1. Introduction:
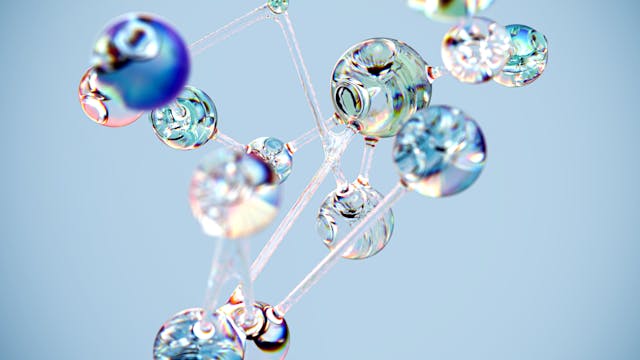
Within contemporary technology, data scientists and data engineers are considered to be formidable beings capable of deciphering the mysteries enshrouded in enormous volumes of data. In "Fantastic (Data)-Beasts and Where to Find Them: Data Scientists and Data Engineers," our blog article explores the world of these talented individuals who are fundamental to the development of our digital environment.
The masters of data analysis, data scientists use their knowledge to glean important insights from large, complicated databases. They have a miraculous capacity to see patterns, trends, and important information that can spur innovation and strategic business decisions. Data engineers, on the other hand, are the unseen architects who create and manage the reliable infrastructure needed to enable data processing, storing, and retrieval.
Data scientists and engineers are vital resources for businesses trying to leverage big data in today's fast-paced technology environment. These experts help companies use data-driven tactics to achieve a competitive edge, streamline operations, and spur growth thanks to their distinctive skill sets and analytical aptitude. Join us on this exciting adventure as we explore the fascinating fields of data science and engineering.
2. Unveiling the Fantastic Beasts - Data Scientists:
Unveiling the Fantastic Beasts - Data Scientists:
The potential of intricate datasets can only be realized with the help of data scientists. Their specialty is drawing insightful conclusions from unprocessed data to guide strategy and decision-making. These experts have a combination of programming, statistics, mathematics, and domain knowledge.
A solid background in statistical analysis, machine learning, data visualization, and programming languages like Python or R are prerequisites for becoming a successful data scientist. The ability to think critically and solve problems is necessary for efficiently evaluating large volumes of data and drawing insightful conclusions.
Data scientists use a variety of methods to extract insights from unprocessed data. They employ machine learning algorithms to forecast future results or provide suggestions, statistical models to uncover patterns and trends in datasets, and data visualization tools to effectively convey their findings. Data scientists enable firms to make well-informed decisions and achieve a competitive edge in today's data-driven world by converting complex data into actionable insights.
3. Taming the Beasts - Data Engineers:
Building and maintaining the data infrastructure, which is the foundation of any company driven by data, is a critical task for data engineers. They develop, build, and oversee pipelines that effectively gather, process, and store data as part of their work. Data scientists wouldn't have access to the high-quality data necessary for insights if they didn't have strong data engineering foundations.
One needs to be very proficient in programming languages like Python, Java, or Scala in order to succeed in a profession in data engineering. Being proficient with databases, especially SQL, is essential for efficiently managing massive datasets. Comprehending big data frameworks such as Hadoop or Spark is imperative for effectively handling vast quantities of data.
By enhancing database performance, putting ETL (Extract, Transform, Load) procedures into place for efficient data flow, and working with other teams to create solid architecture that can expand to meet the needs of the business, data engineers guarantee the scalability and efficiency of data systems. Their knowledge ensures that when data is required for analytics or business operations, it can be accessed promptly and consistently.
4. The Hunt for Talent:
The need for qualified data scientists and engineers is growing across a wide range of businesses in today's data-driven world. These gifted people are in high demand by organizations, which need their assistance in unlocking the potential of their data. But with such great demand also come great rivalry and difficulties in attracting and keeping the best people in the industry.
One of the biggest issues faced by firms in acquiring top talent is the scarcity of experienced individuals. The demand and supply for qualified workers in these positions are now out of balance due to the data science and engineering fields' explosive expansion. As a result, hiring managers frequently have trouble locating applicants who possess the ideal blend of subject knowledge, technical proficiency, and problem-solving skills.
Organizations must take deliberate measures in order to overcome these obstacles and draw in elite professionals. In a competitive job market, it is imperative to maintain your appeal by providing competitive pay and benefits packages. Offering chances for professional improvement, like training courses and certificates, can assist companies in keeping talent by making an investment in their advancement within the firm.
