerns including data privacy, algorithmic biases, and responsibility come into play. Going forward, it will be critical to strike a compromise between resolving these ethical issues and optimizing the advantages of AI. When deploying AI-driven cybersecurity solutions, organizations must put ethical principles and transparency first in order to build confidence and adhere to legal requirements.