1. Introduction to the theme - "Will the Real Data Scientist Please Step Forward?"
The headline "Will the Real Data Scientist Please Step Forward?" draws attention to a common problem in the ever-evolving field of data science: the variety of jobs and identities that exist within it. The term "data scientist" no longer has traditional connotations as decision-making within organizations depends more and more on data-driven insights. This blog post explores the multifaceted nature of data science, illuminating the diverse skill sets, roles, and personas that are involved in this field. Come along as we explore the nuances of what, in the rapidly changing field of data analytics and interpretation today, actually defines a data scientist.🗓
2. Defining the role of a data scientist in today's tech-driven world.
A data scientist's job is very essential in today's tech-driven environment. Professionals with the abilities to glean knowledge and insights from data are known as data scientists. Their distinctive combination of programming ability, domain knowledge, statistical knowledge, and critical thinking skills allows them to extract meaningful insights from intricate datasets.
Data scientists analyze vast amounts of data to find patterns, trends, and correlations, which is how they assist firms make wise decisions. Their work is essential for guiding company strategy, streamlining procedures, maximizing effectiveness, and forecasting future results. Data scientists may extract meaningful insights that spur innovation and growth from data by utilizing sophisticated analytical techniques and machine learning algorithms. 😷
Data scientists are skilled in creating prediction models, planning experiments, gathering and analyzing data effectively, interpreting findings, and presenting information to stakeholders. They frequently collaborate closely with cross-functional teams to use data-driven approaches to cooperatively address problems. Across all industries, there is an increasing need for qualified data scientists as businesses depend more and more on data to obtain a competitive advantage.
A data scientist's work is essentially dynamic and diverse. It calls for a blend of topic knowledge, problem-solving techniques, technical proficiency, inventiveness, and communication skills. In addition to analyzing data, data scientists are essential in developing business plans that are grounded in empirical facts rather than hunches or gut feelings. Their capacity to utilize data for making decisions renders them priceless resources in the fast-paced, information-rich world of today.
3. Exploring the skills and qualifications required to be a successful data scientist.
A combination of technical expertise, domain knowledge, and critical thinking skills are needed to be an effective data scientist. For data processing and analysis, computer languages like Python, R, or SQL proficiency is required. Data scientists can extract insights from large datasets by using their expertise in statistical modeling and machine learning methods.
Making data-driven judgments and comprehending intricate algorithms require a solid foundation in mathematics and statistics. In order to effectively communicate their findings to non-technical stakeholders inside a company, data scientists should also be proficient communicators. The ability to adapt and never stop learning are essential in this quickly changing sector.
Data scientists frequently have formal education in disciplines like computer science, mathematics, statistics, or economics. A master's or doctorate degree might offer more in-depth understanding of particular fields within data science. But gaining real-world experience through projects, internships, or employment is essential to developing the kind of abilities required to succeed in the industry.
Accreditation from respectable companies such as Google (Professional Data Engineer) or Microsoft (MCSE: Data Management and Analytics) can help bolster one's credibility and showcase proficiency in particular tools or technologies. A successful data scientist in today's competitive landscape possesses a combination of technical ability, domain understanding, ongoing learning, and strong communication abilities.
4. The evolving landscape of data science and its impact on businesses and industries.
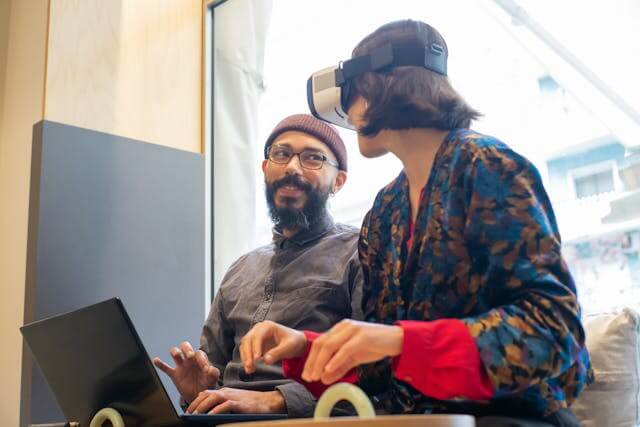
The global business and industry landscape is being revolutionized by the rapidly changing field of data science. Organizations are turning to data scientists in order to gain insightful knowledge and facilitate well-informed decision-making, since the amount of data created keeps growing dramatically. Data science is becoming a vital component of getting a competitive edge rather than a specialized field.
