1. **Introduction**
First of all, Healthcare is just one of the areas that big data has transformed. Medical science generates enormous amounts of data, which have enabled ground-breaking improvements in patient care, illness management, and treatment outcomes. Predicting cardiac disease, a major global cause of death, is one important use of big data in healthcare. Researchers and medical practitioners may now more accurately predict, diagnose, and even prevent cardiac diseases than ever before by utilizing massive datasets and powerful analytics. In this blog article, we explore how big data is revolutionizing the treatment of cardiovascular health and playing a critical role in heart disease prediction.
2. **Understanding Heart Disease**
Heart disease is a general phrase used to describe a variety of heart-related illnesses. The most prevalent kinds include congenital heart abnormalities, which are present from birth, arrhythmias, which are irregular heart rhythms, coronary artery disease, which is characterized by blocked or restricted arteries leading to the heart, and heart failure. It is important to correctly diagnose and treat these illnesses because they might have a variety of causes and symptoms.
Heart disease is a major global health concern. Over 17 million fatalities occur from it each year, making it the world's largest cause of mortality, according to the World Health Organization (WHO). One in every four deaths in the United States alone is attributed to heart disease, which claims the lives of about 655,000 people annually. These figures highlight how urgent it is to create efficient plans for this widespread health issue's prevention, early detection, and treatment. Researchers and medical practitioners can now better detect and treat heart disease than ever before by utilizing big data analytics to provide insightful information.
3. **Challenges in Predicting Heart Disease**
Accurately predicting cardiac disease presents a number of difficulties. The sheer amount and complexity of data required to evaluate cardiovascular health is a significant challenge. Conventional risk assessment techniques frequently depend on incomplete data, including blood pressure, cholesterol, age, gender, and smoking status. These elements by themselves might not give a complete picture of a person's total risk of heart disease.
Because many risk variables interact in a complex way, it might be difficult to predict heart disease without the use of big data analytics. Human biology is extremely complicated, and a person's susceptibility to cardiovascular problems can be influenced by a variety of genetic, lifestyle, environmental, and socioeconomic factors. Healthcare professionals can find it difficult to determine who is most likely to develop heart disease if they don't use advanced analytics to sort through enormous volumes of data and find hidden patterns or correlations.
The dynamic nature of health data is another major challenge in the prediction of cardiac disease. An individual's cardiovascular health outcomes can be impacted by a variety of factors that might vary over time, including medication adherence, stress levels, exercise habits, and food. It becomes difficult to estimate a person's risk of heart disease in a timely and accurate manner if these changing variables are not continuously monitored and analyzed utilizing big data methods.
The difficulties in precisely forecasting cardiac disease highlight how crucial it is for the healthcare industry to use big data analytics. Early detection of heart disease in high-risk persons can be improved by medical professionals by using new technologies to process large volumes of different data sources and reveal hidden insights. Big data analytics integration with cardiovascular health management has great potential to enhance preventive treatment approaches and lessen the toll that heart-related illnesses have on both patients and healthcare systems.💽
4. **Role of Big Data in Healthcare**
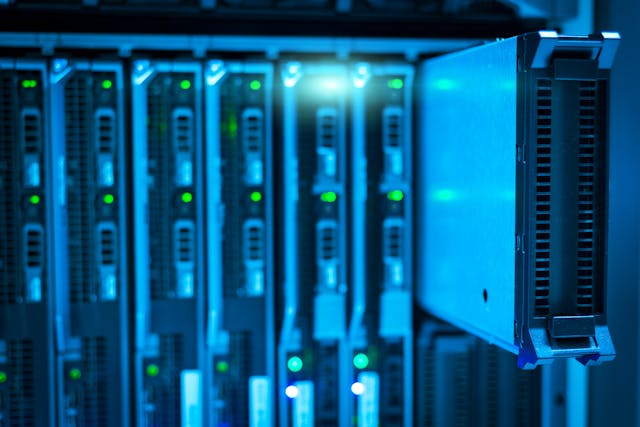
Big data is fundamentally changing the healthcare sector, especially in terms of heart disease prevention and prognosis. Healthcare professionals can find trends and risk factors linked to heart disease by evaluating enormous volumes of patient data, including genetic data, lifestyle choices, medical records, and more. This makes it possible for them to anticipate any problems before they materialize and take proactive measures to enhance patient outcomes.
