1. Introduction: Setting the stage for how data science is reshaping the finance industry.
The power of data science is transforming how firms function and make decisions in today's financial world. The combination of data science and finance has revolutionized conventional methods, opening the door to creative ideas and more effective procedures. Companies can now use enormous volumes of data to obtain deeper insights, make precise predictions, and successfully manage risks thanks to this dynamic junction.
From algorithmic trading and risk management to consumer behavior research and personalized financial services, data science is fundamentally changing the finance sector. The capacity to derive meaningful insights from intricate datasets has enabled organizations to improve their decision-making procedures and create customized plans that address specific needs more broadly.
The more we learn about the world of finance, the more obvious it is that data science represents a fundamental change in the way financial services are provided and used, rather than merely a technical development. The financial industry's future rests in its ability to use data science to boost productivity, spur innovation, and provide clients with better experiences. With data science at its heart, the finance sector is set for major changes that will influence its future course for many years.
2. Historical Perspective: Exploring how traditional finance has evolved into data-driven finance.
The financial sector has seen a dramatic transformation in recent years as a result of the move away from traditional methodologies and toward data-driven processes. In the past, experience, intuition, and a scant review of the available data were the main factors considered while making financial decisions. But as more sophisticated technology and processing capacity have been available, the area of finance has changed to become more driven by data science.
For the purpose of making investment decisions, traditional finance mostly depended on fundamental analysis, qualitative evaluations, and macroeconomic data. These techniques frequently lacked the accuracy and forecasting ability that modern data-driven systems provide. The demand for advanced analytical tools surged rapidly as financial markets grew more intricate and interconnected over time.
The emergence of machine learning algorithms and big data has completely changed the way financial institutions function. Businesses may now make better decisions, spot trends in market behavior, and proactively reduce risks by utilizing enormous volumes of organized and unstructured data in real-time. This change has opened the door to a new era in which data is the primary catalyst for strategic efforts in all facets of finance, rather than only a result.
Financial professionals may now more precisely understand market dynamics, client behavior, and risk management tactics thanks to data science. Institutions can enhance their trading tactics, identify fraud more efficiently, customize services for clients, and streamline operations for greater efficiency by utilizing quantitative models and predictive analytics. This change emphasizes how important data science is to determining how the finance industry will develop beyond what it is currently.
In summary, the transition from traditional finance to data-driven finance signifies a fundamental paradigm shift in the way industrial decision-making is done. Financial institutions can gain a competitive edge, improve operational agility, and stimulate innovation in a dynamic environment by adopting technological innovations and utilizing data science tools and approaches. The convergence of data science and strategic insight will influence the future of finance by enabling us to traverse the complexity of international markets with confidence and foresight, as well as by altering our perception of risk and opportunity.🎚
3. Data Science Applications in Finance: Discussing specific examples like algorithmic trading, risk management, and customer analytics.
Data science is transforming the way firms function and make choices in the banking industry. Algorithmic trading is one such application, in which sophisticated algorithms scan enormous datasets for patterns and quickly execute deals. By using this method, businesses can make better investment choices based on data-driven insights rather than gut feeling or feeling.📱
Another area in finance where data science is useful is risk management. Financial organizations may analyze risk more precisely and instantly by using machine learning and advanced analytics approaches. Proactive risk reduction measures are made possible by this, which improves results and increases stability under erratic market situations.🔷
Another important application of data science in finance is customer analytics. Through the analysis of client feedback, preferences, and behavior, institutions can successfully customize their offerings to match the demands of individual customers. This customized strategy increases client happiness and loyalty, which in turn spurs business expansion.
The banking industry is undergoing a transformation because to data science applications like algorithmic trading, risk management, and consumer analytics, which use data to spur innovation, efficiency, and competitiveness.♌️
4. Importance of Big Data: Highlighting the role of big data in revolutionizing financial services.
The way that the financial services industry is evolving is greatly influenced by big data. Institutions have never-before-seen potential to glean insights, enhance processes, and develop ground-breaking products thanks to the enormous amount, velocity, and variety of data generated today. Financial institutions can improve risk management procedures, tailor consumer interactions, more efficiently identify fraudulent activity, and make data-driven decisions that propel company expansion by utilizing big data analytics.