Attracting talented data scientists and engineers also requires developing an innovative and collaborative work environment. Businesses can differentiate themselves by encouraging a culture that prioritizes diversity, creativity, and lifelong learning. Prominent initiatives and state-of-the-art technologies can attract experts seeking novel challenges and chances to truly change the world.
Furthermore, as I mentioned above, while finding skilled data scientists and engineers may be difficult for businesses, doing so can help them stand out from the competition. Companies can recruit and retain top personnel in the fast-paced field of data science and engineering by providing competitive salary packages, emphasizing professional development, creating a positive work environment, and showcasing fascinating projects.
5. Tools of the Trade:
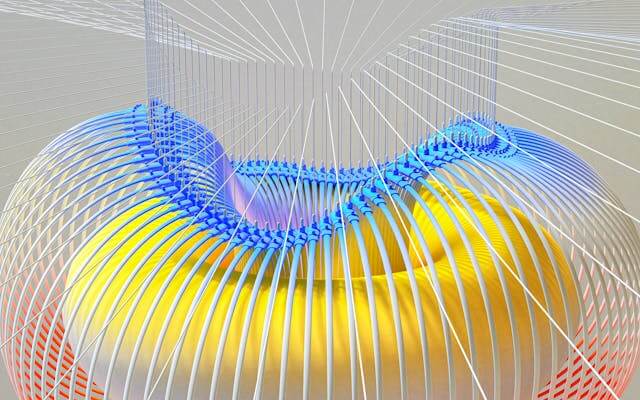
A multitude of technologies are employed by data scientists and data engineers to traverse the huge terrain of big data. With strong libraries like NumPy and Pandas, Python and R are among the most popular programming languages for handling data analytic jobs. While distributed computing is made possible by technologies like Hadoop and Spark, SQL is essential for effectively querying databases. These technologies are essential for optimizing data workflows, from gathering and preparing datasets to carrying out intricate analysis and producing perceptive visuals. Professionals in the field may effectively and efficiently extract significant insights from big data with these technologies at their disposal.
6. From Lab to Boardroom:
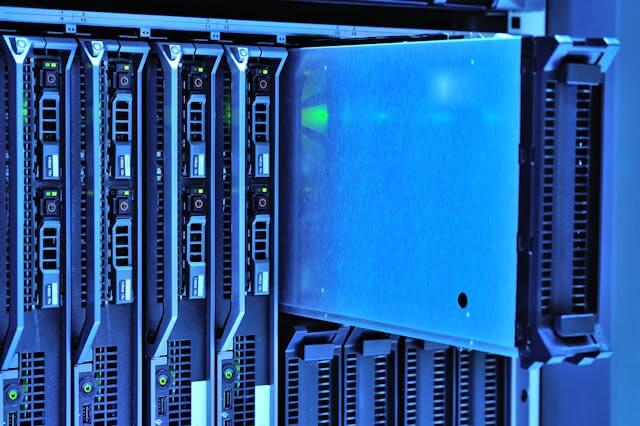
The transfer of ideas from the laboratory to the boardroom is a genuinely revolutionary process in the dynamic realm of data-driven decision making. Data scientists are essential to this process because they use their analytical skills to mine raw data for insightful information. These insights are fascinating narratives that reveal stories hidden within the data, not just numbers or graphs. 📗
Data scientists utilize storytelling with data as a strong tool to convey complicated discoveries in an understandable and interesting way. They simplify complex patterns and trends by creating narratives around the data, which enables corporate stakeholders to understand the implications and take appropriate action. These stories play a crucial role in bridging the gap between data analysis and practical company strategies, whether it is through market opportunity identification, operational process optimization, or customer behavior prediction.
That being said, one does not traverse the path from insight to influence alone. To fully utilize these insights, cooperation between data scientists, engineers, and business stakeholders is essential. In order to ensure that insights are obtained correctly and effectively, data engineers are essential in creating a solid architecture that facilitates seamless data processing and analysis. Conversely, business stakeholders offer subject knowledge and strategic guidance, converting insights into choices that actually affect change inside a company.
The implementation of the project will only be successful if these varied teams work well together. Transforming ideas into real outcomes requires regular communication, shared understanding of objectives, and shared ownership of results. Data scientists may find important insights and make sure those insights are used wisely to inform critical business choices when they collaborate closely with engineers and business stakeholders throughout the project lifecycle.
