1. Introduction to Big Data: What It Is and Why It Matters
The phrase "big data" is frequently used in today's technologically advanced society, but what does it really imply and why is it important? Large amounts of both structured and unstructured data that constantly overwhelm enterprises are referred to as "big data." Numerous sources, including social media, sensors, online searches, and more, provide this data.
Big Data is significant because it has the ability to reveal hidden correlations, trends, and other insights that might aid in decision-making for businesses. Through rapid analysis of vast volumes of data, businesses can learn important lessons about the patterns, habits, and preferences of their customers. These insights can be applied to forecast future market trends, improve client experiences, and improve goods and services.
Big Data is essentially about more than just the amount of data; it's also about how that data is processed and used to spur innovation and corporate expansion. This tutorial will take you deeper into the realm of big data and show you the tools, technologies, and strategies that help businesses use data to their advantage.
2. The Three V's of Big Data: Volume, Velocity, and Variety
In the world of big data, understanding the Three V's - Volume, Velocity, and Variety - is crucial.📗
The sheer volume of data produced every second from numerous sources, including social media, transactions, sensors, etc., is referred to as volume. For effective storing, processing, and analysis of this enormous volume, specialized equipment and technologies are needed.
The pace at which data is generated and must be processed in real-time or almost real-time is referred to as velocity. Organizations need to have systems in place that can effectively manage the constant influx of data that is being generated at an accelerating rate.
Variety is a symbol for the variety of data that is currently available, including unstructured data like text, photos, and videos, as well as structured data from databases. It takes adaptable tools that can easily handle various formats and architectures to manage this variation.
Understanding these Three V's helps organizations leverage big data effectively for insights and decision-making.
3. Understanding Data Analytics in the Big Data Context
Gaining insight from massive amounts of data requires an understanding of data analytics within the context of big data. Analyzing raw data to find trends, patterns, correlations, and other insightful information that might inform decision-making is known as data analytics. Advanced analytics techniques are used in the context of big data, which refers to extraordinarily massive and complicated datasets that are difficult for typical data processing tools to handle.
In the context of big data, data analytics frequently entails the effective processing and analysis of enormous datasets through the use of technologies like deep learning, artificial intelligence, and machine learning. These technologies aid in finding hidden patterns in data, forecasting trends based on past performance, and automating decision-making procedures using models and algorithms. Organizations can obtain a competitive edge by using these cutting-edge analytical tools to make data-driven decisions that spur innovation and expansion.
The capacity to analyze streaming data in real-time or almost real-time is a crucial component of data analytics in the big data environment. This makes it possible for companies to adjust swiftly to changing conditions, streamline processes instantly, and provide clients individualized experiences in a dynamic setting. Organizations can find areas for development, spot anomalies, and react proactively to new trends before they affect business outcomes by utilizing real-time analytics capabilities.
Predictive analytics is an essential part of data analytics in big data, in addition to real-time analytics. Accurately predicting future outcomes is possible through the use of statistical algorithms, machine learning techniques, and historical data in predictive analytics. Businesses can predict market trends, anticipate customer behavior, allocate resources optimally, manage risks, and improve overall strategic planning by employing predictive models constructed on big data sets. These models provide actionable insights derived from a multitude of diverse data sources.
To fully utilize large datasets in the current digital world, one must comprehend data analytics in the context of big data. In an increasingly data-driven world, organizations can gain valuable insights that drive informed decision-making processes that improve operational efficiency, enhance customer experiences, increase competitiveness, and foster sustainable growth by utilizing cutting-edge technologies and analytical techniques designed for efficiently handling large volumes of diverse data types.
