1. Introduction to Fog Data Analytics:
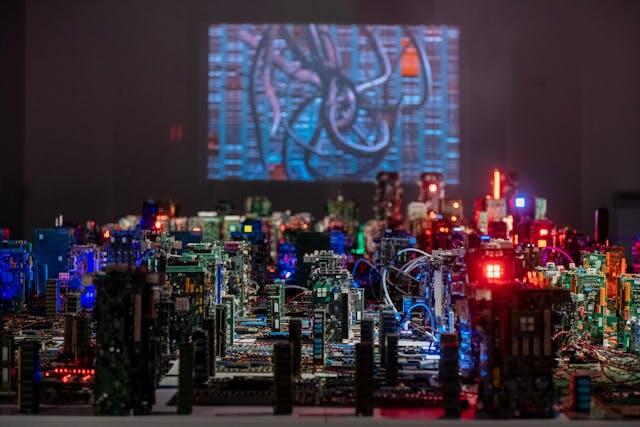
Instead of depending entirely on centralized data processing, fog data analytics analyzes data closer to its source, at the network's edge. By first processing data locally and then transmitting the cleaned data to the cloud for additional analysis, this method allows real-time insights. Fog data analytics is essential for increasing efficiency and response time in the big data era, as huge volumes of data are generated every second. This is because fog data analytics lowers latency and bandwidth utilization.
Fog data analytics is significant because it can handle the problems brought about by the growing amount, velocity, and diversity of data in the modern digital world. Organizations can improve overall operational performance, expedite decision-making, and optimize processes by relocating computing jobs closer to the data source. In addition to improving security and lowering the expense and bandwidth needed to transfer big datasets, this decentralized method enables real-time monitoring and control in crucial applications like autonomous systems and Internet of Things devices.
2. Key Components of Fog Data Analytics:
Fog computing, a decentralized computing architecture that expands the capabilities of the cloud to the edge of the network, is leveraged by fog data analytics. By processing data closer to its source, fog computing lowers latency and boosts productivity. This near proximity facilitates real-time data processing from several sources in fog data analytics, leading to faster insights and decision-making.
Through the integration of fog data analytics with conventional cloud analytics, enterprises may capitalize on the advantages of both methodologies. Fog computing improves real-time data processing at the edge for time-sensitive applications, while cloud analytics offers strong storage and processing capabilities for big datasets over extended periods of time. Through this integration, a more complete and responsive analytics solution that successfully addresses a range of business needs is ensured.
Businesses can build a dynamic and adaptable analytical framework that meets a variety of needs by fusing fog computing with traditional cloud analytics. Businesses can leverage the combined strength of distributed and centralized computing resources thanks to the cooperation of these two elements, which enhances the scalability, dependability, and agility of their data analytics operations.
3. Benefits of Leveraging Fog Data Analytics for Businesses:
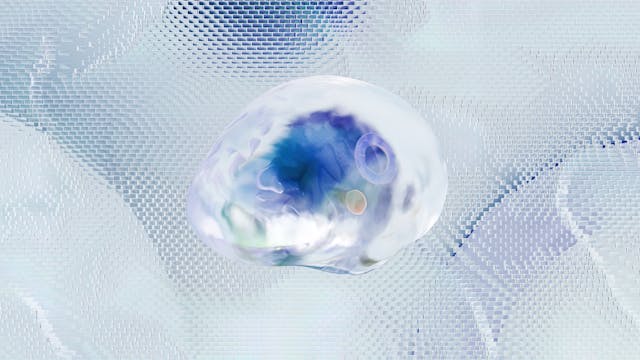
Fog data analytics provides companies with a range of advantages, improving their operations in multiple ways. Making quick, well-informed judgments and gaining real-time insights is one of the main benefits. Organizations may respond swiftly to opportunities and situations that change by processing data closer to the source and analyzing it without depending just on faraway cloud servers. 😀
Businesses may greatly improve data security and privacy by utilizing fog data analytics. There is reduced exposure to security threats during transmission when sensitive data is processed locally at the network's edge rather than being transmitted to a central point. This strategy can guarantee adherence to data privacy laws while assisting in the mitigation of possible cybersecurity risks.
By implementing fog data analytics, organizations may improve their data security and privacy while gaining real-time insights for prompt decision-making. Organizations can get a competitive advantage in the rapidly evolving digital landscape of today by adopting this technology.💿
4. Implementing Fog Data Analytics in Business Operations:
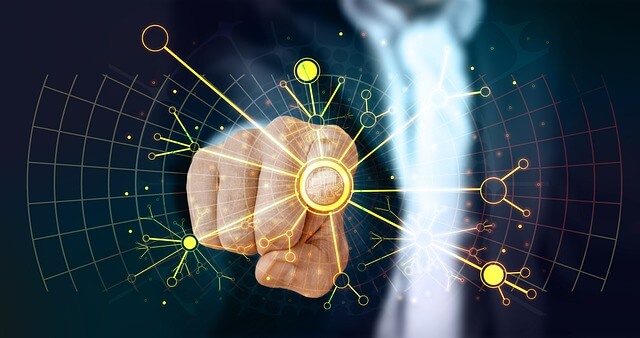
When incorporating Fog Data Analytics into current business processes, there are a number of important factors to take into account for system integration. Prior to anything else, it's critical to evaluate the existing data architecture and comprehend how fog computing can improve or supplement it. To choose the optimal course of action, organizations should consider variables including data volume, velocity, diversity, and authenticity.