The potential of big data to offer a more complete picture of customers' demands, interests, and behaviors is one of the industry's main advantages. Businesses may better serve each client by customizing their offers thanks to this comprehensive insight, which increases client loyalty and happiness. Financial institutions can more precisely segment their customer base and target particular groups with tailored marketing efforts thanks to big data analytics.
Big data is essential to improving risk management in the banking industry. Institutions are able to recognize patterns, trends, and anomalies that indicate possible dangers by instantaneously evaluating enormous volumes of both structured and unstructured data. Organizations can more successfully minimize risks, adhere to regulatory obligations, and prevent fraud by using this proactive strategy.
Based on the aforementioned, we may infer that the financial industry is undergoing a significant transformation as a result of the big data era. Through the utilisation of sophisticated analytics and machine learning algorithms on extensive datasets, enterprises can obtain significant insights that propel innovation, expansion, and competition. Financial organizations that want to prosper in a more competitive and dynamic market environment must embrace big data; it is no longer a choice.
5. Challenges and Opportunities: Addressing the hurdles faced and opportunities presented by integrating data science in finance.
Challenges and Opportunities Integrating data science into finance presents both challenges and opportunities. One significant challenge is the need for robust data governance and quality control, as financial decisions rely heavily on accurate and reliable data. Ensuring the integrity of data sources, managing vast amounts of information, and maintaining data privacy are critical hurdles that must be overcome to fully leverage the power of data science in finance.
A change in organizational culture is necessary for the incorporation of data science in the banking industry. In order to extract meaningful insights from data, financial professionals must adopt new technologies and processes and adopt a more analytical approach. Traditionalists who prefer traditional techniques may oppose this transition, underscoring the significance of appropriate change management strategies.
Notwithstanding these difficulties, there are a plethora of potential when integrating data science into finance. Financial organizations can improve risk management procedures, more efficiently detect fraud, and optimize investment strategies by employing sophisticated analytics techniques like machine learning and artificial intelligence. Through predictive analytics, data science also makes individualized consumer experiences possible, increasing customer loyalty and pleasure.
The application of big data analytics to finance presents previously unheard-of chances for creativity. Financial institutions can obtain a competitive advantage by quickly adapting to consumer preferences and market changes by utilizing real-time data processing and analysis. In addition to improving decision-making procedures, this agility opens up new revenue opportunities by diversifying products and customizing services based on detailed consumer data.
As I mentioned above, there are obstacles to overcome in the integration of data science with finance, but they are greatly outweighed by the potential that arise. Financial organizations may increase client value by operating more effectively, making decisions based on real-time insights, and embracing data science. Organizations that embrace this change stand to prosper in a constantly shifting field as technology and data-driven approaches become more and more integrated into the future of finance.
6. Regulatory Implications: Analyzing how regulations are adapting to ensure ethical use of data science in finance.
The adaptation of legislation to address the ethical application of data science in finance is a growing area of interest for regulatory agencies. Regulators must make sure that data-driven procedures are equitable, open, and comply with current regulations as data becomes more and more essential to financial decision-making. As a result, new policies and guidelines regulating the application of algorithms and machine learning in financial services have been created.
The possibility for bias in algorithmic decision-making is a major topic of concern. To avoid discriminatory results, regulators are closely monitoring the training and application of algorithms in the finance industry. They are advocating for increased openness on the model methodology, data sources, and effects on various client categories. Regulators hope to ensure that data science is applied ethically, fairly, and without maintaining disparities by closely examining these factors.
Regulations pertaining to privacy and data protection will be vital in determining how data science develops in the banking industry. Regulators are imposing stringent regulations to protect consumer privacy rights as financial companies gather and analyze a growing quantity of sensitive personal data. Financial organizations using data science now need to comply with regulations like the CCPA and GDPR, as non-compliance can result in significant fines and reputational harm.
All of the aforementioned points point to the necessity of comprehending and abiding by changing regulations for the long-term application of data science in finance. Financial firms need to give ethical considerations top priority in their data-driven initiatives as regulators adjust to new technology developments in order to gain the trust of both customers and regulatory bodies. High standards of integrity and fairness can be upheld in the data-driven finance of the future by adopting ethical practices and keeping up with legislative changes.
7. Future Trends: Predicting potential advancements and innovations that will shape the future of finance through data science.
Data science has the potential to completely transform the way firms function and make decisions in the finance industry. A number of themes are anticipated to influence how data science will be used in finance in the future.