Big data has had a substantial impact on healthcare in a number of other areas outside heart disease. Big data analytics are assisting researchers in the field of cancer research by revealing novel ideas for therapies and customized care. Big data is a key component of precision medicine, which uses it to customize treatments for each patient based on their specific genetic composition and other variables. Big data is essential for detecting disease outbreaks, keeping an eye on population health trends, streamlining hospital operations, and using predictive analytics to improve patient care. Big data technology integration is changing healthcare delivery by giving doctors insightful knowledge that helps them make better decisions and, ultimately, improves patient outcomes.
5. **Big Data Analytics in Predicting Heart Disease**
By evaluating enormous volumes of data to find patterns and trends that can point to possible hazards or early warning indicators of cardiovascular problems, big data analytics play a critical role in the prediction of heart disease. Healthcare practitioners can sort through large datasets containing patient medical records, genetic data, lifestyle factors, and more using sophisticated algorithms and machine learning techniques to create predictive models for determining a person's risk of developing heart disease.
Using big data in this situation has several advantages, one of which is that it makes early detection and prevention techniques possible. Healthcare professionals can proactively identify people at high risk of heart disease before symptoms appear by utilizing real-time monitoring information and historical patient data. This enables healthcare teams to implement tailored treatments like medication management, lifestyle adjustments, or focused screenings, thereby enhancing patient outcomes and lessening the strain on the healthcare system.🖲
Big data analytics, in short, transforms the way that heart disease is predicted by providing a thorough knowledge of risk factors and prediction markers that may be missed by conventional diagnostic techniques. Big data technology integration improves risk assessment accuracy while also providing healthcare practitioners with useful information to enable proactive, individualized care that may have a long-term, substantial influence on patients' cardiovascular health.
6. **Applications and Tools**
Many tools and technologies are used to efficiently analyze big data in the field of heart disease prediction. Large datasets must be processed in order to find patterns and connections pertaining to heart health. Machine learning methods like logistic regression, decision trees, random forests, and neural networks are essential for this process.
The enormous volumes of health data that are available are mined for hidden insights using data mining techniques like association rules and clustering. These techniques aid in organizing data to improve comprehension and forecast a person's possible risk of developing heart disease.🔶
Apache Spark, an open-source cluster computing platform that offers speed and user-friendliness for big data processing jobs, is one important and often used technology. It is the method of choice for evaluating large-scale healthcare datasets pertaining to cardiac diseases because of its speedy handling of large-scale data processing.
Examples from everyday life demonstrate how these approaches have been effectively applied to the prediction of heart disease. In one study, for example, researchers at a well-known medical center used machine learning algorithms on a dataset made up of electronic health information belonging to patients. The examination identified important markers and cardiovascular disease risk factors, allowing medical professionals to take early preventative action.
An further instance highlights the application of data mining methods in a community health program targeted at lowering the incidence of heart disease. Patterns identifying high-risk groups were found by examining lifestyle habits and demographic data gathered from wearable devices. This resulted in the development of specialized therapies and educational initiatives aimed at reducing the community's exposure to heart disease risk factors.
These applications show the real-world advantages of using cutting-edge instruments and technologies to efficiently use big data for heart disease prediction and prevention. Researchers and healthcare practitioners can improve outcomes for patients at risk of cardiovascular problems and increase their prognostic abilities through ongoing developments and advancements in data analysis approaches.
7. **Benefits for Patients and Healthcare Providers**
When it comes to heart disease prediction, big data analytics predictions are very beneficial to patients and healthcare providers alike. These prognoses can change a patient's life by allowing for early detection and aggressive treatment. Medical experts can give individualized care plans to decrease risks and avoid possible health crises by identifying individuals who are at high risk of acquiring heart disease. With the help of this predictive analysis, patients may take control of their health and improve their quality of life by making educated decisions based on their unique risk factors.
