1. Introduction to Audio Analytics
Introduction to Audio Analytics: Audio analytics is the process of analyzing and understanding audio data using advanced algorithms and technologies. It plays a crucial role in extracting valuable insights from audio sources, ranging from phone calls and customer interactions to music and environmental sounds. The importance of audio analytics lies in its ability to convert raw audio signals into actionable information, providing businesses with valuable intelligence for decision-making.
Applications in Various Industries:
Applications for audio analytics are found in many different industries, and they are transforming how businesses run and engage with their clientele. It helps businesses in the telecom industry to examine call center interactions in order to enhance customer satisfaction and ensure quality control. Audio analytics is used in healthcare to diagnose patients by studying speech patterns or finding anomalies in patient monitoring systems. It can be used in retail environments to track customer behavior by listening to background noise in the store or assessing customer satisfaction through phone calls and social media interactions. These varied applications highlight the adaptability and potential influence of audio analytics in a variety of industries.
2. Basics of Sound and Audio Processing
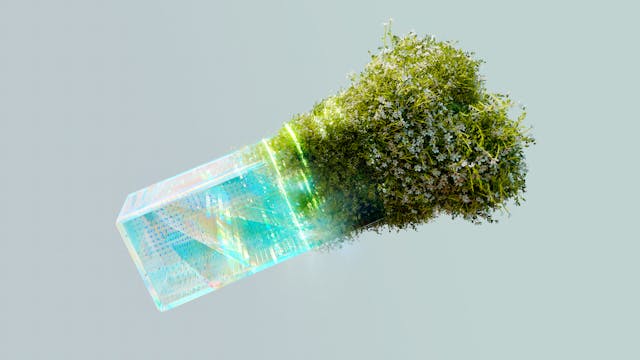
Devices like microphones, which translate sound waves into electrical impulses, are used to record and process sound. After that, these signals are converted to digital format utilizing analog-to-digital converters (ADC) found in computers and smartphones. These digital signals are altered throughout the digital audio processing process in order to improve, compress, filter, or change the sound. Compression, equalization, noise reduction, and other operations can be a part of this process. By applying precise alterations to the audio signal, algorithms for audio processing significantly influence the auditory experience that listeners receive in the end. Anyone exploring the topic of audio analytics or similar fields must have a basic understanding of digital processing and sound capture.🤝
3. Understanding Audio Data
Understanding Audio Data
There are many different formats for audio data, and each has a specific function in the field of audio analytics. Typical formats are uncompressed AIFF and WAV, which are larger but preserve excellent fidelity. Because data reduction techniques enable compressed formats like MP3 and AAC to have smaller file sizes, these formats are preferred. Audiophiles are drawn to lossless compressed codecs like FLAC because they strike a compromise between quality and size.
The distinct features of audio signals affect the way they are examined. A sound's pitch is represented by its frequency, where higher frequencies are associated with higher pitches. Understanding amplitude changes in audio data is essential for comprehending the loudness or intensity of a sound. Duration helps distinguish between short-lived, transitory sounds and continuous tones by showing the length of a sound wave.
Spectral characteristics are essential for audio analysis because they dissect signals into their component frequencies. Spectrograms show variations in frequency content over the course of an audio sample by visualizing these properties across time. Even when sounds have similar pitch and loudness characteristics, their timbre serves as a means of identifying their unique tone color or quality. Gaining an understanding of these traits is essential to deciphering the intricacies of audio data for efficient analysis and interpretation.
Understanding the various forms that audio data can take and the basic properties of audio signals serve as the basis for further exploration of audio analytics. Beginners may confidently and intelligently traverse the complex world of sound processing by becoming familiar with these fundamentals.
4. Key Concepts in Audio Feature Extraction
In audio feature extraction, two key concepts play a significant role: spectrogram analysis and Mel-frequency cepstral coefficients (MFCC).
Spectrogram analysis is the process of transforming audio signals into a picture that illustrates how the signal's frequency content varies over time. It offers a thorough perspective of the frequency content of the audio stream, which facilitates the analysis of patterns and other aspects of the sound.
Conversely, the short-term power spectrum of sound is represented by features that are retrieved from the audio signal and called Mel-frequency cepstral coefficients (MFCC). MFCCs are extensively employed in speech and audio processing because of their ability to efficiently capture important information from the audio signal while cutting down on redundancy. Compared to other conventional spectral features, these coefficients more nearly resemble the response of the human auditory system, which makes them useful for tasks like speech recognition and music classification.
5. Machine Learning Techniques in Audio Analytics
In audio analytics, machine learning is essential because it helps systems identify and analyze sound data efficiently. Convolutional neural networks (CNNs), recurrent neural networks (RNNs), support vector machines (SVMs), and Gaussian mixture models (GMMs) are among the popular machine learning techniques utilized in audio analytics. CNNs are perfect for jobs like audio classification because they are skilled at capturing spatial information in audio data.