Data science is being used by sectors including technology, retail, healthcare, and finance to improve user experiences, streamline operations, and create novel goods and services. Data science is transforming business operations in today's information-driven environment, whether it is through fraud detection, personalization of suggestions, or behavior prediction.
To properly leverage their data, businesses are investing more and more in creating strong data science teams. In addition to being adept at managing intricate algorithms, data scientists are also knowledgeable about business objectives and know how to convert data findings into practical plans. Their function has progressed from simple data entry to become strategic collaborators that influence the course of businesses.
Businesses that embrace data science stand to gain a substantial competitive advantage in this fast changing landscape where digital transformation is critical for survival. In an era where information is ubiquitous but insights are rare, the ability to make data-driven decisions quickly and accurately can mean the difference between success and failure. More breakthroughs and upheavals are likely to come as more sectors see the benefits of data science.
5. Challenges faced by data scientists in their day-to-day work.
Everyday obstacles that data scientists face might have an impact on their productivity and efficiency. One frequent problem is poor data quality, which can produce deceptive results if left unchecked. Examples of these problems include missing numbers, outliers, and inaccuracy. Due to the fast changing nature of data science tools and processes, another challenge is the requirement to continuously upgrade their knowledge and skills.
One of the biggest challenges facing data scientists is explaining complicated findings and insights to stakeholders who aren't technical. They need to successfully communicate the importance of their analysis in a way that others without a training in data science could grasp. There may be additional pressure to generate excellent solutions within short time limits due to time constraints and tight deadlines.📍
Effective data management techniques and strong computational capabilities are necessary when handling massive amounts of data. Another difficulty is ensuring data security and privacy, especially when handling sensitive data. It can be challenging for data scientists working in highly regulated businesses to strike a balance between the requirement for innovation and regulatory compliance.
Taking into account everything mentioned above, we can say that although data science presents fascinating chances for understanding and exploration, data scientists have to overcome a number of obstacles in their daily job to make the most of their knowledge and abilities. Professionals in this sector need to be flexible, always learning, able to communicate effectively, and have strategic problem-solving skills in order to overcome these challenges.
6. The importance of ethics and responsibility in data science practices.
Ethics and accountability are important factors that influence data science techniques and results. Given the enormous power that data scientists possess due to their ability to analyze and draw conclusions from large amounts of data, it is crucial to take the ethical implications of their work into account. Upholding ethical standards in the collection, handling, and analysis of data is essential for preserving social trust and safeguarding people's right to privacy.
Data science ethics cover a wide range of issues, including protecting data privacy, making sure algorithms are transparent and fair, and eliminating biases that can produce unfair results. Data scientists must follow established norms and frameworks that encourage responsible data practices in order to resolve these moral conundrums. Data scientists can reduce the dangers connected with unethical data use while promoting an integrity-conscious culture inside their organizations by adhering to ethical norms in their work.
In data science, accountability goes beyond personal behavior and includes corporate responsibility for the results of data-driven choices. It is the responsibility of data scientists to think about how their work will effect society as a whole and make moral decisions that put the welfare of the people and communities they analyze first. Data scientists may help create a more fair and just digital environment where data is used to drive positive social change by adopting responsibility in their work.
To sum up everything I've written thus far, ethics and accountability are essential data science pillars that direct practitioners toward making a significant effect while respecting moral principles. In order to drive innovation with integrity and guarantee that technology serves humanity's greatest interests, data scientists must embrace ethical principles and accept responsibility for the results of their work. The full potential of this revolutionary profession can only be fulfilled for the benefit of society when the true data scientist comes forward, committed to ethics and accountability.
7. Debunking common myths and misconceptions about data scientists.
Many myths and misconceptions exist in the field of data science, making it difficult for the public to comprehend the actual duties of a data scientist. A prevalent misconception is that data scientists are only interested in programming and coding. Although a data scientist must possess technical skills, their responsibilities go beyond programming. Strong analytical abilities, subject expertise, and the capacity to explain complicated findings to stakeholders who are not technical are further requirements for data scientists.
The idea that data scientists operate alone is another common misperception. As a collaborative career, data science actually necessitates working with other professionals including engineers, business analysts, and subject matter experts. Data scientists need to collaborate well and communicate effectively in order to comprehend business requirements, formulate pertinent queries, and offer insightful data analysis.
There are others who would think that data science is limited to making 100% accurate predictions about the future. On the other hand, data scientists base their forecasts on trends and probabilities seen in past data. Rather than delivering conclusive answers, data science offers insights into potential outcomes, which aids in the process of making educated decisions. It's critical to see data science not as a crystal ball for future prediction but as a tool for improving decision-making processes.