4. Tools and Technologies Shaping the Big Data Landscape
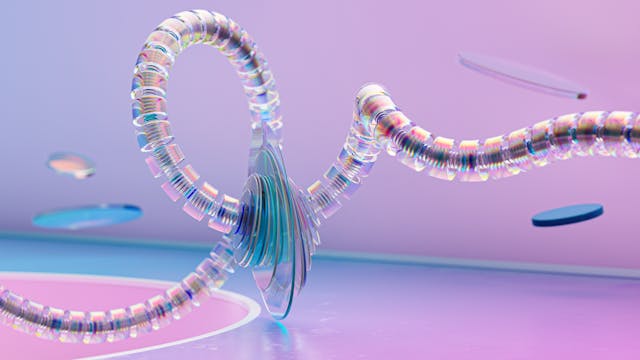
There are many tools and technology in the rapidly developing field of big data that are changing the game and empowering companies to use data in novel ways. Apache Hadoop, an open-source platform that enables the distributed processing of massive data sets across computer clusters using straightforward programming methods, is one of the key technologies in this field. Because of Hadoop's fault tolerance and scalability, it has become a key component in the processing of massive data.
Apache Spark, a potent instrument renowned for its speed and usability, is another important participant in the big data ecosystem. Spark's in-memory processing speed allows users to do intricate analytical tasks at a rapid pace. Because of its adaptability, it can be used for a variety of tasks, such as batch processing and real-time data streaming.
Amazon Redshift and Google BigQuery are two examples of data warehousing platforms that offer effective methods for storing and analyzing massive amounts of structured data. These scalable and flexible cloud-based solutions enable enterprises to easily query large databases and extract insightful information.
When it comes to using big data for predictive analytics and pattern identification, machine learning algorithms are essential. Data scientists may create and implement machine learning models with the aid of frameworks like TensorFlow and scikit-learn, which empowers businesses to make defensible decisions based on insights from their data.
Users can generate interactive dashboards and reports using visualization tools like Tableau and Power BI, which simplify complex datasets into visually understandable representations. By making data available to non-technical stakeholders, these technologies democratize data and promote improved decision-making throughout enterprises.
Newer technologies like Kubernetes for container management, Apache Kafka for real-time streaming, and Apache Flink for event-driven applications are becoming more and more popular as the big data landscape changes. These developments are changing the way businesses handle, store, examine, and display data on a large scale.
Anyone hoping to efficiently traverse the complexity of big data must have a solid understanding of these tools and technology. In today's data-driven world, people may fully utilize big data to spur innovation, acquire a competitive edge, and open up new prospects by keeping up with the newest advancements in the area.
5. The Role of Machine Learning in Big Data Analysis
Machine learning is essential to the processing of large amounts of data because it uses algorithms that let computers learn from their experiences and get better without explicit programming. It gives computers the ability to analyze massive volumes of data in a way that would be hard for humans to do alone—pattern recognition, prediction, and insight generation. Large datasets can be combed through by machine learning algorithms, which can then be used to find hidden patterns, correlations, and outliers that might otherwise go missed.
Machine learning techniques including clustering, classification, regression, and anomaly detection are frequently employed in the field of large data analysis. In order to find naturally occurring groupings within the dataset, clustering algorithms combine related data points according to their shared properties. Classification algorithms use historical observations to group data into predetermined classes or labels. Regression algorithms examine the relationship between variables to predict continuous outcomes. Algorithms for detecting anomalies are used to find unusual occurrences or outliers in data that substantially depart from the norm.
Machine learning forecasts future trends and behaviors based on past data patterns, allowing businesses to use the potential of predictive analytics. With the help of this predictive capability, businesses can make better decisions, foresee client requirements, stop fraud, simplify operations, and spur innovation. Businesses can gain a competitive edge and spur expansion in the data-driven economy of today by utilizing machine learning in tandem with big data analytics tools.
Furthermore, as I mentioned previously, machine learning serves as a cornerstone in the field of big data analysis by making it possible to effectively process enormous amounts of complicated data and extract insightful knowledge that helps with strategic decision-making. Organizations can now use their ever-expanding datasets to acquire a competitive edge and stay ahead in a world driven by an abundance of information, thanks to its autonomous learning and adaptation capabilities. It is imperative for individuals and enterprises to comprehend the function of machine learning in big data analysis if they wish to fully utilize their data resources and effect revolutionary change by making well-informed decisions.