An important step in training models for audio classification is preprocessing the data. One way to do this is to convert unprocessed audio inputs into characteristics that the machine learning model can understand. Relevant information can be extracted from audio sources using methods like wavelet transform, MFCC extraction, and spectrogram computing. Using supervised or unsupervised learning techniques, the preprocessed data is subsequently used to train the machine learning model.
The model learns from labeled training data in supervised learning, where each input signal has a corresponding class label. In order to reduce the discrepancy between the expected and real labels, the model iteratively modifies its parameters. In contrast, unsupervised learning uses unlabeled data to train models that find patterns and structures in audio signals that do not fit into pre-established categories.
To create precise and effective audio categorization models, well-preprocessed data must be combined with advanced machine learning methods. Optimizing performance and expanding the capabilities of audio analytics systems can be achieved through experimenting with various methods and fine-tuning hyperparameters.
6. Real-world Applications of Audio Analytics
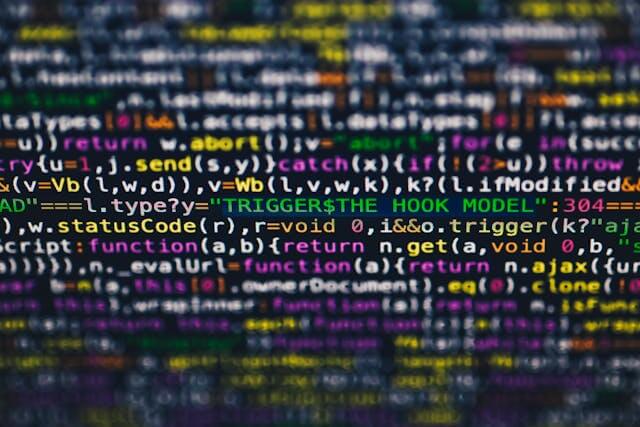
Real-world uses of audio analytics are transforming a number of industries. Virtual assistants and customer service automation have improved thanks to speech recognition technologies driven by audio analytics. Large volumes of media files can be effectively categorized and arranged using audio analytics for automated content tagging in media, which simplifies business content management procedures. These examples demonstrate how audio analytics can be used to improve user experiences and streamline operational procedures.
7. Tools and Software for Audio Analysis
There are a number of well-liked tools and software choices available for audio data analysis that can assist novices in exploring the field of audio analytics. One such program is Audacity, an open-source, free program praised for its powerful features and easy-to-use interface. With Audacity, users may examine spectrograms, apply different effects, and visualize audio waveforms.
Sonic Visualiser is another program that is frequently used in the audio analysis industry. With the help of this program, audio recordings may be visualized and annotated, which helps novices comprehend the various components of the audio data. Additionally, Sonic Visualiser has compatibility for a number of plugins that increase its capability and enable more complex studies.
For individuals who prefer working with programming languages like Python, libraries such as Librosa and PyAudio give extensive features for audio analysis. Librosa is an audio and music analysis tool that includes features for beat tracking, feature extraction, and spectrogram generation. In contrast, PyAudio is the best choice for real-time audio input capturing and processing.
For those new to speech analysis, open-source software such as Praat are also excellent resources. With Praat, users can examine formants, pitch contours, speech sounds, and other acoustic characteristics that are crucial for language acquisition or study.
Examining these well-liked software choices in conjunction with open-source tools gives novices a strong basis to begin efficiently analyzing audio data and gaining knowledge from diverse auditory sources.
8. Challenges in Audio Analytics
Experts and novices alike face substantial obstacles when it comes to audio analytics. Managing the distortion and noise in audio data is one frequent problem. It is difficult to discern significant insights when these factors degrade the sound quality. To mitigate these issues, strategies like signal processing tools and noise reduction techniques are crucial.