It's a common misunderstanding that anyone can learn a computer language or technology and become a Data scientist overnight. More than simply technical know-how are needed to become successful in data science; ongoing education, critical thinking, problem-solving skills, and a grasp of statistical ideas are also necessary. To remain current and productive in their positions, data scientists must adjust to new technologies and procedures that are continuously being developed in the industry.
We can have a better grasp of the complex nature of data scientists' work by dispelling these myths and misconceptions about them. When it comes to gleaning valuable insights from massive volumes of data to inform organizational decision-making, data scientists are essential. In this dynamic and ever changing sector, knowing the true meaning of what it means to be a data scientist can help close the gap between expectations and reality.
8. The future of data science: emerging trends and technologies to watch out for.
Future developments in the rapidly developing field of data science are being shaped by a number of new trends and technology. Machine learning operations (MLOps), which simplify the deployment and management of machine learning models at scale, are one such trend that is becoming more and more significant. Improved model performance and efficiency are ensured by enhanced collaboration between data scientists and IT operations teams through the integration of DevOps principles with machine learning workflows.
The emergence of AutoML, which speeds up model creation and makes machine learning more approachable for non-experts by automating certain phases of the process, is another significant trend. Businesses can now use predictive analytics without requiring a high level of expertise because to the democratization of AI, which speeds up understanding and decision-making.
Explainable AI, or XAI, is becoming more and more popular as businesses look for accountability and transparency in AI-driven choices. By making complex algorithms understandable to people, XAI techniques hope to help stakeholders comprehend how a model comes to a specific result. This helps guarantee ethical use in delicate areas like healthcare and finance and also builds confidence in AI systems.
By lowering latency, increasing privacy compliance, and putting computation closer to data sources, edge computing is transforming data science. Organizations can execute real-time analysis without depending entirely on cloud resources by processing data locally on devices or edge servers. This opens the door to quicker insights in IoT installations or remote locations.
Finally, while maintaining user privacy, federated learning has the potential to revolutionize the way data is used across dispersed networks. This cooperative method is perfect for sectors like healthcare and finance that have stringent data privacy laws since it enables several parties to create a shared model utilizing decentralized data sets without distributing them centrally.
Professionals hoping to fully realize the promise of data-driven insights in a quickly evolving digital ecosystem will need to stay updated and adjust to new technologies as these trends continue to impact the future of data science. Accepting these developments will ensure that businesses stay competitive in a world that is becoming more and more data-centric while also spurring innovation.
9. Spotlight on successful data scientists: case studies and interviews.
As part of our investigation into the fundamentals of becoming a great data scientist, we focus on the experiences and perspectives of individuals who have had a major influence on the industry. We hope to shed light on the many career routes these data scientists have followed as well as the essential qualities that have helped them succeed through case studies and in-depth interviews.
Sarah Chen, a seasoned data scientist known for her ground-breaking work in predictive analytics, is one such outstanding person. Sarah has years of experience, and many aspiring data aficionados find encouragement in her journey. She explores the value of constant learning, flexibility, and an unwavering curiosity to spur innovation in the rapidly changing field of data science in our interview.
James Park, a data scientist with experience at the nexus of machine learning and artificial intelligence, is the subject of another intriguing case study. James's ability to combine technical expertise with creative problem-solving abilities has allowed him to lead innovative projects that have transformed a variety of industries, including healthcare and finance. Examining his approaches to solving difficult problems and keeping ahead of the curve gives us important insights into the thinking of a real data science trailblazer.
We aim to shed light on the complex and varied nature of this exciting field of data science through these engrossing case studies and perceptive interviews with accomplished data scientists like Sarah Chen and James Park. We give an insight into what it takes to succeed in the fast-paced field of data science by showcasing their accomplishments, approaches, and life experiences. While we recognize and honor these people's achievements, we also extend an invitation to others to take advantage of the opportunities that lie ahead for those who are prepared to push the envelope and reimagine what it means to be a genuine data science leader.
