1. Introduction
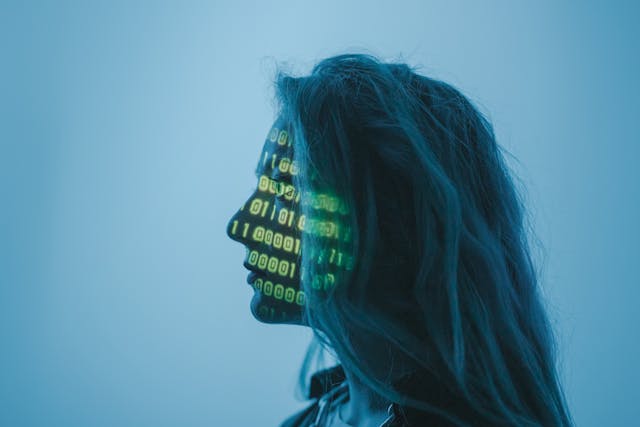
Big data, as used in the context of the stock market, refers to enormous amounts of data that can be examined to identify patterns, trends, and insights about stock prices, market movements, investor mood, and other topics. Numerous sources, including social media exchanges, financial reports, trading volumes, and economic indicators, are included in this data set. Big data's application in the stock market has transformed investing methods by giving investors access to crucial information that helps them make wise choices.
Because big data makes it possible for analysts and investors to quickly absorb and comprehend vast amounts of information, it is changing the dynamics of the stock market. Through the use of sophisticated analytics tools and machine learning algorithms, market players are better equipped to spot new trends, find hidden correlations, and anticipate changes in the market. The capacity to use big data has given investors the flexibility to respond quickly to shifting market conditions and take advantage of previously unnoticed possibilities. As a result, agile rivals who successfully use big data to obtain a competitive edge in trading activities pose a threat to established stock market firms.
2. The Rise of Big Data in Stock Trading
Big data analytics has been a game-changer in the world of stock markets in recent years. In the constantly evolving world of stock trading, traders are depending more and more on big data to obtain insights, make wise judgments, and maintain an advantage. Traders can now analyze massive volumes of data at previously unheard-of speeds because to the power of big data, which improves their ability to accurately detect patterns, trends, and possible trading opportunities.
Big data is transforming the study of the stock market by bringing in previously unexplored sources of information. These days, traders use a wide range of big data sources, including sentiment analysis from social media, web scraping to gather financial news and corporate reports, satellite imagery to monitor economic activity like oil storage levels or agricultural yields, and alternative datasets from sources like credit card transactions or foot traffic data.
With these effective tools at their disposal, traders may gain a competitive edge by identifying early warning signs of market changes and using a thorough understanding of the complex dynamics influencing stock prices to inform their trading decisions. Big data's emergence in stock trading heralds a change in strategy toward one that is more data-driven, altering investor tactics and creating new avenues for navigating the intricacies of today's markets.
3. Disruption Potential: Big Data vs Traditional Methods
In the stock market, big data analytics is posing a challenge to established stock trading strategies. In contrast to conventional methods that mostly depend on historical data and fundamental analysis, big data makes use of large and varied datasets to reveal correlations, patterns, and trends that were previously undiscovered. By using real-time insights, investors may now make better educated judgments instead of depending just on their intuition or scant information.
Big data has the potential to upend established stock market players because of its capacity to handle and analyze enormous volumes of data at a speed and scale that are not possible for traditional systems or human traders to match. Big data platforms can more accurately forecast trends, detect market signals, and swiftly uncover hidden possibilities by utilizing machine learning and artificial intelligence algorithms. This increased flexibility offers big data investors a competitive advantage over those who stick to traditional techniques, which could cause major changes in the dynamics of the market.
On the other hand, conventional approaches frequently find it difficult to keep up with the volume and complexity of the financial markets of today. Manual analysis takes a lot of time, is prone to biases, and could miss important aspects that affect stock prices. Big data provides an answer through process automation, quick dataset synthesis, and dynamic insights that adjust to shifting market conditions. Big data is posing a serious threat to the status quo in the stock market environment as it develops and improves its predictive powers.
Big data's potential to upend conventional approaches to stock trading is demonstrated by its ability to completely transform the way investors make decisions. Market players can obtain a competitive edge by adopting sophisticated analytics tools driven by big data technology, which can result in quicker insights, more astute plans, and stronger risk management procedures. Big data has the power to upend existing companies and provide a larger variety of market participants with democratized access to sophisticated trading instruments by reshaping the process of making and executing investment choices.
