1. Introduction to Insurance Data Analytics
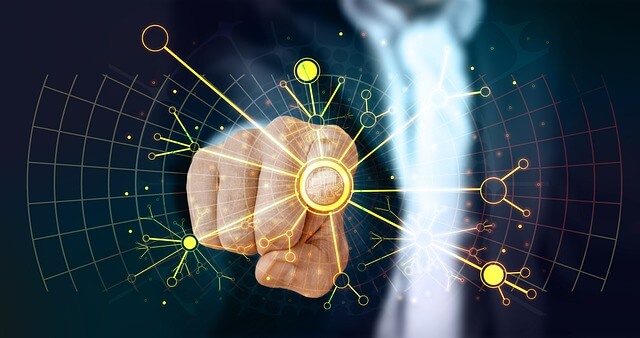
Introduction to Insurance Data Analytics:
The process of examining sizable data sets from the insurance sector in order to glean insights that can direct decision-making is known as insurance data analytics. In order to enable enterprises to make data-driven decisions, this process entails interpreting complicated data using sophisticated analytical techniques and tools. The potential of insurance data analytics to raise client satisfaction, streamline risk management procedures, increase operational effectiveness, and eventually spur corporate expansion makes it crucial.
Data analytics is transforming the insurance sector by giving insurers with the capabilities to better understand their clients, predict future trends, and adjust goods and services to meet evolving expectations. With the vast amount of data generated in the insurance sector, from customer information to claims history and market trends, leveraging this data through analytics can lead to more accurate pricing models, improved fraud detection, streamlined operations, and enhanced customer engagement strategies. Insurance companies that want to remain relevant and competitive in the digital era must use data analytics to their advantage as technology develops and competition in the market heats up.
2. Key Challenges in Insurance Sector
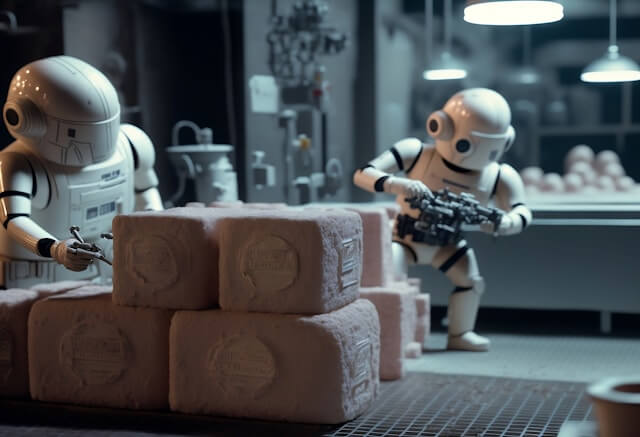
Businesses in the insurance industry face a number of obstacles that may hinder their productivity and financial success. Identifying risk accurately is one common problem. Insurance firms need to examine large volumes of data to determine the possibility of a claim being made. In the absence of sophisticated data analytics tools, this procedure can be laborious and prone to mistakes.
Fraud detection presents a serious obstacle as well. Every year, insurance fraud costs businesses billions of dollars. Businesses might avoid huge financial losses by using data analytics to identify odd trends or behaviors that can point to fraudulent activities.⌨️
Additionally, data analytics is essential for maintaining and satisfying customers. Insurance firms can personalize their services and improve customer service by using data analysis to understand client behavior and preferences. This can ultimately result in higher rates of customer loyalty and retention.
One of the main issues facing the insurance industry is regulatory compliance. Through real-time monitoring and reporting capabilities that enable organizations to quickly spot any discrepancies or issues, data analytics may assist businesses in making sure they are complying with all regulatory obligations.
In the insurance industry, data analytics plays a key role in solving common problems including precise risk assessment, fraud detection, customer retention, and regulatory compliance. Insurance firms may make well-informed decisions, efficiently minimize risks, improve operational efficiency, and ultimately outperform their competitors in the market by utilizing data analytics technologies.
3. Types of Data Used in Insurance Analytics
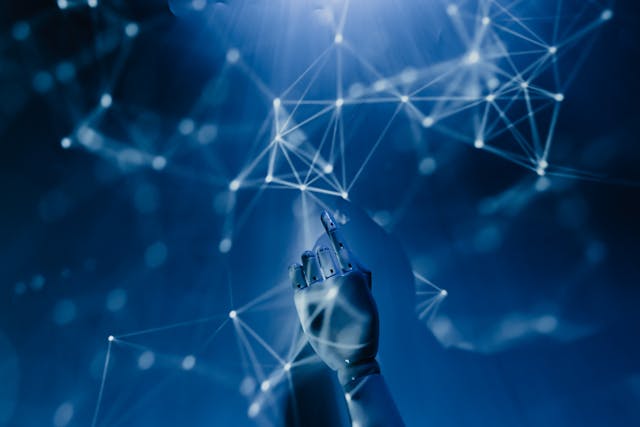
Both structured and unstructured data sources are used in insurance analytics to glean insightful information. Information that is arranged according to a predetermined format, such as policyholder information, claim amounts, and premium payments, is referred to as structured data. Conversely, less structured data such as emails, contact center records, and social media posts is referred to as unstructured data.