6. Ethical Considerations in Handling Big Data
Ethical considerations are critical when working with large datasets. Numerous ethical issues are brought up by the sheer amount and sensitivity of the data gathered, and both individuals and organizations need to address them. Upholding core concepts such as privacy rights, data security, and transparency in data utilization are vital. Navigating the ethical issues around big data requires finding a balance between protecting individual rights and using data for advances.
Consent is an important factor to take into account. Getting people's informed consent is crucial before gathering and utilizing their data. This entails outlining precisely who will use their data and for what reasons. Transparency shows a commitment to ethical big data handling methods and aids in establishing confidence with users. Ensuring the integrity of data and protecting privacy concerns requires the implementation of strong data security measures to prevent breaches or unauthorized access.
Making sure that algorithms and machine learning models are used fairly and with accountability is a crucial component of ethical big data practices. Algorithm bias has the potential to provide discriminatory results, exacerbating already-existing social injustices. In order to advance justice, equity, and inclusion, biases in the data sets used to train these algorithms must be routinely evaluated and addressed.
It is crucial to take into account the long-term effects of big data usage on people and society at large. When working with massive datasets, ethical considerations include conversations about data retention regulations, anonymization procedures, and responsible data sharing practices. We can ethically utilize the power of big data while reducing possible dangers and harms to people's privacy rights and the general well-being of society by proactively addressing these ethical challenges.📉
A multifaceted strategy that places a high priority on respecting individual privacy rights, transparency in data usage, fairness in algorithmic decision-making processes, accountability for results, and long-term considerations regarding data retention and sharing practices is needed to navigate the ethical landscape of big data. We can harness the revolutionary power of big data while building user trust and establishing a more fair digital society by adhering to these ethical norms.
7. Real-World Applications of Big Data Across Industries
Big data has enabled better decision-making, streamlined operations, and enhanced consumer experiences, which have completely changed a number of businesses. Big data is used in healthcare to forecast disease outbreaks, evaluate patient records, and customize treatment regimens. Better health outcomes and more effective healthcare delivery result from this.
Big data helps the retail industry by enabling targeted marketing efforts, better inventory control, and price optimization based on customer behavior analysis. This lowers operating expenses, improves consumer satisfaction, and enables businesses to customize their products to suit the interests of specific customers.
Big data is essential to algorithmic trading, fraud detection, risk assessment, and consumer segmentation in the financial sector. Institutions can identify suspicious activity, reduce risks, automate trading methods, and provide individualized financial services by instantly evaluating massive amounts of financial data.
Big data is used by transportation and logistics firms for supply chain management, demand forecasting, predictive maintenance of infrastructure and vehicles, and route optimization. Through increased fuel efficiency, decreased asset downtime, and simplified operations, this leads to cost savings.
Big data is essential to the manufacturing industry because it makes it possible to optimize production processes through predictive analytics, monitor quality control with real-time sensors, and maintain equipment predictively. This raises operational effectiveness and lowers unplanned downtime expenses brought on by malfunctioning or defective equipment.
In the end, the integration of big data analytics transforms how businesses operate, make decisions, service customers, optimize operations, and increase competitiveness in a quickly expanding digital landscape across industries like healthcare, retail, finance, transportation, logistics, and manufacturing.
8. Overcoming Challenges in Implementing a Big Data Strategy
Overcoming Challenges in Implementing a Big Data Strategy
For businesses of all sizes, putting a big data strategy into practice may be a difficult undertaking. Even though using big data has many advantages, there are a few major obstacles that could prevent its use. The sheer amount of data that needs to be processed and examined is a common problem. To handle this data successfully, organizations need to invest in the appropriate technology and infrastructure.