The growing use of machine learning algorithms and artificial intelligence (AI) in financial services is one trend. These technologies enable more precise risk assessment, fraud detection, and trading strategies by analyzing massive amounts of data at rates faster than human comprehension.
The use of predictive analytics in personalized finance is another trend. Financial institutions can provide customized services and products to individual clients by utilizing machine learning models and historical data, which are based on the customers' distinct demands and behavior patterns.
Blockchain technology is probably going to have a big impact on how safe and open transactions are done in the financial industry. Its decentralized structure lowers expenses and speeds up cross-border transaction processing while improving security.
Scalability will be essential for financial firms to effectively extract meaningful insights from the exponential growth of big data. In order to manage and analyze large datasets, sophisticated data processing methods like cloud computing and edge computing will become more and more important.
Essentially, the financial industry's future rests in its ability to leverage data science technology to propel innovation, improve decision-making procedures, and provide customers with tailored experiences in a quickly changing digital environment.
8. Impact on Investment Strategies: Examining how data science is changing investment decision-making processes.
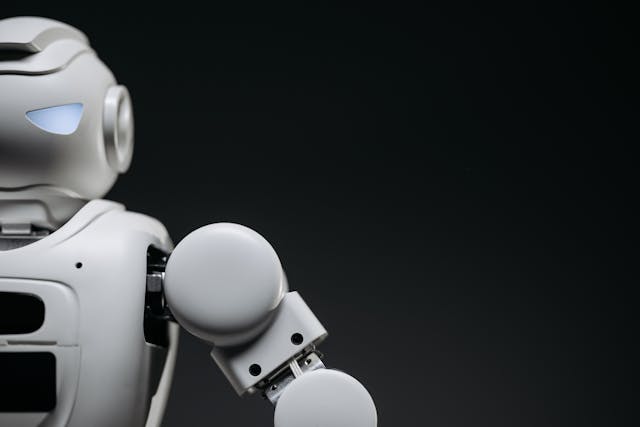
Investment strategies are being revolutionized by data science because it is enabling rapid and effective analysis of large amounts of data. Historically, historical trends and qualitative analysis have constituted a major basis for investment decisions. Investors may now use algorithms and machine learning models to quickly search through massive datasets for insightful information thanks to the development of data science. This has resulted in data-driven, better-informed financial decisions that may increase profits while lowering risks.
Finding patterns and trends that conventional approaches might miss is a crucial component of using data science in financial strategy. Investors can better predict market moves and find correlations between different elements by utilizing advanced analytics approaches. Proactive decision-making made possible by this predictive skill enables investors to quickly modify their positions in response to shifting market conditions.
Investors can now customize their strategies according to their own risk tolerance and preferences thanks to data science. Financial institutions are able to provide customized investment solutions that are in line with the goals and objectives of their clients by analyzing customer data and behavioral trends. Through the optimization of asset allocation tactics, this customized strategy not only increases customer happiness but also improves portfolio performance overall.
To put it succinctly, the adoption of data science in investment strategies represents a dramatic change toward a more flexible and agile method of making financial decisions. In an increasingly complicated and volatile market environment, investors can gain a competitive edge by utilizing the power of big data analytics and machine learning algorithms. Financial professionals can make better, evidence-based decisions that benefit their clients and themselves by embracing data science.
9. Role of AI and Machine Learning: Delving into the significance of artificial intelligence and machine learning in transforming financial operations.
AI and machine learning play a critical role in finance, transforming operations through insights derived from data. Large volumes of data may be quickly and reliably analyzed by these technologies, improving risk assessment, fraud detection, and customized consumer experiences. AI systems in investment management are able to identify trends in market data and use that information to make wise judgments. By optimizing trading tactics based on past data, machine learning models can help fintech companies maintain their competitiveness. In the finance industry, artificial intelligence and machine learning improve decision-making and expedite procedures.
10. Cybersecurity Concerns: Discussing the rising importance of cybersecurity measures in safeguarding financial data in a data-driven world.
Cybersecurity is critical in the data-driven financial industry. Financial institutions now depend more on data science, which puts them at higher danger from cyberattacks. Preserving confidential financial data has become crucial in order to avert security breaches and uphold client confidence. Advanced cybersecurity measures must be integrated in order to defend against harmful assaults that could jeopardize important data.