Taking care of privacy issues with voice data is another significant task. Voice has distinct biometric markers, thus there's a chance that private data could be compromised. Implementing powerful encryption mechanisms, assuring data anonymization, and complying with tight privacy standards are key aspects in maintaining voice data integrity and user privacy in audio analytics procedures. Analyzers and organizations alike must navigate the constant challenge of balancing the advantages of audio analysis with privacy protection. 😜
9. Future Trends and Innovations
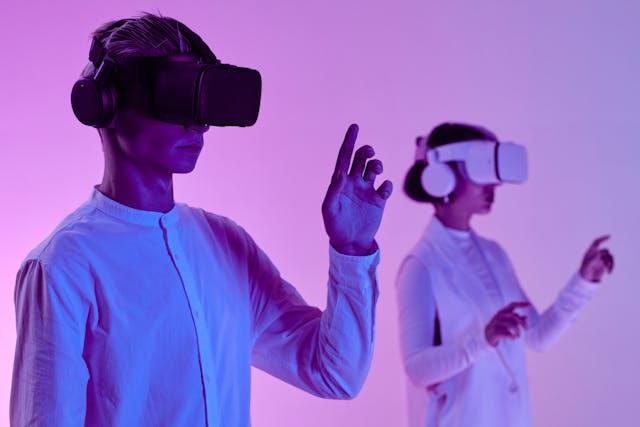
The future of audio analytics is full with exciting possibilities as new technologies continue to reshape and transform how we understand and use sound data. The use of machine learning algorithms and artificial intelligence (AI) into audio analytics tools is a significant emerging topic. These technologies allow for automated pattern identification, amazing precision in sentiment analysis, and voice biometrics, which improves the efficiency and accuracy of audio analysis.
The development of natural language processing (NLP) capabilities in audio analytics systems is another noteworthy innovation to keep an eye on. This breakthrough makes it possible to extract valuable information from spoken conversations in new ways, giving businesses a better knowledge of customer calls, meetings, and other audio sources.
Adding audio sensors to these networked systems will transform the way we gather and process audio data as Internet of Things (IoT) devices proliferate in our everyday lives. The transition to ubiquitous audio sensing opens up the possibility of real-time acoustic environment monitoring for a range of uses, including ambient noise management, smart security surveillance, and health tracking.
The future of audio analytics is expected to see a boom in bioacoustic sensing technologies, which use the distinctive sound signatures of biological organisms like animals or even human vital signs, in addition to AI integration and improvements in natural language processing. These developments have potential applications in a variety of domains, including tailored diagnostics, healthcare monitoring, and wildlife protection.
The next trends in audio analytics are poised to bring about dramatic improvements that will not only enrich our understanding of sound data but also uncover new prospects for innovation across industries. Businesses can leverage the power of audio analytics to drive informed decision-making, improve customer experiences, and remain ahead in a fast expanding digital landscape by keeping up with these emerging technologies and possible developments in the sector.
10. Ethics and Regulations in Audio Data Collection
Understanding the ethical ramifications of gathering and using audio data is essential when pursuing audio analytics. It is essential to guarantee security, consent, and privacy at every stage of the procedure. Compliance with the increasingly stringent standards in place worldwide, especially those pertaining to data protection such as the CCPA and GDPR, is mandatory. Maintaining moral principles not only helps your company gain the trust of people, but it also keeps you out of legal hot water. It is important to promote transparency and stay up to date on evolving legislation in order to safely navigate this complex landscape.
11. Resources and Learning Pathways
There are lots of learning paths and materials available to assist novices in navigating the difficult field of audio analytics. Online tutorials and courses are excellent resources for learning audio analytics since they provide a structured learning environment and practical experience to enhance comprehension. A wide range of courses on subjects including signal processing, machine learning for audio analysis, and speech recognition are available on platforms like Coursera, Udemy, and edX.
Reading pertinent books and research papers can be quite helpful for people who want to learn more than just what is taught in introductory courses. The books "Fundamentals of Music Processing" by Meinard Müller, "Speech and Audio Signal Processing" by Ben Gold and Nelson Morgan, and "Pattern Recognition and Machine Learning" by Christopher M. Bishop are a few that are suggested reading. Reading research articles published in journals such as IEEE Transactions on Audio, Speech, and Language Processing can provide information about the most recent developments in the field of audio analytics.
Through the use of these tools and an all-encompassing learning path that blends literature study with online classes, novices can build a strong foundation in audio analytics and advance their comprehension.
12. Conclusion: Recap of key concepts, importance of continuous learning, and potential career paths in the field of audio analytics
Furthermore, audio analytics is an effective tool that uses a variety of strategies to draw conclusions from sound data, as I said above. Important ideas in the analysis process include spectrograms, machine learning methods, and auditory characteristics. Anyone who wants to work in this profession has to understand these ideas.
Because technology and data are always changing, audio analytics relies heavily on continuous learning. Keeping up with the most recent technologies, trends, and algorithms helps guarantee that professionals continue to be productive and competitive in their career. People can increase their understanding and proficiency in audio analytics by taking online classes, attending workshops, and networking.
Those who are interested in working with sound data have a variety of job options available in the field of audio analytics. There are opportunities in a variety of businesses, including security systems, speech recognition, and music production. Potential job possibilities in this fascinating profession include positions as an audio engineer, data scientist, sound designer, or machine learning engineer, to name just a few. People can find fulfilling careers in audio analytics that fit their interests and objectives if they have the necessary abilities and a passion for sound analysis.