1. Introduction to Data Products:
Data products are becoming increasingly important in the digital age, helping to shape business strategy and decision-making procedures. In essence, these goods are instruments, services, or programs designed with data in mind to offer insights, streamline workflows, or improve user experiences. Simple reports to intricate algorithms and machine learning models are some examples of them.
It is impossible to overestimate the significance of data products in today's corporate environment. They give businesses the ability to discover the value concealed in their data assets, giving them a competitive advantage, increasing productivity, and spurring innovation. Businesses may anticipate market trends, personalize consumer experiences, make better decisions, and streamline internal operations by utilizing data products efficiently.
Businesses hoping to maximize the value of their data assets must grasp the lifecycle of data products as data volumes and complexity continue to rise. To create solutions that have an impact and propel corporate growth and success, it is essential to comprehend every stage of the data product lifecycle, from conception and development to deployment and maintenance.
2. Understanding the Lifecycle of Data Products:
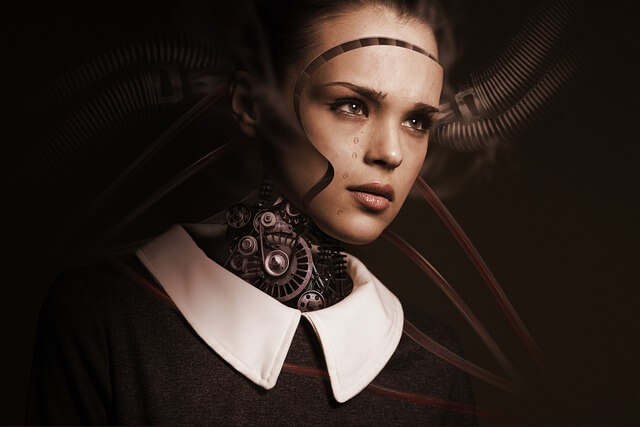
Data products usually have a lifecycle that spans multiple stages, starting from conceptualization and concluding with product retirement. Planning, development, deployment, monitoring, and optimization are typical steps. Setting performance criteria, locating data sources, and defining project goals are important tasks that must be completed during the planning stage. By offering insights and establishing expectations, stakeholders such as product managers, business analysts, and data scientists play critical roles throughout this phase.
Data engineers and analysts collaborate during the development stage to collect and prepare data, construct models or algorithms, and produce reports or visualizations. Preparing the data, testing, refining the model, and training it are all part of this phase. Stakeholders work closely together to make sure that the finished product satisfies technical criteria and business objectives.
When the data product is made available to users, deployment signifies the change from development to implementation. Integrating the product into current platforms or systems, providing continuous product access, and, if required, holding user training sessions are important tasks in this phase. In order to guarantee a seamless deployment process, stakeholders including IT teams, end users, and customer support are involved.
Stakeholders regularly monitor system health, user feedback, and key performance indicators (KPIs) during the crucial monitoring phase. IT personnel keep an eye on system performance and security, while data scientists examine trends in user behavior or problems with data quality. Frequent KPI reporting assists stakeholders in making well-informed judgments about future product optimization and potential issues resolution.
During the optimization phase, stakeholders use the information gathered from monitoring to iteratively improve the product. While developers work on adding new features or fixing bugs, data scientists might retrain models using fresh data inputs. In order to effectively lead future revisions, business analysts offer feedback on how the product influences important KPIs or business processes.
By including pertinent stakeholders at each stage to achieve successful product results that correspond with business goals, businesses may manage their data assets more efficiently by having a better understanding of various phases in the lifetime of data products.
3. Scope of Data Product Management:
Because it manages the lifespan of data products from inception to delivery, data product management is essential to enterprises. This entails formulating the product's vision, planning its advancement, and guaranteeing its effective implementation. Data product managers translate business requirements into workable plans by serving as a connection between technical teams and stakeholders. They are in charge of allocating tasks, overseeing supplies, and coordinating the product roadmap with business objectives.
Managing data products has unique opportunities and problems. Balancing technical complexity with user requirements and making sure the solution satisfies both usability standards and functional objectives is a typical difficulty. To maintain compliance with industry rules and data privacy legislation, data product managers need to negotiate constantly changing regulatory landscapes. Conversely, good data product management can result in better decision-making, better customer experiences, and more operational efficiency. Adopting cutting-edge technology like artificial intelligence (AI) and machine learning offers chances to generate fresh perspectives and spur product development innovation.