The banking sector is particularly vulnerable to cyberattacks in the connected digital world of today. Hackers are always changing their strategies to take advantage of weaknesses in banking systems, from ransomware assaults to phishing scams. As data science is essential to reshaping the financial industry, it is imperative to have strong cybersecurity protections.
Financial institutions are using cutting-edge technology like artificial intelligence and machine learning for real-time danger detection and analysis in order to successfully reduce these risks. Institutions can proactively identify possible security breaches and take preventive measures before any substantial damage happens by utilizing predictive analytics. Strongening cybersecurity in a data-driven world also requires putting multi-factor authentication and encryption measures into practice.
There is an increasing demand for comprehensive cybersecurity standards as financial transactions move more and more into digital platforms. Strengthening defenses against new cyber threats can be achieved by emphasizing a proactive approach to cybersecurity through ongoing monitoring, frequent audits, and employee training. In this data-driven era, strong cybersecurity measures protect not only sensitive financial data but also the integrity and stability of the entire financial ecosystem.
11. Education and Skill Development: Addressing the need for specialized skills and education in preparing professionals for the future of data-driven finance.
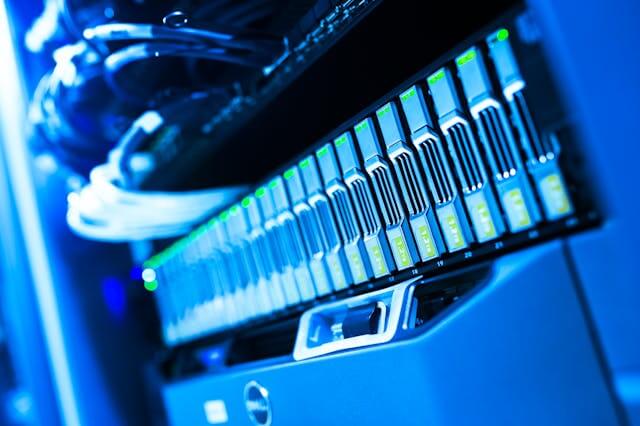
A major factor in determining how data-driven finance develops in the future is education and skill development. Professionals with specific knowledge in data science, analytics, machine learning, and related subjects are in greater demand as the sector develops. Educational establishments and training programs are modifying their curricula to include these crucial abilities in order to satisfy this demand.
In order to prosper in the data science era, finance professionals need to develop a strong grasp of machine learning algorithms, statistical analysis, programming languages such as Python and R, and data visualization techniques. With the use of these abilities, they are able to glean insightful information from voluminous financial data and make deft decisions that foster innovation and corporate expansion.
Upskilling and constant learning are essential for being competitive in the quick-paced world of data-driven finance. Professionals who want to further their skills and maintain their competitiveness in the job market can pursue postgraduate degrees, certificates, or specific courses in data science. People can position themselves as valuable assets to companies looking to use data for strategic decision-making by investing in their education and skill development.
For finance professionals looking to advance their careers, embracing lifelong learning and gaining specific knowledge in data science are crucial first steps. People can significantly contribute to the transformation of the financial sector through data-driven insights and open up new career progression prospects by staying ahead of industry trends and mastering cutting-edge tools and technology.
12. Conclusion: Summarizing key points and emphasizing the transformative potential of data science in shaping the future of finance.
Taking everything into consideration, we can say that data science will undoubtedly play a major role in the future of finance. By improving fraud detection, risk management, decision-making processes, and customer experience, data science has completely transformed the financial sector. By combining artificial intelligence, machine learning, and advanced analytics, financial institutions are now able to extract priceless insights from massive volumes of data.
Financial organizations can increase overall efficiency, personalize services, and optimize operations by utilizing data science techniques. Data science's predictive skills enable firms to anticipate market trends, proactively manage risks, and develop creative solutions that cater to changing client demands. Adopting a data-driven strategy increases profitability and encourages innovation in the banking industry.
Data science has the potential to revolutionize the financial industry since it can lead to strategic adjustments that adjust to the ever-changing business environment. Using data science to its fullest potential will be essential to remaining competitive and relevant in the digital age as technology continues to advance quickly. Financial institutions may set themselves up for long-term success by supporting a data-centric culture at all organizational levels and investing in personnel with experience in data analytics. Adopting data science is essential to reshaping the financial industry, not merely a passing fad.