10. Tips for aspiring data scientists: how to kick-start a career in this field.
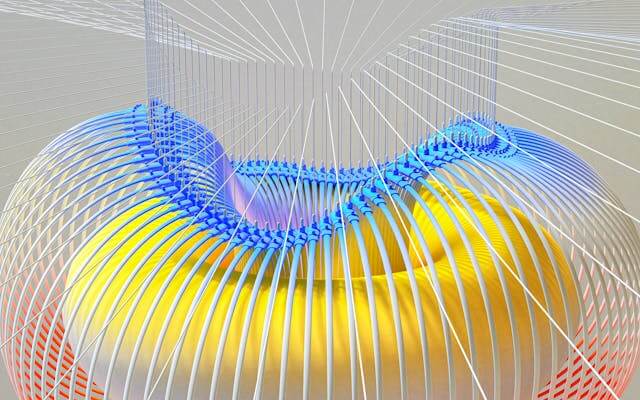
If you're aspiring to become a data scientist, here are some tips to kick-start your career in this exciting field:
1. **Learn the Basics:** Start by mastering essential skills like programming languages (Python, R), statistical analysis, and data manipulation.
2. **Understand the Math:** Brush up on your statistics, linear algebra, and calculus fundamentals. These form the backbone of data science.
3. **Hands-On Practice:** Gain practical experience by working on datasets, participating in Kaggle competitions, or contributing to open-source projects.
4. **Build a Portfolio:** Showcase your skills through projects that demonstrate your ability to analyze data, derive insights, and create visualizations.
5. **Specialize:** Identify a niche within data science that interests you - whether it's machine learning, big data analytics, or natural language processing - and delve deeper into it.
6. **Networking:** Connect with other professionals in the field through platforms like LinkedIn, attend meetups, conferences, or join online communities to stay updated on industry trends.
7. **Continuous Learning:** Data science is a fast-evolving field; commit to lifelong learning by staying abreast of new tools, techniques, and algorithms.
8. **Obtain Certifications:** Consider earning relevant certifications such as those from Coursera (e.g., IBM Data Science Professional Certificate) or industry-recognized credentials like Certified Analytics Professional (CAP).
9. **Seek Mentorship:** Find a mentor who can provide guidance, share insights from their own journey in data science, and help you navigate challenges along the way.
10. **Apply for Internships/Entry-Level employment:** Employers seeking to hire aspiring data scientists can help you obtain practical experience by offering internships or entry-level employment. This could serve as a springboard for a lucrative data science job.
Following these pointers and staying committed to improving your data science abilities and knowledge will put you well on your path to becoming a prosperous data scientist who can significantly impact this exciting profession.
11. Collaborations between data scientists and other professionals for holistic problem-solving.
Working together, individuals from different backgrounds and data scientists may solve problems in a holistic way. Data scientists provide their analytical abilities, massive data handling experience, and algorithmic mastery of machine learning. They can efficiently tackle complicated issues by collaborating with domain experts like business analysts, engineers, or healthcare professionals.
This cooperative method guarantees that data-driven solutions are both technically sound and in line with the real-world requirements of the relevant sector or field. For example, in the healthcare industry, a data scientist working with medical professionals can create prediction models based on previous data analysis that enhance patient outcomes or maximize hospital resources.
Multidisciplinary teams encourage different approaches to problem-solving. Data scientists can learn things from interacting with experts in fields other than their own, such as marketing, finance, or sociology, which may not be evident when looking at things only from a technical standpoint. This variety of opinion frequently results in creative fixes and a deeper comprehension of the problems at hand.
The capacity to collaborate across disciplines is becoming more and more important in the fast-paced, globally connected world of today. Data scientists with strong communication and teamwork abilities can use these abilities to close the gap between technical jargon and practical applications, resulting in more seamless collaborations and eventually significant outcomes across a range of businesses and areas.
12. Conclusion: highlighting the crucial role of data scientists in shaping our digital future.
In summary, data scientists are essential in determining the direction of our digital future. Businesses, governments, and society at large depend on their proficiency in evaluating intricate data sets and extracting insightful conclusions in order to make well-informed decisions. Professional data scientists will become more and more in demand as technology continues to progress at a rapid pace.
To unleash the power of data, data scientists combine programming abilities, statistical understanding, and domain expertise. They assist enterprises in accurately projecting future results in addition to helping them comprehend historical trends. The true difficulty in today's data-driven environment is sifting through enormous volumes of data to draw insightful conclusions; this is a process that data scientists are adept at.
Data scientists have an impact on more than just company operations; they also have an impact on environmental sustainability initiatives, healthcare developments, urban planning techniques, and other areas. Through the utilisation of big data analytics and machine learning algorithms, data scientists are promoting innovation in many fields and revolutionising the approach to tackling worldwide issues.
Data scientists are essentially the ones who designed our digital environment. Their capacity to transform unprocessed data into useful insights enables decision-makers to confidently traverse challenging environments. The work of data scientists will be essential in guiding us toward a more promising and effective future as our world becomes more interconnected. Let's honor and recognize the unsung heroes who work behind the scenes to transform data into significant change.