In summary, the field of data product management comprises a broad variety of duties that are essential for promoting corporate performance. Businesses can use data to drive growth, innovation, and strategic decision-making by harnessing possibilities and surmounting obstacles in data product management.😍
4. Ideation Phase:
The ideation stage of the data product lifecycle is essential for coming up with creative concepts. Teams come up with ideas and concepts for new data products that cater to the needs of developing markets throughout this phase. To assure the success of the product, it entails comprehending consumer requirements, market trends, and technological viability. Prior to allocating resources to its development, a data product's viability can be ascertained by evaluating the market's demands and its feasibility. Through comprehensive market research and feasibility studies, businesses may pinpoint gaps in the market and efficiently customize their data products to fit unique client needs. This stage lays the groundwork for producing useful data products that connect with target markets and spur company expansion.
5. Development Phase:
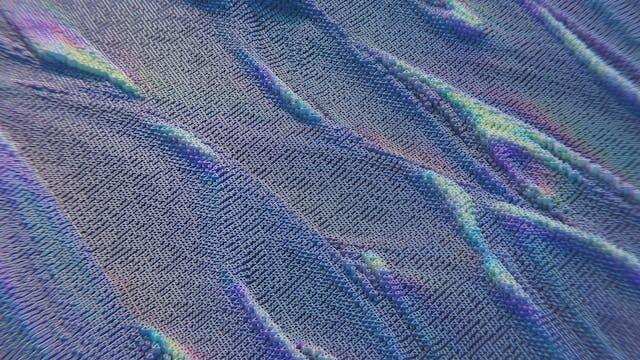
Teams concentrate on creating prototype iterations of the product during the Development stage of a data product lifecycle. These early iterations serve to aid in visualizing the eventual product's appearance and functionality. Data scientists, engineers, and other stakeholders work together during the development phase to make sure the final result is in line with the original plan.
During this stage, testing is essential since it helps find any problems or defects in the prototype. Teams can get input on how to improve the prototype's functionality and user experience by putting it through a variety of tests and situations. After then, iteration procedures are implemented in response to these tests and comments, enabling ongoing improvement until a version that meets user needs is reached.
Teams can fine-tune their data products to satisfy user requirements and business objectives by using iterative procedures and thorough testing. By introducing feedback loops that promote continual improvement and guarantee that the finished result is reliable and efficient in meeting particular business goals, this phase establishes the groundwork for a successful data product.
6. Launch and Deployment:
A data product's launch and deployment phase is a crucial stage that needs to be carefully planned and carried out to guarantee its smooth integration into the current systems. User education, extensive testing, and stakeholder communication are all essential launch strategies. To monitor progress, it is imperative to establish a well-defined rollout plan that includes deadlines, roles, and completion dates.
It is essential to track performance after deployment in order to evaluate how the data product affects business goals. It is advisable to establish key performance indicators (KPIs) in advance in order to gauge the efficacy of the product. Frequent monitoring makes it possible to see problems or potential improvement areas and make the necessary quick adjustments to improve performance and stay relevant over time.
Taking into account everything mentioned above, we can say that the development of a data product comes to an end at the launch and deployment phase. Organizations may optimize the advantages of their data products and improve decision-making procedures by putting into practice efficient launch plans and closely observing the product's performance after deployment.
7. Maintenance and Optimization:
Maintenance and Optimization are crucial phases in the data products lifecycle. Techniques for maintaining and updating data products include regular monitoring, performance tuning, debugging, and addressing issues promptly. Continuous improvement strategies involve leveraging user feedback, implementing advanced analytics for better insights, and staying abreast of technological advancements to enhance product efficiency and accuracy. Maintaining data products ensures that they continue to perform effectively over time while optimization involves refining algorithms, updating models, and adapting to changing requirements. Continuous improvement strategies are key to maximizing the value of data products by incorporating feedback loops, conducting A/B testing, and iterating on solutions iteratively for better outcomes. By focusing on maintenance and optimization with a strategic approach to continuous improvement, organizations can ensure that their data products remain relevant and valuable in the long term.
Embracing these practices not only enhances the performance and reliability of data products but also enables organizations to stay competitive in an ever-evolving data-driven landscape.
8. Data Product Monetization:
An essential part of the lifecycle of a data product is data product monetization. Investigating different monetization approaches becomes crucial as businesses want to extract value from their data assets. Direct sales of data products to outside clients or partners are one such strategy. With this strategy, the data is priced according to subscription costs, usage volume, or perceived value.🗒
Using freemium business models, in which a basic data product is provided for free and premium capabilities are chargeable, is another well-liked approach. With the help of this tactic, businesses can draw in more users and make money from more sophisticated features. Common monetization strategies include licensing and royalties, which allow businesses to charge for the use or distribution of their data products.
There are a number of things to consider while figuring out how to monetize data goods. It is essential to comprehend the unique selling proposition and market need for the data product in order to establish competitive pricing and draw in clients. Building customer trust and safeguarding sensitive data necessitates making sure data privacy laws are followed and data security is maintained.