Policyholder demographic information such as age, gender, location, and occupation are a few instances of data points that are frequently utilized for predictive modeling in insurance analytics. Predicting future claim behavior involves a substantial quantity of data related to claim history, including frequency, payout amounts, and prior claims made. Vehicle information for auto insurance, such as make, model, and year of manufacture, as well as driving record records, are additional critical data elements.
Insurance analytics models can be made more predictive by adding external data sources, such as weather trends, economic indicators, or healthcare information. Insurers can make smarter judgments, analyze risks more accurately, and operate more efficiently by utilizing both structured and unstructured data efficiently.
4. Benefits of Data Analytics in Insurance
Data analytics is essential for enhancing decision-making procedures in the insurance sector. Underwriting and risk assessment provide a major advantage. By using data analytics, insurers may more correctly analyze risks, leading to better underwriting decisions and pricing strategies.
In the insurance industry, data analytics has the potential to greatly improve the client experience. Data can be used by insurers to customize products for specific clients, allowing them to customize plans to meet their needs and preferences. This degree of personalization helps increase client happiness as well as retention and loyalty rates.
Utilizing data analytics in the insurance industry helps insurers to provide more individualized experiences that address the changing needs of their clients, strengthening client relationships and boosting market competitiveness. It also results in more accurate risk assessments and underwriting procedures.
5. Tools and Techniques for Insurance Data Analysis
Various analytics tools and strategies are utilized by insurance businesses nowadays to effectively utilize their data for well-informed decision-making. Staying ahead in the fast-paced insurance industry necessitates not only gathering data but also doing insightful analysis on it. The insurance industry makes extensive use of SAS, IBM SPSS, and Tableau as analytics tools. With the use of these tools, insurers can sort through enormous volumes of data, spot trends, and derive insightful knowledge that may inform business plans.
The way insurers interpret their data is being revolutionized by a cutting-edge method called machine learning. Machine learning uses statistical models and algorithms to forecast results based on past data. This makes it possible for insurance companies to identify fraud, expedite the processing of claims, estimate risk more precisely, and customize their offers to customers. Another effective method is predictive modeling, which uses past data to project future patterns. This helps insurers foresee shifts in the market and take preemptive measures.🥧
These methods and tools improve consumer experiences while also increasing operational efficiency for insurance companies. By employing advanced analytics, insurers may offer tailored policies and services that fit individual needs more effectively. Insurance firms can react to changing market dynamics and provide value to their clients and stakeholders by using a data-driven approach to decision-making.
6. Case Studies on Successful Implementation
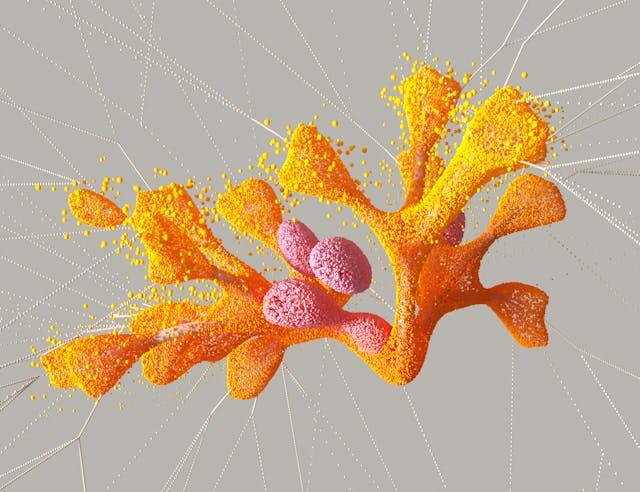
Data analytics is essential to the insurance industry as it helps organizations make well-informed decisions and transform. Let's examine a few interesting case studies that demonstrate how data analytics can be successfully applied in the insurance sector and have a real influence on business choices and results.
1. **Case Study 1: Lemon Insurance**
To improve client satisfaction and streamline risk management, Lemon Insurance, a made-up insurance company, incorporated cutting-edge data analytics technologies into its business processes. Lemon Insurance was able to increase customer satisfaction and retention rates by using predictive analytics models on customer data to create plans based on unique needs. They considerably enhanced fraud detection methods by applying machine learning algorithms to analyze historical claims data. This resulted in a large decrease in claim payouts and an increase in profitability.
