1. Introduction
With the advent of AI and machine learning in 2023, the area of data engineering saw tremendous progress. Real-time data processing, cloud-native technology, and improved data governance procedures became the main points of emphasis. Data engineers play a critical role in maintaining reliable data pipelines and effective processing systems as firms use data for decision-making more and more.
Anticipating 2024's data engineering trends is essential to maintaining competitiveness in a quickly changing technology environment. Organizations can proactively seize fresh possibilities and get ready for impending obstacles by anticipating future events. Data engineers may efficiently handle the increasing needs of modern data architectures by adapting their skill set and tools by recognizing changing trends and technology.
2. Prediction 1: Rise of Automated Data Pipelines
By 2024, there will be major developments in the field of data engineering, and automation will be essential in determining the direction that data pipelines go. The emergence of automated data pipelines, fueled by the growing use of advanced technologies intended to optimize and speed data pipeline management procedures, is one important prediction. The way businesses manage their data operations is about to change dramatically thanks to these automated technologies, which offer unprecedented scalability and efficiency.
There is anticipated to be a paradigm shift in the way businesses handle their data infrastructure with the implementation of automated tools for data pipeline management. Organizations can streamline operations and implement changes more quickly by utilizing automation technology to decrease the amount of manual intervention required to manage data pipelines. This results in increased operational efficiency as well as the release of significant time and resources that can be allocated to more strategic projects.
It is impossible to overstate how much automated data pipelines affect scalability. The exponential growth of data volumes is making old manual approaches to pipeline management increasingly untenable. By enabling systems to change dynamically to shifting workloads and requirements without requiring significant manual adjustments, automated technologies help businesses scale their data operations effectively in response to expanding demands.
The emergence of automated data pipelines heralds a new age in data engineering techniques, one in which scalability and efficiency are integral parts of a contemporary, flexible data architecture rather than trade-offs. Organizations may create corporate growth and competitive advantage in 2024 and beyond by adopting these advancements and positioning themselves at the forefront of innovation and data asset utilization.
3. Prediction 2: Evolution of Real-Time Processing
Prediction 2: Evolution of Real-Time Processing Real-time data processing and analysis are set to take center stage in 2024 as businesses increasingly rely on up-to-the-minute insights for decision-making. This shift towards real-time processing is being primarily driven by advancements in technology, particularly in stream processing frameworks. Stream processing frameworks like Apache Kafka, Apache Flink, and others will play a crucial role in enabling organizations to process and analyze data as it arrives, allowing for quicker decision-making and more agile operations. The ability to harness real-time data will give companies a competitive edge by providing instant insights into customer behavior, operational efficiency, and emerging trends.
Stay tuned as we delve into more predictions for the future of data engineering in 2024!
4. Prediction 3: Enhanced Focus on Data Quality and Governance
Data quality and governance will receive more attention in data engineering by 2024. Organizations will place a higher priority on making sure that their data pipelines are both effective and compliant with strict regulations, as worries about data privacy and compliance grow. This change will result in the application of tactics meant to preserve compliant, high-quality data pipelines.
Businesses will make investments in systems and procedures that allow them to track and improve the quality of their data all the way through its lifecycle in order to address these issues. Workflows for data engineering in the current era will require automated tools for data profiling, cleansing, and enrichment. Strong governance frameworks will be created by organizations to guarantee that data is handled sensibly and in compliance with laws like the CCPA and GDPR.
Businesses can reduce the risks of non-compliance while fostering confidence with stakeholders and customers by concentrating on data quality and governance. By encouraging a culture of responsibility and openness around data usage, these initiatives will not only assist promote innovation but also increase the dependability of analytics and decision-making processes. Prioritizing quality and compliance in the quickly changing field of data engineering will be essential to realizing big data's full potential as a driver of company growth.
5. Prediction 4: Integration of Machine Learning with Data Engineering
Prediction 4: Integration of Machine Learning with Data Engineering
Data engineering and machine learning (ML) combined are expected to revolutionize the way businesses use data by 2024. At this point of convergence, more sophisticated ML algorithms will be included into conventional data engineering techniques at the interface between ML models and data pipelines. Organizations can anticipate more automated and intelligent data processing procedures by incorporating machine learning into their data pipelines.
The creation of more intelligent data infrastructures will be significantly impacted by this connection. Data engineers will put more and more effort into creating tools that streamline data management and processing while also making it easier to train, deploy, and monitor machine learning models all inside the same pipeline. Businesses may now automate decision-making processes based on real-time analysis and extract important insights from their data at scale thanks to the transition towards more sophisticated data infrastructures.
Businesses need to invest in upskilling their people so they are knowledgeable in both domains as machine learning gets more and more entwined with data engineering methods. For data engineers to successfully incorporate predictive analytics into their current workflows, they must become knowledgeable about machine learning principles and methodologies. In a similar vein, data scientists will gain from comprehending the foundational ideas of data engineering in order to facilitate smooth teamwork and optimize the performance of their machine learning models in real-world settings.