Conversely, big data predictions have the potential to significantly benefit healthcare practitioners by optimizing the delivery of heart disease care. Healthcare professionals can maximize resource allocation within healthcare systems, personalize therapies to each patient's needs, and speed diagnostic processes by utilizing advanced analytics and machine learning algorithms on massive datasets. This improves patient outcomes and lowers overall healthcare costs by allowing medical practitioners to provide more targeted and efficient interventions. It also increases the efficiency of care.
To conclude my previous writing, applying big data analytics to the prediction of heart disease not only transforms our understanding of cardiovascular health but also has real benefits for patients and healthcare professionals. We are better able to address this widespread health issue proactively and successfully by utilizing the predictive insights obtained from massive volumes of data, opening the door to a healthier future for everybody.
8. **Accuracy and Reliability**
When it comes to big data analytics for heart disease prediction, accuracy and dependability are critical concerns. For healthcare interventions to be effective, these forecasts must be credible. Severe steps are taken to guarantee that the outcomes are reliable in order to allay these worries.
Improving data quality is essential for raising the prediction models' accuracy and dependability. Accurate forecasts are enhanced by robust datasets that include a wide variety of patient data. To preserve data integrity and raise the dependability of results, extensive data cleaning procedures, validation tests, and frequent updates are crucial.
Utilizing cutting-edge algorithms and analytics methods can improve big data models' capacity for prediction. Large datasets can contain complex patterns that can be found using machine learning methods like random forests, support vector machines, and neural networks. This can lead to predictions that are more accurate. Over time, these algorithms' reliability is increased by ongoing validation and improvement.
Strict testing procedures and validation studies are essential for guaranteeing the reliability of predictive models created using big data analytics. Researchers can evaluate prediction accuracy and optimize algorithms to increase their dependability in clinical contexts by comparing model predictions against real-world outcomes and carrying out thorough validation testing.
Working together, researchers, data scientists, and medical professionals may confirm the validity and accuracy of predictive models. Through the utilization of interdisciplinary skills, integration of domain knowledge, and peer reviews, stakeholders can collaborate to improve predictive models grounded in big data analytics and guarantee their congruence with clinical realities.
9. **Ethical Considerations**
The utilization of big data to forecast cardiac disease is contingent upon ethical considerations. As researchers and healthcare providers use massive patient data sets to enhance predictive analytics, privacy, consent, and data security issues become more apparent. The necessity to strike a balance between safeguarding people's private health information and the possible advantages of developing prediction models is what gives rise to the ethical difficulties.
Concerns concerning the storage, accessibility, and sharing of sensitive patient data are brought up by the collection of such data for predictive analytics. Establishing precise policies for data governance and transparency is crucial to guaranteeing that patients' right to privacy is upheld at every stage of the procedure. Getting people's informed consent before using their data is essential to maintaining moral principles and building confidence in the medical community.😎
As big data technologies continue to change healthcare, privacy concerns remain a major concern. When using big data to forecast cardiac disease, protecting patient privacy and limiting unwanted access to private medical records are essential factors to take into account. Strong security measures, like access limits and encryption procedures, can help reduce the risks of data misuse or breaches.
Predictive analytics using big data raises ethical concerns that call for a multifaceted strategy that puts patient autonomy, privacy, and trust first. The use of strict security measures, respect for individuals' right to privacy, and promotion of transparency in data practices might help healthcare stakeholders effectively traverse the ethical issues that arise when utilizing large datasets to improve cardiovascular risk prediction models. In an era powered by big data technology, ethical considerations must be woven into every step of the predictive analytics process to promote responsible innovation and safeguard patient welfare.ðŸ¤
10. **Future Trends**
Promising developments are anticipated in the use of big data to forecast cardiac disease in the future. Predictive models should become much more accurate and efficient as artificial intelligence (AI) and machine learning technologies advance quickly. These cutting-edge tools have the ability to completely change how medical practitioners examine enormous volumes of data to spot trends and anticipate possible heart-related problems.