4. Improving Market Predictions with Big Data
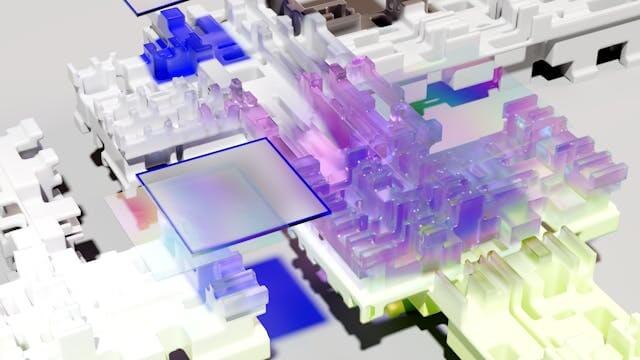
Big data's ability to improve market forecasts has transformed stock market forecasting by offering more precise insights and projections than were before possible. By evaluating enormous volumes of historical and current data to find patterns, trends, and correlations that human analysts would miss, big data improves predictive modeling in the stock market. Better risk management techniques and more informed investing decisions result from this.
Case studies show how big data analysis has been used in the stock market to make effective forecasts. For instance, before it became commonplace, a well-known investment firm used big data analytics to forecast a significant shift in consumer behavior toward digital shopping platforms. They were able to invest in pertinent industries ahead of time because to this information, which helped their clients make large profits.
In a different case study, a tech business uses big data algorithms to examine social media sentiment on particular equities. Through sentiment analysis and discussion monitoring on the internet, they were able to forecast changes in stock values based on what the general public thought. Their investors had a competitive advantage in making prompt buy or sell choices because to this proactive approach.
5. Utilizing Machine Learning and AI for Stock Trading
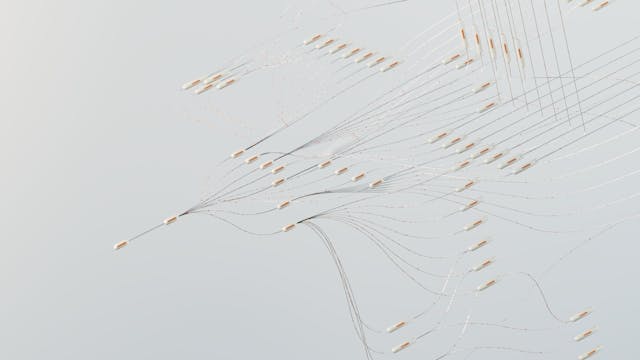
Making sense of large amounts of data to make trading decisions with machine learning and artificial intelligence is essential. These fast data analysis tools spot patterns and trends that human traders would overlook by sifting through enormous volumes of data. Traders can make better selections based on past data, market movements, and even social emotion by employing machine learning algorithms.
There are numerous advantages to employing ML and AI in stock trading. By precisely forecasting market trends, improving portfolio allocations, and automating trade executions, they make improved risk management possible. These tools also lessen human biases that may skew judgment and assist investors in more quickly identifying lucrative prospects. AI-driven algorithms have a competitive advantage over conventional trading tactics because they can instantly adjust to shifting market conditions.
There are obstacles associated with integrating ML and AI into stock trading techniques, though. The "black box" effect, in which these algorithms' decision-making becomes opaque and challenging to understand, is one of the main causes for concern. In the financial sector, concerns about trust and accountability are brought up by this lack of openness. An over-reliance on machine-driven models could result in unanticipated risks like systemic failures or algorithmic errors, which could have a significant impact on the market. Achieving a balance between automation and human supervision is essential for maintaining the stability and efficacy of trading systems driven by artificial intelligence.
6. Regulatory Challenges and Ethical Considerations
When it comes to stock market operations, using big data presents a number of regulatory issues. There are worries that data may be manipulated or misused in ways that could compromise the fairness and stability of the market. The regulatory authorities are confronted with the formidable challenge of keeping up with the swiftly changing technology and tactics utilized by industry players to guarantee adherence to current laws and to properly predict and handle new problems.
When it comes to trading operations driven by big data, ethical concerns are very important. Concerns around privacy violations and the possible exploitation of sensitive information for financial benefit are raised by the vast volume and granularity of data that is readily available. When algorithms analyze massive volumes of data to make split-second trading decisions—sometimes with far-reaching effects on markets and investors—transparency becomes an increasingly important concern. In an increasingly digitalized trade scene, market participants face a critical challenge: balancing the use of big data for competitive advantage with the upholding of ethical standards.