Making sure data is consistent and of high quality is another major difficulty. Decisions and insights that are based on inadequate or inaccurate data might be faulty. To overcome this obstacle, strong data quality procedures and guidelines must be put in place. To safeguard confidential information and guarantee regulatory compliance, organizations must think about data governance and security procedures.
Another challenge in putting a big data strategy into practice is the integration of various data sources. To obtain significant insights, data from multiple sources, including enterprise systems, social media, and sensors, must be smoothly linked. Careful planning, standardization, and the application of cutting-edge integration tools are necessary for this.
In today's competitive labor market, hiring and maintaining qualified workers with knowledge of big data technology is essential but difficult. To draw in top talent, organizations must fund training initiatives and cultivate a culture that embraces data-driven decision-making.
Finally, another challenge that firms encounter when putting a big data strategy into practice is scalability. Organizations need to be sure that their infrastructure can scale with the exponential growth in data volume without sacrificing performance.
From all of the foregoing, it is clear that overcoming these obstacles calls for an all-encompassing strategy that includes scalable infrastructure solutions, integration plans, people management initiatives, investments in technology, and strict procedures for monitoring data quality and governance. Organizations can realize the full benefits of big data and obtain a competitive advantage in the current digital environment by proactively tackling these issues.
9. The Future of Big Data: Trends and Predictions
Big data is expected to see major breakthroughs in the future as long as technology keeps developing. The emergence of edge computing, which processes data closer to the source rather than in a centralized data center, is one development to keep an eye on. This change will allow for higher processing rates and lower latency, which are essential for applications such as driverless vehicles and the Internet of Things (IoT).
The growing use of machine learning and artificial intelligence (AI) in the analysis of massive volumes of data is another important prediction. At a never-before-seen scale, these technologies are able to identify patterns, anticipate outcomes, and automate decision-making procedures. The increasing sophistication of AI is expected to stimulate innovation in the ways that firms utilize their data to get insights and make strategic decisions.
Big data-related privacy and security issues will always be in the spotlight. To keep customers trusting them, businesses must prioritize data protection procedures in light of more stringent legislation like GDPR and the increased public awareness of data breaches. In the future, there will probably be more focus on using data ethically and being transparent about how businesses handle personal data.
As I mentioned before, big data has enormous potential to change industries all around the world in the future. Businesses can leverage the power of big data to fuel innovation and maintain competitiveness in an increasingly digital world by keeping up with developing trends, adopting new technologies like edge computing and artificial intelligence, and placing a high priority on data protection.
10. Key Terms and Concepts Every Beginner Should Know
When starting to delve into the world of big data, it's essential to grasp some key terms and concepts. Here are several fundamental ones that every beginner should know:
1. **Big Data**: Refers to large volumes of data that cannot be easily processed or managed using traditional data processing applications.
2. **Structured Data**: Data that is organized in a pre-defined manner, such as in rows and columns like in a database.
3. **Unstructured Data**: Information that lacks a specific format or structure, such as text documents, images, videos, and social media posts.
4. **Data Mining**: The process of discovering patterns and insights from large datasets using various techniques like machine learning and statistical modeling.ðŸ’
5. **Machine Learning**: A subset of artificial intelligence that enables systems to learn from data without being explicitly programmed, allowing them to improve performance over time.
6. **Predictive Analytics**: The use of historical data to predict future outcomes or trends by analyzing patterns and relationships within the data.
7. **Data Visualization**: The graphical representation of data to provide insights and aid in understanding trends, patterns, and outliers within the dataset.
8. **Hadoop**: An open-source framework for distributed storage and processing of big data across clusters of computers using simple programming models.
9. **Data Warehousing**: The process of collecting and managing data from various sources into one central repository for analysis and reporting purposes.
10. **NoSQL**: A type of database management system designed for handling large sets of distributed data across multiple servers efficiently.
Understanding these key terms and concepts will lay a solid foundation for anyone venturing into the dynamic realm of big data analytics.