In order to better satisfy market demands, monetization techniques can be improved and offerings can be tailored by analyzing customer feedback and usage trends. Businesses can maximize their revenue streams and provide their target audience with useful data products by regularly assessing performance metrics and modifying price structures.
9. Data Governance and Compliance:
A key part of the data product lifecycle is data governance and compliance, which concentrates on upholding security, privacy, and legal requirements. Organizations may make sure that sensitive data contained in data products is shielded from breaches and unauthorized access by putting strong data governance procedures in place. In order to protect data throughout its lifecycle, policies, procedures, and controls must be established.
Providing data products with privacy and security guarantees is essential to winning over stakeholders and customers. Access controls, data encryption, and frequent security audits are crucial steps in reducing the risks related to data breaches. Adherence to industry-specific norms and laws, such as GDPR, is crucial for the responsible management of personal or sensitive data.😐
Requirements for regulatory compliance greatly influence how businesses handle their data products. To keep out of trouble or face legal ramifications for their data handling procedures, businesses need to be aware of how laws and regulations are changing. Gaining a thorough awareness of these standards helps firms to properly coordinate their data governance plans and adjust to changes in the legal environment.
10. Data Product Performance Measurement:
Measuring performance throughout the lifecycle of a data product is essential to comprehending its influence and success. Metrics are essential for monitoring how well data products are working. Depending on the objectives of the product, these metrics may differ, however they could include ROI, conversion rates, user engagement, and usage statistics. Organizations can make well-informed decisions about their data products by routinely monitoring these parameters.
Managers can use a range of tools and strategies to measure the performance of data products in an efficient manner. Platforms for data analytics like as Tableau, Mixpanel, and Google Analytics can offer comprehensive insights into user behavior and product performance. A/B testing makes it possible to compare and assess which iteration of a data product performs better. Heatmaps and session recordings are two examples of tracking tools that can provide visuals of how consumers engage with the product.
By putting these performance measurement tools and approaches into practice, organizations may constantly optimize their data offerings. Managers may effectively accomplish corporate objectives and improve customer experience by making data-driven decisions based on the insights gathered from these measurements.
11. Evolving Trends in Data Product Management:
The noteworthy influence of developing technologies on the field of data product management is one of the evolving trends in the industry. Big data analytics, machine learning, and artificial intelligence are some of the technologies that are changing the way that data products are created, used, and managed. These developments improve the possibilities of data products by enabling more sophisticated data processing, automation, and predictive analytics.
We may anticipate more development and expansion in the field of data product management in the future. We expect increasingly complex data product offerings that make advantage of real-time data processing, tailored insights, and improved user experiences as technology develops. Future developments may also include more stringent procedures for guaranteeing data security and compliance within data product creation and management processes, given the growing emphasis on ethical issues and data privacy laws.
12. Case Studies on Successful Data Product Lifecycle Management:
Case studies are an important tool for studying successful data product lifecycle management because they provide practical examples of solutions that work. Examining these instances helps us understand the complex procedures and choices that go into making data products successful. These case studies offer a lot of information for individuals navigating the challenges of efficiently managing data products, not only by highlighting best practices but also by sharing insightful lessons gained from their implementation.
The adoption of a customer analytics platform by a top e-commerce business is one example of a case study. The organization was able to enhance user engagement overall, optimize recommendations, and tailor the customer experience by utilizing advanced predictive analytics and machine learning algorithms. They significantly improved key performance indicators and obtained a competitive advantage in the market by carefully managing data quality, integration, and governance throughout the product's lifespan.
A financial institution's use of a big data analytics-powered risk assessment tool is the subject of another fascinating case study. With the use of this program, possible dangers might be monitored in real time, facilitating proactive risk assessment and mitigation plans. Agile development approaches, stringent testing procedures, and ongoing cooperation amongst cross-functional teams were key components of this data product lifecycle's effective management. Organizations can gain important insights into efficiently managing risks and utilizing big data capabilities to inform strategic decision-making by studying this case study.
The aforementioned case studies highlight the crucial function of proficient administration tactics in actualizing the complete possibilities of data goods throughout their entire existence. Every stage, from the first conception and development to the deployment and optimization, needs to be carefully planned, carried out, and adjusted based on information obtained from data-driven feedback loops. Achieving lasting success in managing data products requires careful attention to detail, alignment with corporate objectives, and flexibility in adapting to shifting market conditions, as demonstrated by successful implementations.
To rephrase what I just said, companies wishing to improve their skills in this area can learn a great deal by studying case studies on effective data product lifecycle management. Through real-world examples, businesses may gain significant insights into effective management techniques and lessons learned from successful implementations. This helps them comprehend the complexity and nuances involved. Using these case studies can help firms optimize their own methods for managing data products successfully in the fast-paced business environment of today as they continue to leverage the power of data-driven decision-making.