7. Competitive Advantage through Big Data Adoption
Smaller companies are increasingly figuring out how to use big data strategically to provide them a competitive edge over larger companies in today's cutthroat stock market environment. Smaller firms may react swiftly to market changes and seize new possibilities by utilizing the power of data analytics to make more agile and precise informed decisions.
Predictive analytics is one method for using big data to outperform market leaders in stock trading. Traders are able to spot patterns and trends that can point to changes in the market or price movements in the future by examining large amounts of historical and current data. This knowledge enables them to anticipate events more precisely and complete trades more quickly than their rivals.
mood analysis, which entails scouring news articles, social media, and other sources for information on investment behavior and market mood, is another important strategy. Traders can gain a considerable advantage in timing their bets by anticipating market movements ahead of time by analyzing public opinion and emotions surrounding particular stocks or sectors.
Trading methods based on intricate data patterns and signals can be automatically automated with the use of machine learning algorithms. These algorithms can reduce bias and human error in trading decisions by continuously learning from fresh data inputs and making adjustments. This allows traders to take advantage of opportunities in real-time.
Smaller companies in the stock market can compete on an equal footing with larger companies and potentially outperform them in terms of profitability, efficiency, and agility by using big data analytics tools and methodologies. Those who capitalize on the potential of big data have the opportunity to reshape the conventional dynamics of the stock market and establish themselves as strong competitors alongside industry titans in this age of information becoming power.
8. Risks and Limitations of Big Data in Stock Market Trading
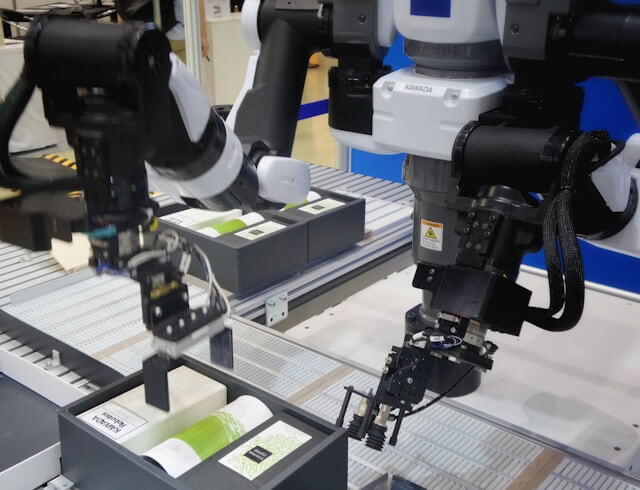
Using big data in stock market trading can be a double-edged sword that presents enormous benefits but also substantial hazards and constraints that investors should take into account. The potential for data breaches to compromise trade tactics and sensitive market information is one significant concern. Errors in the data that is gathered or used can result in poor analysis and poor choices, which can affect the results of investments.
Model biases in big data algorithms can distort outcomes and lead to worse than ideal trading decisions. In order to prevent making decisions based on inaccurate or incomplete information obtained from big data research, investors need to be aware of these biases. Investors looking to properly harness the potential of big data in stock market trading scenarios must be aware of its limitations.
Big data has limitations that investors should be aware of, even while it can provide insightful information about patterns and trends in the market. One drawback is that historical data patterns could be over-relied upon, which might not necessarily be an accurate indicator of how the market would behave in the future. When evaluating big data analytics, investors should proceed with caution and take into account additional aspects that might affect stock values in addition to those revealed by traditional datasets.
If not managed efficiently, the sheer volume of data created can overwhelm investors and cause paralysis in making decisions. It takes advanced analytical techniques and experience to quickly extract useful insights from massive amounts of data. When incorporating big data into trading decisions, investors must be aware of these limits and put strong risk management techniques into place for a well-rounded strategy that reduces inherent risks.
9. Tools and Technologies Empowering Small Traders
Small traders are getting a competitive edge in the current stock market environment by utilizing readily available tools and technology that make use of big data analytics. These developments have challenged the dominance of large players and democratized the capacity to make wise investment decisions. These tools enable tiny traders to make data-driven investing decisions, detect new trends, and evaluate enormous volumes of data in real-time.
Small traders are empowered in their stock market pursuits by a number of crucial tools and technologies. Through interactive charts and graphs, data visualization tools like Tableau and Power BI assist them in extracting insights from large, complex datasets. By automating trades based on predetermined criteria, algorithmic trading software such as QuantConnect or MetaTrader reduces human error and maximizes trade execution speed.