A new era when businesses can leverage AI-driven insights to improve customer experiences, streamline processes, and spur innovation across the board is heralded by the convergence of ML and data engineering. Businesses may maintain an advantage in a highly competitive environment where data-driven decision-making is the driving force behind operations by embracing this convergence and modifying their infrastructure and skill sets accordingly.
6. Prediction 5: Cloud-Native Architectures for Data Engineering
Prediction 5: Cloud-Native Architectures for Data Engineering
We predict a sharp increase in the use of cloud-native big data workload management systems in 2024. Companies will use cloud computing platforms such as AWS, Azure, and Google Cloud more frequently in order to take advantage of the cost-effectiveness, scalability, and flexibility that these environments provide. Organizations will be able to better manage the challenges of processing and storing massive volumes of data thanks to the move toward cloud-native architectures.
Data engineering techniques gain a great deal from using cloud-native strategies. These include better resource usage through variable allocation of compute power and storage, more agility in deploying and scaling infrastructure based on changing demands, and simplified administration of intricate data pipelines. Teams can concentrate more on innovation with cloud-native architectures, as opposed to handling infrastructure maintenance responsibilities.
Nevertheless, difficulties arise despite cloud-native solutions' growing appeal. Enterprises have to deal with challenges such guaranteeing data safety and adherence in dispersed cloud settings, maximizing expenses as tasks grow in size, and efficiently coordinating various parts within intricate data structures. Managing the risks associated with vendor lock-in and keeping up with the swift advancement of cloud technologies are ongoing problems that require data engineering teams to strategically plan and adapt.
Understanding the nuances of cloud-native architecture implementation will be essential for enterprises looking to realize the full benefits of their big data projects as we approach 2024 and beyond. Through a comprehensive comprehension of the benefits and challenges linked with these methodologies, enterprises may proactively arrange themselves to prosper in an ever-more data-centric environment.
7. Prediction 6: Influx of Edge Computing in Data Engineering
Prediction 6: Influx of Edge Computing in Data Engineering
By putting processing capacity closer to the point of data generation, edge computing has the potential to completely transform data engineering by 2024. This change will improve real-time processing capabilities and drastically lower latency. The capacity of edge computing to analyze and act on data locally without continuously transferring it back to centralized servers is highlighted by its role in processing big data at the edge.
Numerous use cases and opportunities arise when edge computing is integrated into data engineering processes. For example, in Internet of Things applications, sensor data analysis at the edge might lead to instantaneous responses based on predefined thresholds, improving the responsiveness and efficiency of processes. By analyzing crucial information closer to the source, edge computing and data engineering enable smarter decision-making, resulting in quicker insights and better outcomes.📲
8. Prediction 7: Emphasis on Ethical AI Practices in Data Engineering
A significant forecast for data engineering in 2024 is the growing focus on moral AI procedures. This change demonstrates how ethical issues are becoming more and more important while creating AI solutions. It is anticipated that data engineers would devote more time to incorporating ethics into the pipeline at every level of data processing.
Strategies like building clear governance frameworks, ensuring regulatory compliance, encouraging transparency and accountability in data collection and usage, and integrating bias detection mechanisms into algorithms will all be critical to successfully implementing ethical practices in data engineering. Building ethical AI systems will require data teams to cultivate a culture that values diversity and ethics.
Organizations may reduce risks associated with prejudice, privacy infringement, and unfair decision-making by algorithms, as well as build trust with users and stakeholders, by giving ethical AI practices top priority in data engineering processes. Adopting these tactics will make it possible to guarantee that AI solutions preserve moral principles and protect individual rights while making a constructive contribution to society.
9. Prediction 8: Advanced Analytics and Visualization Techniques
By 2024, advanced analytics and visualization approaches in data engineering should have improved significantly. This forecast anticipates a profound exploration of advanced analytics instruments and visualization technologies that will transform the comprehension and application of data.👍
Given the ongoing advancements in technology, data engineers are expected to investigate novel approaches that extend the limits of conventional analytics. Businesses can anticipate a breakthrough in their ability to make decisions by utilizing these cutting-edge tools.
Data engineers can extract meaningful insights from large, complicated information by using advanced analytics technologies. This allows businesses to make well-informed decisions quickly and precisely. By using state-of-the-art visualization technologies, it will be easier to analyze and share these insights, leading to a better comprehension of intricate data trends and patterns.
By putting more of an emphasis on advanced analytics and visualization methods in 2024, businesses will be better equipped to realize the full value of their data assets. This pattern emphasizes how crucial it is becoming to use technology to inform strategic decision-making and provide businesses a competitive advantage in the data-driven world of today.
