1. Introduction
A new method to data management called Data Mesh signifies a change in how businesses arrange and use their data. Conventional data designs frequently concentrated data inside monolithic systems, which made collaboration, scalability, and adaptability difficult. By supporting a decentralized strategy that treats data as a product and is managed by cross-functional teams, Data Mesh seeks to address these problems.
The volume and diversity of data are growing dramatically in the current big data landscape due to rapid technological breakthroughs. Effective information processing and comprehension necessitate new approaches in light of the explosion in data complexity. With the help of Data Mesh's design, businesses may take advantage of dispersed resources while encouraging team accountability and autonomy. Data Mesh facilitates more effective teamwork with data by dismantling data silos and promoting domain-driven ownership, which is in line with the demands of individual businesses.
2. Evolution of Data Architecture
Traditional centralized data architectures have given way to decentralized, domain-oriented ones in the evolution of data architecture. Centralized data architectures, in which every piece of data was kept and controlled by a single, monolithic system, were popular in the past. However, this strategy became ineffective and difficult to maintain as the volume and complexity of data in businesses increased.
A change toward more domain-oriented, decentralized systems resulted from this difficulty. This paradigm allows for more scalability and agility by distributing data across multiple domains or business units. Since each domain owns its own data, the data is more aligned with business requirements and of higher quality.
The idea of Data Mesh evolved in reaction to these modifications in data architecture. According to the Data Mesh paradigm, managing data inside an organization should be approached like a product, with decentralization and domain-driven design principles being applied. It recommends dismantling data silos, giving domain teams ownership and management authority over their data, and offering standardized platforms and tools for governance and communication.
In managing contemporary data landscapes, there is an increasing requirement for flexibility, scalability, and alignment with business objectives. This is shown in the movement from classic centralized data architectures to decentralized, domain-oriented structures. For businesses trying to fully utilize their data assets and successfully manage these challenges, the Data Mesh method provides a viable framework.
3. Core Principles of Data Mesh
A Data Mesh framework's ability to successfully alter data management within enterprises is largely dependent on its guiding principles. To begin with, domain-oriented decentralization places a strong emphasis on dismantling data silos according to particular business domains, enabling improved data ownership and governance. By ensuring that data is handled by cross-functional teams accountable for distinct domains, this method enhances the general quality and usability of data.
Second, teams can access and use data independently without heavily depending on centralized IT departments thanks to self-serve data infrastructure. Through the provision of tools and platforms that enable users to independently discover and manipulate data, businesses promote creativity and agility within their data operations.
Thirdly, while preserving general consistency and adhering to organizational rules, the federated governance model makes sure that decision-making authority is divided across several domains. This strategy encourages effective coordination and collaboration among heterogeneous teams by striking a balance between autonomy and alignment.
Finally, treating data as a valued product that generates real business value is encouraged by adopting a product thinking attitude. Organizations can improve overall data culture, stimulate innovation, and drive better results by concentrating on providing high-quality, useable data products that are customized to meet the demands of particular end users.
4. Implementing Data Mesh: Key Components
In implementing a Data Mesh framework, several key components play pivotal roles in its success.
Data goods are crucial, to start with. These are filtered and selected data sets for certain business requirements. These data products efficiently satisfy domain teams' objectives because of their precise definition and efficient creation procedure.
Second, with a Data Mesh architecture, data ownership is essential. It promotes responsibility and competence by giving domain teams ownership of their data sets. Better results result from teams taking ownership of the usability and quality of their data according to this ownership model.💍
Federated data governance is the final tool used to guarantee uniformity and compliance throughout the company. This method offers standards and rules that support data security, integrity, and general quality assurance inside the Data Mesh ecosystem while decentralizing governance activities.
5. Benefits of Adopting a Data Mesh Approach
Using a Data Mesh method provides businesses with a number of important advantages. The scalability and agility it offers in data management is among its main benefits. Data Mesh facilitates faster adjustments to changes in data requirements and more effective scaling of data infrastructure to meet increasing demands by decentralizing data ownership and processing.
An further noteworthy advantage is the enhancement of data accessibility and quality. Data sets are divided into more manageable, domain-specific chunks under a Data Mesh architecture, each overseen by a separate team. A culture of accountability and ownership over data quality is fostered within each team by this decentralized strategy, and as a result, data quality is generally improved throughout the business. Organizations can gain insights more quickly and make better decisions by facilitating easier access to data for the relevant teams that are directly in charge of using it.
Using a Data Mesh method helps cross-functional teams collaborate more effectively. Through the dismantling of data silos and fostering communication across diverse domain teams, Data Mesh fosters knowledge exchange and cooperative efforts on data initiatives. In addition to fostering greater creativity, this collaborative atmosphere aids in the removal of organizational obstacles, which frequently obstruct the efficient use of data across departments.
6. Challenges in Implementing Data Mesh
Organizations frequently encounter difficulties while deploying a Data Mesh, including overcoming cultural hurdles and working with old systems. Cultural impediments may manifest as thought silos within departments, opposition to change, or a lack of data literacy. Strong leadership support, the development of a data-driven culture, and ongoing training and education expenditures to encourage teamwork and understanding are all necessary to overcome these obstacles.