Small traders should think about using cloud-based services like Amazon Web Services (AWS) or Google Cloud Platform (GCP) for scalable storage and analysis of massive datasets as affordable ways to adopt big data tactics. Python and other open-source programming languages, along with libraries like Pandas and NumPy, offer a robust framework for effectively processing and analyzing financial data. At a reasonable cost, financial professionals can enroll in big data analytics courses from online learning platforms like Coursera or Udemy.
Small traders can level the playing field in the stock market by adopting these tools and technologies and using big data analytics to make well-informed judgments that are competitive with those made by larger institutional players.
10. Future Trends: Big Data's Evolution in Stock Trading
Big data has the potential to completely transform stock trading in ways that were previously unthinkable in the years to come. One development that will influence how big data is integrated into stock market operations in the future is the growing usage of AI and machine learning algorithms for fast and effective analysis of large amounts of data. By revealing hidden patterns and trends, these technologies give traders important information they need to make wise selections.
We can expect substantial technological breakthroughs that will keep influencing the dynamics of trade. For example, real-time data processing combined with predictive analytics will be used more often, allowing investors to respond quickly to changes in the market. The stock market ecosystem may benefit greatly from the use of blockchain technology in terms of increased security and transparency.
Big data's development in the stock market has the ability to level the playing field for all players by empowering institutional and individual investors to make more informed investment decisions based on thorough data analysis. As these fascinating developments at the nexus of technology and finance emerge, keep an eye out.
11. Case Studies: Successful Applications of Big Data in Stock Trading
Case Studies: Successful Applications of Big Data in Stock Trading
1. **Renaissance Technologies**: Renowned for its groundbreaking application of big data analytics in stock trading, Renaissance Technologies was founded by mathematician and former codebreaker James Simons. The organization's Medallion Fund has continuously beaten the market by utilizing intricate models and advanced algorithms that examine enormous volumes of financial data.
2. **BlackRock**: To improve its investing methods, BlackRock, the biggest asset manager in the world, has embraced big data. BlackRock gains a competitive edge in the market by using vast datasets to influence risk management and stock selection decisions through its Aladdin platform.
3. **QuantConnect**: A platform that enables individual traders and developers to use big data to create and test algorithmic trading methods is called QuantConnect. QuantConnect democratizes quantitative finance by providing strong computing resources and access to large financial datasets, making institutional players more competitive for regular investors.
4. **Two Sigma Investments**: Another hedge fund that has benefited from big data analytics to make effective trading selections is Two Sigma Investments. Two Sigma uses state-of-the-art technology in conjunction with a team of mathematicians and data scientists to analyze massive amounts of market data and find hidden patterns that guide their investment strategies.
5. **Robinhood**: With its user-friendly interfaces and zero-commission trades, Robinhood has completely changed stock trading for retail investors, upending the established brokerage sector. Through the use of big data analytics, Robinhood is able to better understand the behavior and preferences of its users, which allows them to customize investment suggestions and offer a smooth trading experience.
These case studies show how big data is changing the stock trading industry by empowering businesses and individuals to use enormous volumes of data for improved risk management, better decision-making, and more market profitability.
12. Conclusion
This blog post examined how big data is revolutionizing the stock market sector. We talked about how investors might benefit from big data analytics by making better decisions and possibly outperforming more established competitors in the market. Individual investors and smaller enterprises can now compete on an even playing field with larger institutional players by utilizing machine learning algorithms, large datasets, and real-time information. Rapid data analysis has the potential to completely redefine success in the stock market and transform investment methods.
We talked about how big data techniques, such as sentiment analysis, alternative data sources, and predictive analytics, are changing the way the stock market industry does things. With the use of these technologies, one can now measure market sentiment, spot new trends, and more accurately predict price fluctuations. Investors that use big data to their advantage are therefore in a better position to recognize profitable possibilities, adjust to shifting market conditions, and efficiently manage risks.
To put it simply, big data is a game-changer in the investment industry, not just a trendy term. Its ability to democratize information access and level the playing field for all stock market players is what gives it its transformational potential. Those who adopt big data analytics stand to acquire a competitive edge that could upend existing conventions and threaten well-established competitors in the stock market industry as we move forward into this era of unparalleled data dissemination. Those that can effectively harness the potential of big data and use it to spur innovation, streamline decision-making procedures, and produce better investment results will be the ones in the future.