In summary, the journey of data scientists and engineers—fantastic (data)-beasts—from the lab to the boardroom serves as an example of the value of teamwork in utilizing data for tactical advantage. Through the use of data to create stories and the development of strong cross-functional partnerships, businesses may fully utilize their data assets to make decisions that will lead to success in the current competitive landscape.
7. Navigating Ethical Challenges:
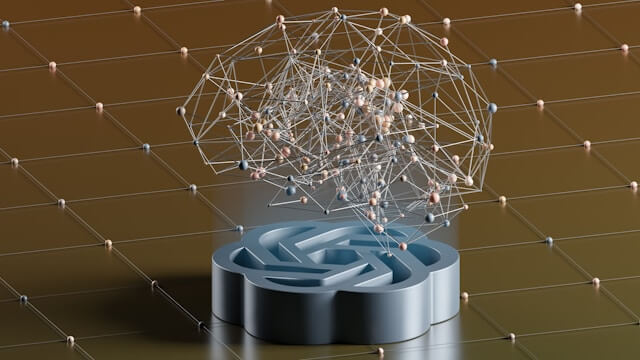
For data workers, navigating ethical concerns in the big data consumption space is essential. Algorithm bias and privacy issues are two important ethical issues that require close analysis. To preserve ethical norms, data scientists and technologists need to be watchful about safeguarding private information and reducing algorithmic bias.🙃
Data workers have a lot of responsibilities when it comes to ensuring that data-driven technologies are used ethically. They are essential in creating, putting into practice, and managing systems that adhere to moral principles. To gain the trust of users and stakeholders, data practices must uphold accountability, fairness, and transparency.
It is critical for data scientists and engineers to be up to date on developing ethical problems and to engage in continuous learning in the rapidly changing field of technology. These specialists can help ensure a more sustainable and ethical use of data in the current digital era by proactively addressing potential hazards and cultivating a culture of ethical awareness.
8. Mastering the Art of Continuous Learning:
Acquiring proficiency in the discipline of continuous learning is essential for remaining current in the rapidly evolving fields of data science and engineering. Professionals in this industry need to continuously upskill in order to stay up with the rapid evolution of technology. Conferences, workshops, and online courses are excellent sources for learning new techniques and remaining up to date with data science and engineering trends. By making time for continuous learning, professionals may hone their skills and stay competitive in this fast-paced field.
9. Beyond Borders: Global Perspectives on Data Science:
The global scene in data science and engineering is a vibrant tapestry of many techniques and viewpoints. Different parts of the world are taking use of data science innovations in ways that are specific to their cultural influences and methods of managing, evaluating, and interpreting large-scale datasets.
Data science innovation is leading the way in North America, where tech behemoths like Silicon Valley are driving forward-thinking advancements in the field. The area is excellent at building a vibrant ecosystem that supports innovative studies and cutting-edge data analytics technology. Its culture of entrepreneurship drives an unwavering search for data-driven solutions in a variety of sectors, including healthcare and finance.
As we move eastward to Asia, we see how tradition and technological innovation are combining to shape the data science scene. In order to spur innovation, nations like China and India are embracing AI and machine learning technology at a rapid pace. Their approaches to using big data for societal improvement are deeply rooted in cultural norms that emphasize teamwork and comprehensive problem-solving.
In the field of data science, Europe has a rich tapestry of historical influences that come together with a dedication to scientific rigor. Reputable nations like the UK and Germany are known for emphasizing accuracy and high-quality engineering techniques in data processing. European countries, with a cultural legacy rooted in privacy and transparency, frequently give ethical issues top priority when handling sensitive datasets.
Data science is being applied more and more on the African continent to tackle complicated issues in fields like agriculture and healthcare. Innovative uses of mobile technology are transforming underprivileged people' access to vital information in places like South Africa and Kenya. Initiatives that stress inclusivity and empowerment through data-driven solutions are greatly influenced by cultural diversity.
Data science advances with an emphasis on social effect are being driven by a rising digital ecosystem throughout Latin America. Big data is being used by nations like Brazil and Mexico to address challenges like environmental sustainability and urban development. The emphasis on human-centric design thinking brought about by cultural influences gives these programs a people-first strategy that puts community involvement and sustainable development first.