2. **Case Study 2: Beacon Health**
One well-known health insurance company, Beacon Health, used data analytics tools to increase policyholders' access to better treatment and optimize operational efficiency. Beacon Health found ways to reduce costs without sacrificing the quality of service it provided by using big data analytics to analyze healthcare consumption and expense trends. In addition to optimizing resource allocation, this proactive strategy allowed Beacon Health to provide creative wellness programs catered to the specific requirements of each policyholder, which eventually resulted to healthier covered populations and lower medical expenditures.
3. **Case Study 3: SecureNet Insurance**
SecureNet Insurance adopted data analytics to modernize their underwriting procedures and pricing methods for numerous insurance products. SecureNet Insurance obtained important insights into the risk profiles and behavior of its customers by utilizing real-time data streams from Internet of Things (IoT) devices, such as wearable technology and smart home sensors. With the help of this dynamic technique, they were able to dynamically modify premiums in response to real usage patterns rather than sweeping generalizations, which improved pricing models and made them more competitive in the market.
These case studies highlight the effective use of insurance data analytics by forward-thinking firms to make strategic decisions that have a significant impact on their entire performance in addition to driving corporate growth. Adopting data analytics has become essential for insurers hoping to prosper in the current competitive market, from improving client interaction and lowering risks to streamlining operations and encouraging innovation.
Insurance companies can seize untapped opportunities, efficiently manage risks, and anticipate market trends by adopting data-driven insights obtained from advanced analytical tools and technologies. This approach ultimately paves the way for sustainable growth and operational excellence within the constantly changing insurance ecosystem.
7. Ethical Considerations in Insurance Data Analytics
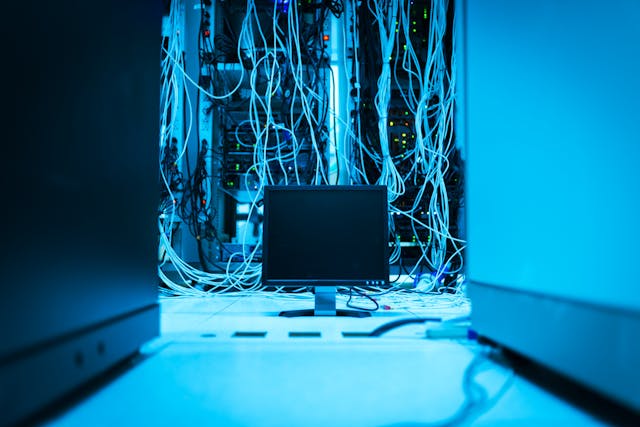
Ethical considerations are critical to protecting individual privacy and data security in the field of insurance data analytics. The delicate balance between using consumer data to improve services and protecting against potential misuse or breaches is a topic of discussion that comes up frequently. Because insurance businesses acquire sensitive personal information, privacy concerns arise. This highlights the importance of implementing strong security measures to thwart unwanted access and data leaks.
Since regulations govern the collection, storage, and use of client data, regulatory compliance is yet another crucial component of insurance data analytics. Respecting these rules demonstrates ethical duty to clients in addition to guaranteeing legal compliance. Maintaining openness regarding data procedures helps protect policyholders' right to privacy while also fostering trust with them.
Insurance firms need to take extra care to handle sensitive client data ethically. This entails getting express consent before collecting data, putting strong security measures in place to prevent against online attacks, and protecting individual identities by using anonymization methods. Insurance companies can reduce risks related to possible data breaches or misuse while cultivating a culture of trust and accountability with their clients by emphasizing ethics in their data analytics procedures.
8. Future Trends in Insurance Data Analytics
A number of new technologies are influencing the direction of the insurance data analytics industry, which is a constantly changing field. It is anticipated that machine learning and artificial intelligence (AI) would be key components in enhancing risk assessment, fraud detection, and client customisation. The utilization of Internet of Things (IoT) gadgets, including wearable health tracking devices and car telematics, will give insurers access to real-time data for more precise underwriting and pricing models.
Insurance companies will use predictive analytics more and more as they try to better predict trends, risks, and customer behavior. Predictive modeling will provide insurers with crucial insights into client wants and market dynamics as big data becomes more widely available and thorough. Insurance companies will be able to extract valuable data from unstructured data sources such as social media platforms and customer reviews thanks to advancements in natural language processing (NLP).
In the future, the development of insurance data analytics has the potential to completely transform the way the sector works. Automating repetitious operations like policy administration and claims processing can be made more efficient with robotic process automation (RPA). With the establishment of tamper-proof records and smart contracts, blockchain technology shows potential for improving data security, decreasing fraud, and increasing transparency within the insurance industry.
Decision-making procedures will be improved as time goes on by fusing these state-of-the-art technologies with conventional statistical models in all aspects of the insurance value chain. The combination of technical advancement and human skill will spur innovation, boost operational effectiveness, and ultimately benefit policyholders and insurers alike.