Adoption of Data Mesh is also significantly hampered by legacy systems and procedures. These antiquated systems can make it difficult to combine them with contemporary data infrastructure or get in the way of a data mesh's ability to scale and adapt. In order to close the gap between historical systems and the specifications of a Data Mesh architecture, organizations must evaluate their current systems, create migration plans, and maybe make investments in new technologies. For a Data Mesh framework transition to be successful, it is imperative to find a balance between adopting new technologies and maximizing the returns on current investments.
7. Real-World Examples of Successful Data Mesh Implementation
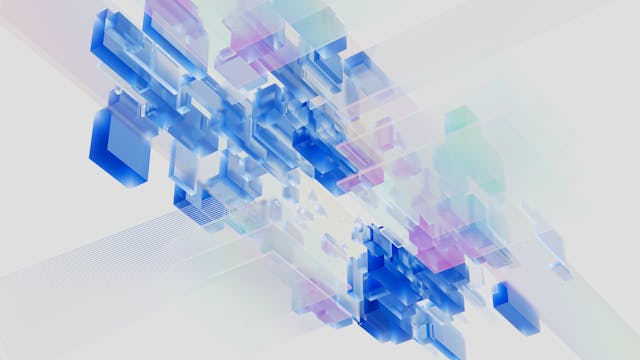
Real-World Examples of Successful Data Mesh Implementation
Organizations aiming to update their data architecture may find it quite difficult to implement a Data Mesh framework. In order to have a deeper comprehension of the real-world uses of this idea, let us examine a few instances of effective Data Mesh implementations.
1. **Netflix**:
Netflix is widely recognized for its inventive utilization of data to inform content suggestions and enhance user satisfaction. Netflix has decentralized its data infrastructure and given different business units the autonomy to own and govern their own data domains by implementing a Data Mesh method. The organization's many teams are now able to collaborate better and innovate more quickly thanks to this deployment.
2. **Wealth Management Firm XYZ**:
Wealth Management Company XYZ used a data mesh design to solve the problems caused by disparate data systems in its insurance, investment banking, and wealth management sectors. Wealth Management Firm XYZ witnessed improved data quality, expanded analytics capabilities, and higher agility in responding to changing market conditions by introducing a federated data model where domain-specific data products are owned and administered by particular domain teams.
3. **E-commerce Company ABC**:
ABC, an online retailer, used a data mesh approach to solve scalability problems brought on by the volume of transactional and customer data it was collecting on a daily basis. By emphasizing the development of self-serve data products overseen by subject matter experts, E-commerce Company ABC enabled its workforce to provide insights more quickly and independently. This change allowed for more individualized customer experiences with focused marketing campaigns and product recommendations, in addition to increased operational efficiency.
These examples show how businesses in a variety of sectors have effectively adopted Data Mesh frameworks to maximize the value of their data assets and promote a cross-functional team culture of autonomy, cooperation, and creativity.
Businesses hoping to start their own Data Mesh journey can learn a lot from these real-world examples, including best practices, typical implementation problems, and the revolutionary effects that adopting a decentralized approach to data management can have on organizational growth and competitiveness in today's data-driven environment.
7.1 Company A: How they transitioned to a Data Mesh architecture
Business The first step in A's move to a data mesh architecture was realizing the shortcomings of their conventional centralized data strategy. Acknowledging the necessity for increased flexibility and expandability, they set out to put a Data Mesh structure into place. Forming cross-functional teams with domain specialists in charge of data management inside their respective disciplines was the first step. These groups were given the authority to control and own the data they generated, guaranteeing its accuracy and applicability.
Company A made a significant investment in creating a solid data infrastructure that enabled easy integration and access to dispersed data sources in order to support this new decentralized model. In order to create a more responsive and effective data ecosystem, they implemented technologies like Apache Kafka for streaming data intake and Apache Flink for real-time processing. The use of a Data Mesh architecture promoted experimentation and discovery within each area, which not only enhanced data quality but also stimulated innovation.
Company A gave top priority to creating transparent data governance procedures as part of their shift to guarantee adherence to rules and guidelines in all areas. They used technologies like metadata libraries to trace data consumption and lineage, and they put in place standards for data sharing, security, and privacy. This proactive strategy guaranteed accountability and transparency across the entire business in addition to enhancing trust in the data.
The effective implementation of a Data Mesh architecture by Company A has completely changed how they utilize and manage their data assets. Their adoption of decentralization, empowerment of domain-driven teams, investment in state-of-the-art technology stacks, and implementation of strong governance procedures have positioned them for sustained success in an increasingly data-driven global environment.
7.2 Company B: Implementing a federated governance model
Company B had special possibilities and challenges as they worked to build a federated governance model inside the framework of a Data Mesh architecture. They wanted to decentralize decision-making while upholding governance norms across different data domains, thus they adopted this architecture. By using this strategy, Company B was able to assign tasks to several domain teams, giving them the freedom to independently make data-driven decisions without sacrificing data compliance or quality.