10. Prediction 9: Increased Adoption of Serverless Architectures
The ninth prediction for data engineering in 2024 suggests that serverless architectures will be used more frequently. The advantages of serverless computing in simplifying data engineering procedures are what are causing this change. With serverless architectures, teams can concentrate more on creating useful data pipelines and analytics since they provide scalability, cost effectiveness, and less operational overhead.
Automatic scaling is one of the main advantages of serverless computing in data engineering. Serverless architectures provide cost-effective and efficient resource use by dynamically provisioning resources according to workload demands. This elasticity offers flexibility for changing data processing requirements by allowing it to handle varied workloads without the need for user intervention.
Data engineers are relieved of the responsibility of overseeing infrastructure maintenance duties such as allocating servers and overseeing software updates by serverless architectures. Teams may focus more on developing and improving data workflows than on the underlying infrastructure by abstracting away these worries, which will increase productivity and speed up the innovation cycle.
Notwithstanding these benefits, there are drawbacks to using serverless architectures in data engineering. To find performance problems and maximize resource usage, serverless functions must be properly monitored and observable. To effectively debug any potential bottlenecks and obtain insights into system behavior, data engineers need to build comprehensive logging and monitoring systems.
Managing interdependence amongst serverless services in intricate data pipelines presents another difficulty. Effectively maintaining data consistency and integrity across distributed systems requires careful design considerations when coordinating event-driven workflows and orchestrating different microservices.
The best practices for building serverless architectures include using a modular approach to divide large, complicated operations into smaller, independently deployable and scaleable units in order to overcome these issues. High availability and fault tolerance can be ensured while streamlining deployment and operational management with the help of managed services like AWS Lambda or Google Cloud Functions.
An increasing number of data engineers are using serverless architectures, which represents a paradigm change in favor of more flexible, scalable, and affordable solutions. Organizations may effectively leverage serverless computing's potential to foster innovation in data processing pipelines and analytics processes in 2024 and beyond by comprehending its advantages and drawbacks.
11. Prediction 10: Expansion of Graph Databases in Data Engineering
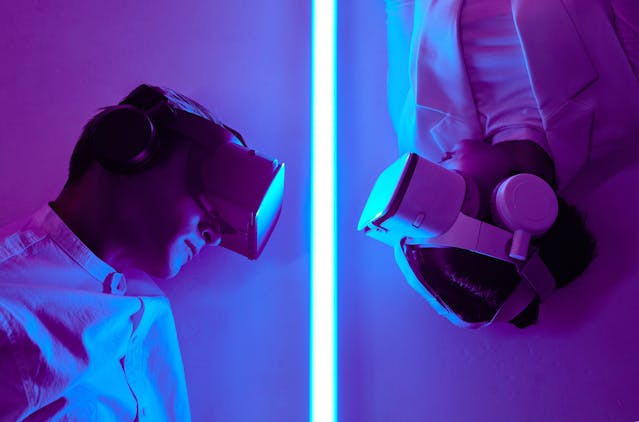
One significant forecast for 2024, given the continuous progress in data engineering, is the growth of graph databases in this domain. It is expected that graph databases will become increasingly important since they have certain advantages and uses that standard relational databases do not have.
Graph databases are becoming more and more important in data engineering because of their capacity to model and depict intricate relationships between data items. Their ability to comprehend interconnection and patterns makes them very useful in situations like social networks, fraud detection, recommendation systems, and network analysis.
Graph databases provide quicker query performance than relational databases because of their efficient relationship traversal and flexibility in managing dynamic schemas. The querying method for interconnected entities is made simpler by their intrinsic support for connected data.
On the other hand, great thought must go into integrating graph databases into data engineering processes. Even while they are quite good at some kinds of queries, such pattern matching and graph traversals, they might not be appropriate for every situation. Evaluating factors like data volume, query complexity, and transaction requirements is important when choosing whether to use a graph database system.
Data engineers are exploring graph databases as a promising option to improve their ability to handle complex relationships in datasets, as more and more organizations look for more robust methods to handle and analyze their data. Businesses can decide whether to integrate graph databases into their data-driven initiatives going forward by having a thorough awareness of the applications, benefits, and issues related to doing so.
12. Conclusion
To summarize my previous writing, the data engineering landscape in 2024 is expected to be dynamic and transformational. Important forecasts include heightened awareness of ethical data practices, the emergence of AutoML and MLOps, improved data security protocols, a wider acceptance of serverless architecture, and the incorporation of AI-powered automation technologies.
It is advised of professionals in the area to keep up with these changing trends. Data engineers can position themselves for success in a quickly changing digital environment by staying up to date with industry changes, adding new skills to their skill set, and embracing new technologies. In order to remain competitive and relevant in the data engineering industry in 2024 and beyond, it will be crucial to embrace ongoing learning and take the initiative to adopt new technologies.