The combination of wearable technology and sensors to continuously track a person's activities and vital signs is one trend for the future. Early identification of cardiovascular issues is made possible by this continuous stream of real-time data, which can offer insightful information about an individual's health. Through the integration of wearable technology data with big data analytics, healthcare providers can customize preventative care plans to meet the specific needs of each individual patient.
Heart disease prediction is one area where big data analytics holds significant potential for the advancement of precision medicine. Researchers can develop highly individualized risk assessment models by utilizing lifestyle characteristics, medical history, genetic information, and environmental data. By identifying those who are more likely to acquire heart disease early on, these models can help prevent or slow the progression of cardiovascular problems.
Future developments in machine learning algorithms will improve predictive power because huge data will always be essential in forecasting cardiac disease. Artificial intelligence (AI)-powered predictive models are more effective than conventional techniques at sorting through large datasets and spotting minute connections and patterns that could otherwise be missed. Thanks to this fine-tuning of predictive analytics, medical providers will be able to estimate an individual's risk of heart disease more accurately by analyzing a wide range of characteristics.
In summary, there are a lot of opportunities for innovation and better patient outcomes in the use of big data to forecast cardiac disease in the future. The way that wearable technology, artificial intelligence, machine learning, big data analytics, and precision medicine work together is going to change the way that cardiovascular health is managed. Healthcare practitioners can work toward proactive preventive and individualized care strategies that have the potential to completely change how we approach the diagnosis and treatment of heart disease by skillfully utilizing these cutting-edge technology.
11. **Case Studies**
To comprehend the practical implications of big data in cardiac disease prediction, case studies are essential. One such noteworthy case study centers on a massive research endeavor that gathered millions of people's anonymized health data. Through the use of sophisticated analytics to this extensive dataset, scientists were able to create predictive models for the early identification of heart disease risk factors.
Comparing this study's results to more conventional approaches, they showed a noteworthy improvement in heart disease prediction. By examining multiple factors such genetic susceptibility, way of life, and medical background, the prediction algorithm was able to precisely identify people who were at a high risk of heart problems years before symptoms appeared. Preventive actions could then be put into action quickly, improving health outcomes and lowering medical expenses.🔖
A partnership between data scientists and healthcare professionals to apply machine learning algorithms for heart disease prediction is the subject of another interesting case study. Through the use of a variety of datasets, including patient demographics, clinical records, and diagnostic test results, these algorithms were trained to produce prediction models that were highly accurate in identifying patterns suggestive of cardiovascular risk factors.
When compared to traditional risk assessment methodologies, the initiative's results showed a noteworthy decrease in false positives and false negatives. Patients identified by the prediction model as high-risk were given individualized therapies based on their particular profile, which led to prompt risk factor management and better overall outcomes for cardiovascular health. These success stories highlight how big data has the ability to completely change how heart disease is predicted and prevented.
12. **Conclusion**
Furthermore, as I mentioned previously, using big data to forecast cardiac disease has improved early detection and individualized treatment strategies in a promising way. Machine learning algorithms have the ability to recognize trends and provide more precise predictions about potential dangers by employing large datasets connected to health. Better patient outcomes result from healthcare providers intervening earlier thanks to this proactive strategy. 📗
There will likely be even more advancements in the use of big data to anticipate cardiac disease in the future. We can anticipate more accuracy in risk assessment models and treatment suggestions with continued study and technology advancements. The amalgamation of genetic data, wearable technology, and real-time monitoring has the potential to provide a more all-encompassing comprehension of personal health profiles, hence facilitating customized preventive interventions.
Big data analytics will probably play a bigger part in predictive medicine as it develops, completely changing how we manage cardiovascular health. We are getting closer to a time when heart disease can be more accurately predicted and prevented, which will ultimately result in healthier lives for people all over the world, by utilizing the power of data-driven insights.