The way that technical advancements and cultural quirks are coming together all around the world is changing data science and engineering. Through the adoption of varied viewpoints and techniques derived from regional customs, communities across the globe are discovering novel avenues for utilizing big data to effect significant transformations.
10. Harnessing AI and Machine Learning:
The way organizations study and use data has changed dramatically in the data-driven world of today thanks to the current Data Scientists and Engineers' incorporation of AI and machine learning methodologies. These experts can extract insightful information, forecast results, and automate decision-making processes thanks to AI and ML algorithms. Data scientists use artificial intelligence (AI) to create sophisticated models that can interpret enormous volumes of data. Data engineers, on the other hand, are responsible for creating the framework required to support these models and guarantee smooth data flow for analysis.
Applications in the real world clearly demonstrate the amazing potential of AI and ML for predictive analytics. For example, in e-commerce, machine learning algorithms are used to power recommendation systems that study user activity and make product recommendations based on individual tastes. Based on past data, predictive analytics algorithms in the healthcare industry can predict patient diagnoses or treatment outcomes. Artificial intelligence is used by fraud detection systems in the banking industry to identify suspicious activity and provide proactive protection against financial crimes.
Businesses across a range of industries are discovering new opportunities thanks to the partnership of data scientists and data engineers in the application of AI and ML approaches. Organizations that understand these cutting-edge technologies stand to benefit from increased competitiveness, increased creativity, and better decision-making thanks to predictive insights gleaned from massive amounts of data.
11. Future Horizons: Emerging Trends and Technologies:
It is critical to consider the upcoming trends and technologies that will influence data science and engineering as these professions continue to change and evolve. The increasing focus on automation and AI ethics is one such trend that is anticipated to have a big influence. The use and usage of artificial intelligence raises ethical issues that must be addressed as technology develops. In contrast, automation is designed to optimize workflows and boost productivity when managing large datasets.
The way that distributed computing is changing career paths in data-related fields is another fascinating trend to watch. Due to the exponential growth of data, distributed computing provides effective ways to process large amounts of data. Data scientists and technologists now have more chances and obstacles to overcome as they figure out creative ways to draw conclusions from decentralized data sources.📚
Future-gazing introduces us to innovative technologies such as Big Unstructured Data and Quantum Computing. By utilizing quantum processes to do sophisticated computations at previously unheard-of rates, quantum computing has the potential to completely transform data processing capabilities. Big Unstructured Data makes it possible to analyze a variety of datasets without the need for pre-established models or schemas, leading to more comprehensive insights into enormous volumes of unstructured data.
A noteworthy development in these emerging technologies is the growing trend of data science and engineering experts developing generalist skill sets. It is expected that there would be a significant increase in demand for people in these professions who are skilled in a variety of disciplines and have a broad range of talents. Proficiency in a variety of areas, such as statistical modeling and programming languages, will be more and more necessary to remain competitive in the face of changing employment demands.
As businesses realize how important effective data management is to their success, marketplaces dedicated to providing data-related services should grow. These platforms will give users access to a pool of knowledgeable experts, instruments, and resources that are suited to certain requirements like the creation of machine learning algorithms, predictive analytics, or data visualization services.
Taking into account everything mentioned above, we can draw the conclusion that a key to successfully navigating the ever-changing field of data science and engineering is keeping up with new developments in areas such as AI ethics automation, distributed computing, quantum computing, big unstructured data exploration, and generalist skill development. Accepting these revolutionary technologies not only advances career advancement but also gives people the flexibility they need to survive in a world where data is used more and more.
12. Conclusion:
The field of data science and engineering is an intriguing one, home to a wide range of strong and interesting beings known as data scientists and engineers. We have examined the distinct qualities, functions, and abilities of these experts who are essential in turning unprocessed data into insightful knowledge. Data Engineers create the framework required for these discoveries to flourish, while Data Scientists have the magic touch to find patterns in data and create captivating tales from it.
It's evident that opportunities exist in this dynamic area for people with a passion for problem-solving, analytics, and creativity as we come to the end of our examination of these amazing (data)-beasts. There is a place for you in the always changing field of data science and engineering, regardless of your hobbies, which can include deciphering intricate algorithms or building reliable systems that guarantee data integrity. Seize the opportunity to explore this fascinating field where every dataset is a possible source of discovery and change. Develop your abilities and embrace your curiosity. The data world is waiting for you; are you up to the difficulties it presents?