A crucial element of Company B's federated governance framework involved the formulation of unambiguous policies and procedures concerning data ownership, access limits, and compliance obligations. They underlined how crucial it is to establish clear roles and duties within every domain team in order to guarantee transparency and accountability in data operations. Through the establishment of a collaborative and communicative culture among several teams, Company B managed to fortify stakeholder trust and enable them to efficiently manage data at the local level.
Company B realized how important it was to support their federated governance approach with technology solutions. They put in place strong data governance solutions that provide automatic compliance checks, lineage tracking, and visibility into data assets. These technologies helped Company B monitor and uniformly implement governance policies throughout the firm, in addition to facilitating smooth domain team collaboration.🖊
After putting everything above together, we can say that Company B's effective application of a federated governance model inside a Data Mesh architecture shows the value of centralized governance principles combined with decentralized decision-making. Through the delegation of authority to domain teams and the maintenance of shared standards, Company B was able to fully realize the value of its data assets and stimulate innovation throughout the company. This strategy creates a strong basis for scalable and long-term growth in the dynamic field of digital transformation, while simultaneously encouraging agility and flexibility in data management.
8. Future Trends in Data Management with Data Mesh
Data Mesh is becoming more and more popular as a viable strategy as data management keeps changing. With this decentralized design, distributed domain-oriented data ownership becomes more important than centralized data lakes or warehouses. Data Mesh seeks to enhance data analytics' scalability, flexibility, and autonomy by dividing up data ownership among several domains.
The use of AI and machine learning technology is one emerging trend in data management with Data Mesh. Within the Data Mesh framework, utilizing AI can improve data processing capabilities, automate data quality checks, and optimize data pipelines. Organizations may make better decisions based on real-time analytics and predictive modeling with the help of AI-driven insights.
The focus on data security and governance in Data Mesh systems is another trend. Strong governance procedures are essential as enterprises use Data Mesh to decentralize their data infrastructure. It will be crucial to put in place uniform standards for encryption, access control, and compliance rules in order to safeguard sensitive information and enable smooth cross-domain cooperation.
We expect to see an increase in platforms and solutions designed specifically for managing Data Mesh topologies. These tools will solve issues including managing metadata across domains, tracking dispersed pipelines, and coordinating intricate operations that are unique to decentralized data ecosystems. Purchasing solutions with specific functions will make it easier to apply Data Mesh concepts and optimize workflows for groups utilizing this paradigm.
To sum up what I mentioned, as long as businesses adopt a decentralized strategy for managing massive amounts of disparate data sources, the future of data management using Data Mesh appears bright. In the era of big data, businesses can discover new avenues for innovation and expansion by utilizing cutting-edge technology like artificial intelligence (AI), giving governance procedures first priority, and implementing specific solutions for Data Mesh environments.📚
8.1 AI and Machine Learning applications in optimizing data mesh ecosystems
An important factor in data mesh ecosystem optimization is the application of AI and machine learning. These technologies aid in the effective management and processing of massive data quantities, allowing businesses to gain insightful knowledge. Businesses may automate data pipelines, enhance data quality, and enable more precise decision-making processes within the data mesh architecture by utilizing AI algorithms.
By safeguarding sensitive data, guaranteeing regulatory compliance, and discovering abnormalities, machine learning models can be included into the data mesh to improve data governance procedures. Within the company, these apps aid in building a more stable and safe environment for managing a variety of facts from various areas.📢
Predictive analytics, based on the interconnected data within the mesh, is made possible by AI-driven technologies. These tools may forecast trends, customer behavior, and business results. This enables businesses to successfully leverage the power of their decentralized data infrastructure, spur innovation, and quickly adjust to changes in the market.
9. Conclusion
From the foregoing, it is clear that comprehending the Data Mesh necessitates adopting a decentralized approach to data design that places a premium on self-serve data infrastructure, domain-oriented data ownership, and a collaborative data culture. Organizations may extend their data capabilities more effectively and give domain teams more autonomy by decentralizing data ownership and governance. The organization can manage and use data more nimbly, flexiblely, and scalablely by moving away from a centralized data lake or warehouse paradigm and toward a Data Mesh framework.
The main ideas covered in this blog post demonstrate how the Data Mesh paradigm offers a fresh perspective on data management in contemporary businesses. The significance of dismantling data silos via domain-oriented architecture, utilizing self-serve data platforms to facilitate democratized data access, cultivating a decentralized innovation and collaboration culture, and putting strong governance mechanisms in place to guarantee consistency and dependability in data usage is emphasized.
Organizations can change their approach to analytics, insights production, and decision-making processes by implementing the Data Mesh model's concepts. This paradigm change facilitates cross-functional collaboration and large-scale innovation while providing a route towards more responsive and agile data processes that closely correspond with the demands and specifications of specific business domains. In today's fast-paced business context, comprehending the Data Mesh means rethinking conventional ideas of centralization in favor of distributed data ecosystems that more effectively support digital transformation efforts.🫡