It is imperative to guarantee interoperability between new fog data analytics solutions and legacy systems. Investments in middleware, APIs, or interoperability technologies can be necessary to enable smooth data transfer between edge and cloud systems. Thorough reviews and updates of security policies are also necessary to safeguard confidential data on dispersed networks.
Numerous sectors have seen successful Fog Data Analytics installations. In the manufacturing industry, for example, the use of edge devices with real-time analytics capabilities has made predictive maintenance tactics possible, which drastically lower operating costs and downtime. Manufacturers are able to optimize maintenance schedules proactively by seeing possible equipment problems before they happen by processing sensor data at the edge.
Personalized marketing campaigns have improved customer experiences in retail thanks to the use of fog analytics at store locations. Retailers can efficiently increase sales and customer happiness by studying local customer behavior data and making real-time adjustments to promotions based on those insights. These instances show how businesses from a variety of industries are using Fog Data Analytics to boost their competitiveness and spur operational innovation.
5. Challenges in Adopting Fog Data Analytics:
Adoption of fog data analytics may be hampered by network limitations and connectivity problems. Real-time data processing and analysis may be impacted by intermittent connections or bandwidth limitations resulting from the scattered nature of the fog computing environment. For the fog network to function well and overcome these obstacles, a strong network architecture is necessary.
Ensuring data quality and accuracy throughout the fog data analytics process is another crucial challenge. There is a greater chance that mistakes or inconsistencies will find their way into the analysis when data is handled at edge devices that are closer to the data source. Strict data validation procedures, encryption standards, and secure communication channels must be put in place in order to protect data integrity and uphold confidence in the insights obtained by fog analytics.
A comprehensive strategy that takes into account both operational issues and technological constraints is needed to overcome these obstacles. In today's digital landscape, businesses can effectively leverage fog data analytics for enhanced decision-making and competitive advantage by investing in dependable networking solutions, putting in place stringent data quality control measures, and encouraging a culture of continuous innovation and improvement.
6. Tools and Technologies for Fog Data Analytics:
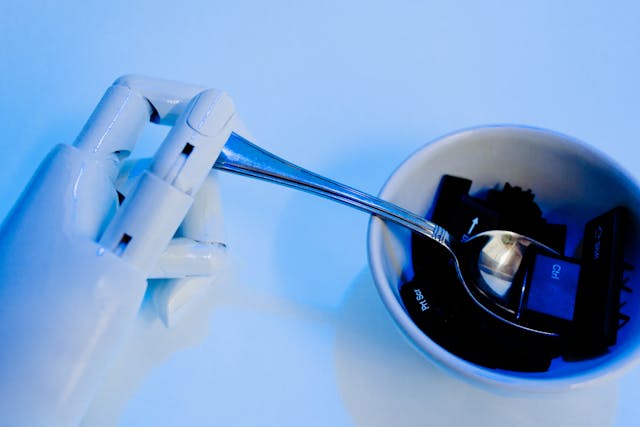
A plethora of potent tools and technologies are at businesses' disposal to help them fully realize the promise of fog data analytics. For fog data analytics, some well-liked platforms and tools are Cisco IOx, Google Cloud IoT Edge, Microsoft Azure IoT Edge, and Amazon Greengrass. These platforms offer the infrastructure required to set up analytics programs closer to the data generation location, allowing for quicker decision-making and real-time insights.
With an emphasis on industrial Internet of things applications, Cisco IOx is a comprehensive platform that facilitates edge computing. At the network edge, it permits the direct execution of applications on Cisco networking devices. With the help of Microsoft Azure IoT Edge, edge devices can benefit from cloud-based solutions that extend intelligence to real-time analytics and machine learning. By extending AWS capabilities to local devices through software, Amazon Greengrass ensures safe connectivity even in offline conditions. By leveraging Google Cloud Platform services, Google Cloud IoT Edge makes it possible to execute machine learning models directly on edge devices.
Businesses should compare various solutions available on the market based on attributes such as cost-effectiveness overall, support for several programming languages, scalability, security features, and simplicity of interface with current systems. Every platform has advantages and disadvantages, therefore it's critical to assess them in light of particular use cases and company requirements. Businesses can realize new opportunities for operational optimization, efficiency gains, and improved customer experiences by implementing fog data analytics with the right tools and technologies.
7. Future Trends in Fog Data Analytics:
Fog data analytics is predicted to keep developing quickly in the future to satisfy the expanding needs of companies in a variety of industries. An important development that is projected is the growing incorporation of machine learning and artificial intelligence (AI) into fog computing systems. More sophisticated data processing at the edge will be made possible by this integration, improving the capacity for making decisions in real time.
The emphasis on security and privacy protections is a crucial facet of fog data analytics' future. Strong security protocols will be required to protect sensitive data as more devices are connected to edge networks. The integrity and secrecy of data processed by fog computing systems will be dependent on advancements in encryption methods and secure communication connections.
It is anticipated that an increase will occur in edge-native apps created especially to take use of the special powers of fog data analytics. These apps will be designed with low processing latency, scalability, and adaptation to changing network circumstances in mind, enabling companies to gain important insights from their data closer to the source.
We believe that as fog data analytics develops further, it will become a vital resource for companies trying to take advantage of edge real-time data processing. In an increasingly interconnected world, organizations may remain ahead of the curve and unlock new chances for growth and innovation by keeping up with these developing trends and implementing them into their strategy.